October 9, 2019
How Digital Transformation Enables Big Data Analytics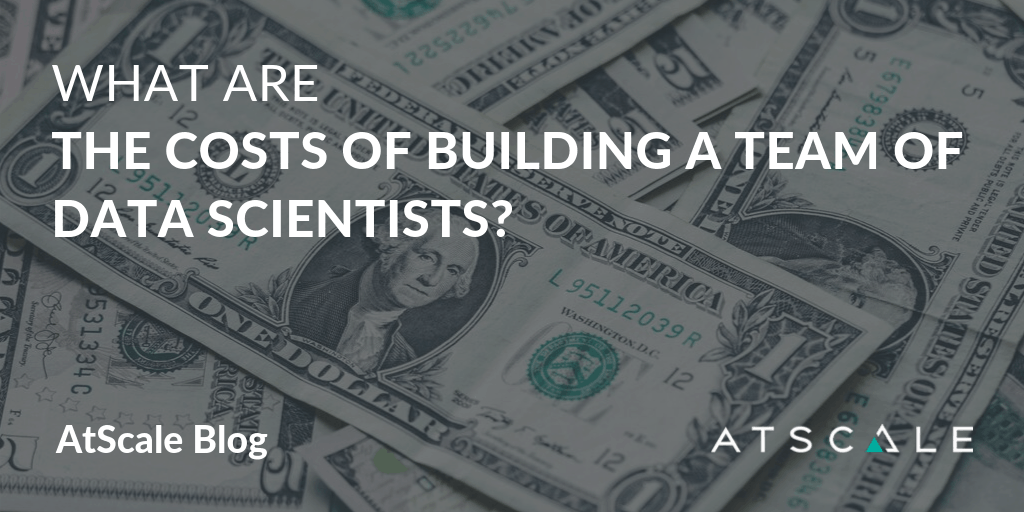
What does it take build and support a team of data scientists?
Conducting exploratory data analysis or even basic business intelligence on Hadoop often requires input from data scientists who:
- Create models for related information across Hadoop.
- Structure databases to contain that data.
- Ensure different BI tools generate consistent results when referencing identical data elements.
How many data scientists you’ll have to hire depends on the size of your organization and the composition of the data under its control. In this post we review how much it typically costs to hire a data scientist, the factors you’ll have to consider when assembling a team and ways you can alleviate the workload placed on data scientists.
“The average US-based data scientist earns $113,436 annually.”
The supply and demand of data scientists
According to Glassdoor, the average salary for a data scientist working in the U.S. is $113,436. What’s behind this price tag? Low supply, high demand and the skill sets associated with the role.
McKinsey Global Institute conducted a study on how the Big Data economy is impacting demand for people who can formulate data models, aggregates and other dimensions to support continuous analysis. Despite the rise of data science degrees, open online courses, bootcamps and certificates, McKinsey predicted the U.S. would experience a shortage of 250,000 data scientists by 2024.
Also, being a data scientist isn’t an easy job. Mary Kypreos and Lindsey Thorne, who both work as HR managers at Greythorn’s open source and big data practice, wrote that many companies seeking data scientists desire candidates who can apply statistical reasoning in engineering roles. Others ask for PhDs in anything from mathematics to computational linguistics.
Considerations for building a data science team
There isn’t what you would call an “average price tag” for a team of data scientists because expertise needs vary among organizations. What about those with massive Hadoop clusters and multiple data analysis tools?
In a situation such as this, you would at least need someone who’s familiar with building data models across large sets of information. According to Data Science Central’s Maloy Manna, the primary role of this data scientist would be to figure out which algorithms would enable analysts to derive insight from a particular data set.
“You may need to hire as many as 20 data scientists to support ongoing data analysis projects.”
In addition, you’ll need someone who’s capable of managing the Hadoop infrastructure, has a working knowledge of SQL, and can configure relational databases, data marts and analysis environments to run the models his or her associate developed.
You may need to hire as many as 20 data scientists to support ongoing data analysis projects. For example, a pharmaceutical company with global operations not only has to analyze drug sales data, but also information regarding R&D, the supply chain, advertising, manufacturing and dozens of other business components.
Reducing the data science workload
Decreasing the labor costs associated with data scientists entails utilizing Big Data intelligence platforms that apply data aggregates to information within Hadoop clusters.
This technology applies the models data scientists develop directly to Hadoop clusters. As data scientists develop new queries to accommodate analysts’ needs, the Big Data intelligence platform uses machine learning algorithms to learn which aggregates particular users employ the most.
Essentially, platforms capable of executing BI on Big Data prevent data scientists from having to continuously develop aggregates from scratch.
Check out our recent post on ‘Solving the Unrelated Dimension Dilemma to learn more about how Big Intelligence Platforms can help you make the world of Analytics on Big Data less of a complex burden on data scientists, and more accessible and empowering to data analysts.
NEW BOOK