A Semantic Layer for:
Trusted, Consistent Metrics
Cloud Cost Management & Performance
Business Context for Text-to-SQL Gen AI
The first and most complete universal semantic layer platform
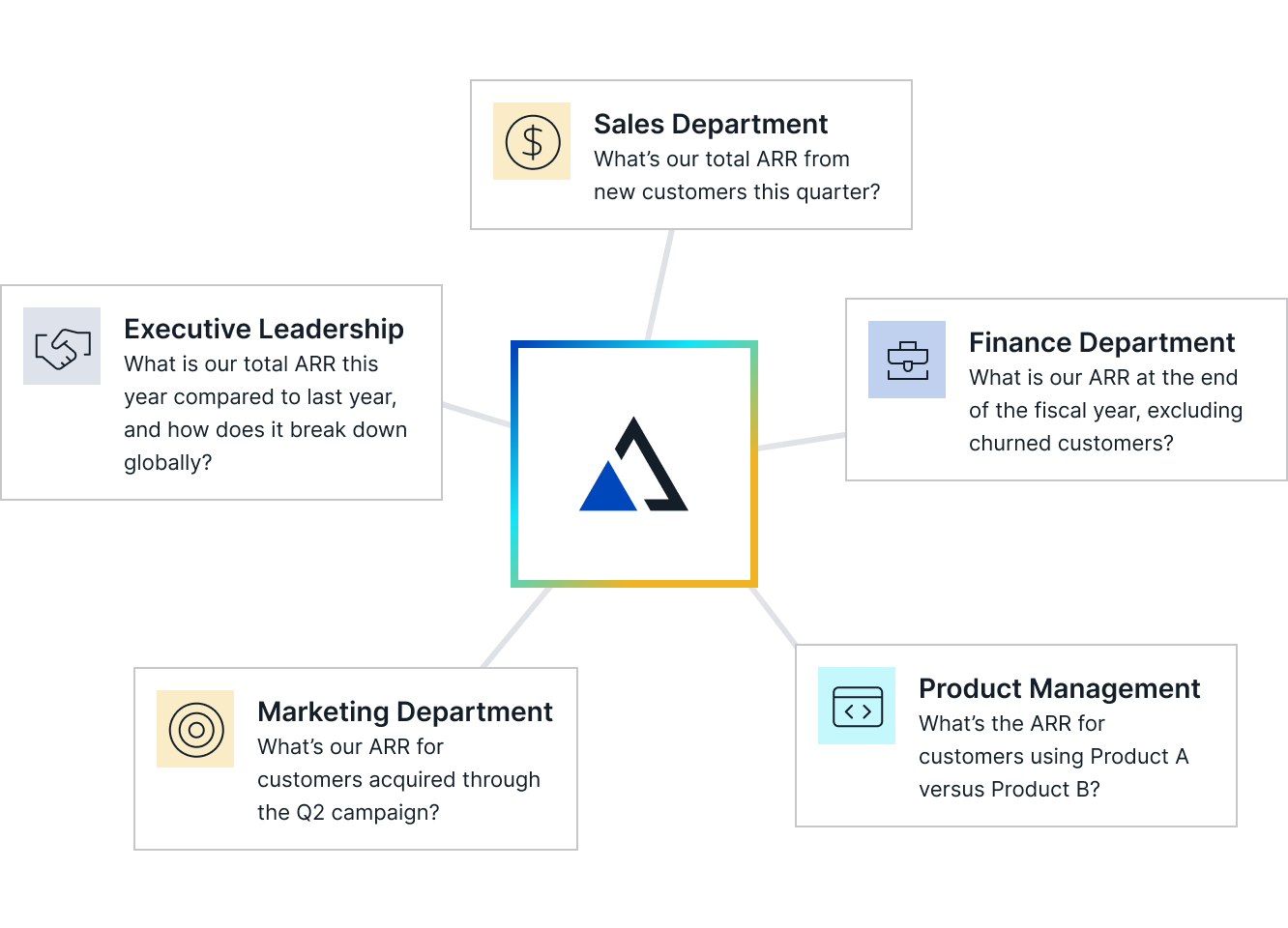
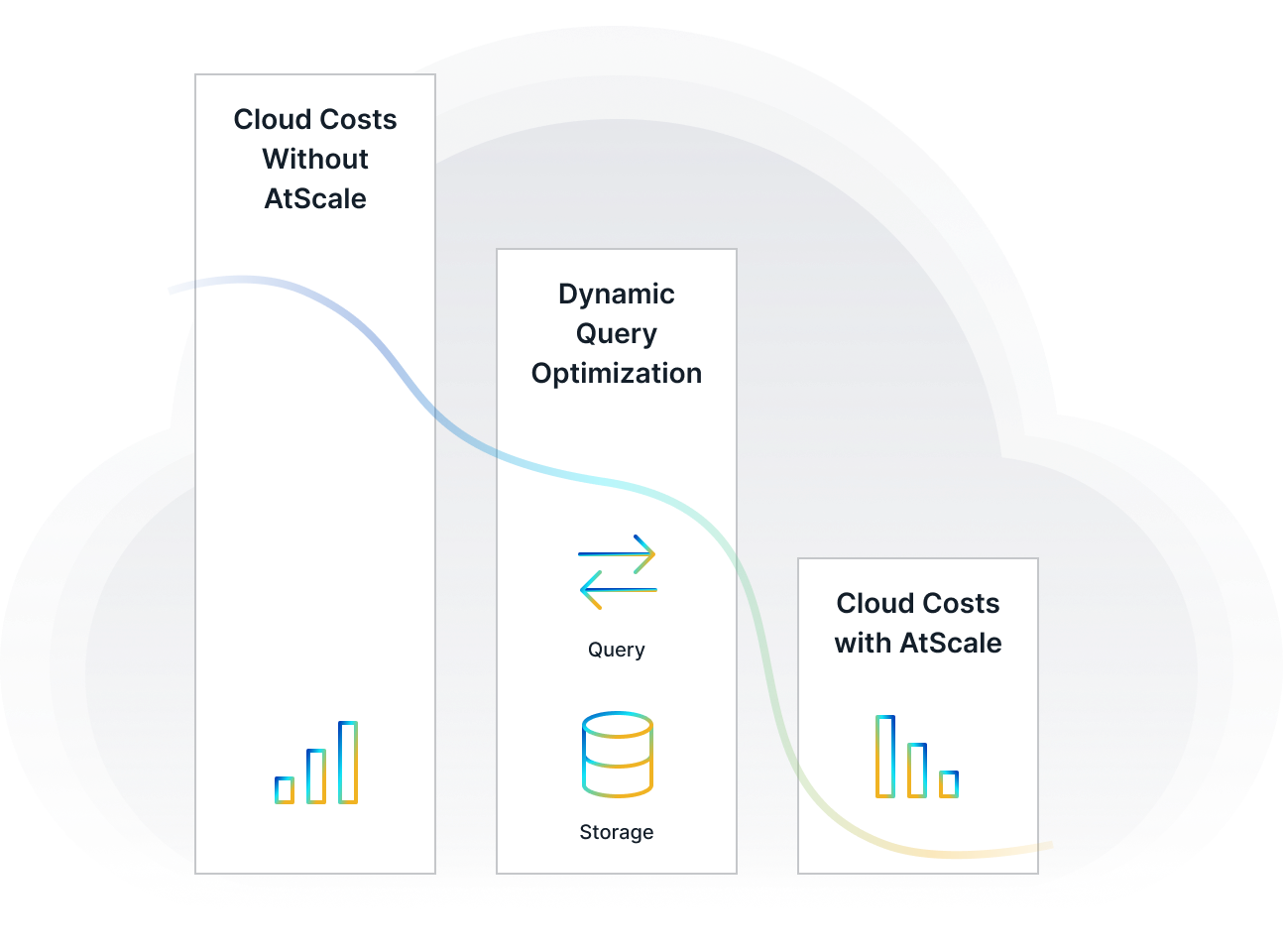
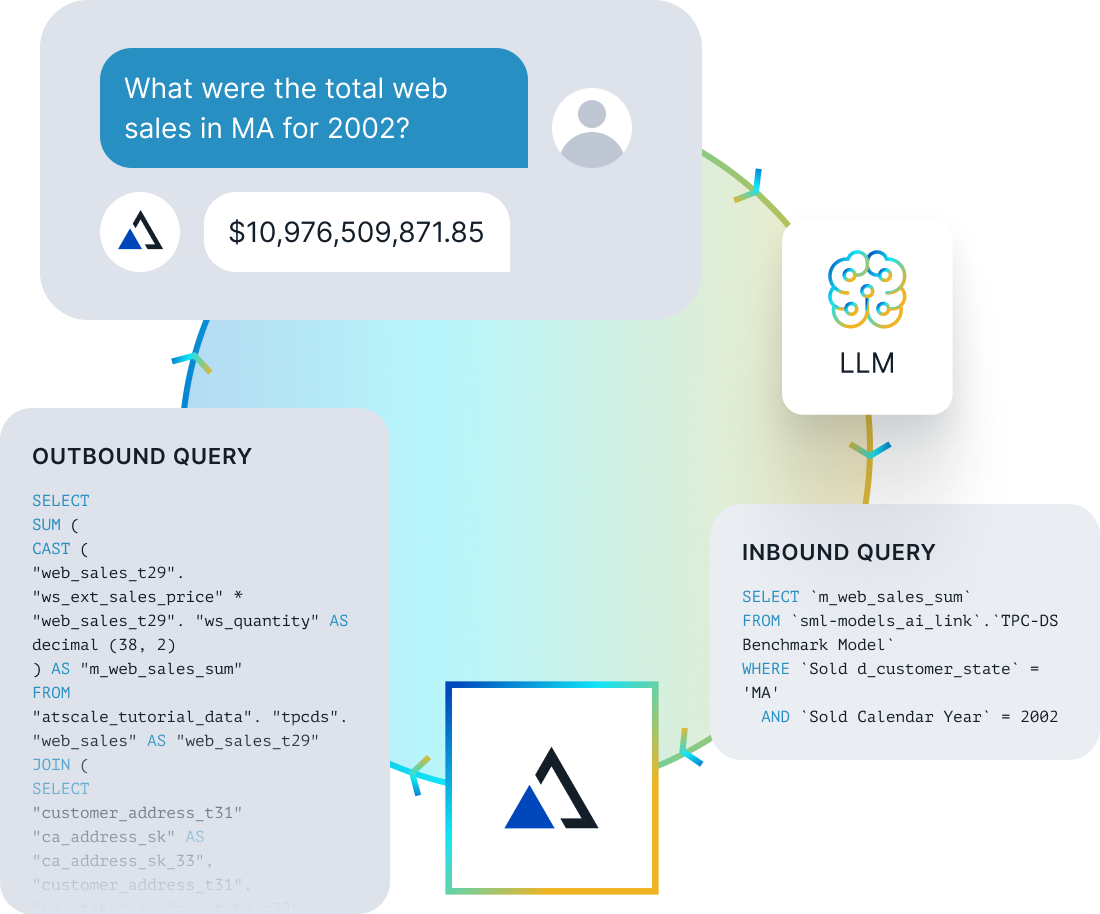
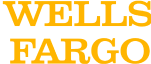

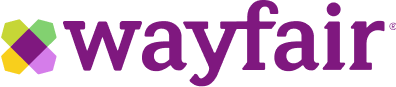
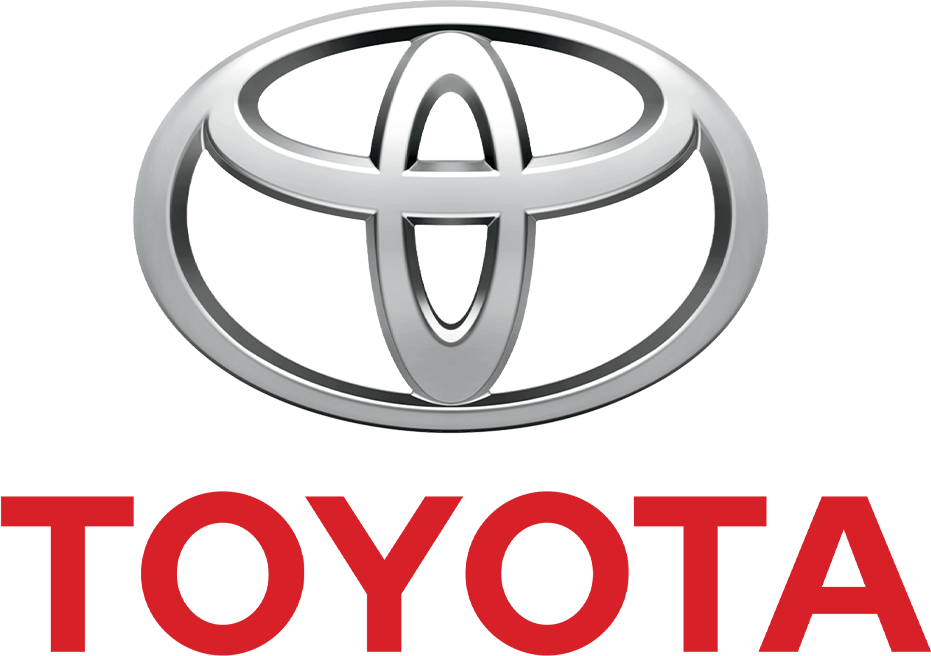
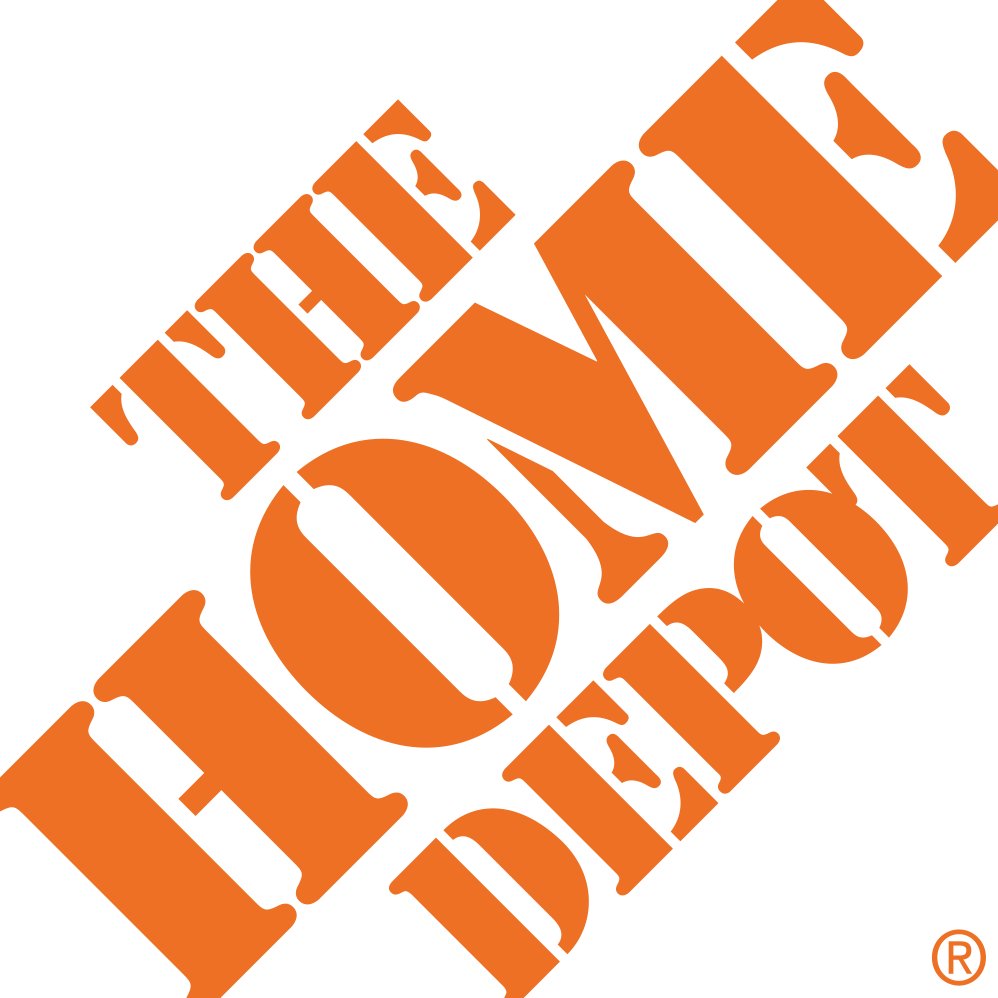
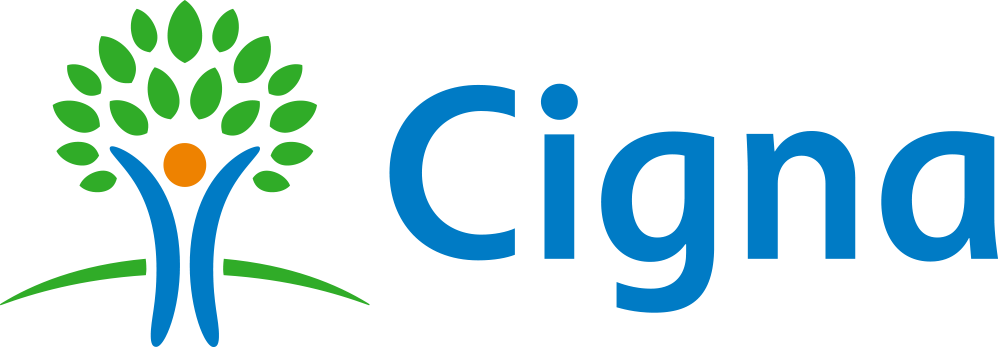
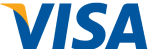
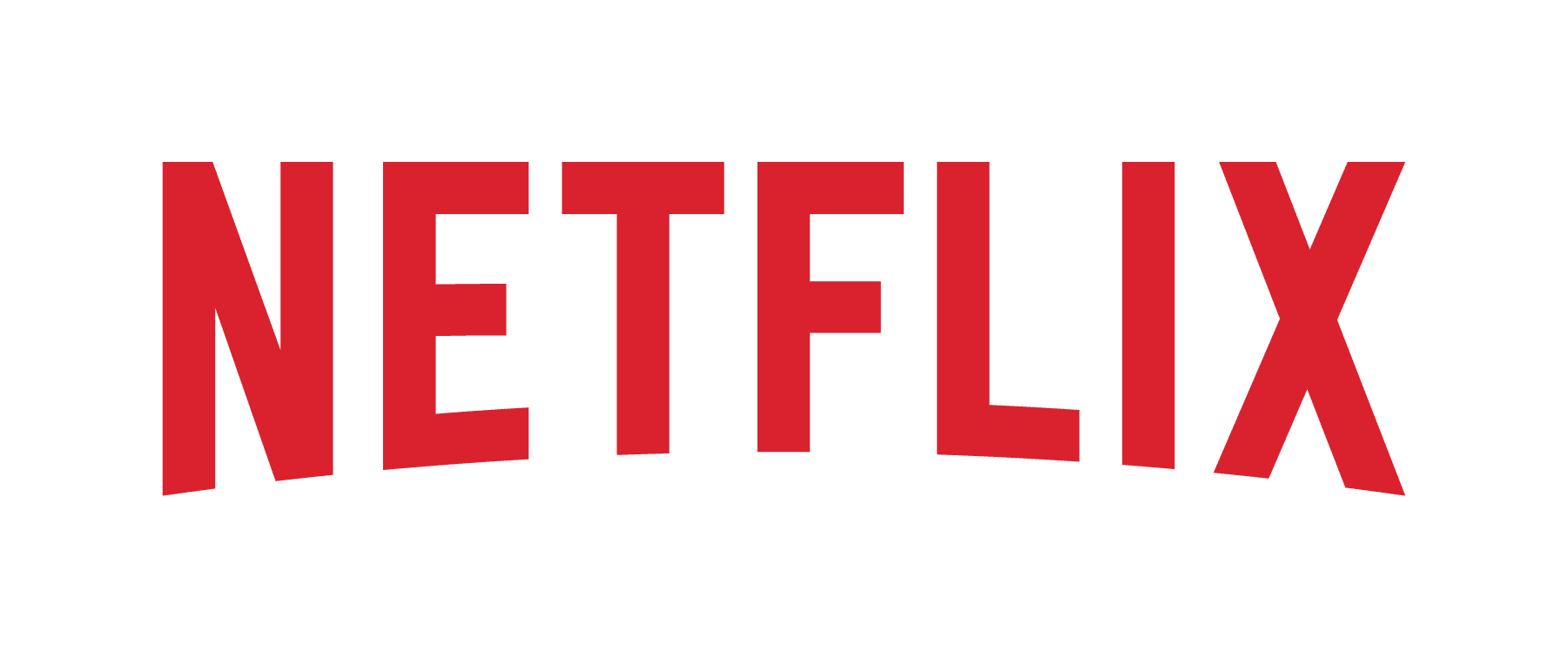
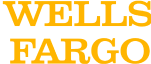

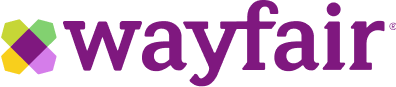
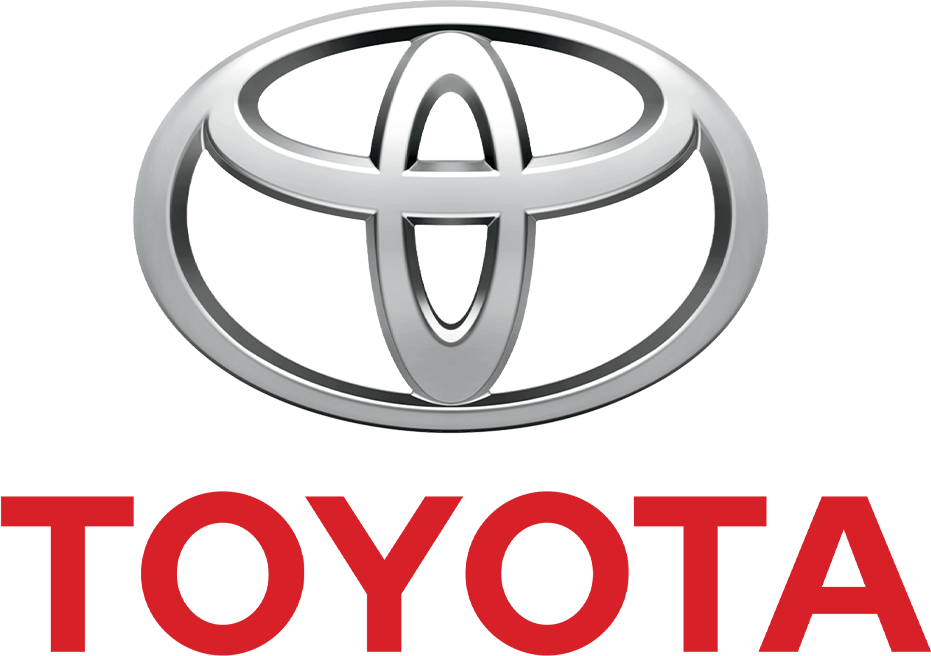
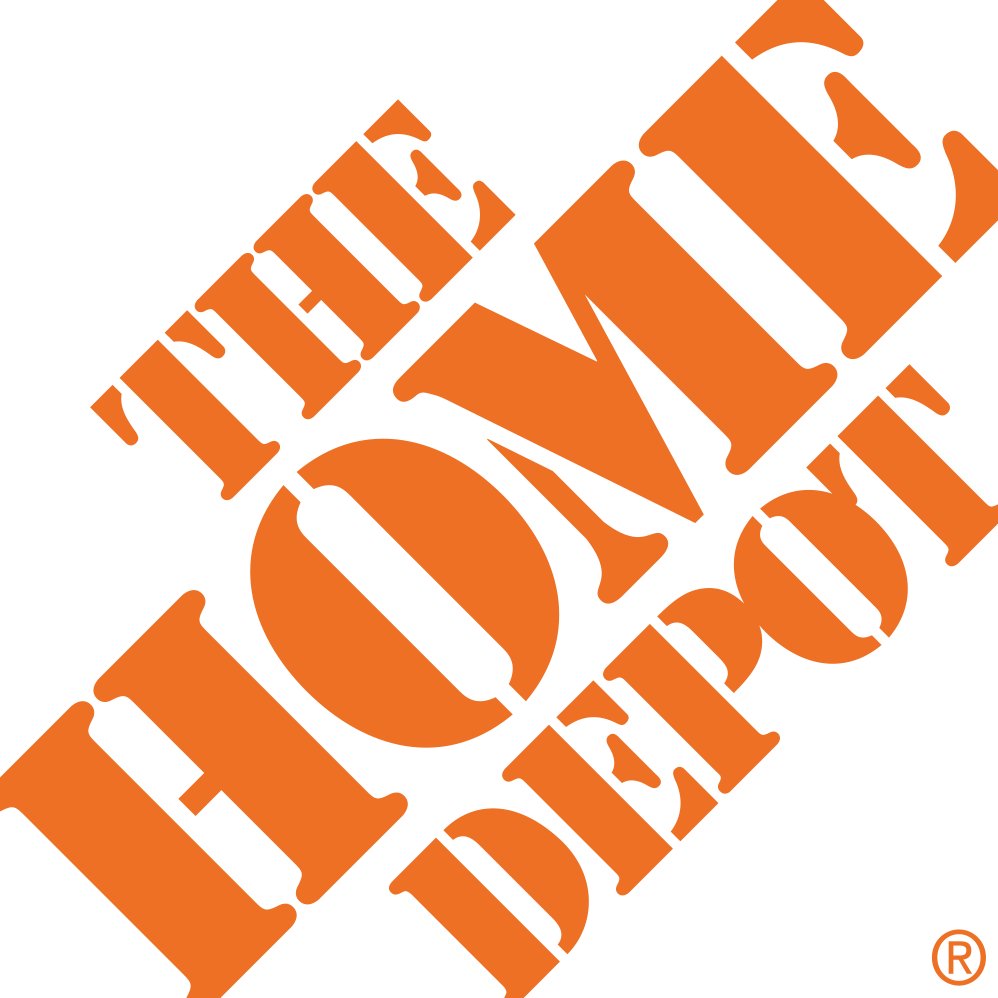
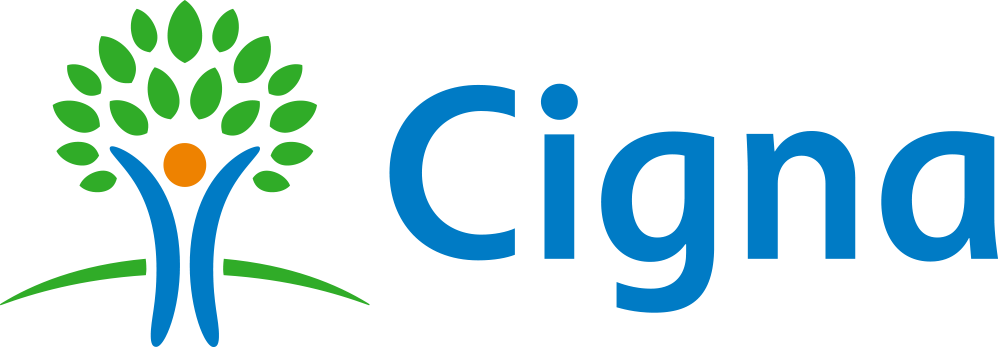
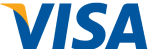
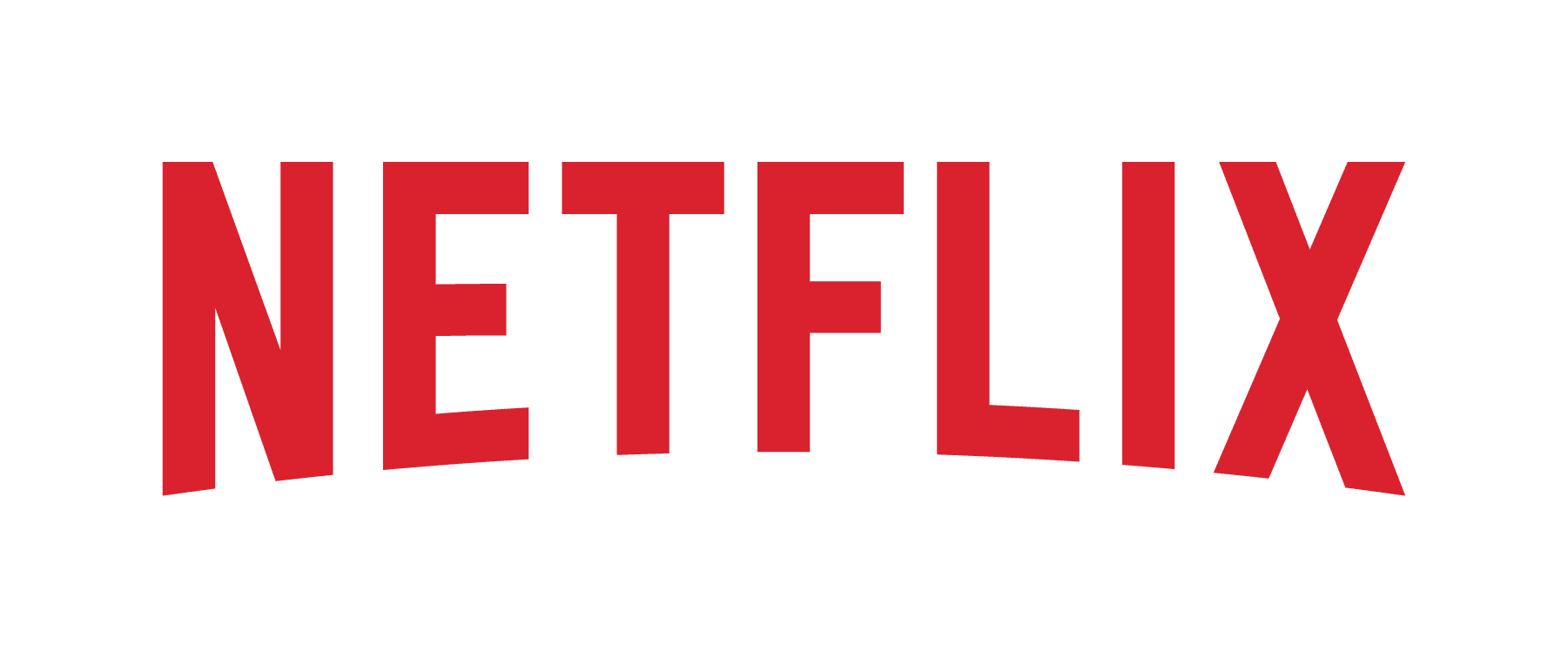
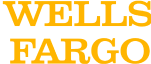

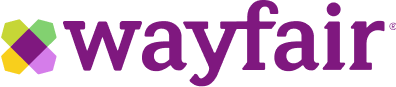
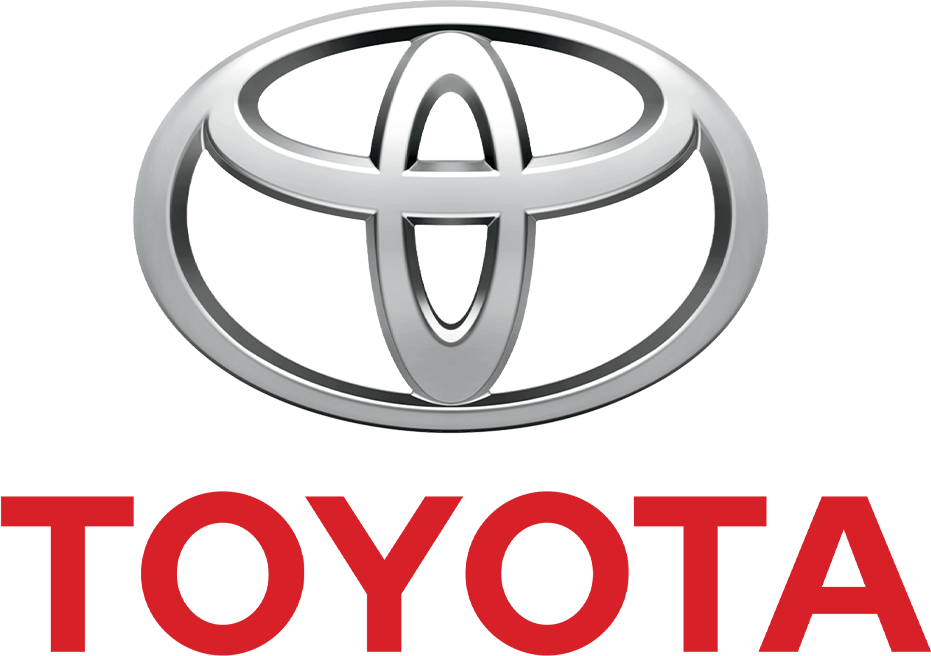
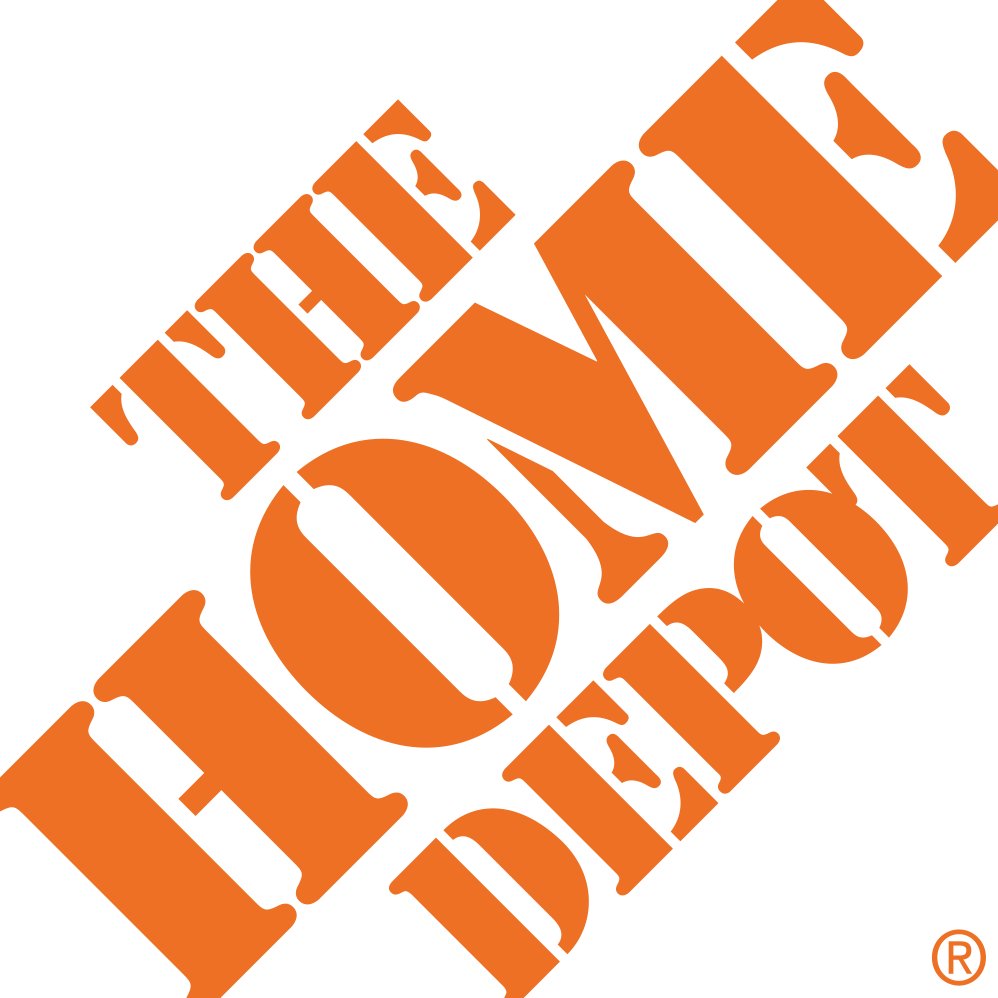
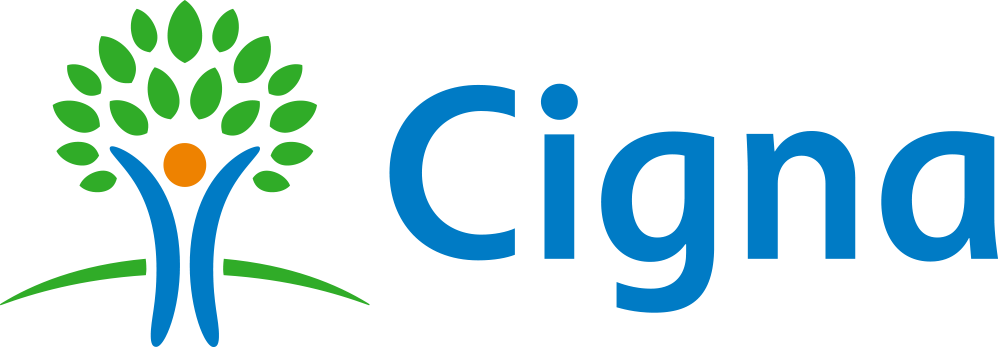
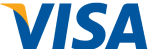
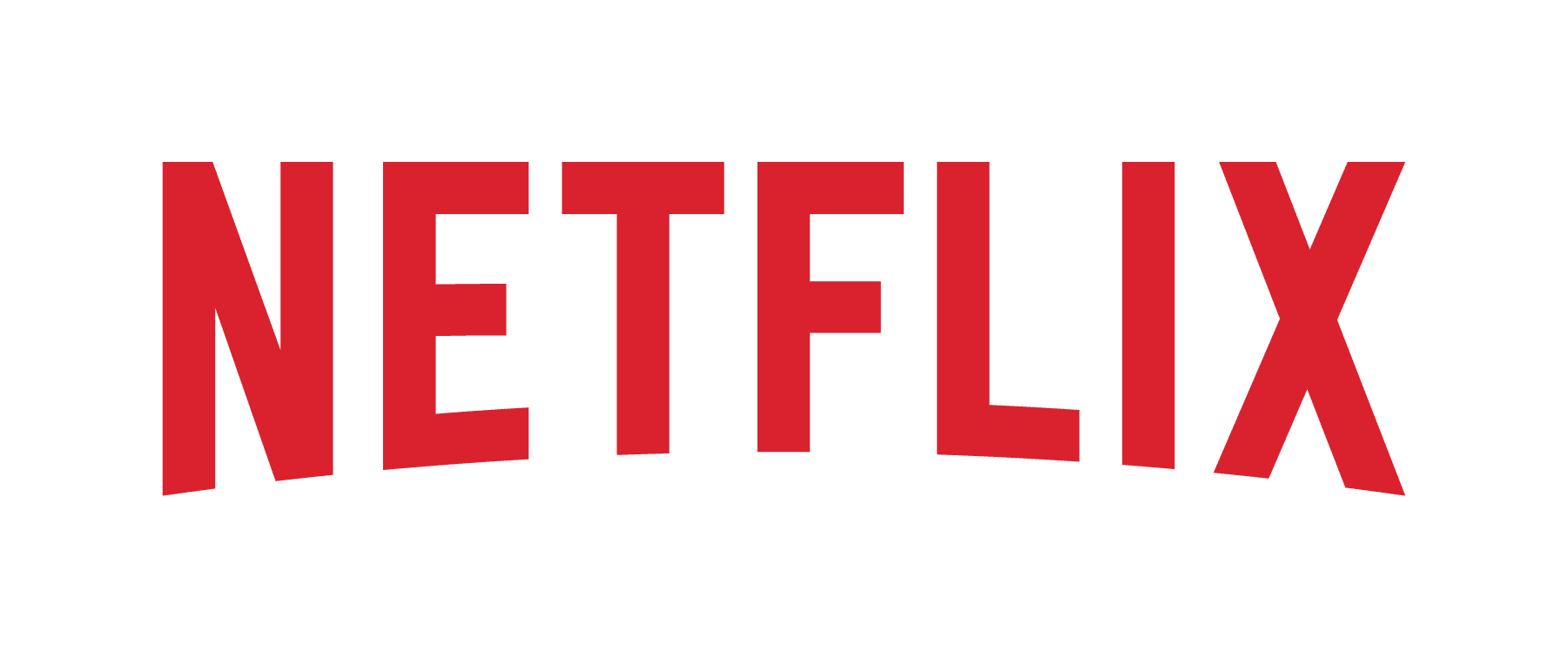
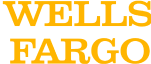

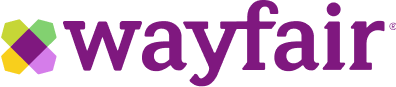
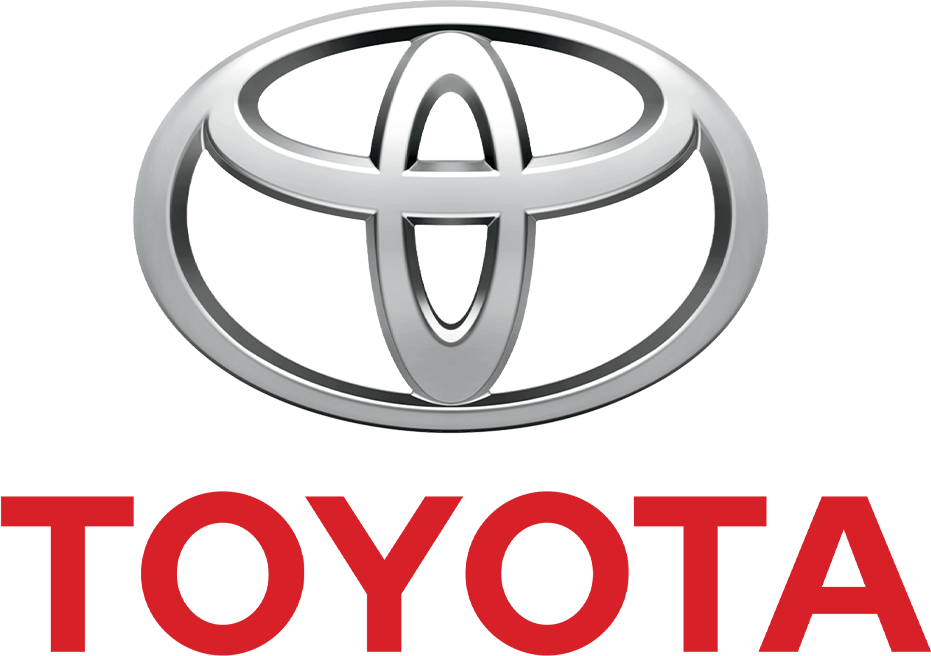
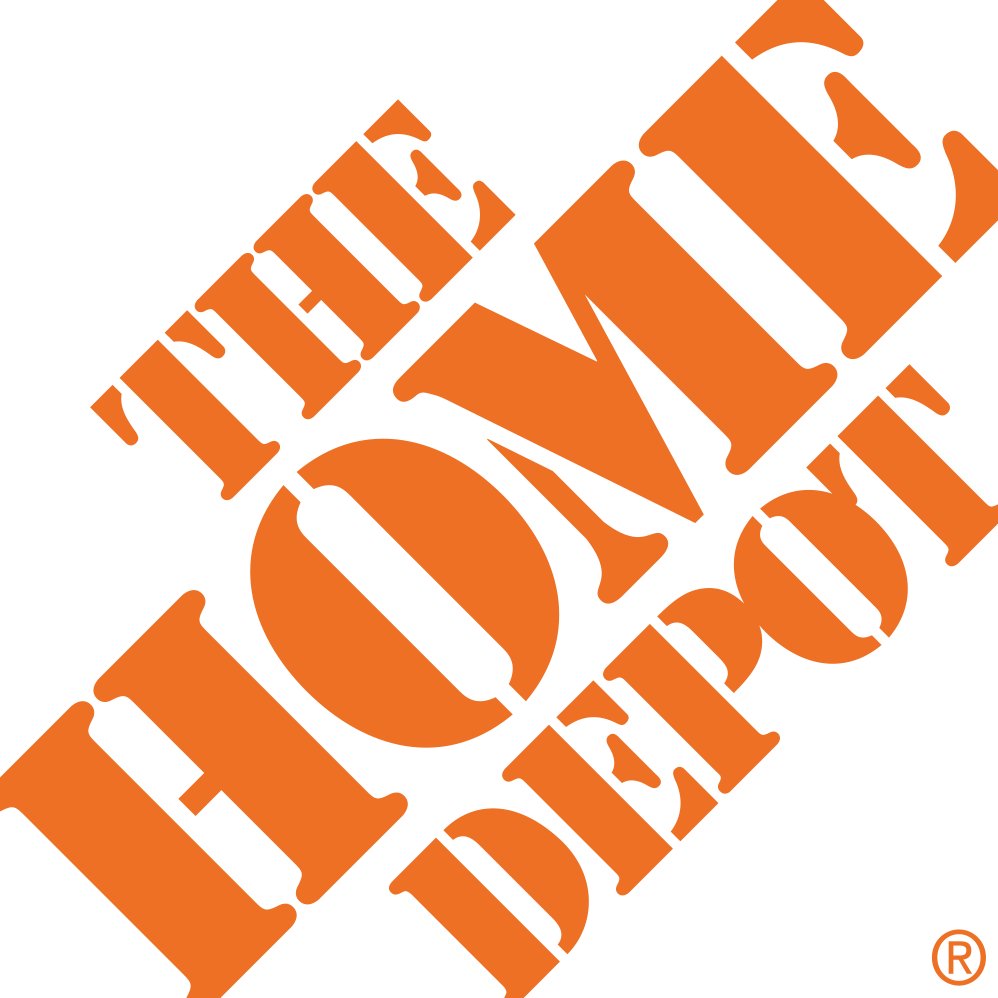
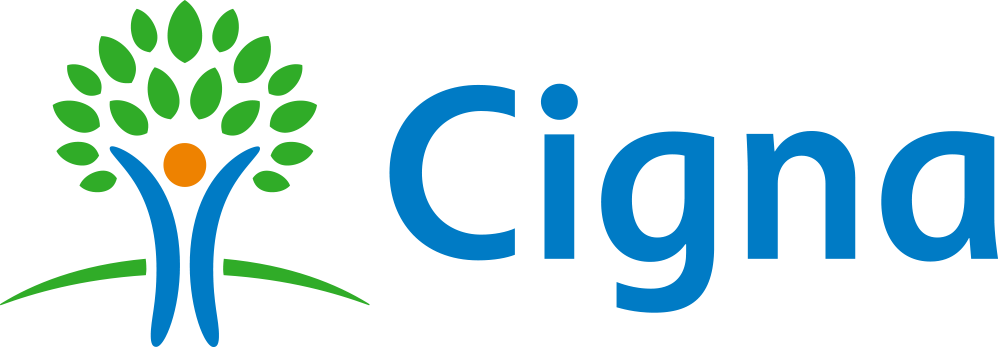
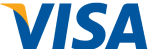
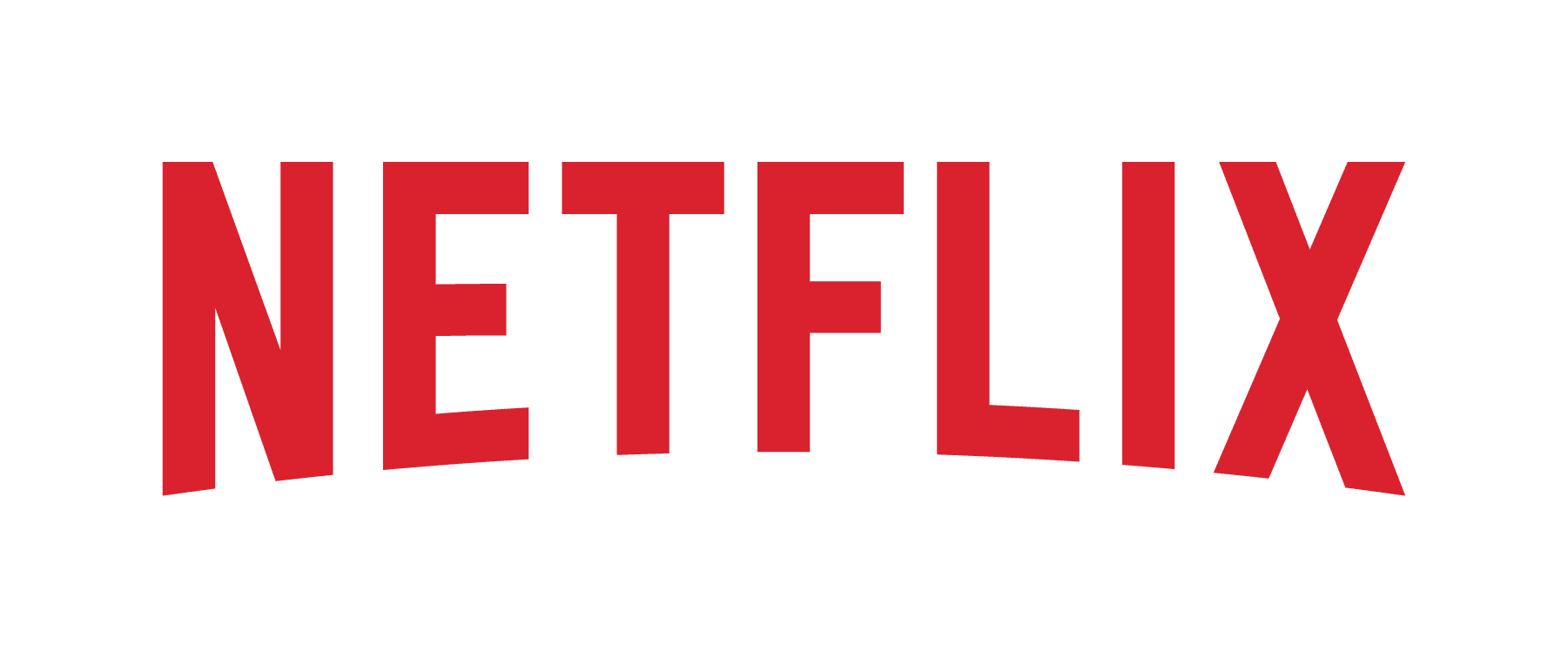
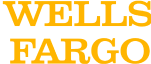

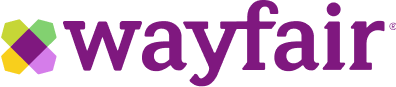
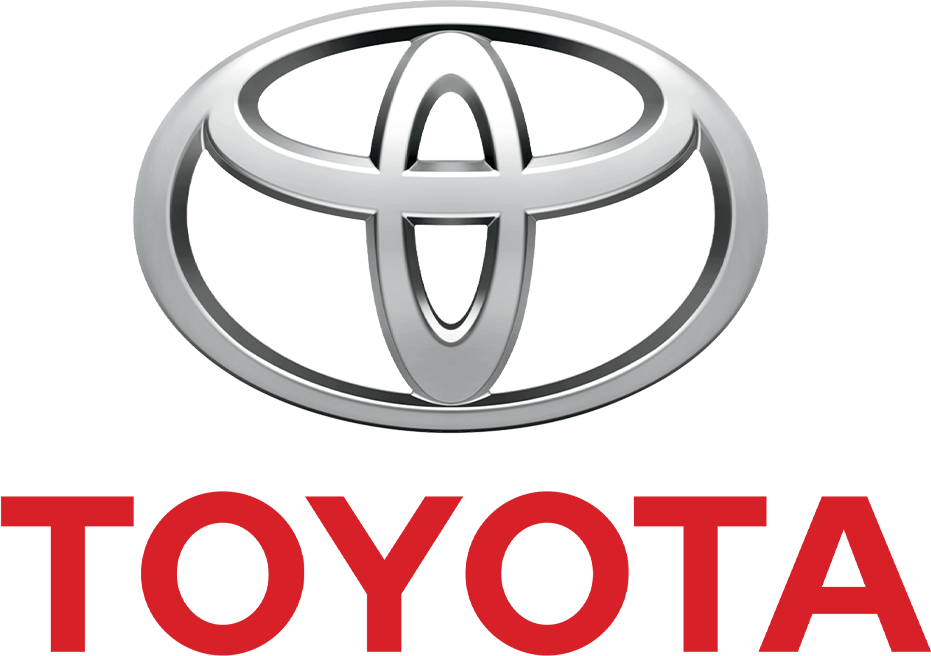
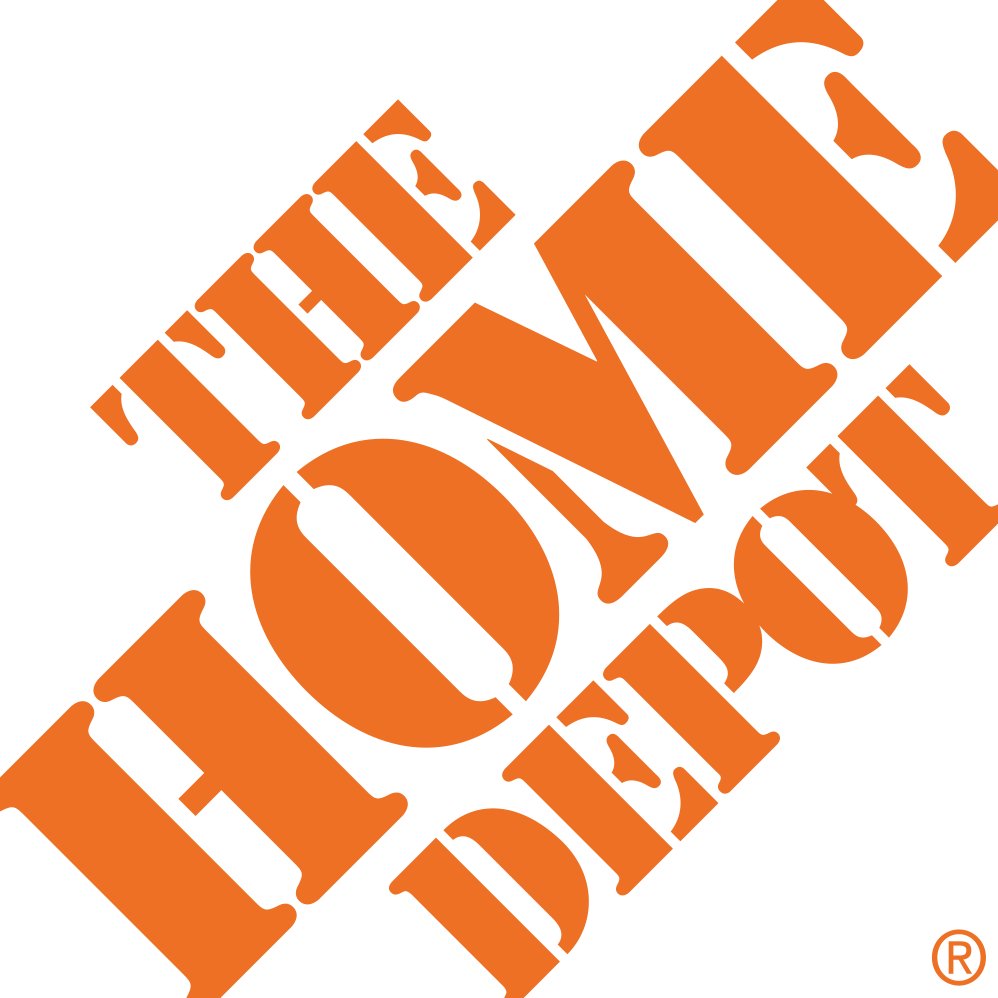
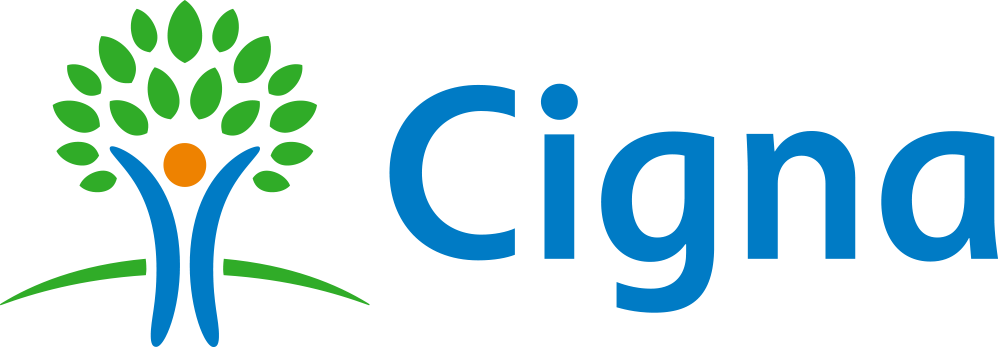
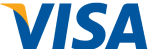
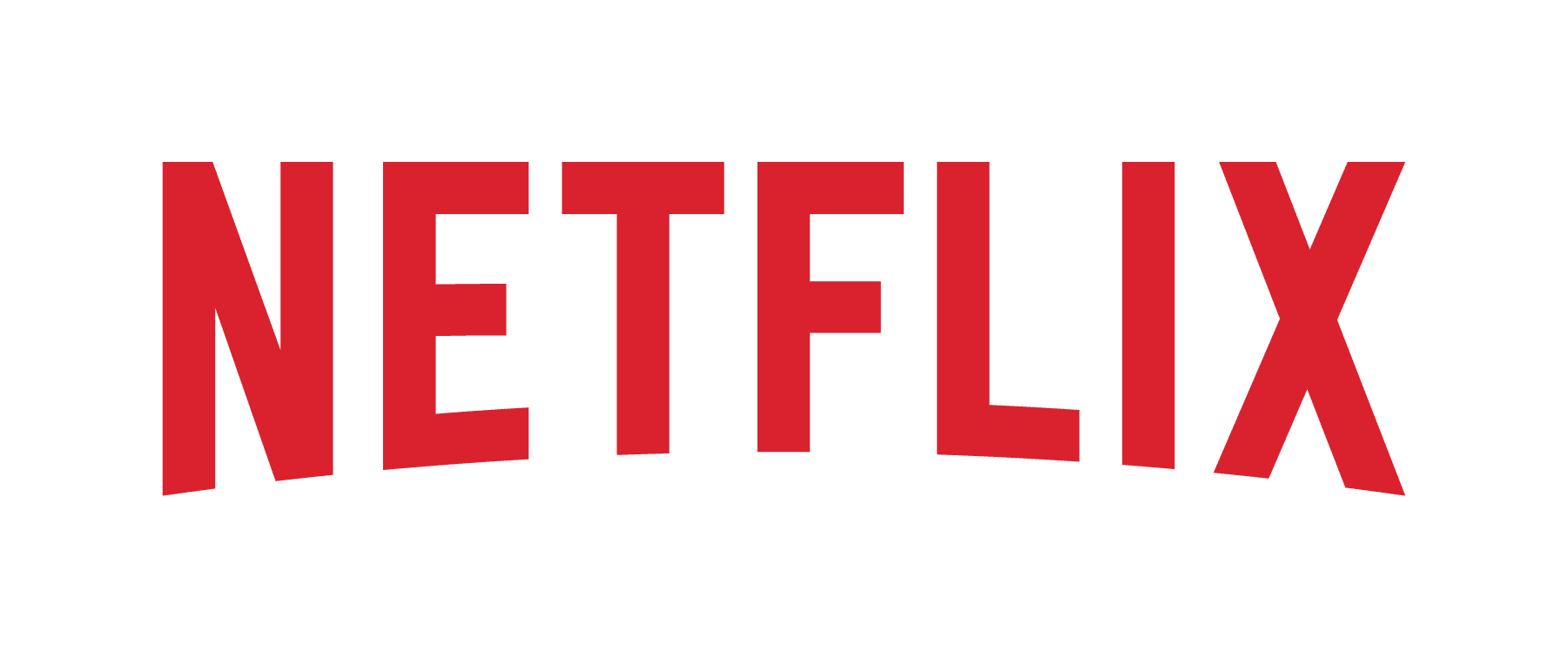
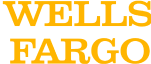

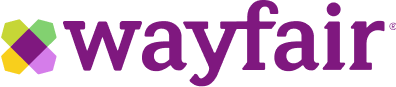
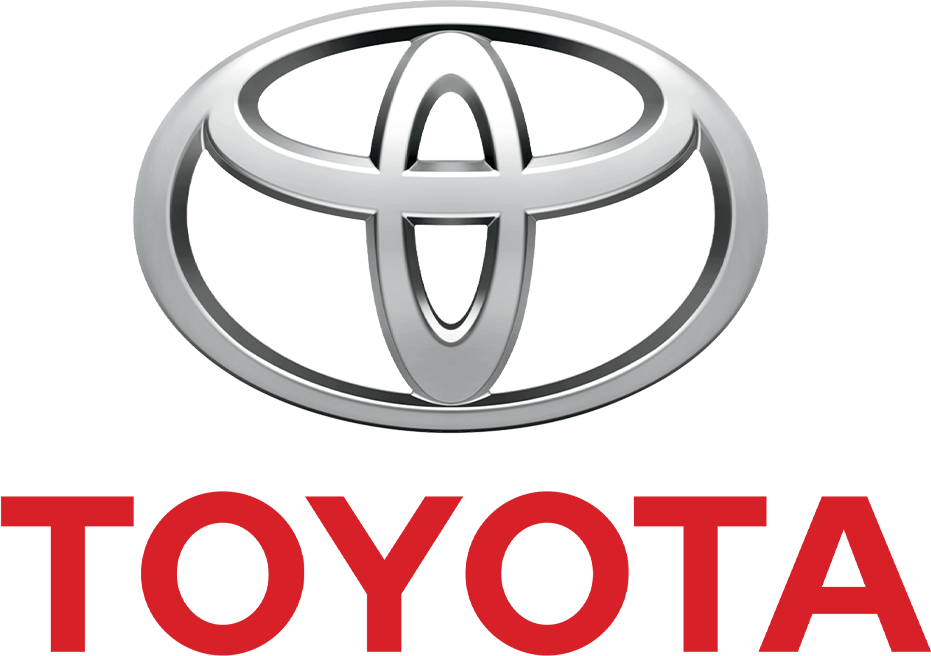
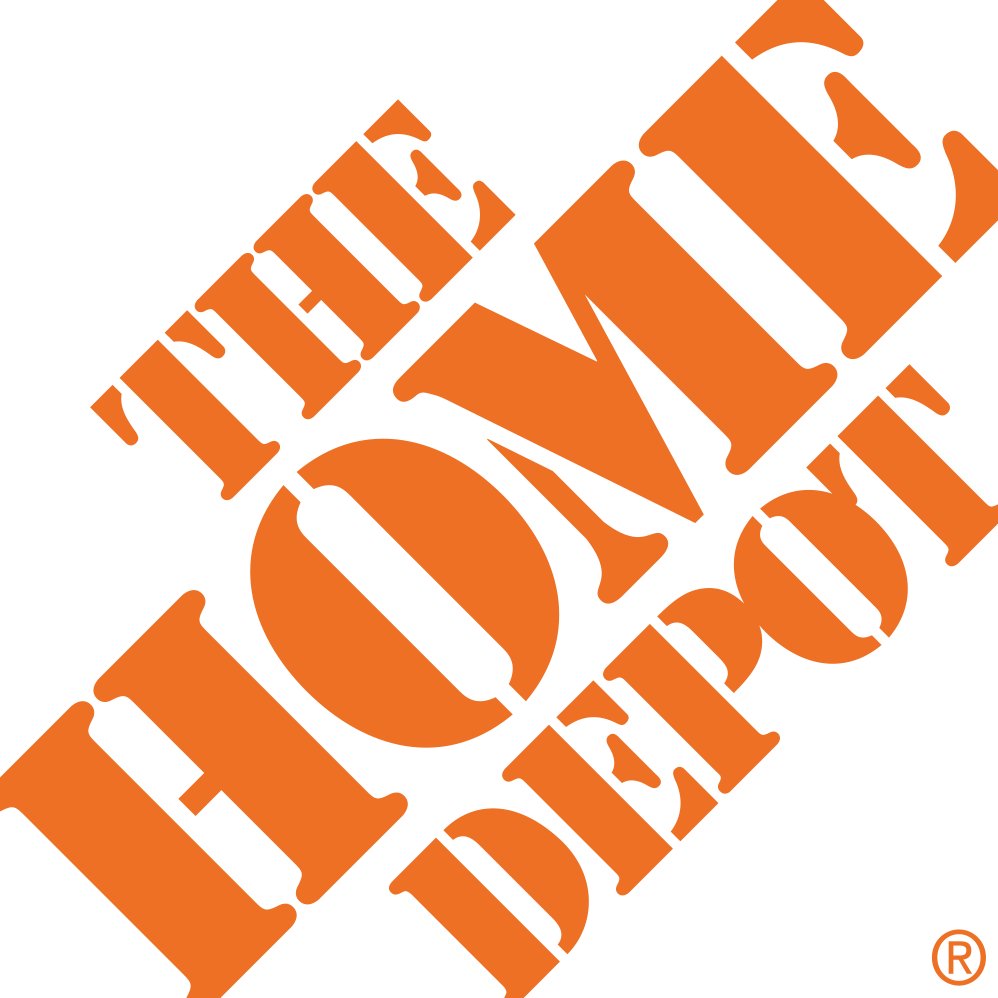
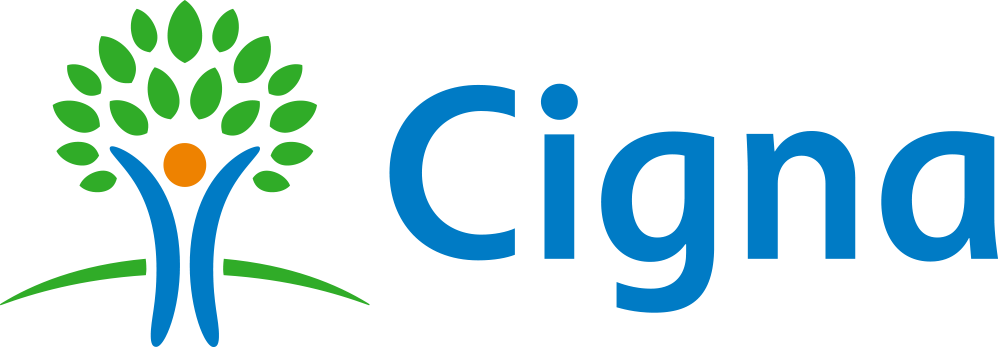
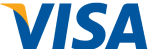
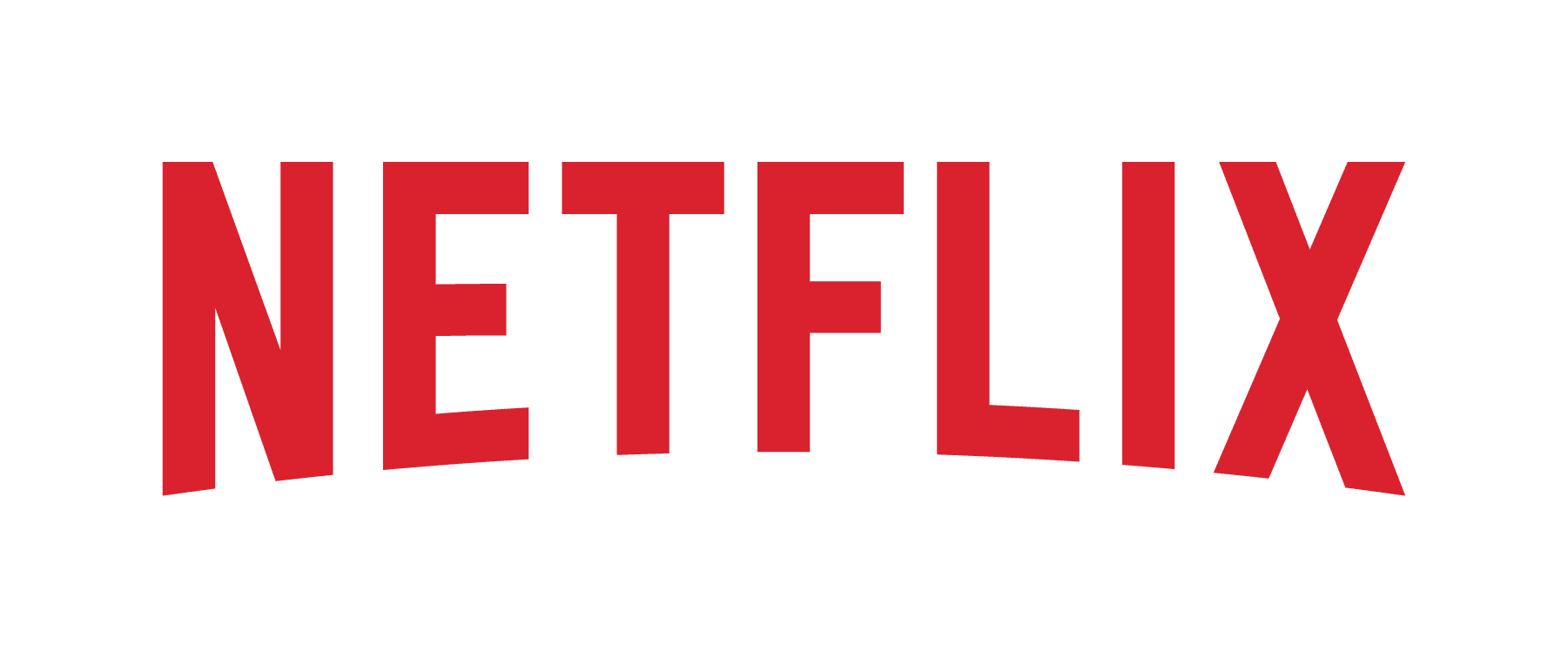
Connect any data source to any AI/BI tool without moving your data
What is a semantic layer? A semantic layer is a business representation of corporate data that helps end users access data autonomously using common business terms. A semantic layer maps complex data into familiar business terms such as product, customer, or revenue to offer a unified, consolidated view of data across an organization.
Data Source
Cloud Data
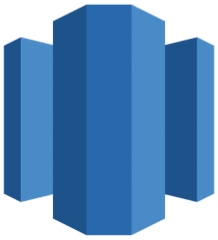
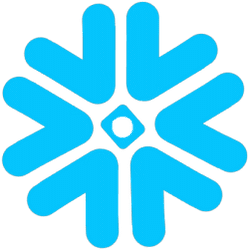
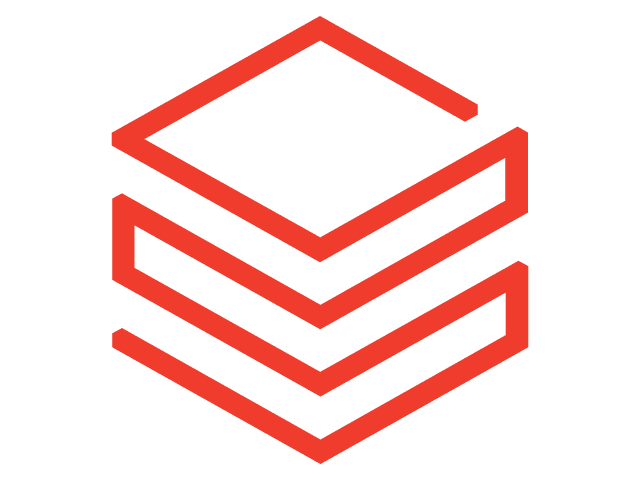
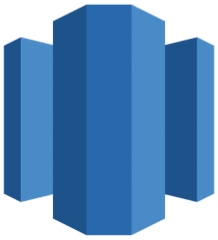
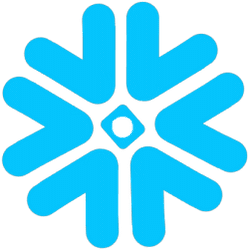
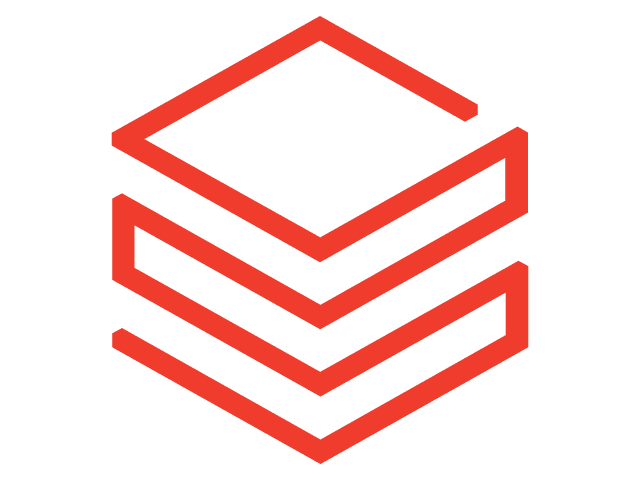
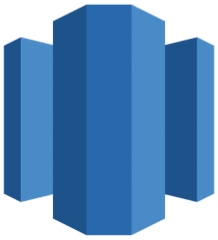
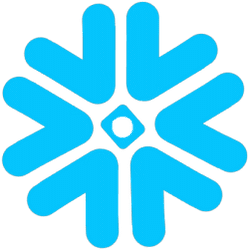
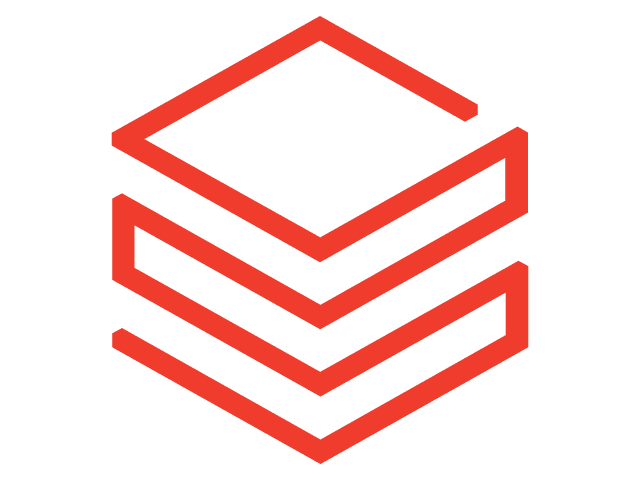
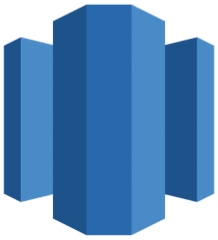
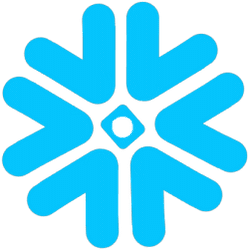
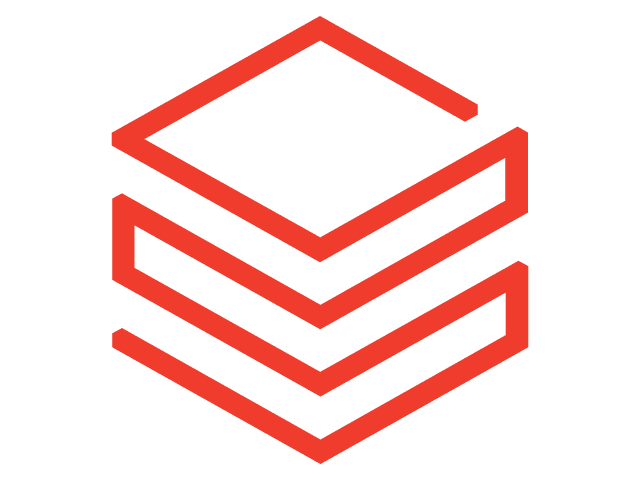
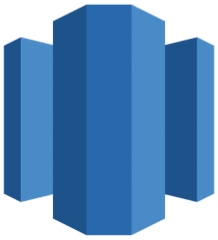
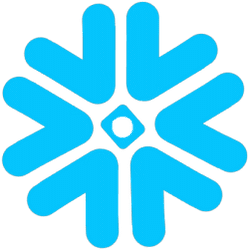
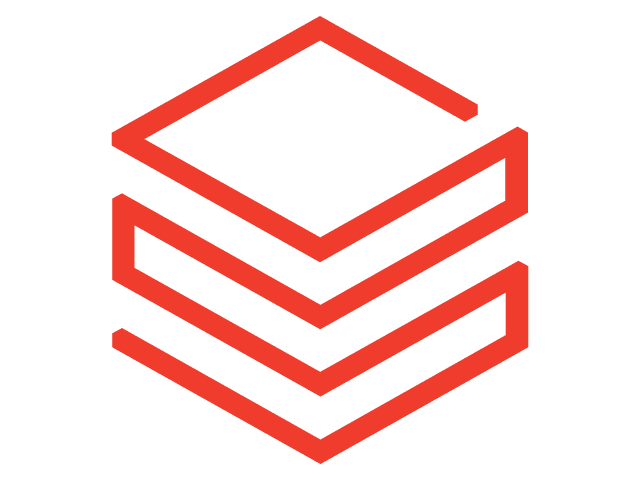
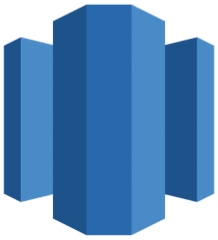
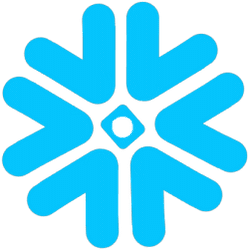
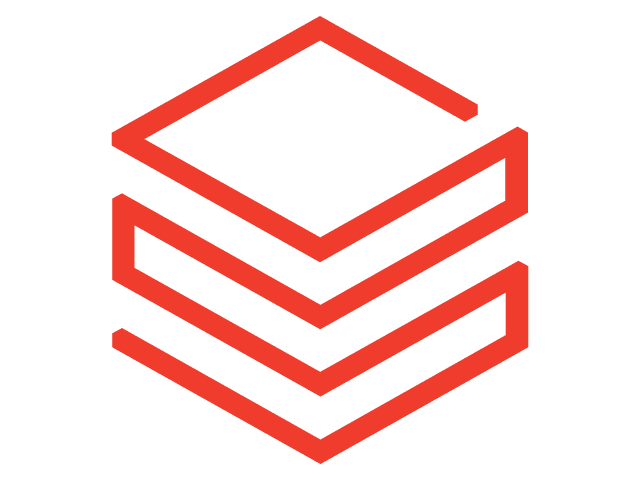
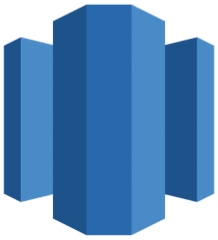
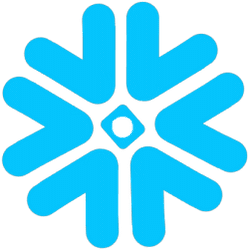
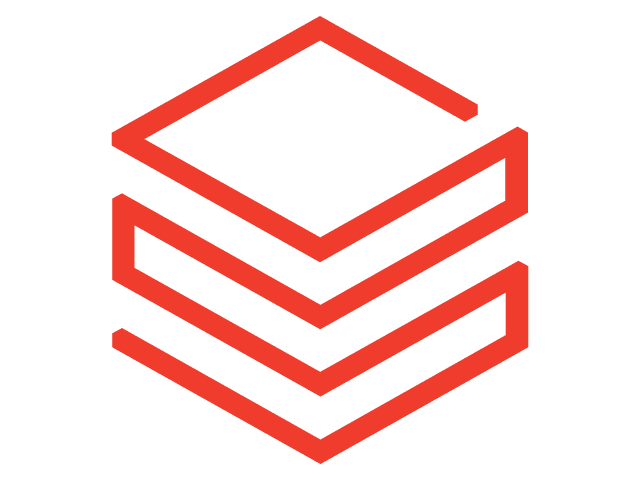
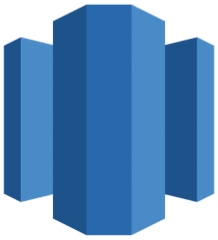
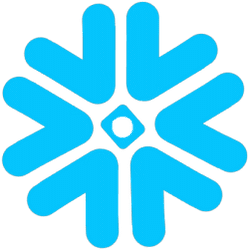
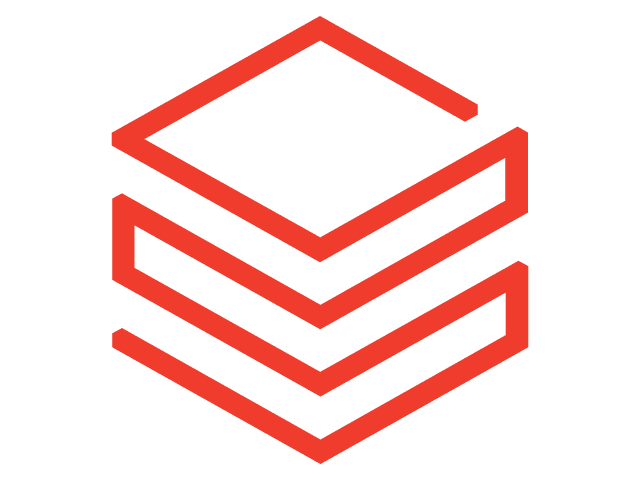
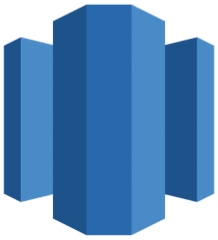
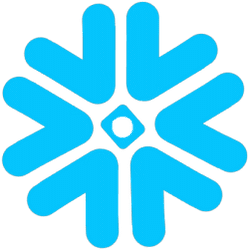
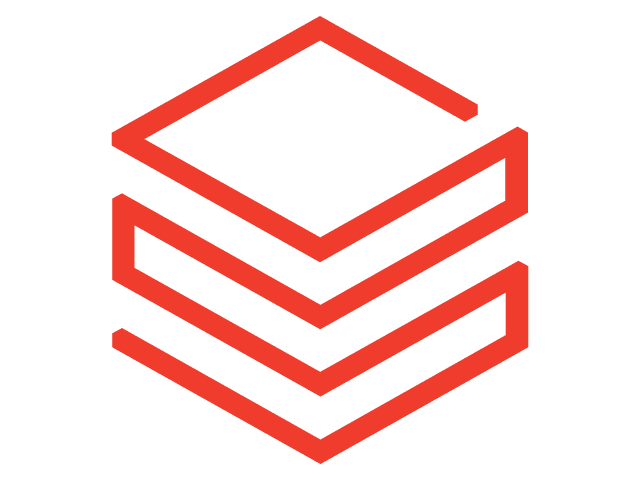
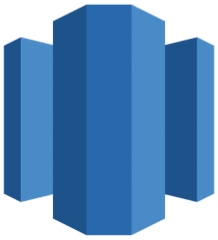
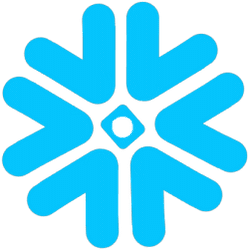
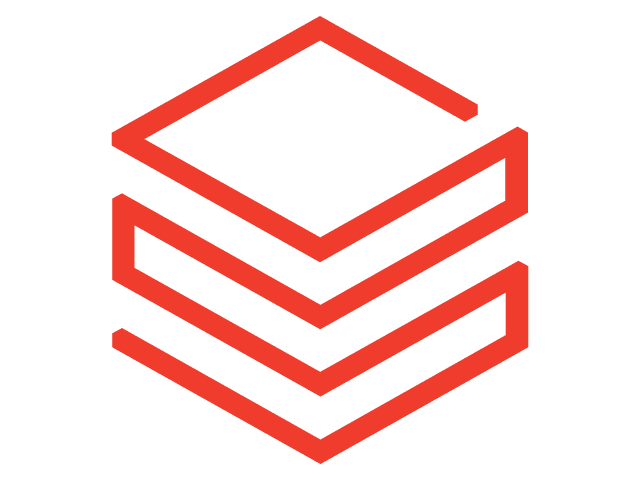
Semantic Layer
Data Democratization & Governance
Define metrics, dimensions, and relationships once, providing consistent KPIs and reusable data models across all tools; eliminating discrepancies and delivering accurate, trusted results organization-wide.
Cloud Cost Management & Performance
Control cloud data platform cost and deliver “speed of thought” analytics with automated query performance tuning.
Enabling Gen AI with Business Context
Provide business context to AI models and train your LLM using a semantic engine to prevent hallucinations and improve the accuracy of natural language queries—no deep technical expertise required.
Insight Layer
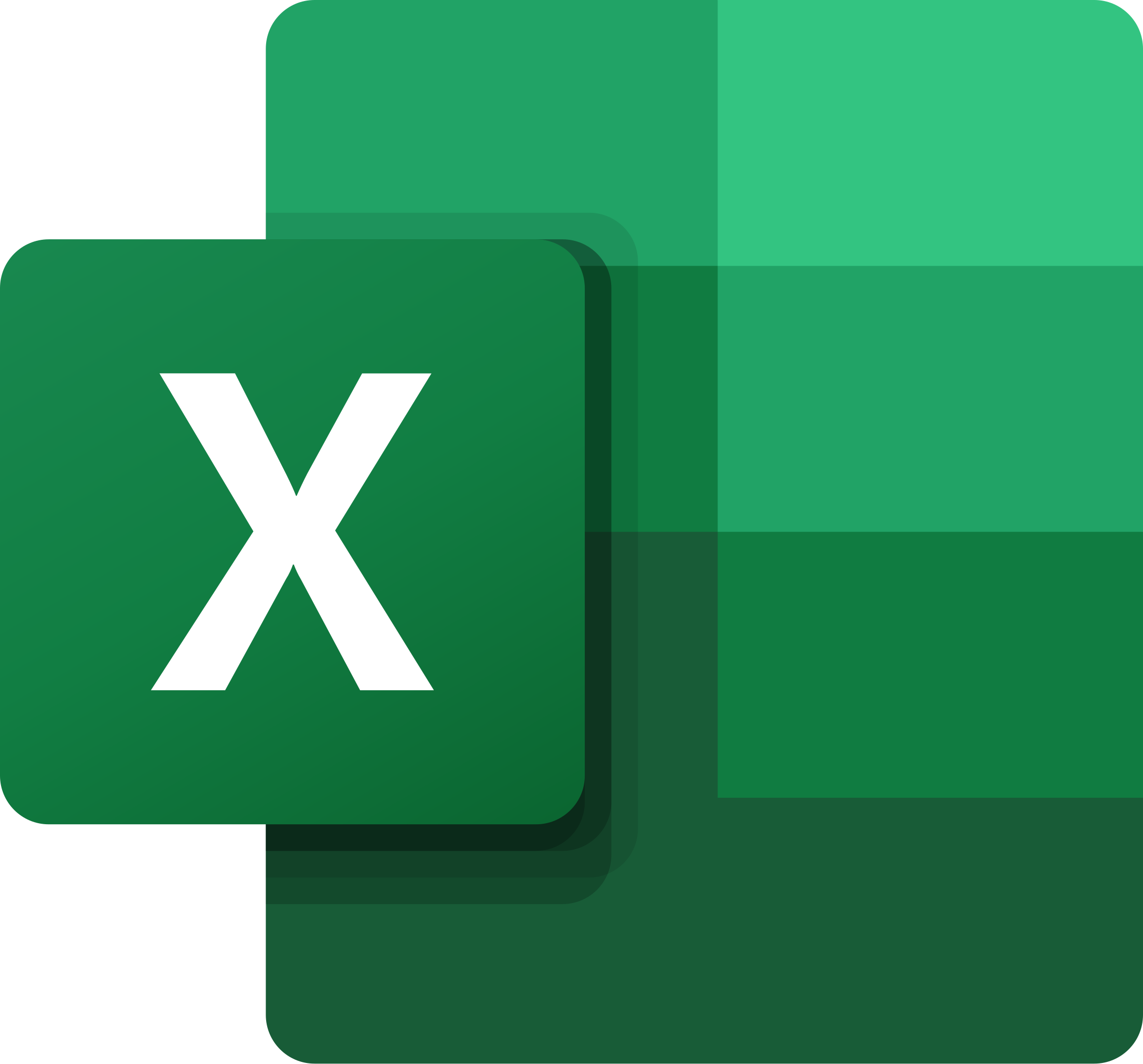
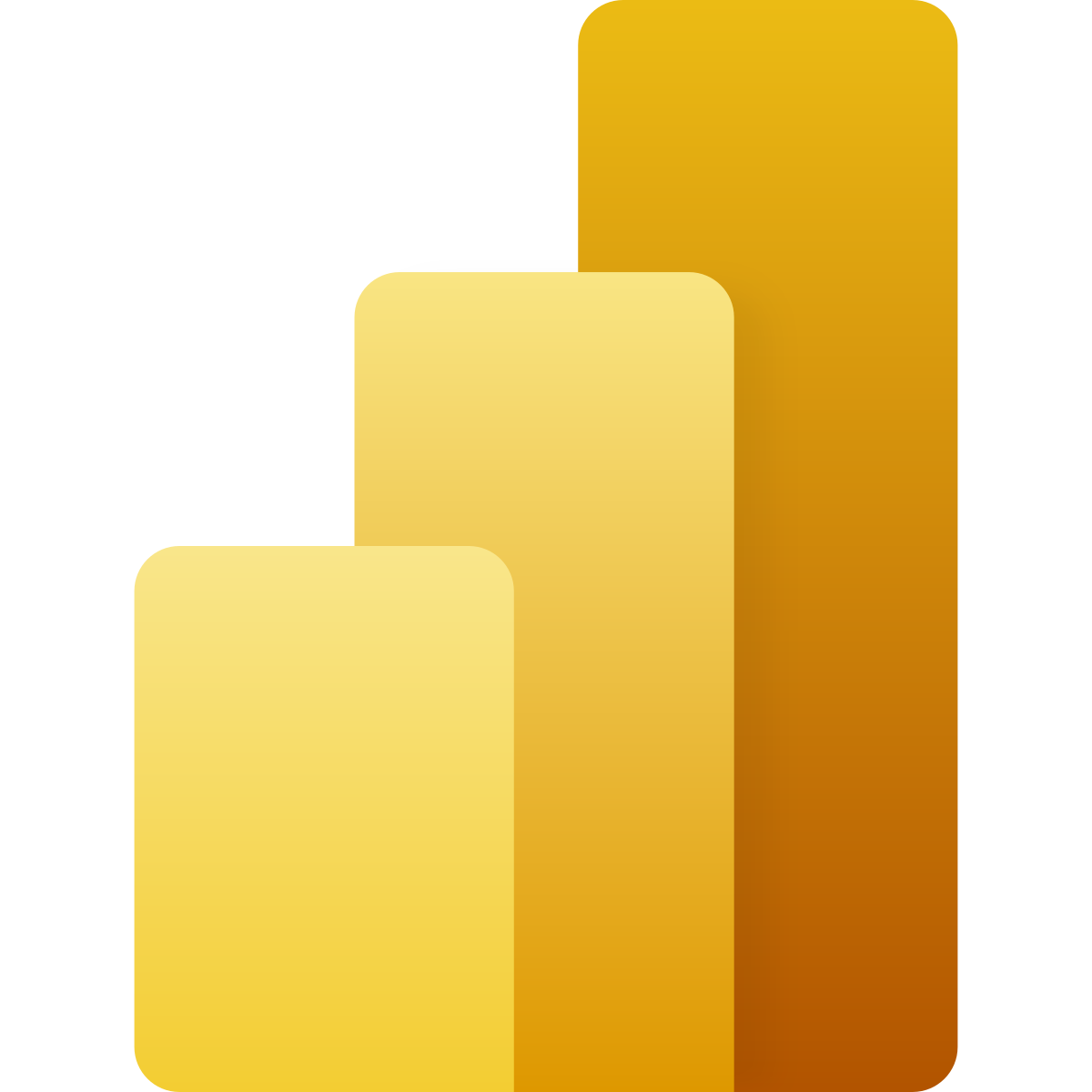
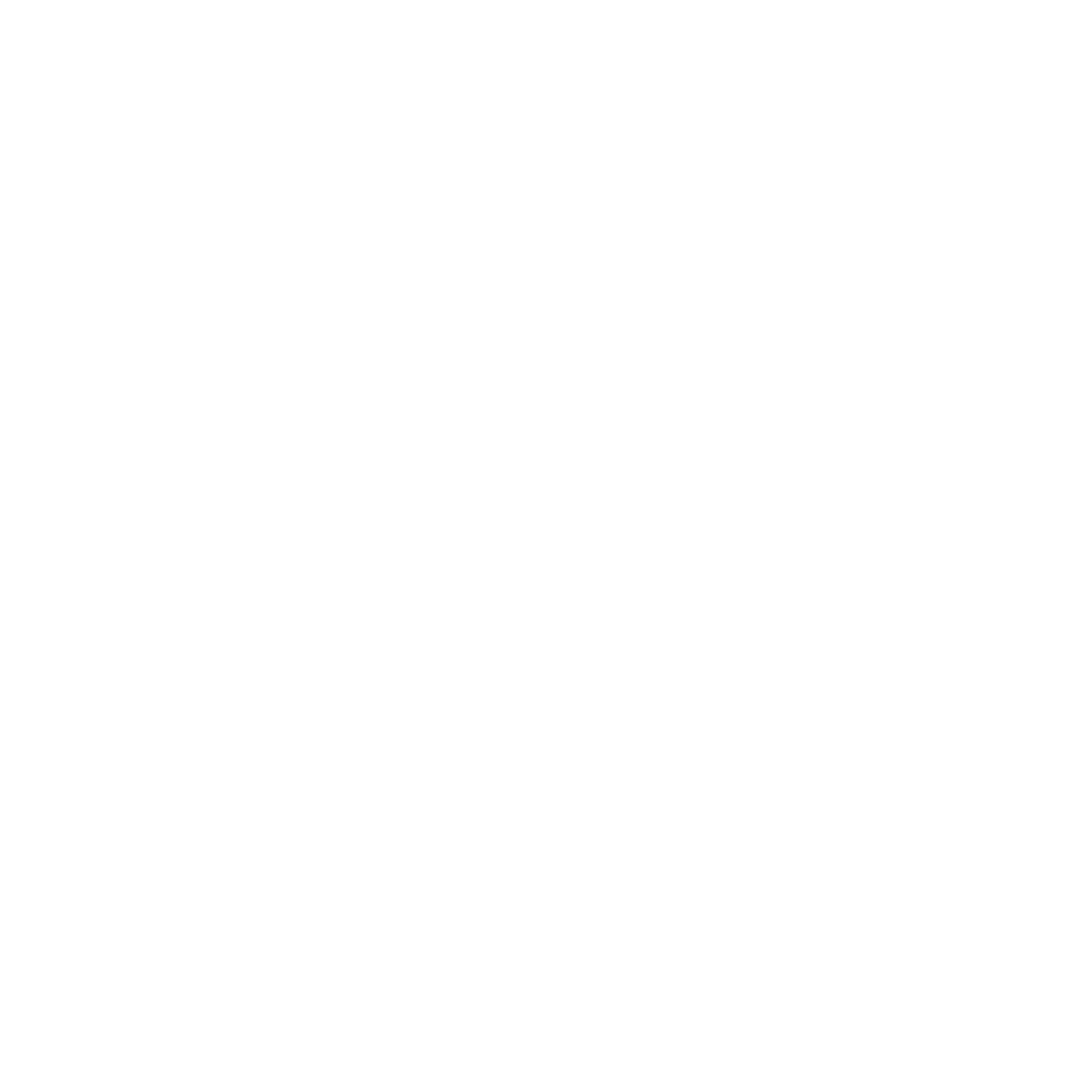
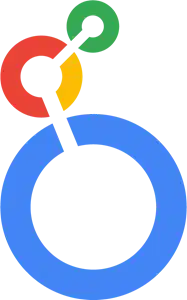
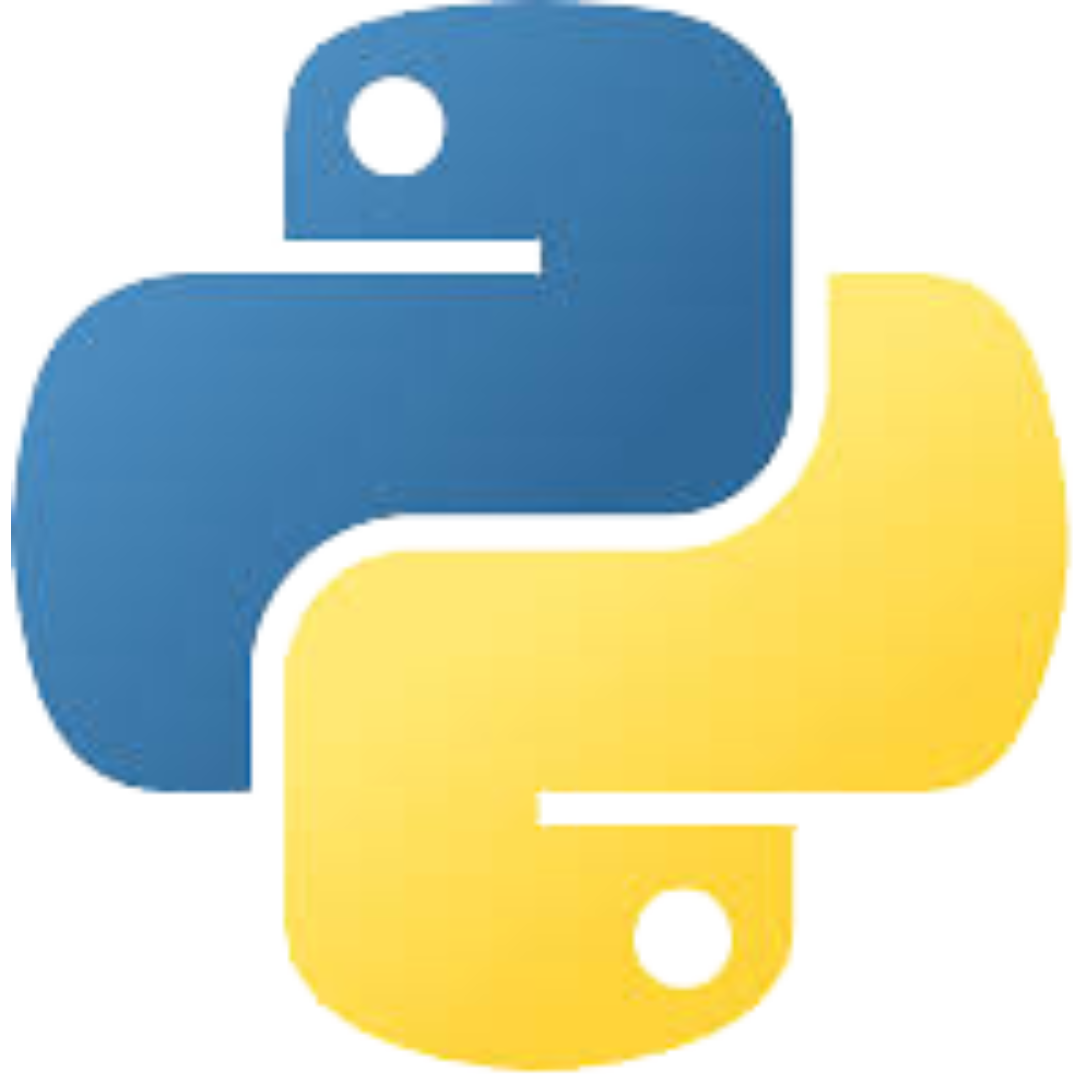
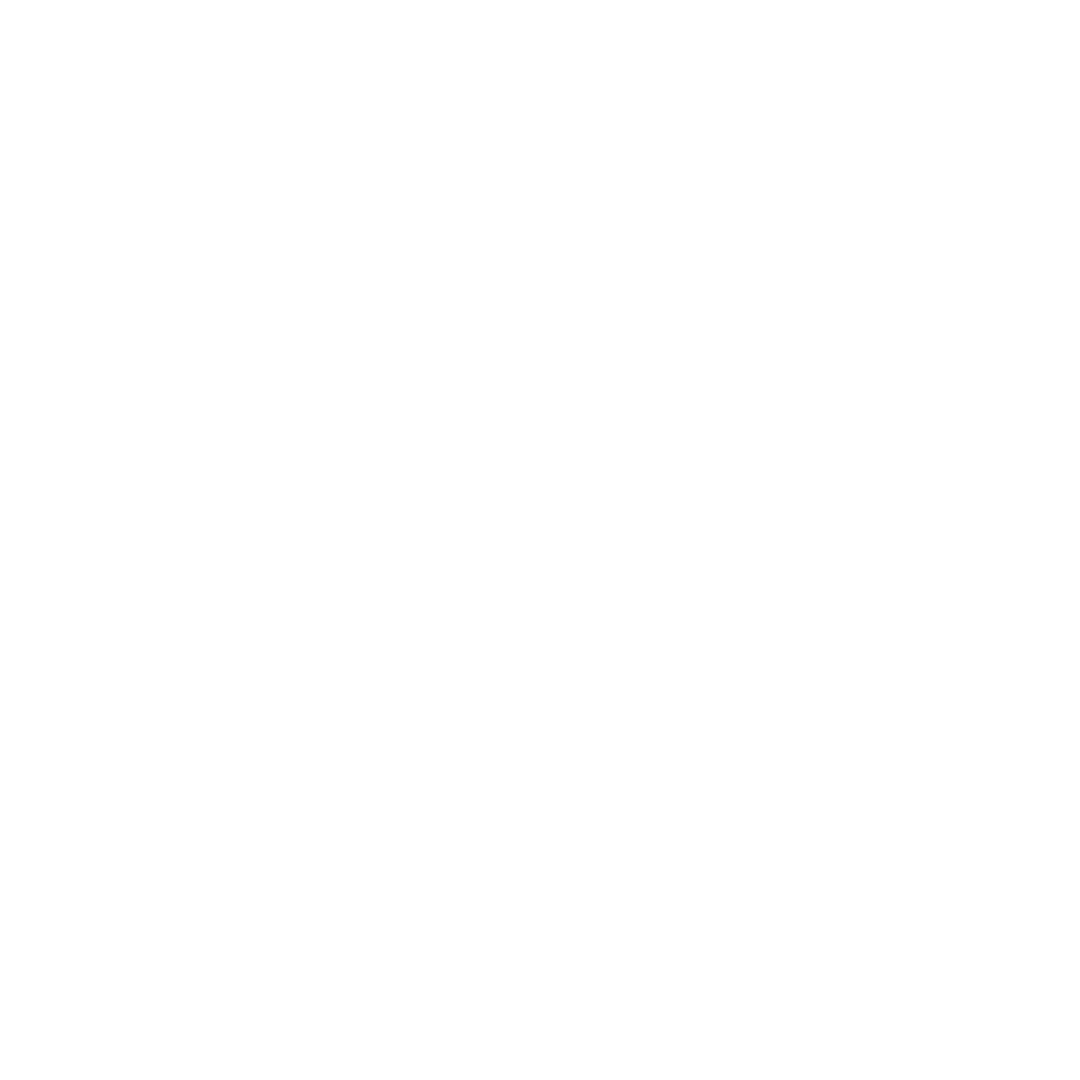
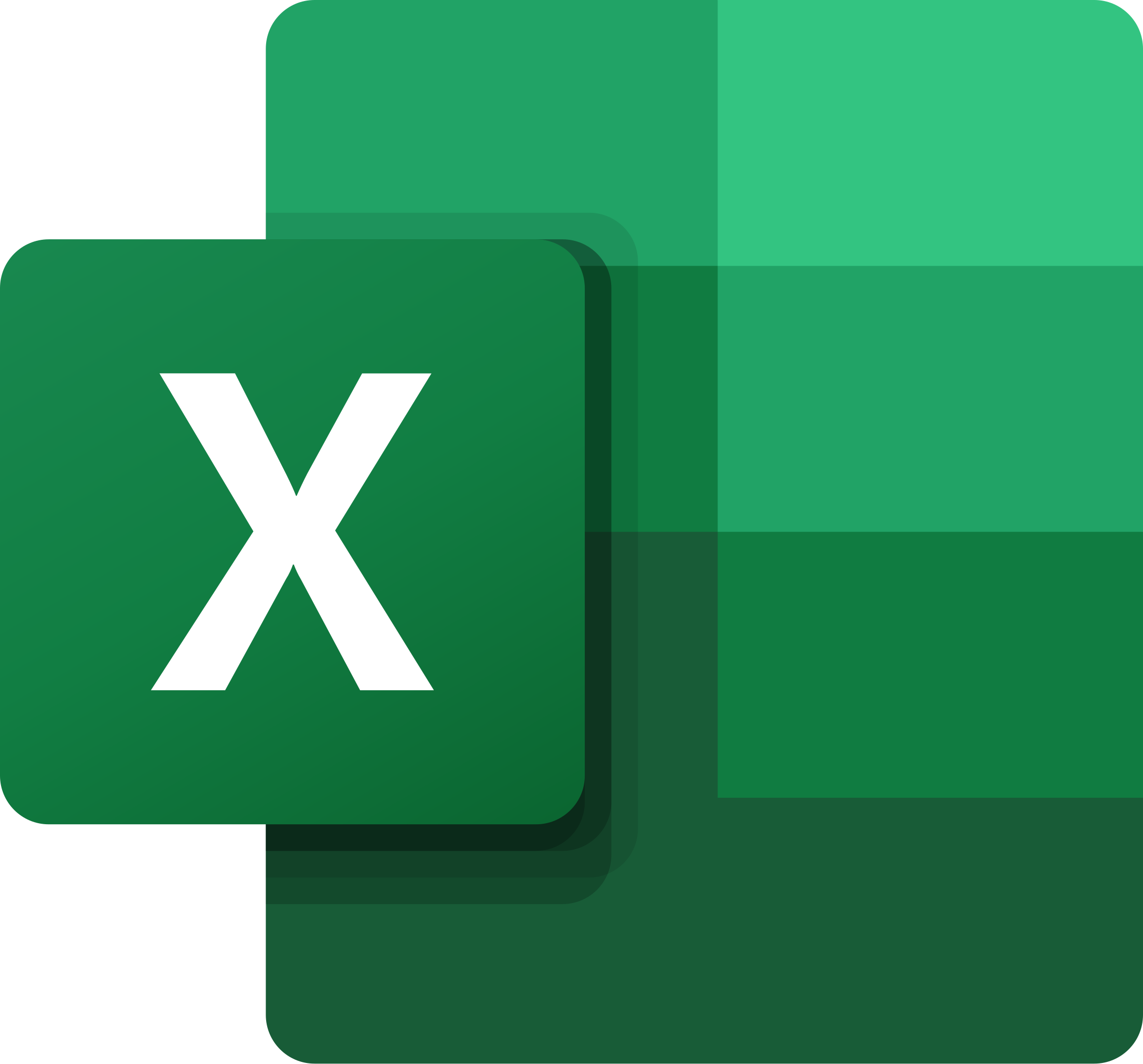
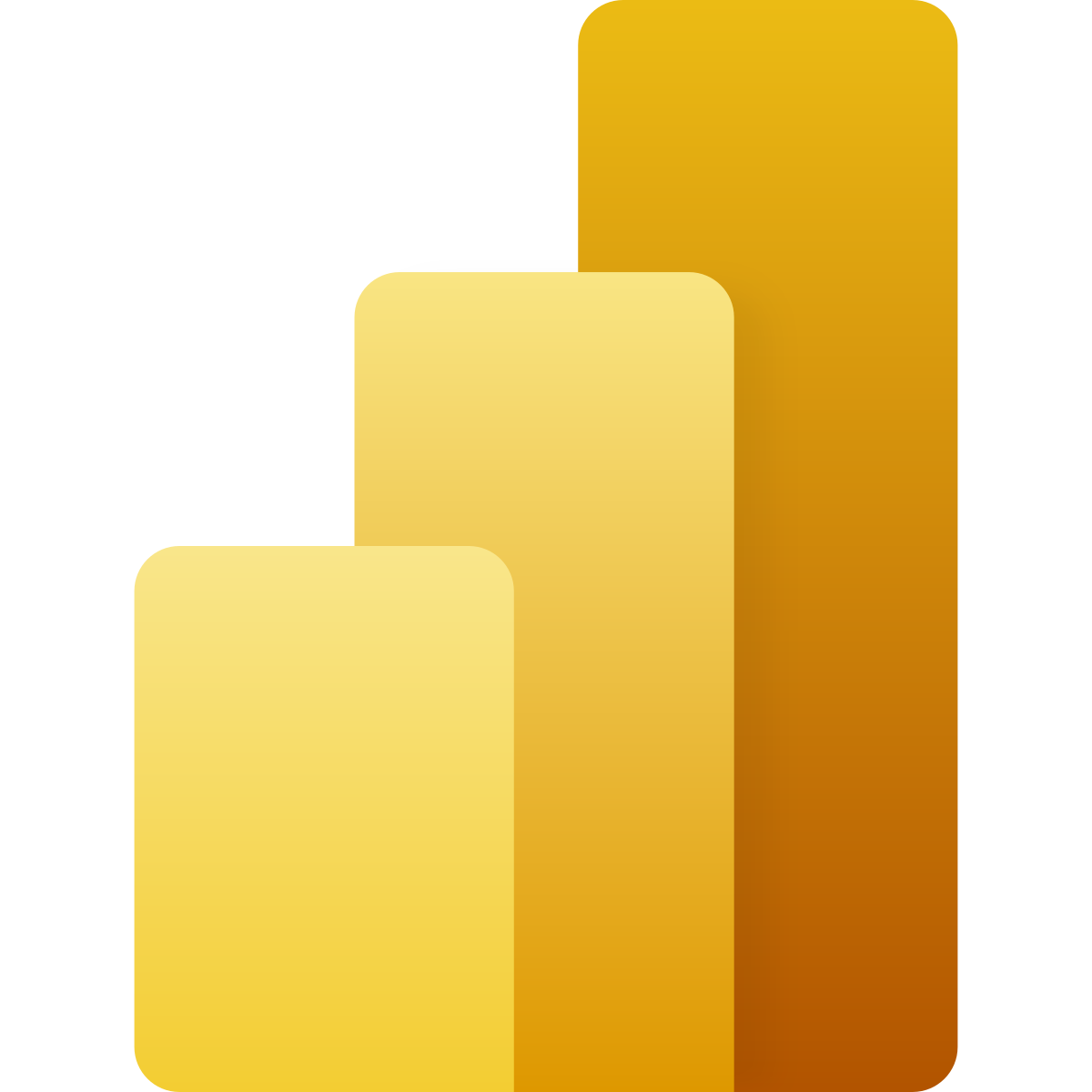
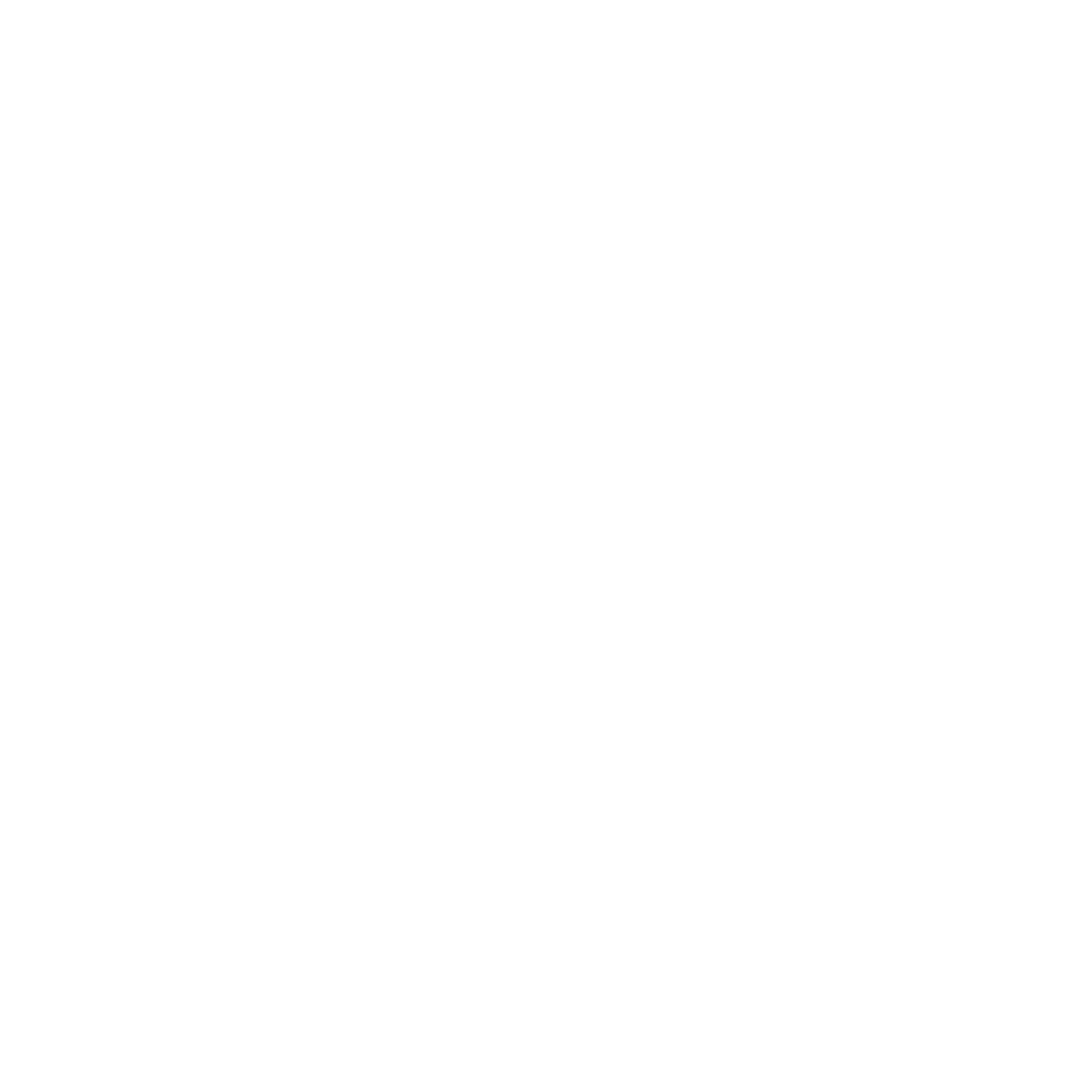
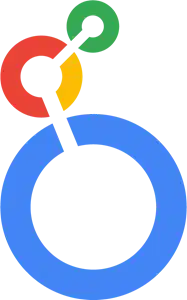
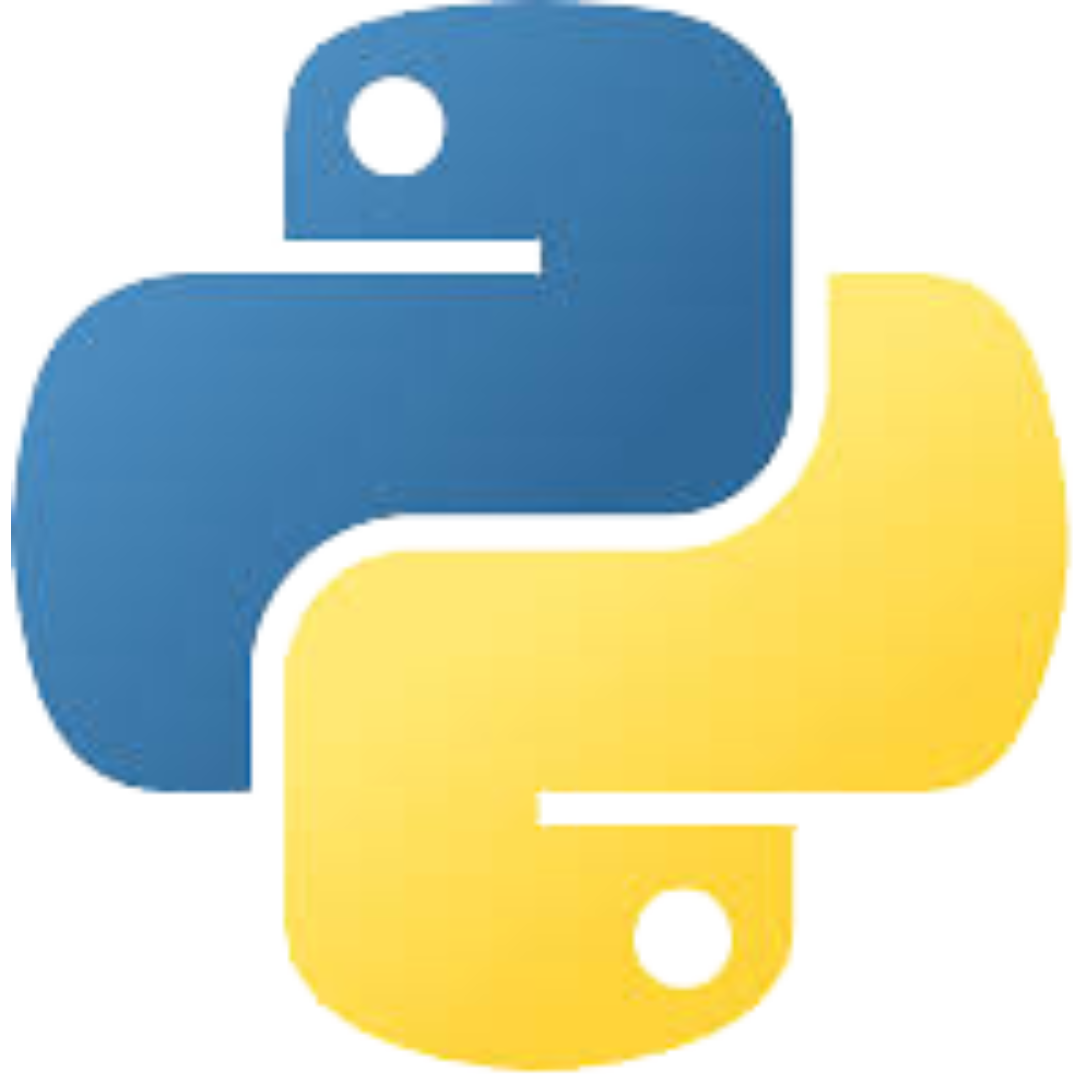
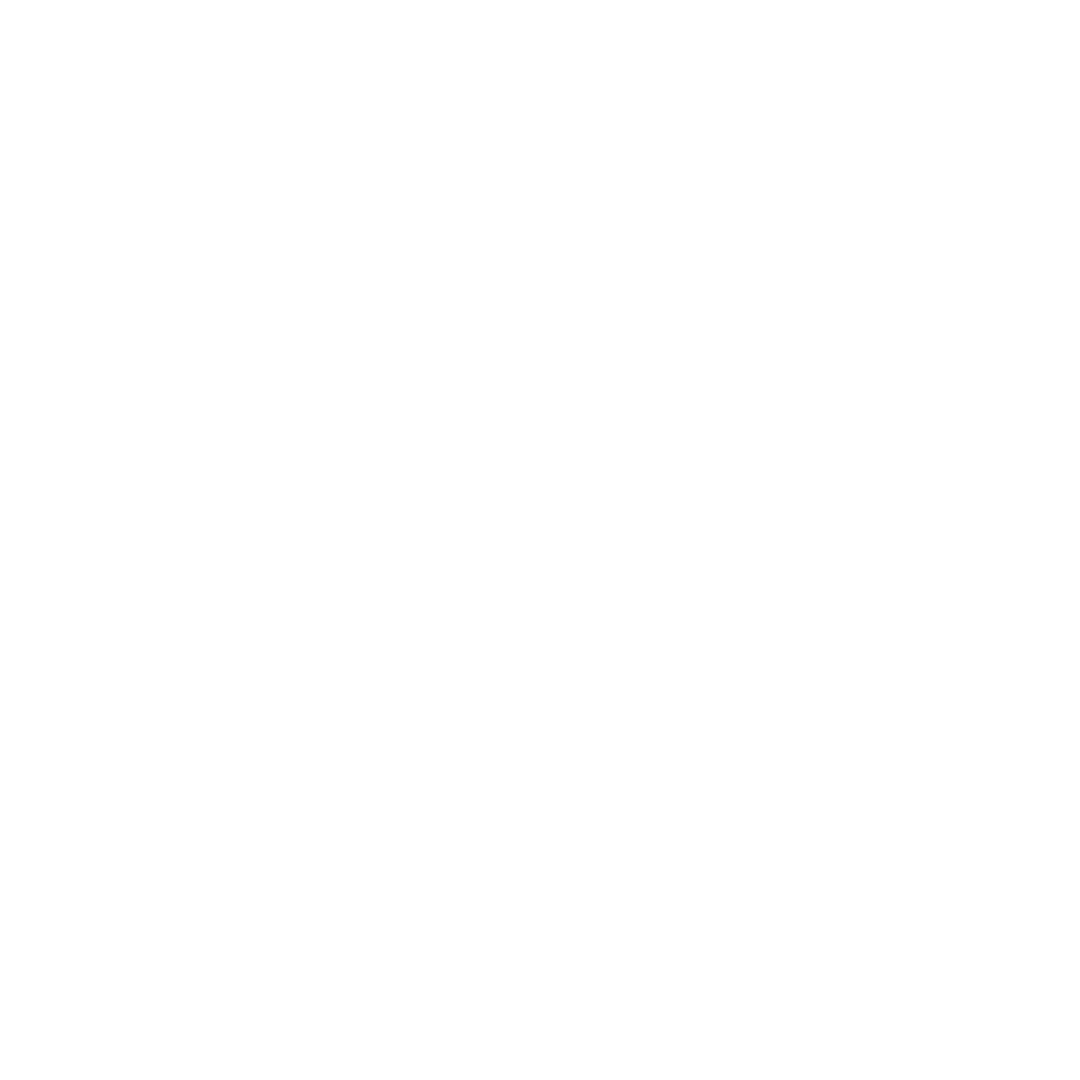
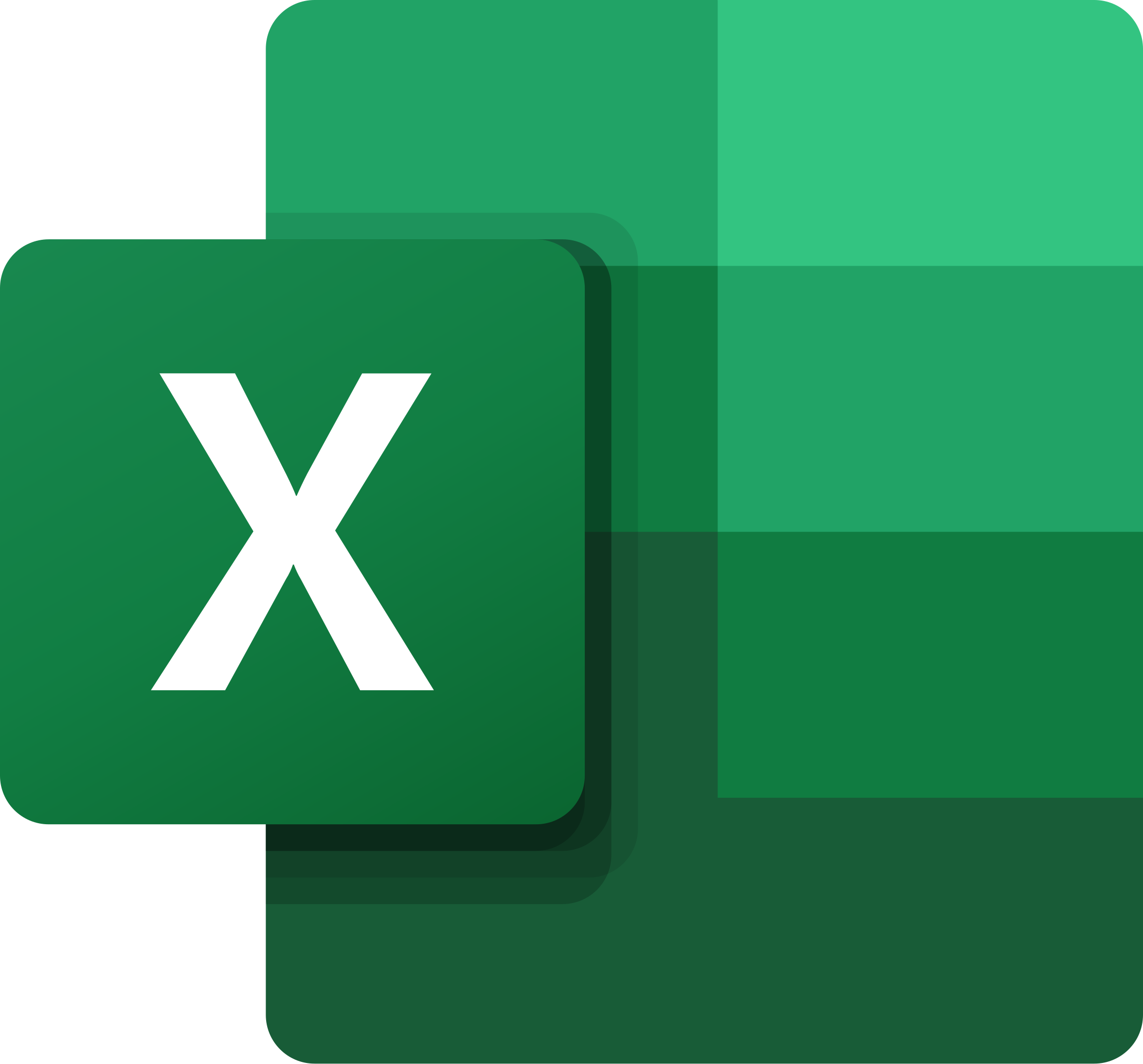
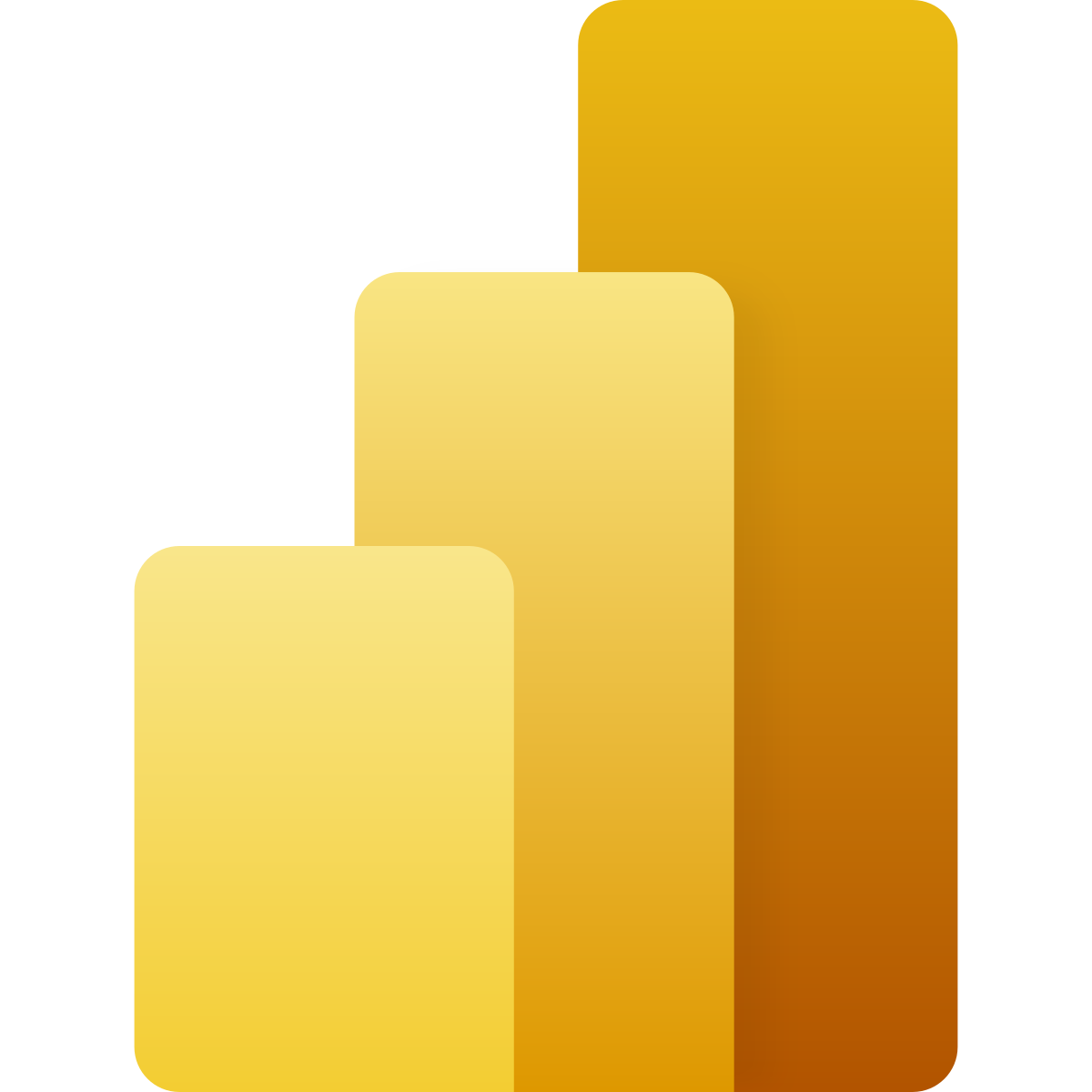
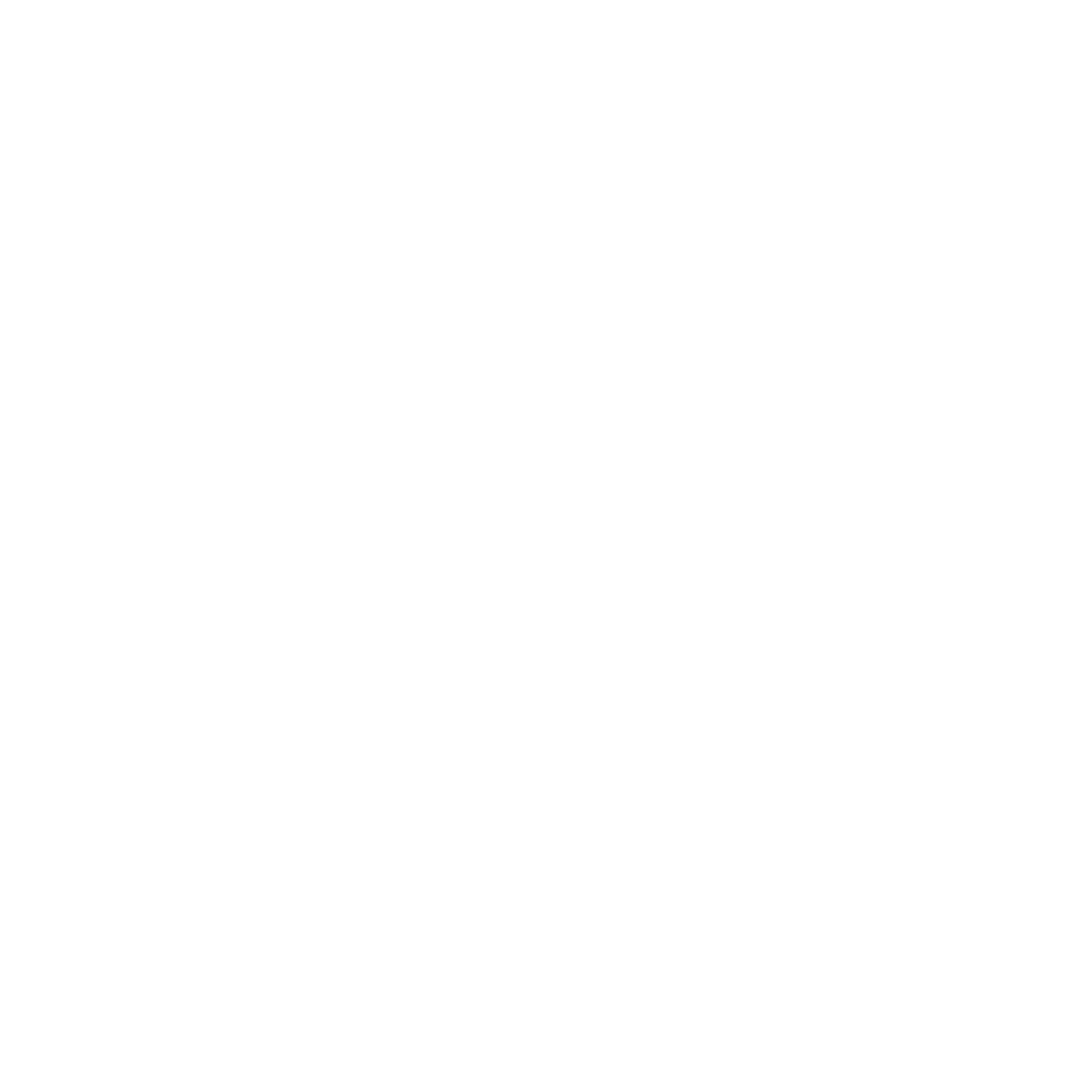
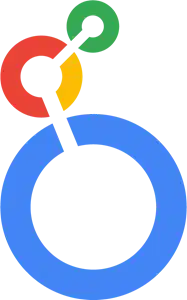
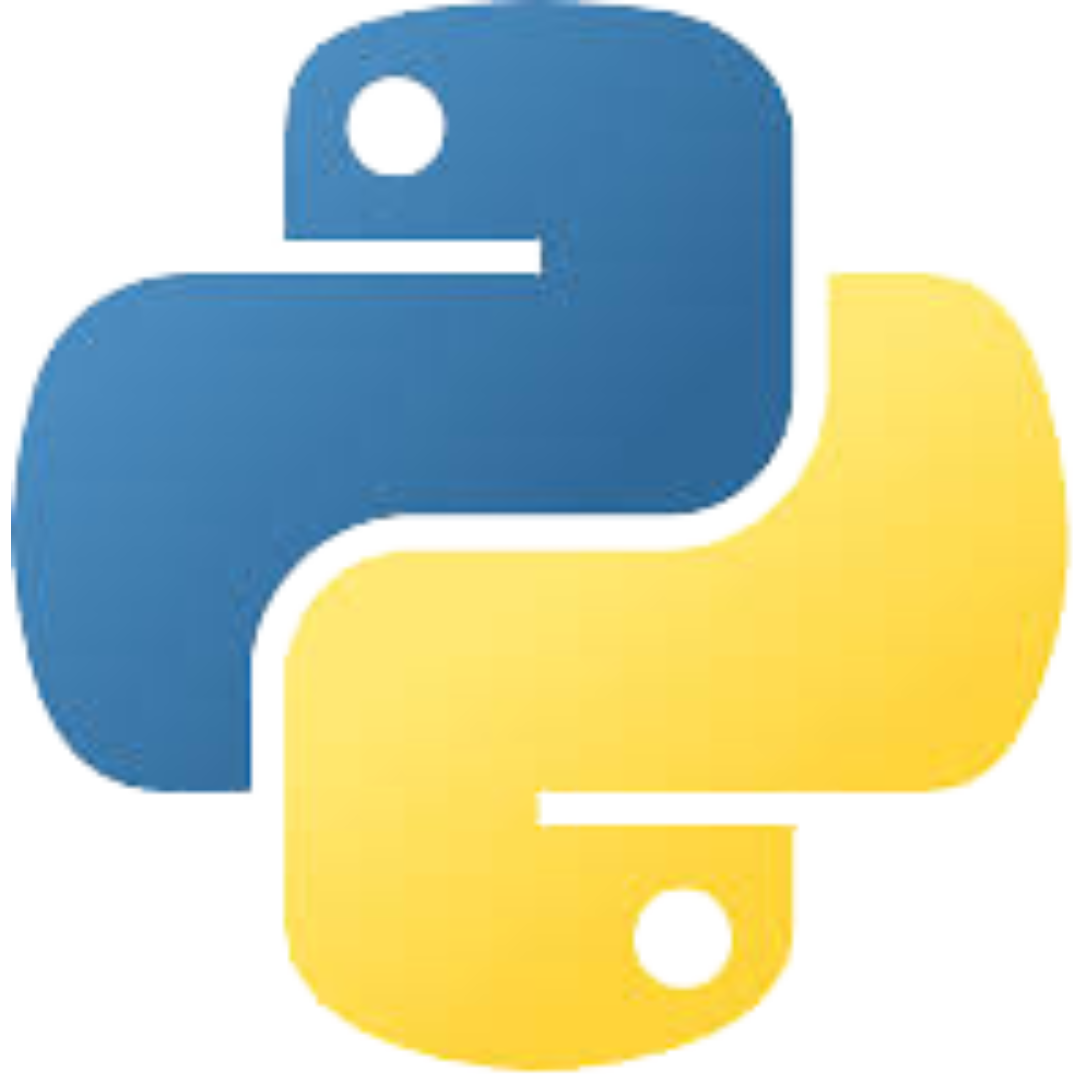
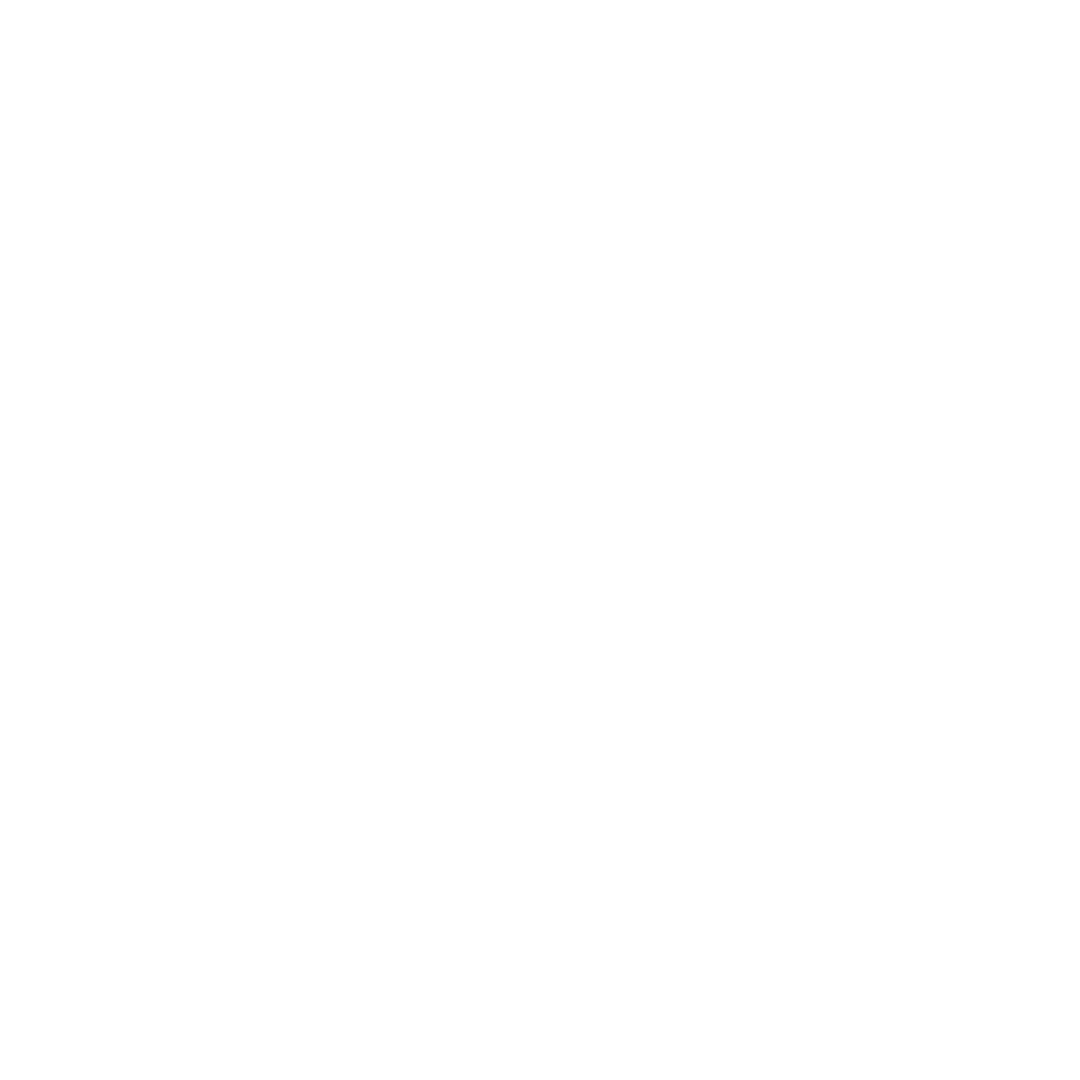
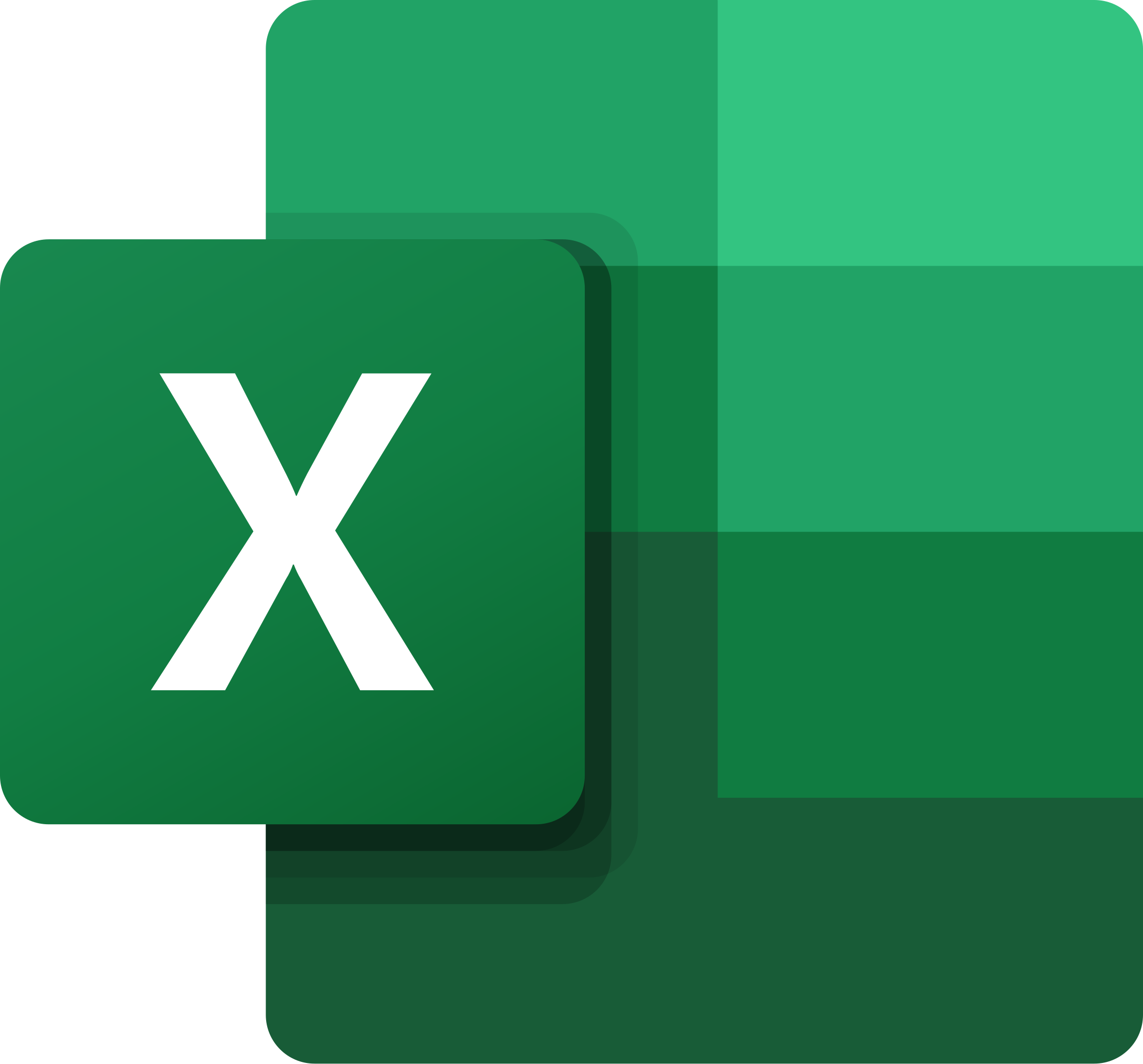
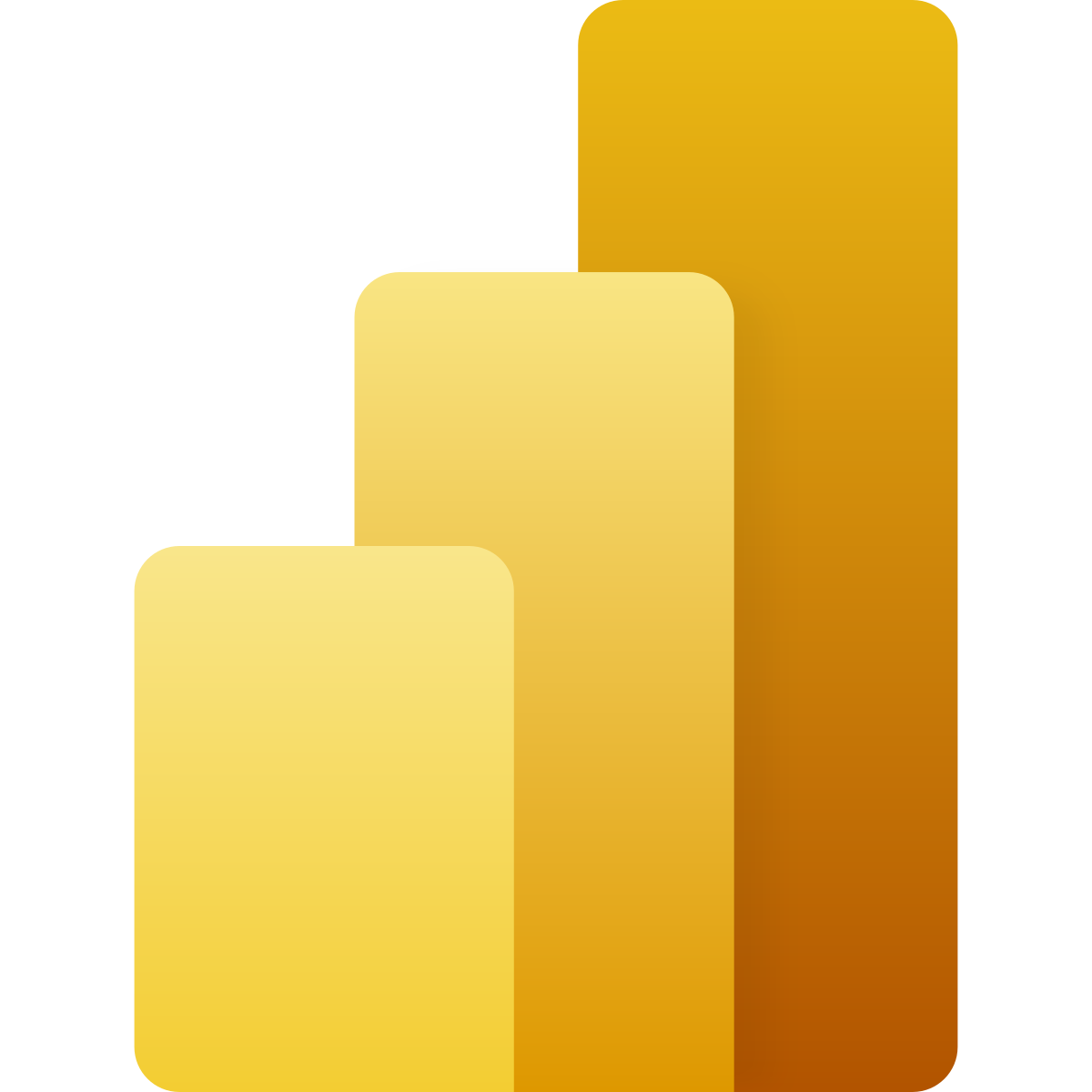
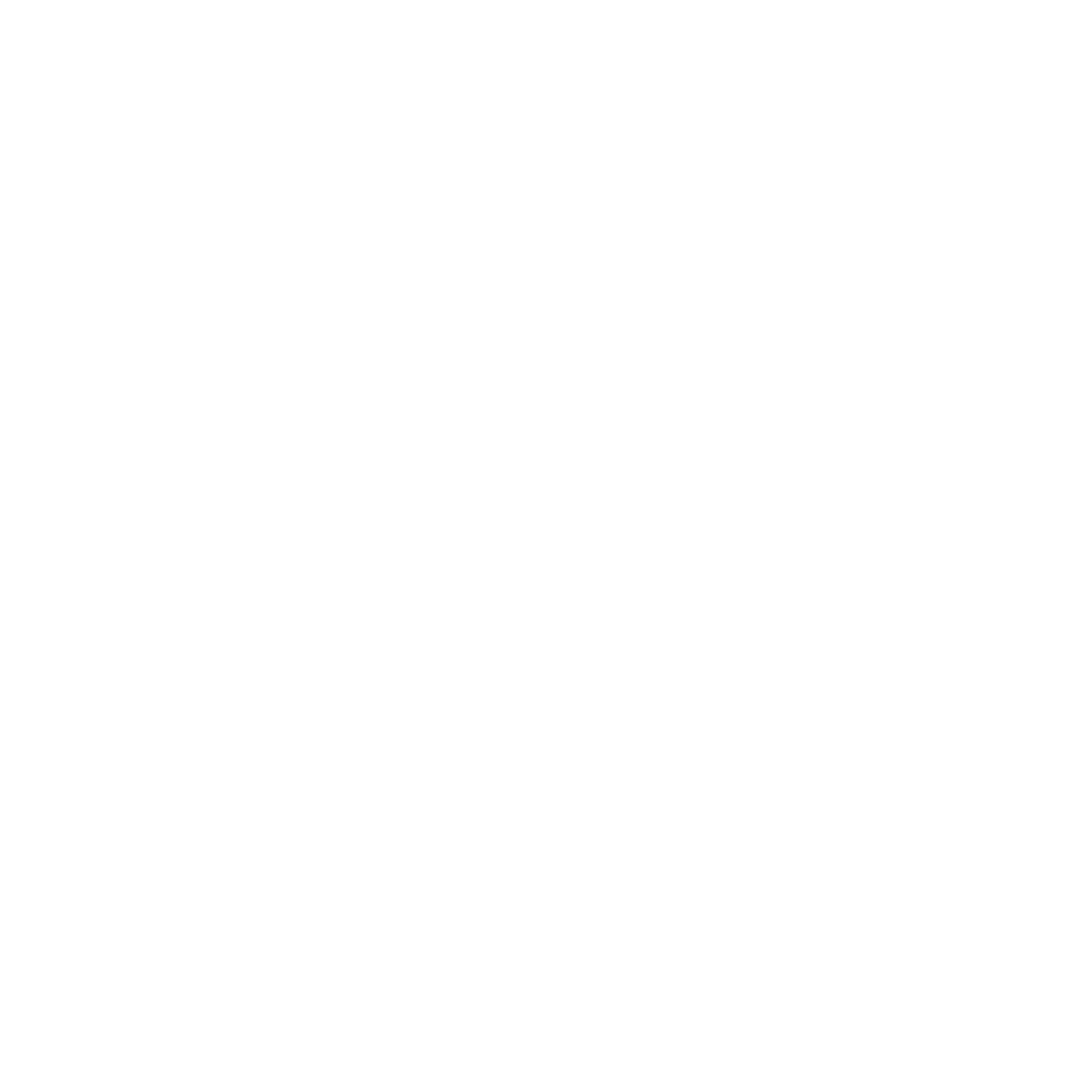
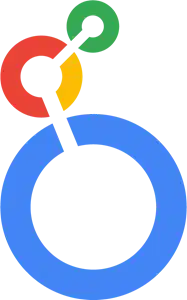
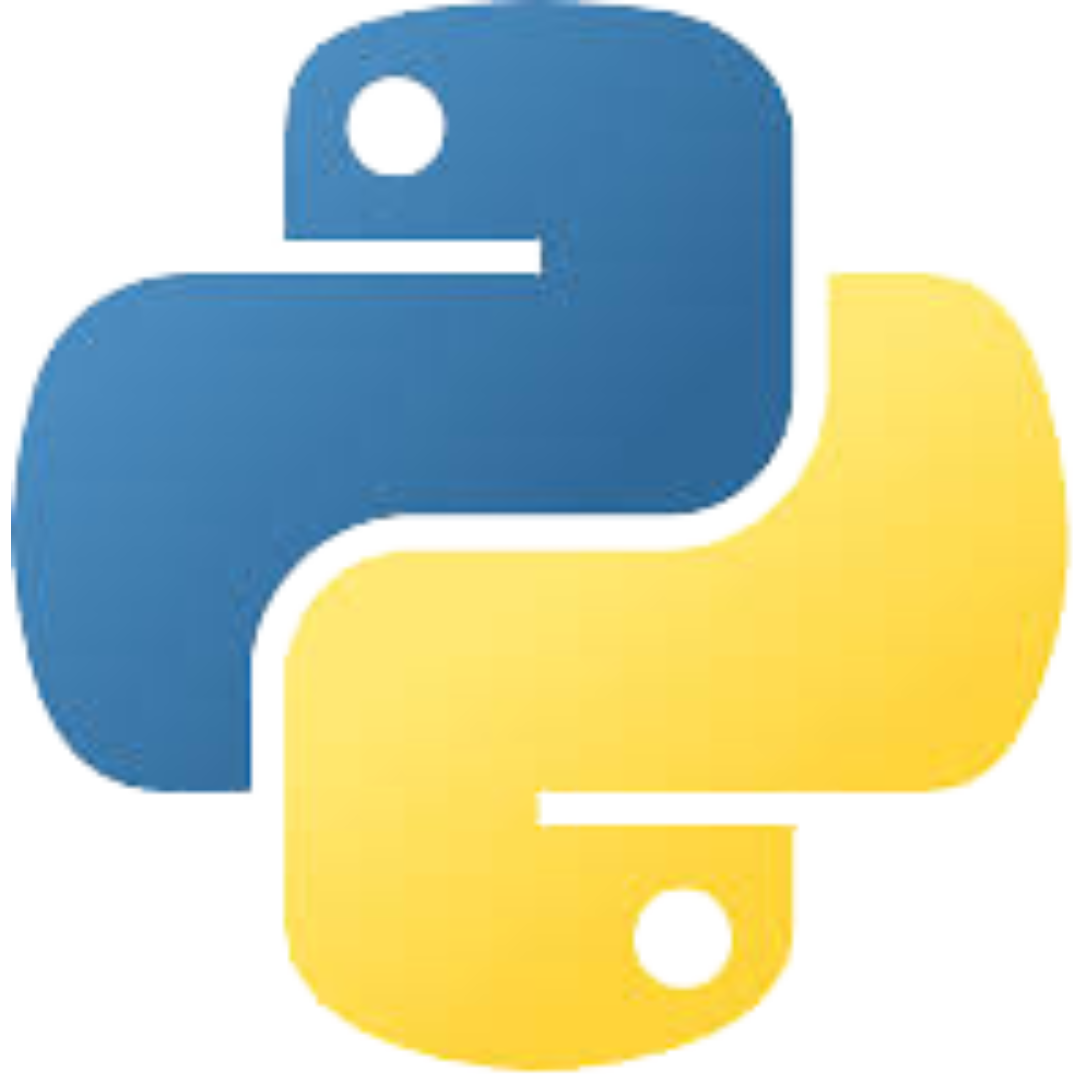
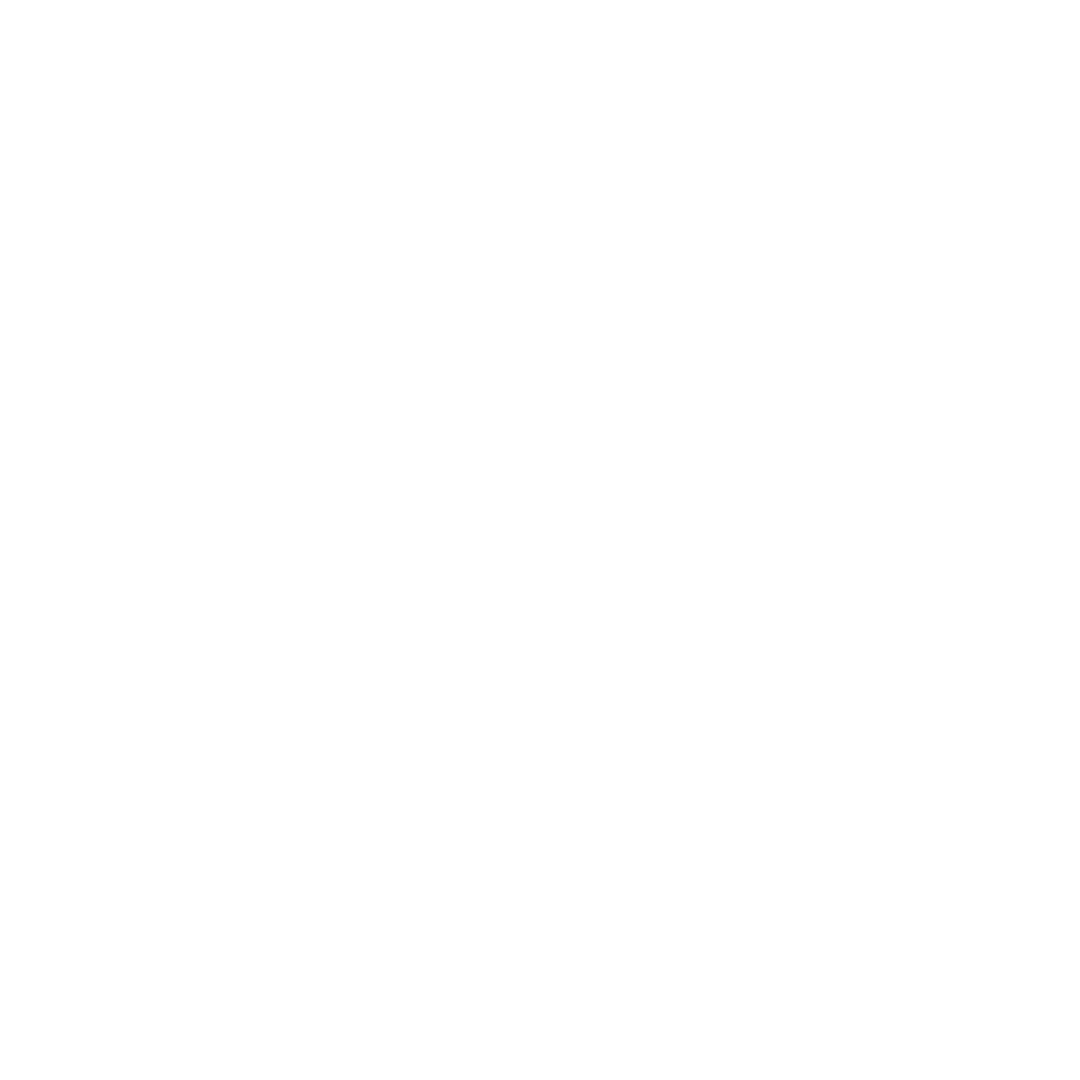
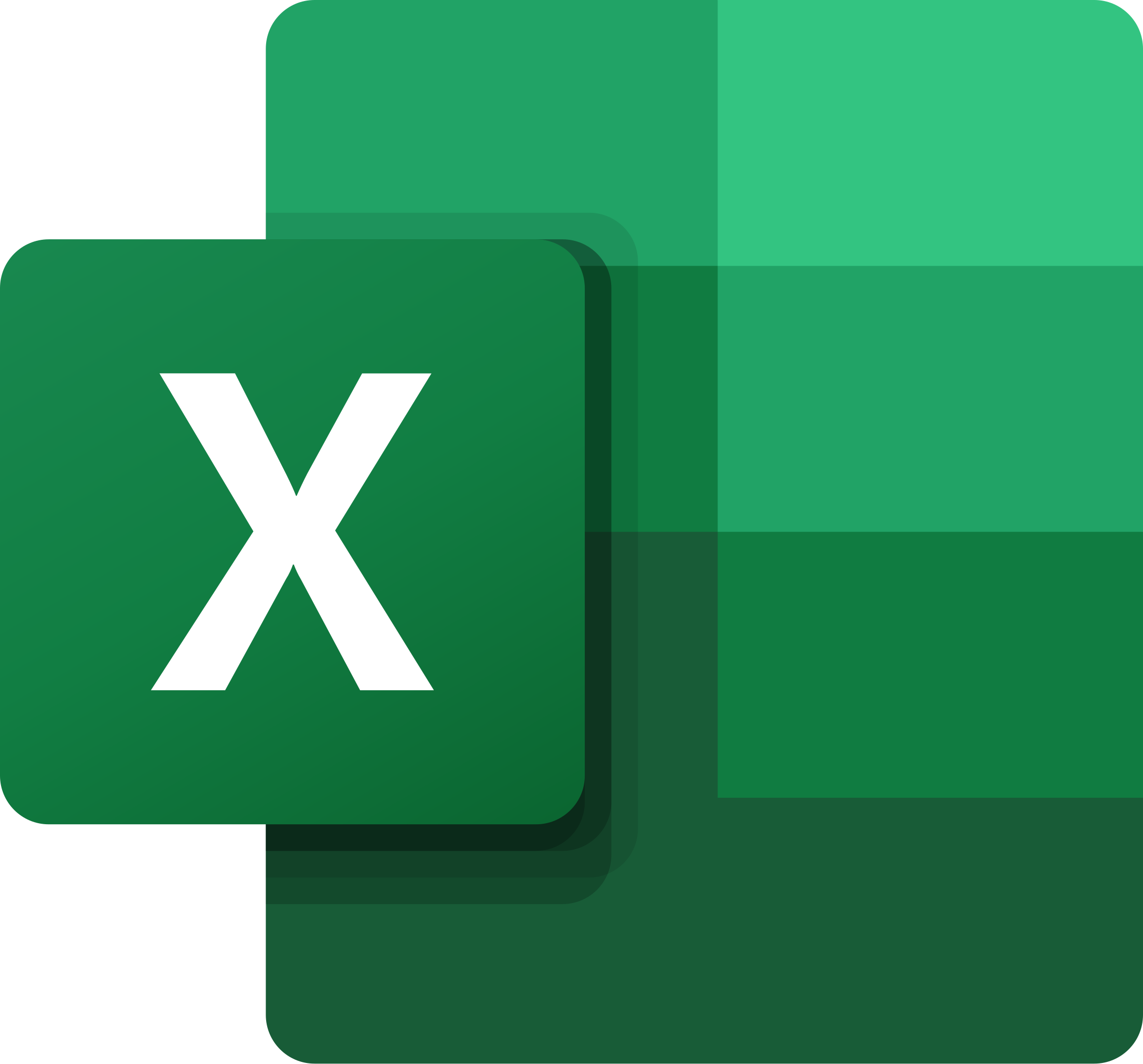
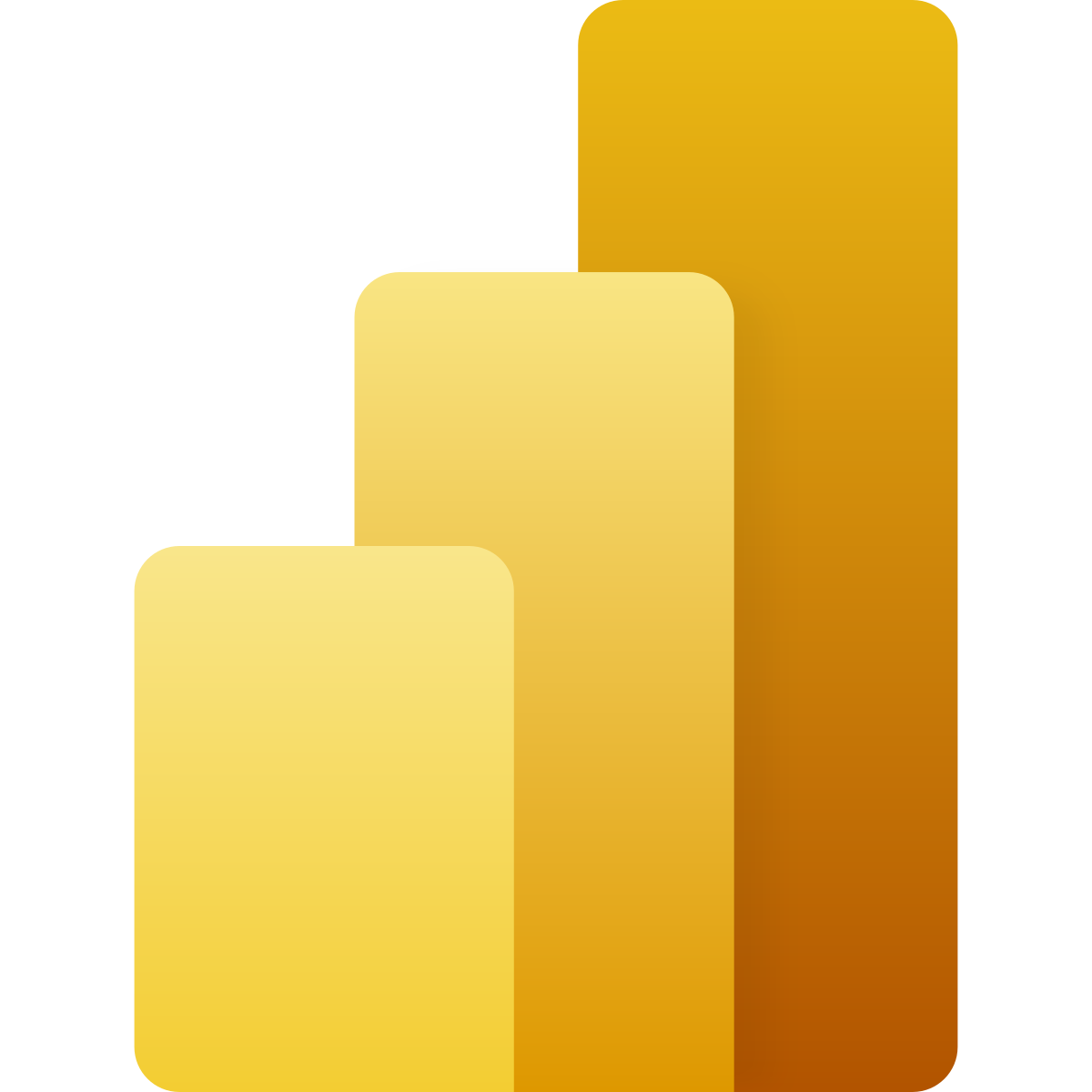
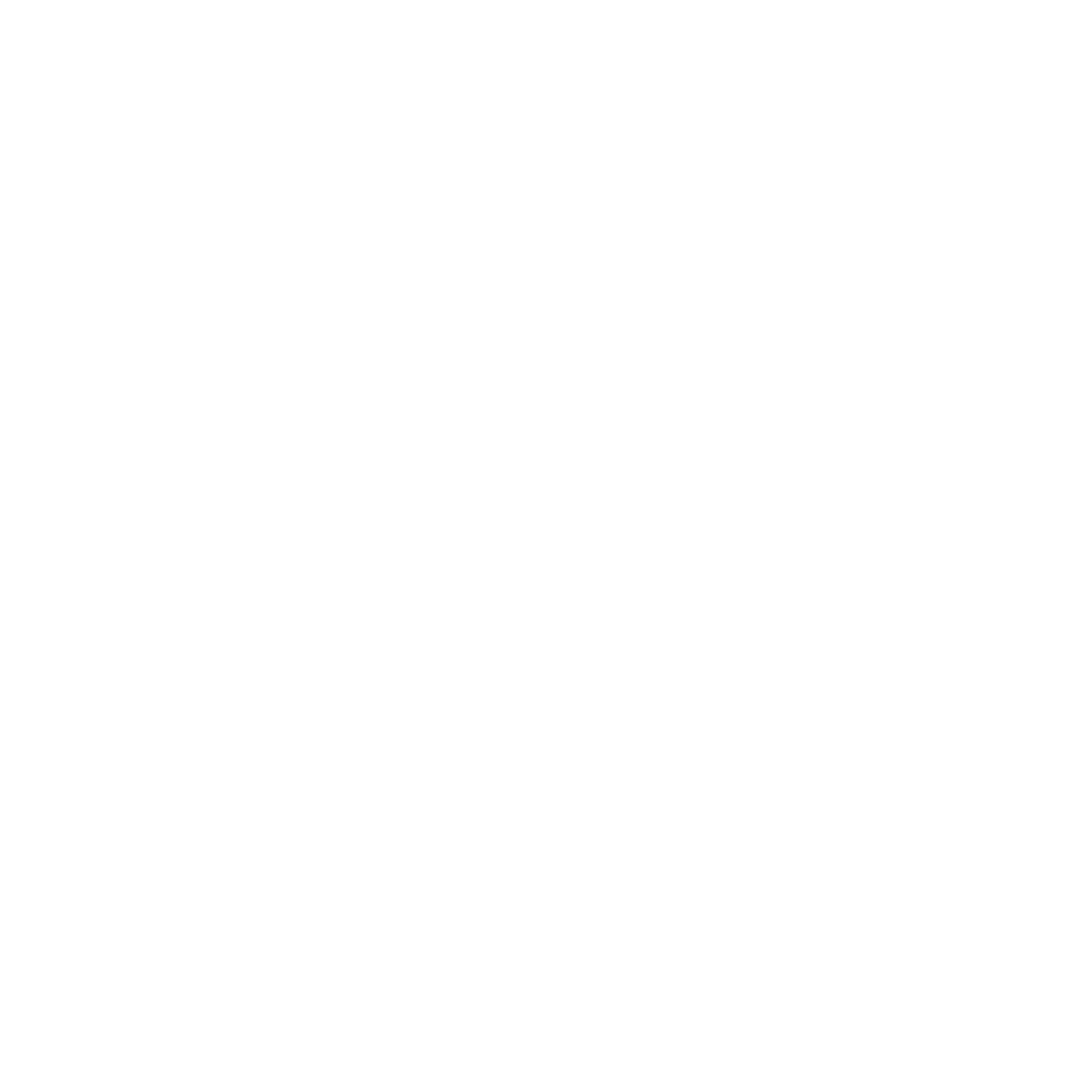
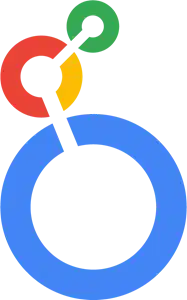
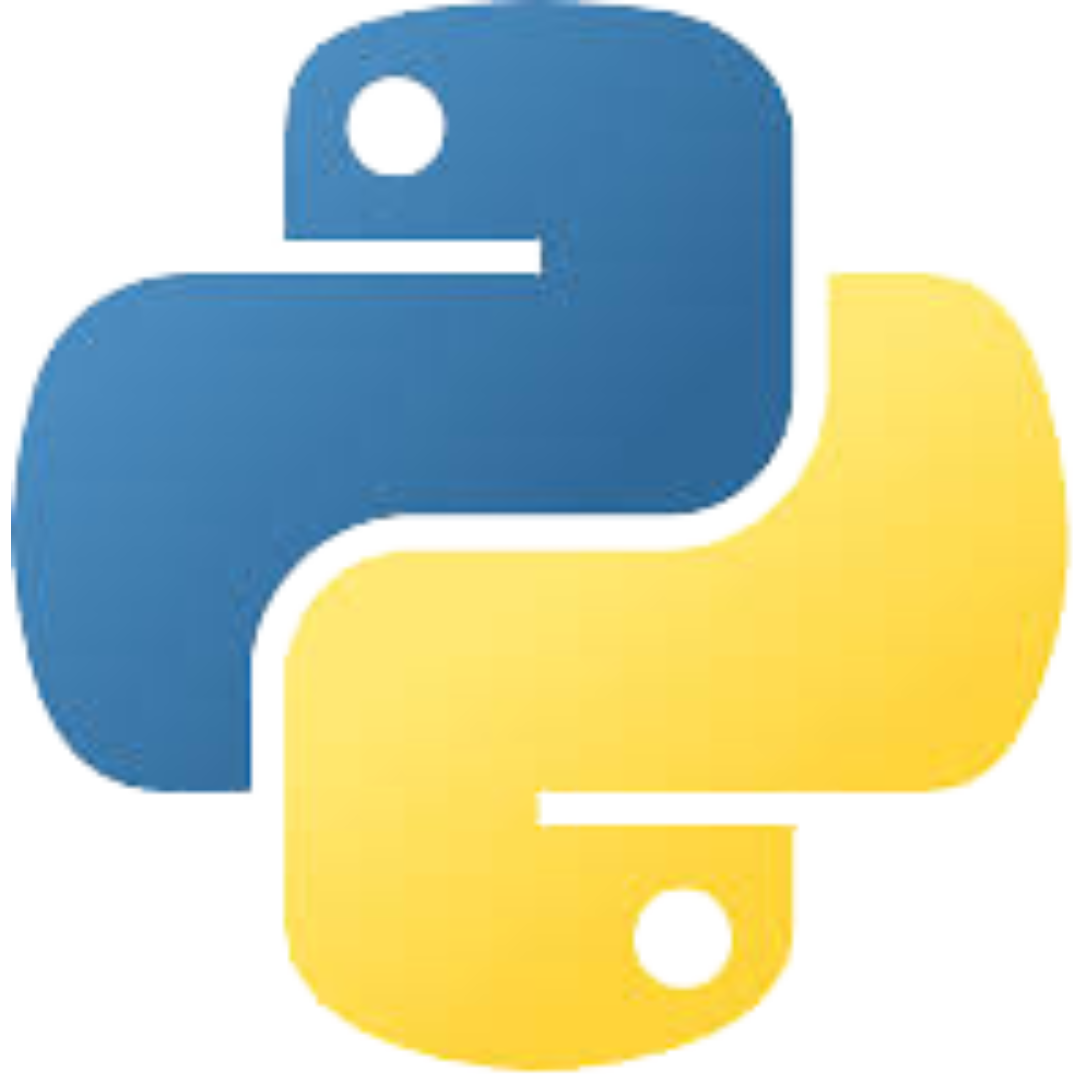
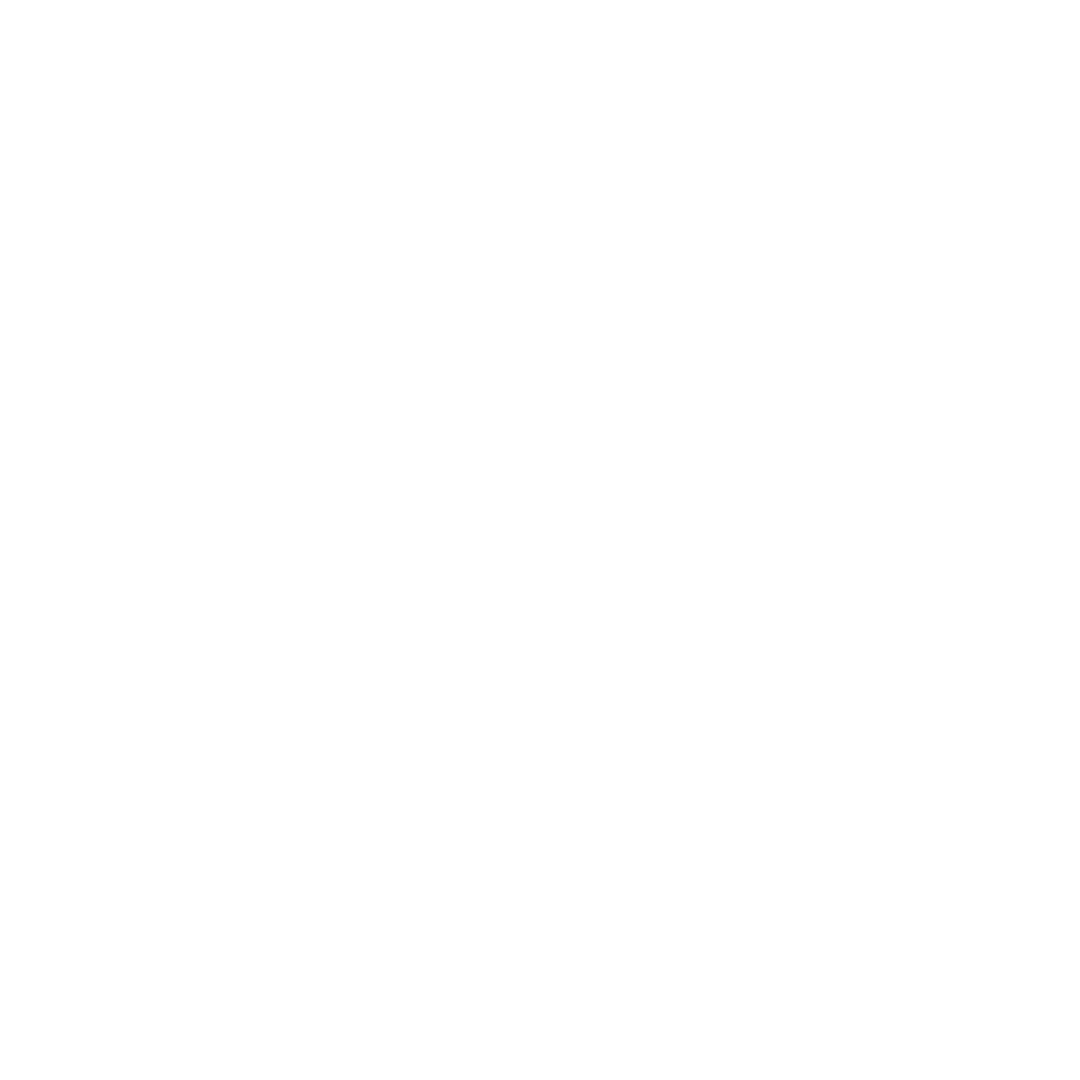
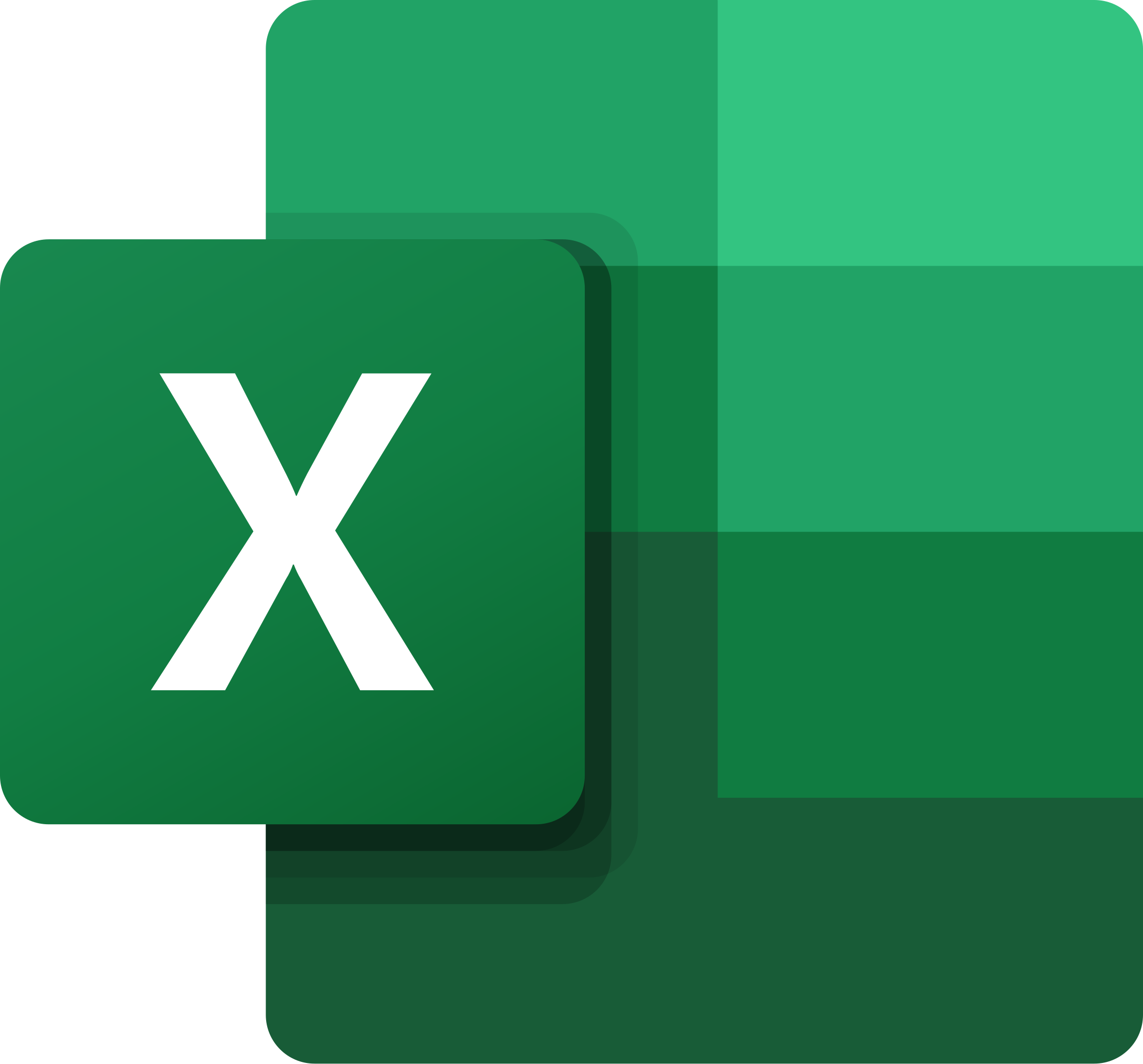
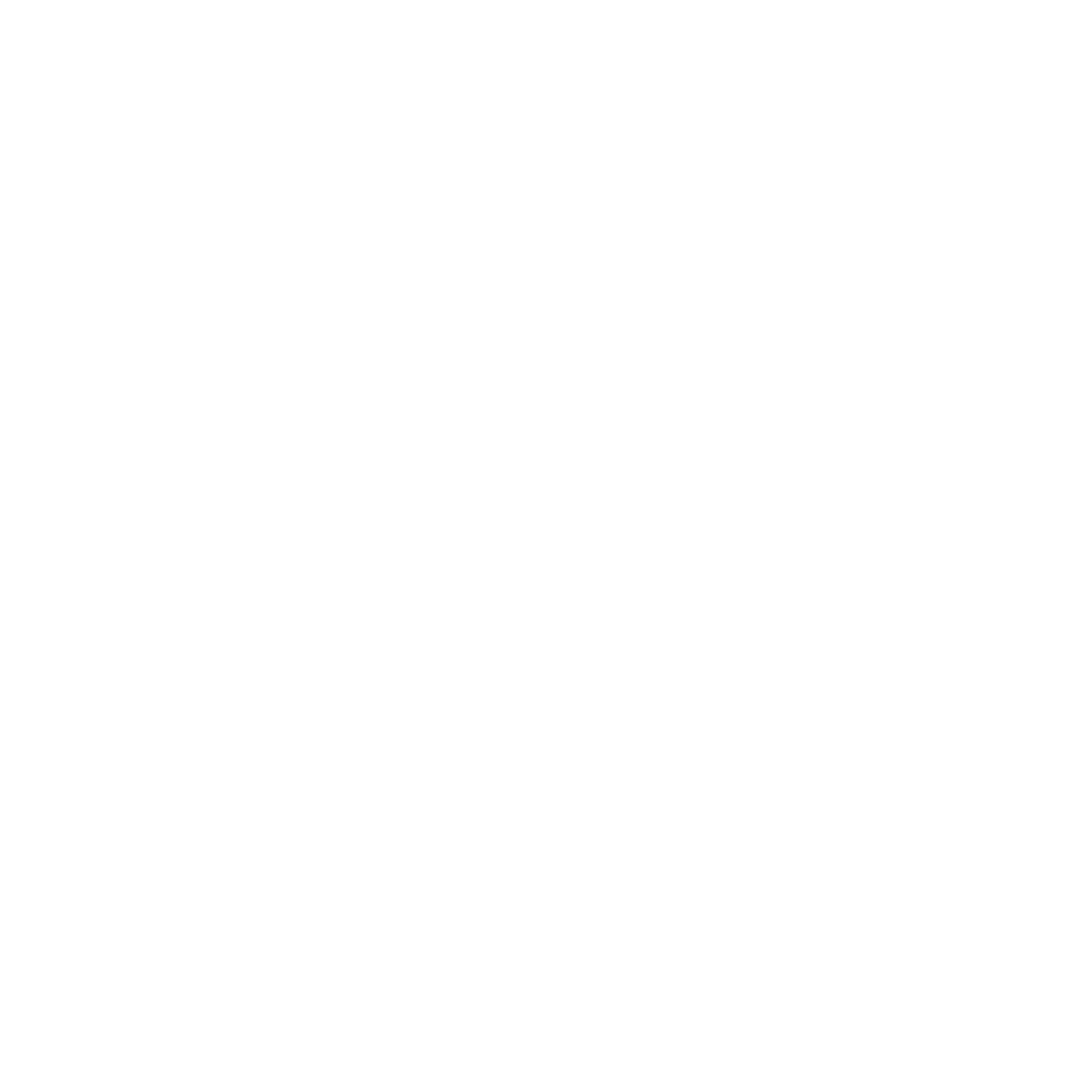
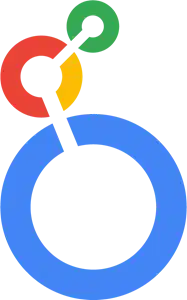
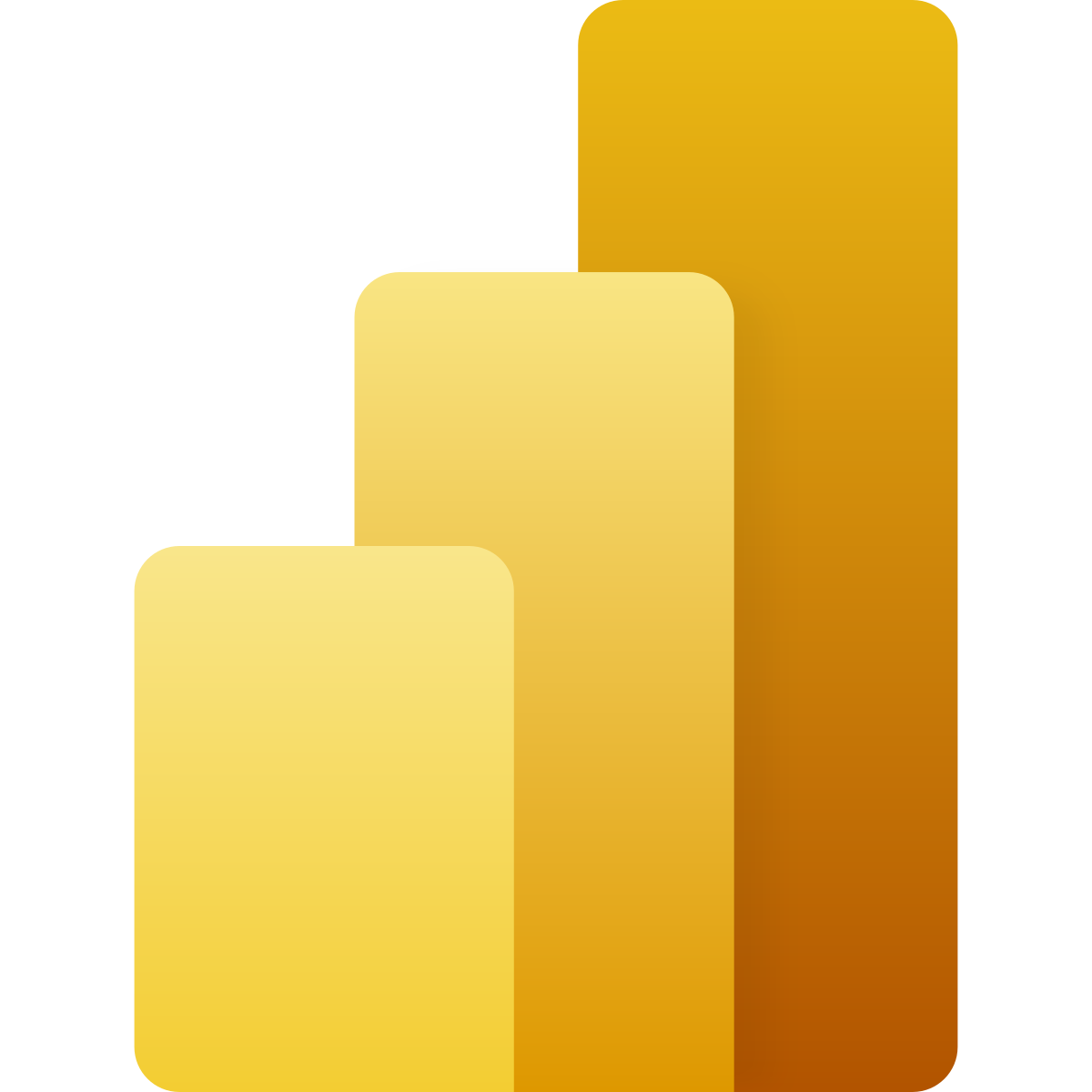
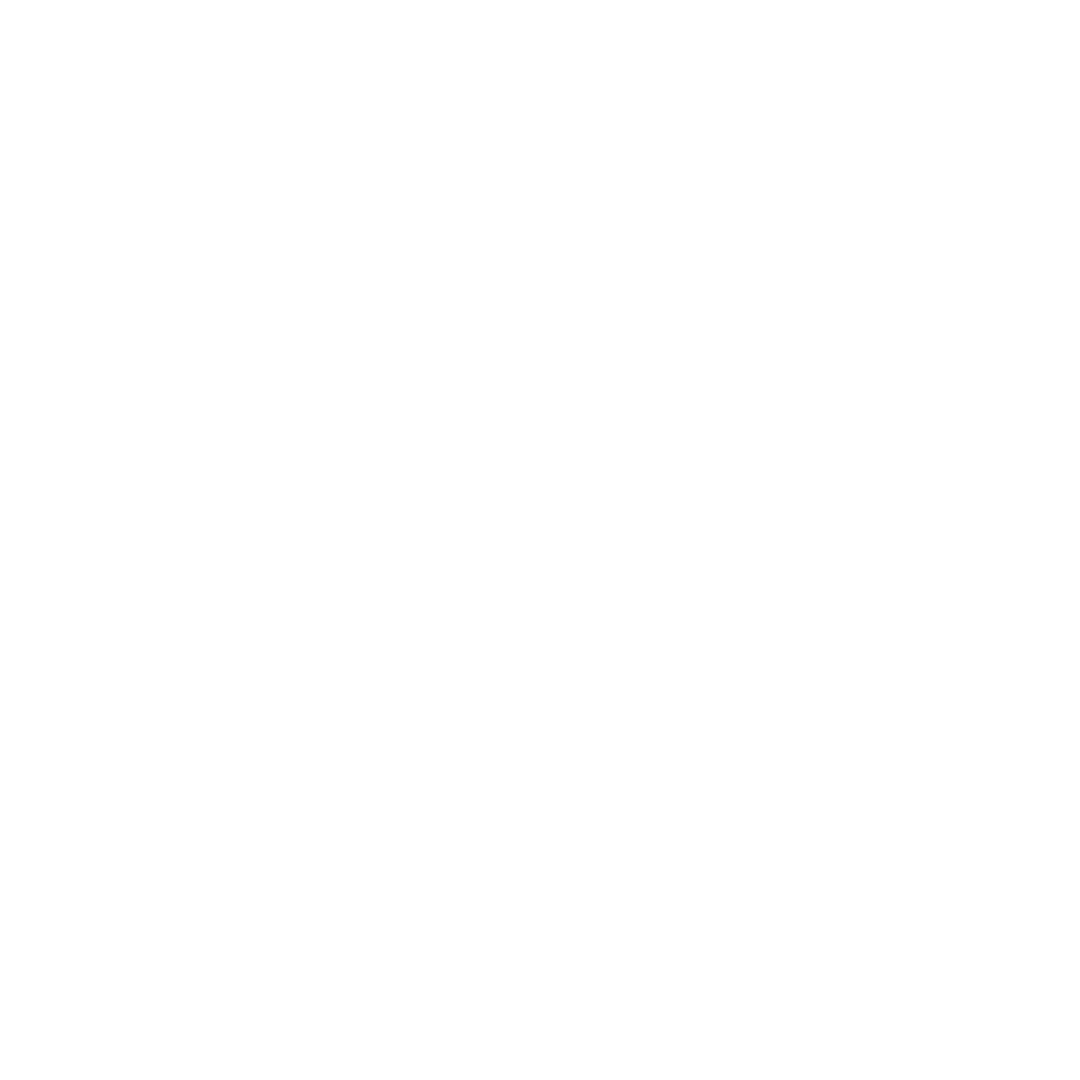
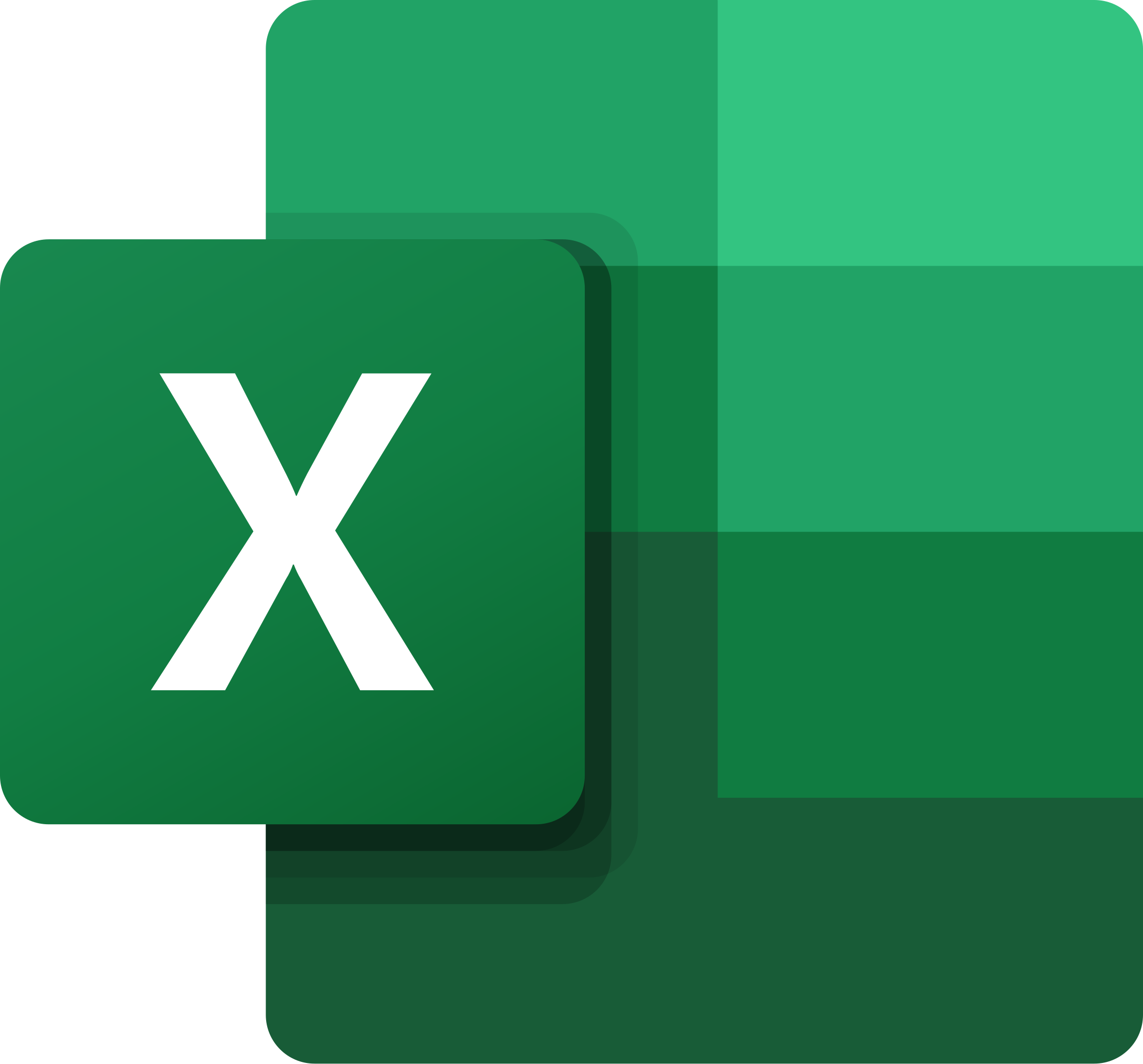
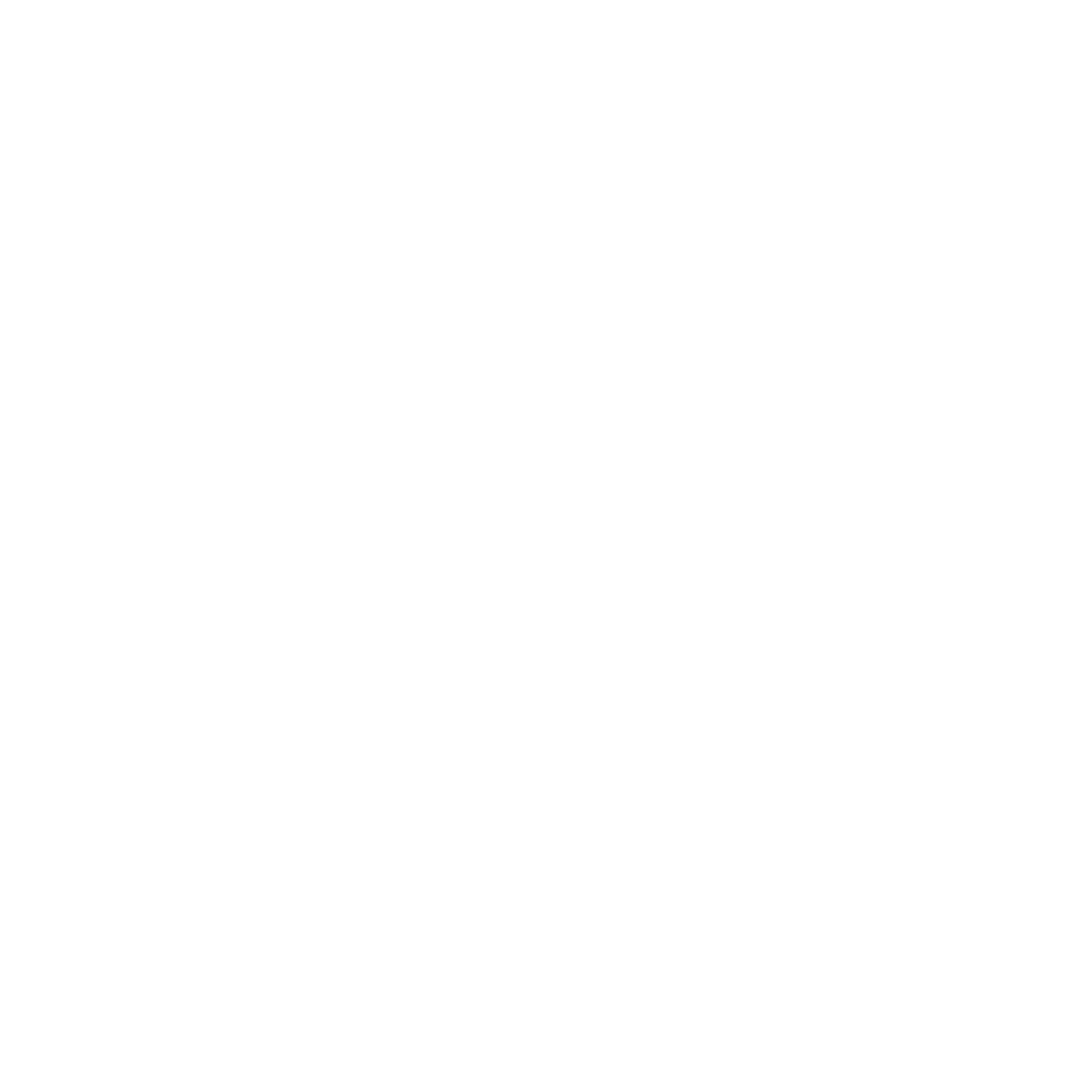
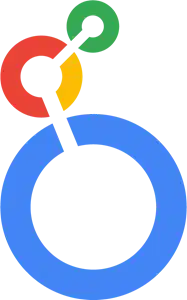
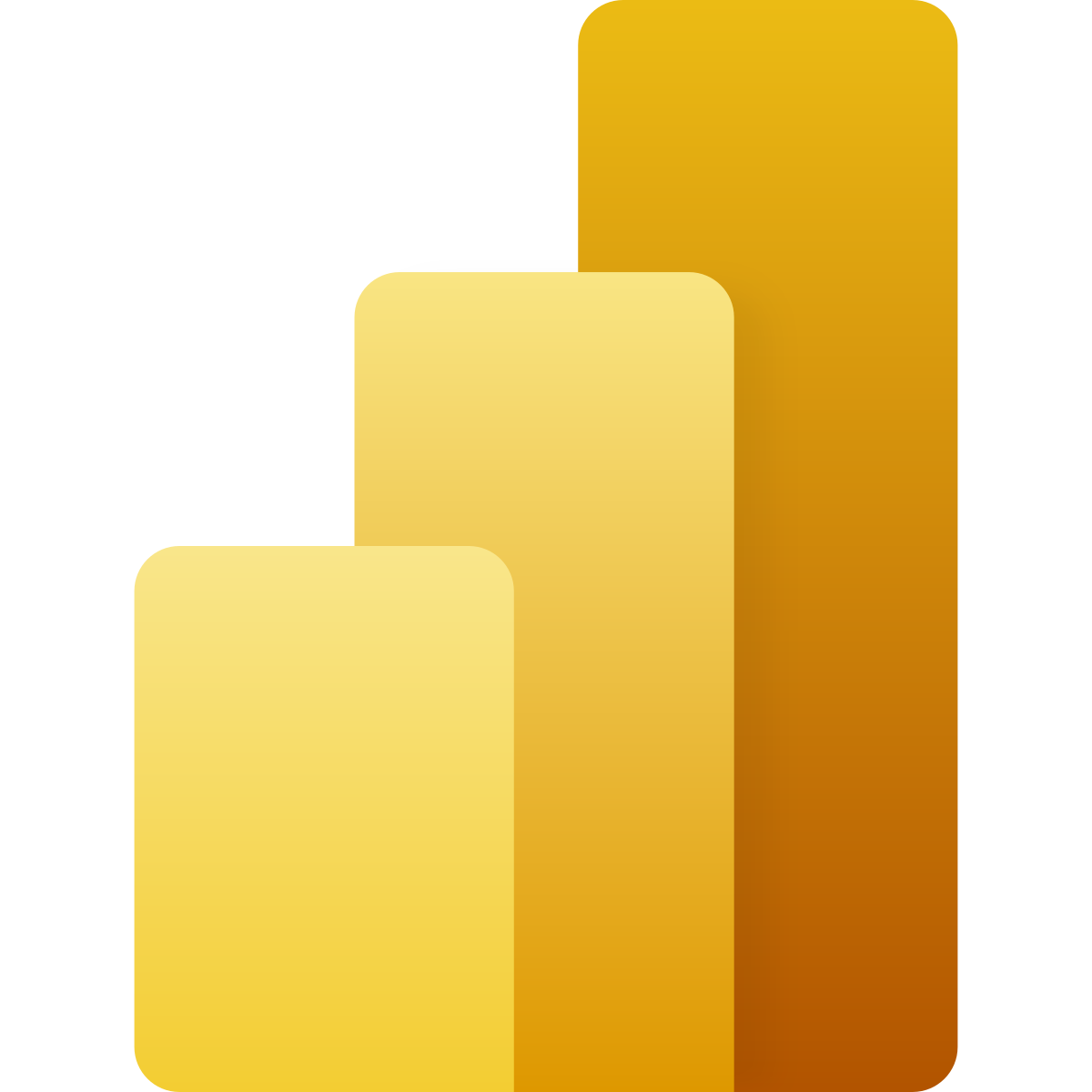
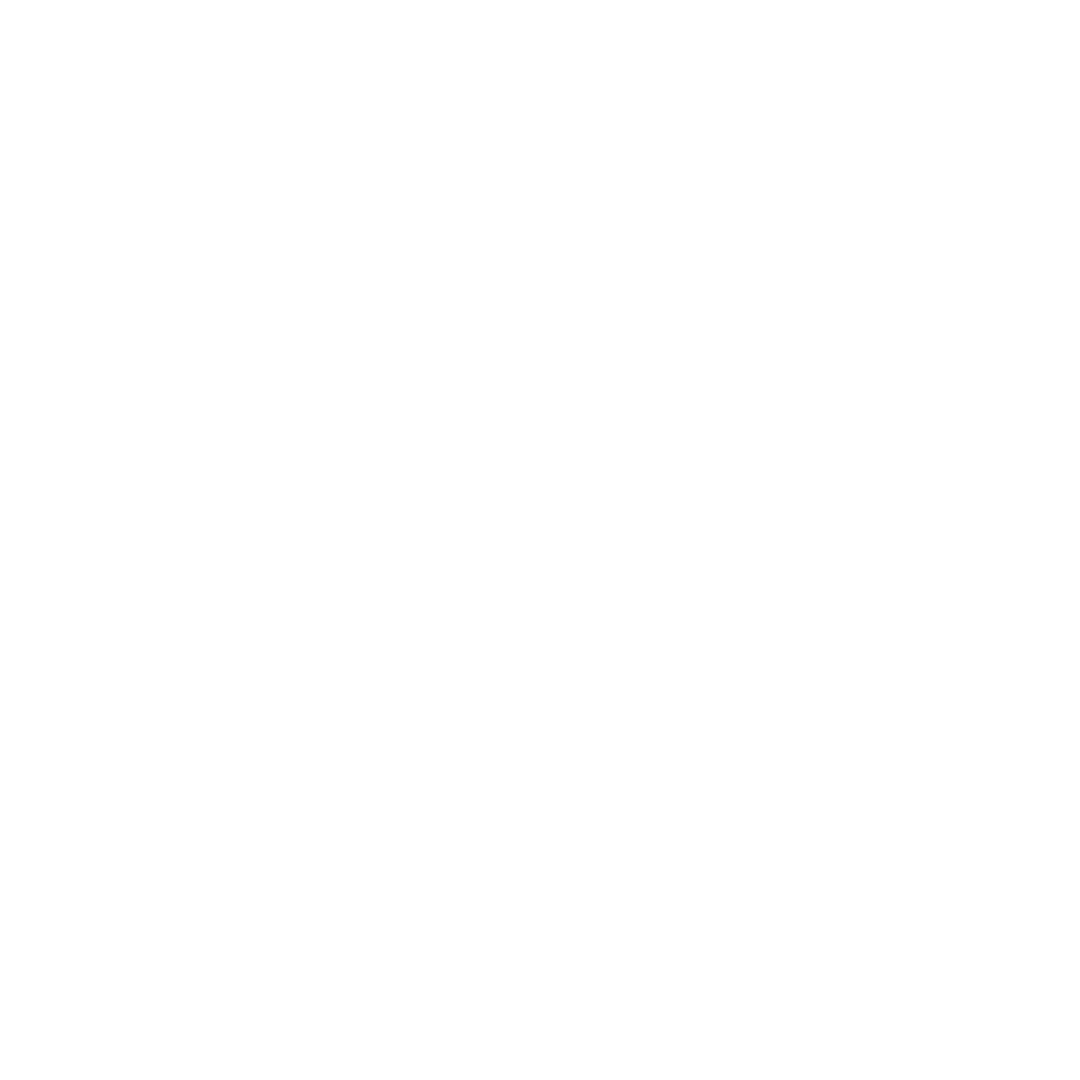
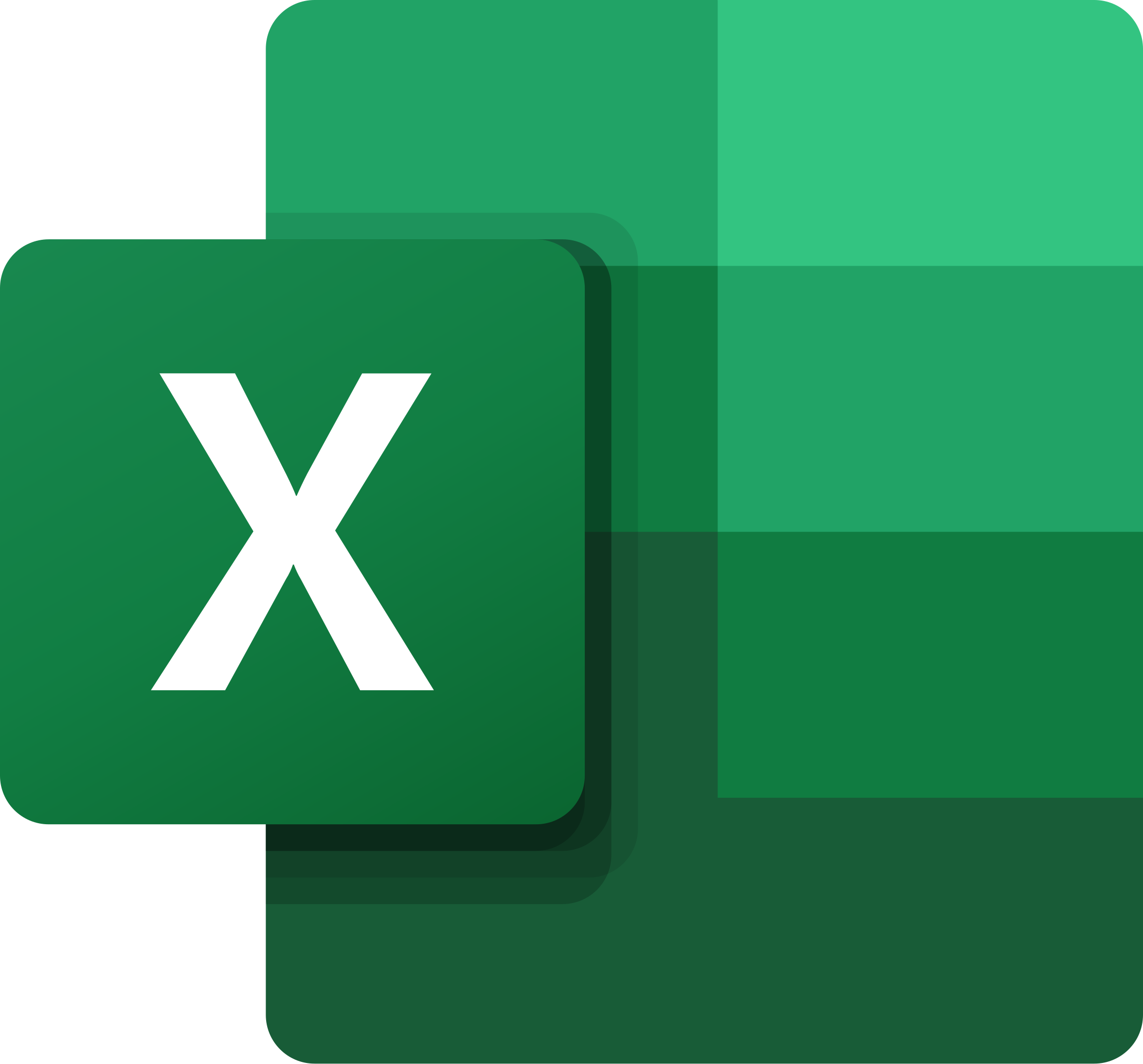
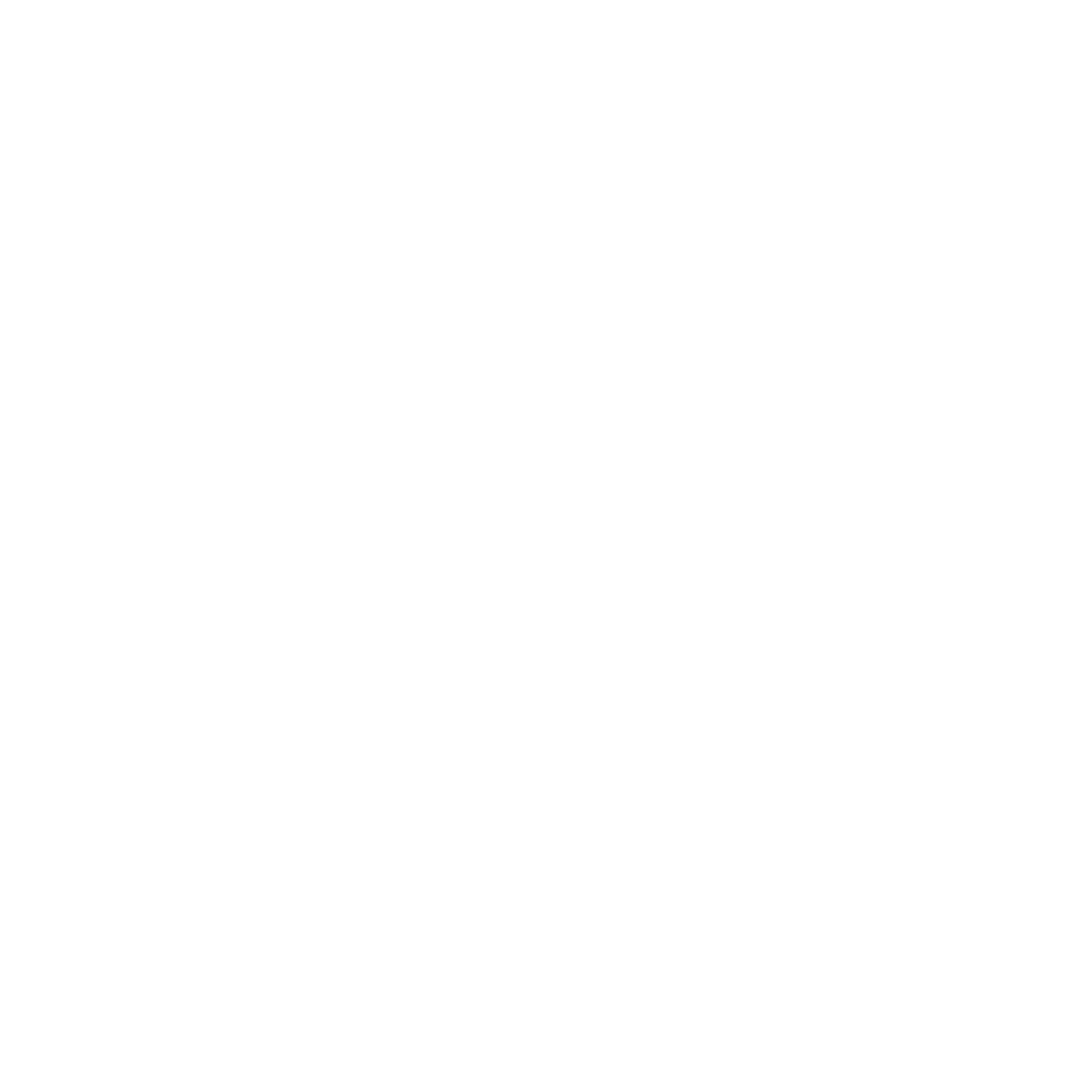
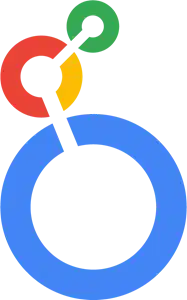
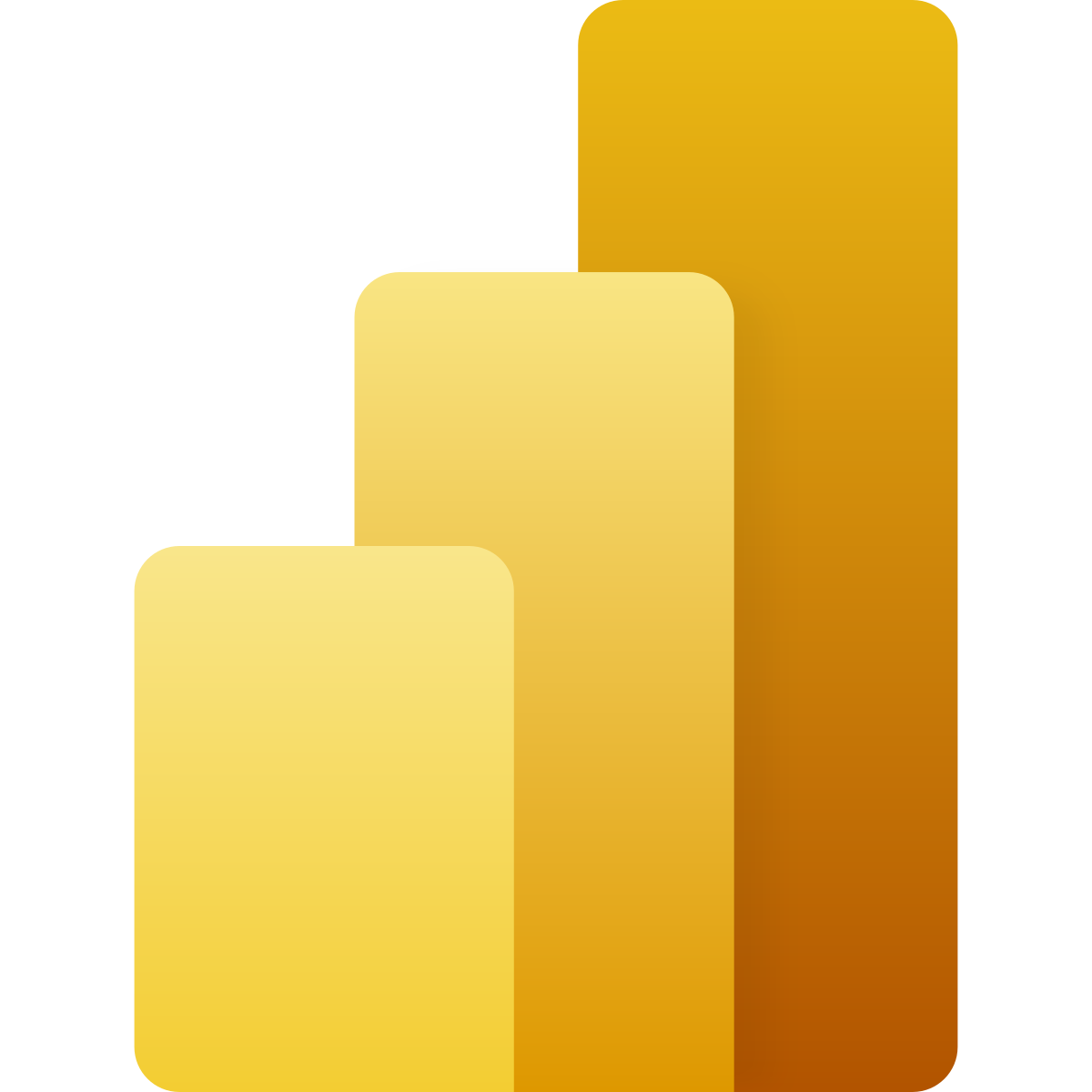
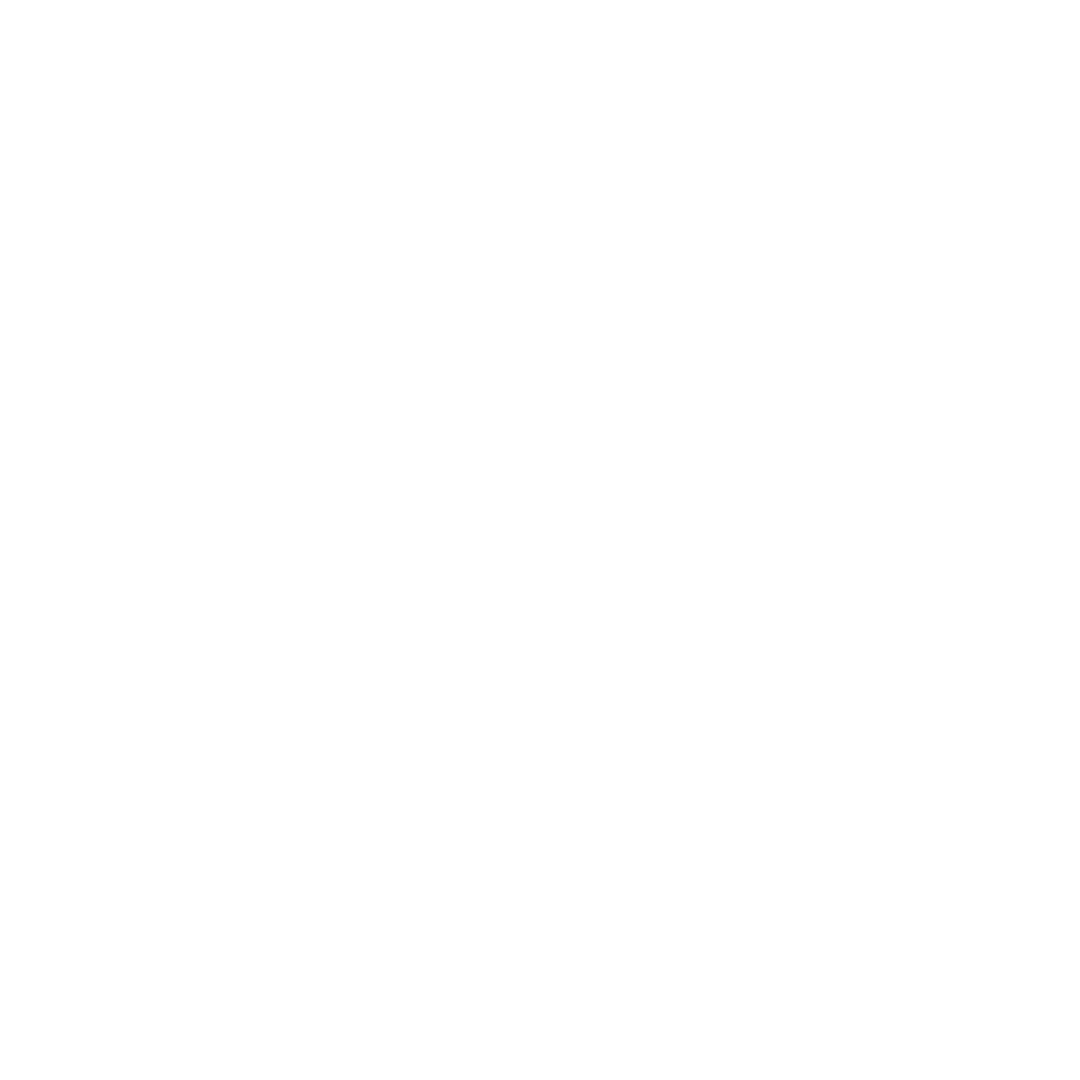
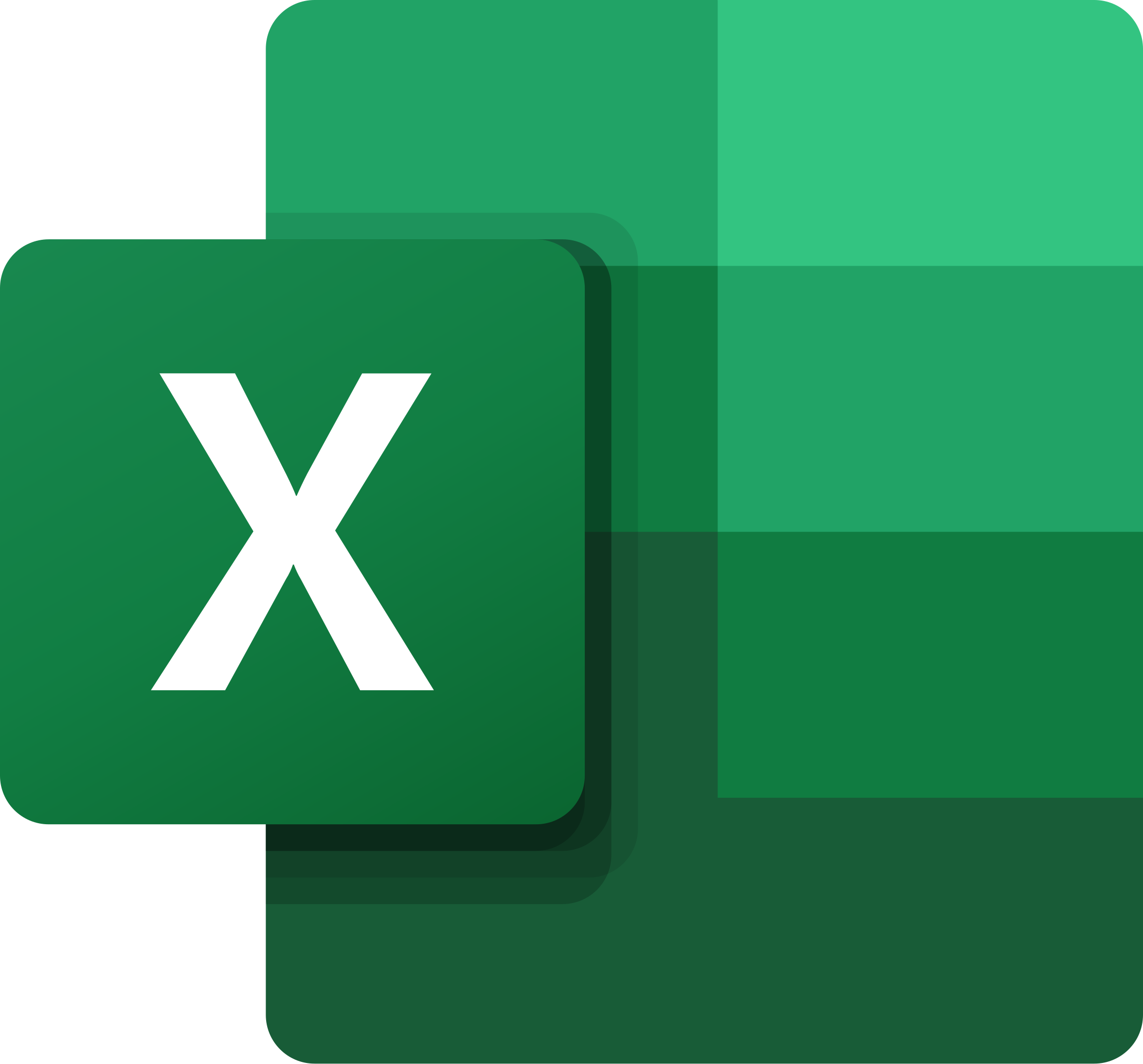
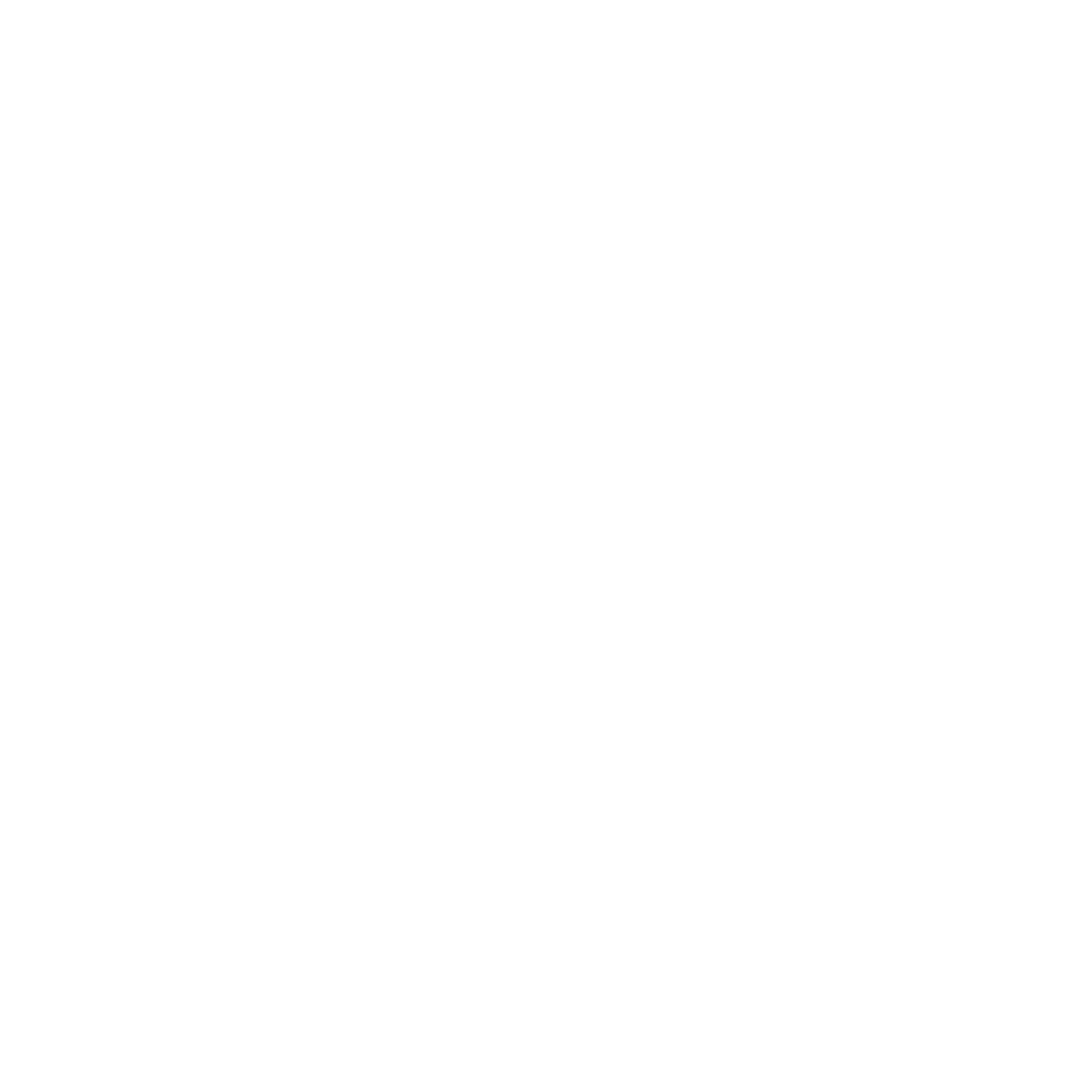
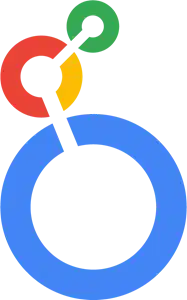
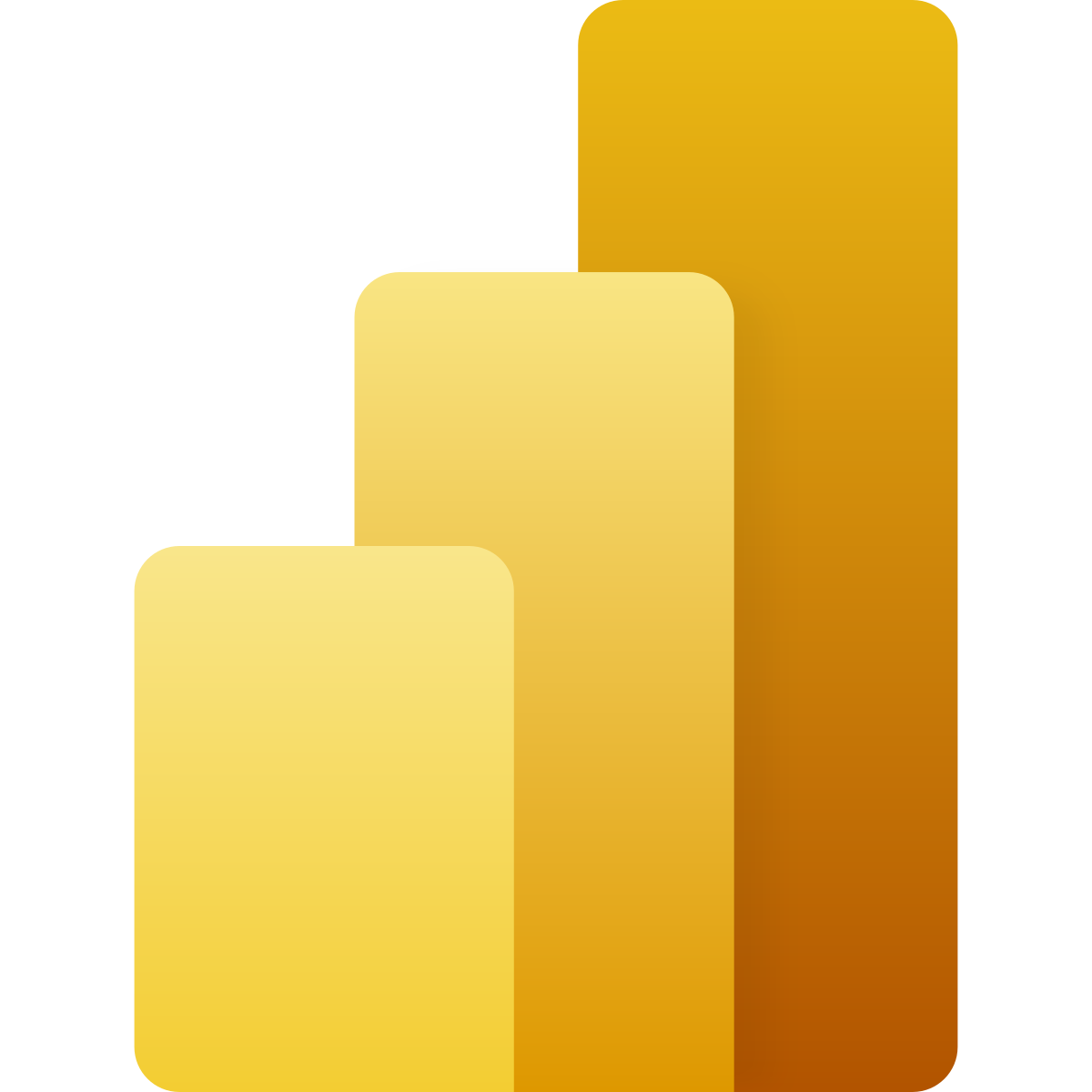
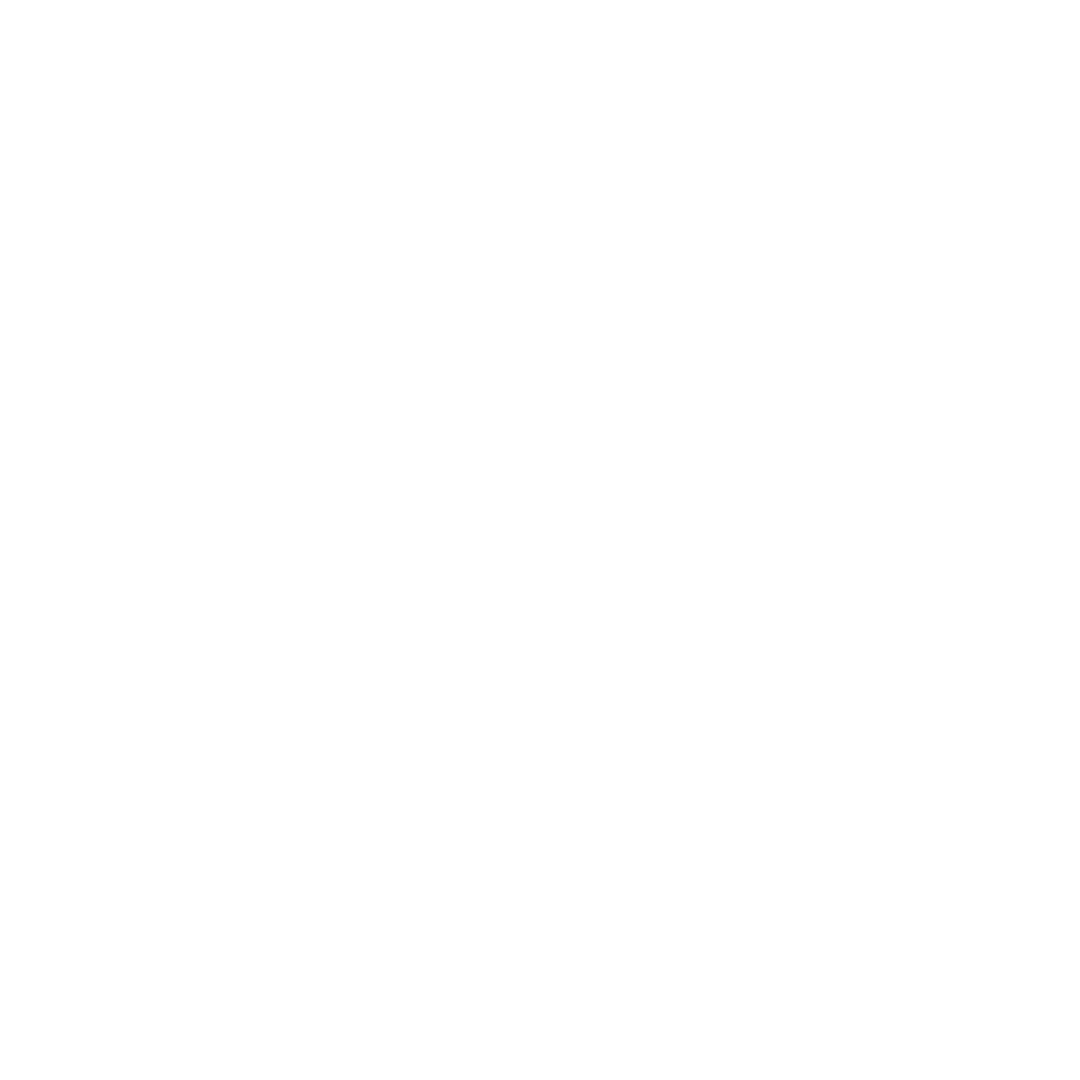
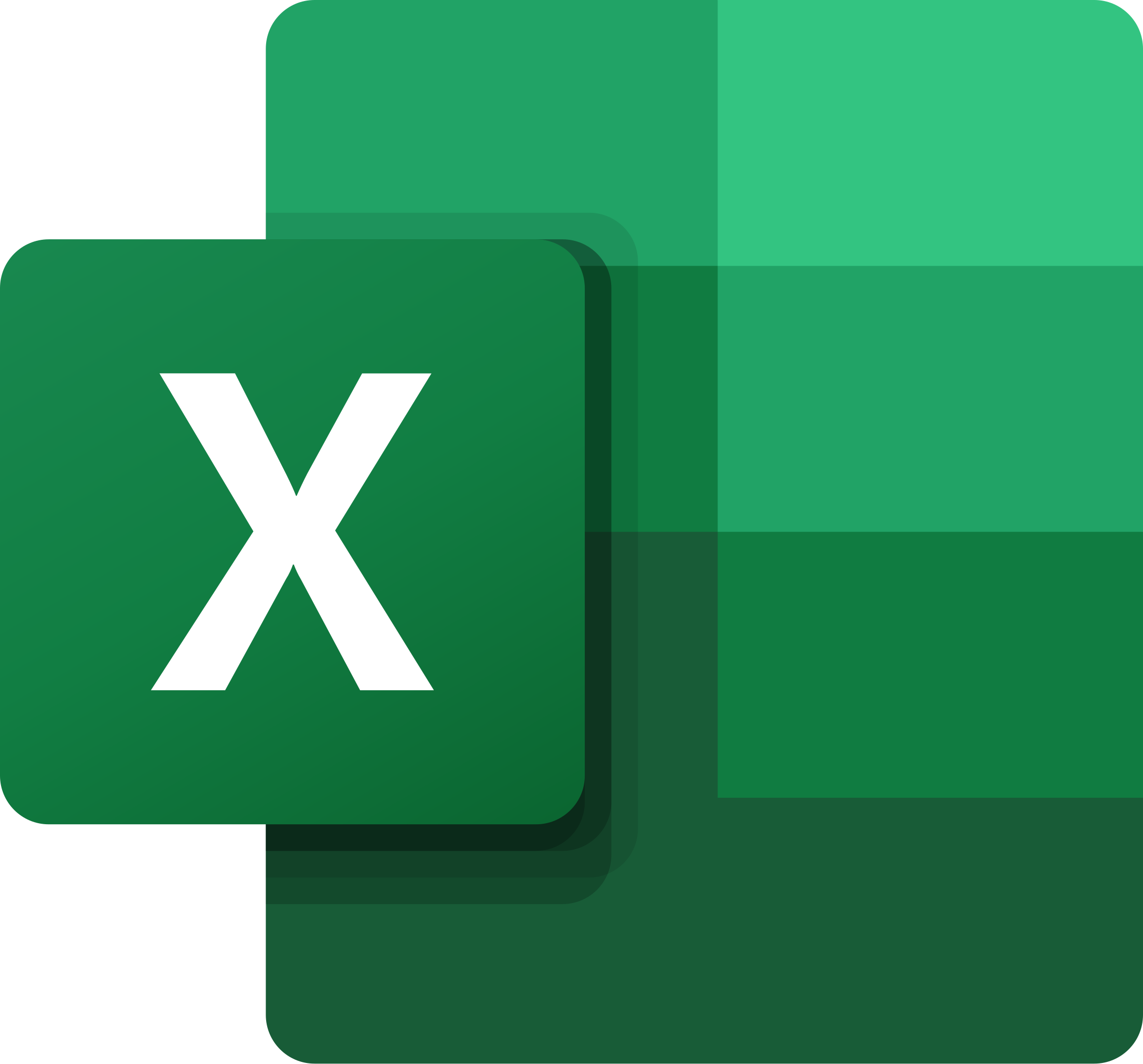
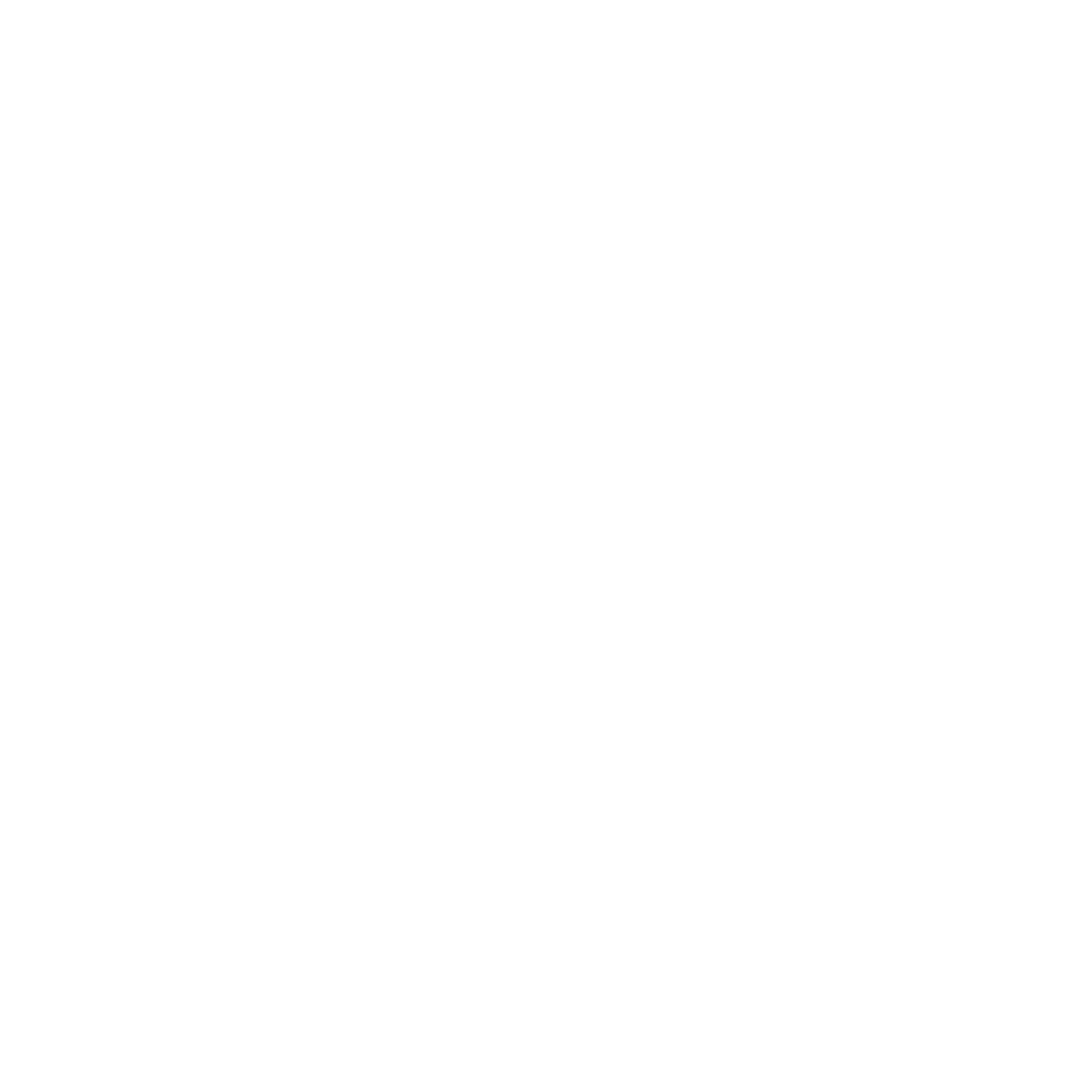
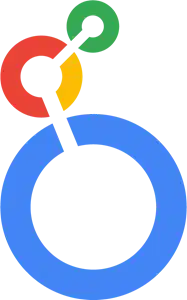
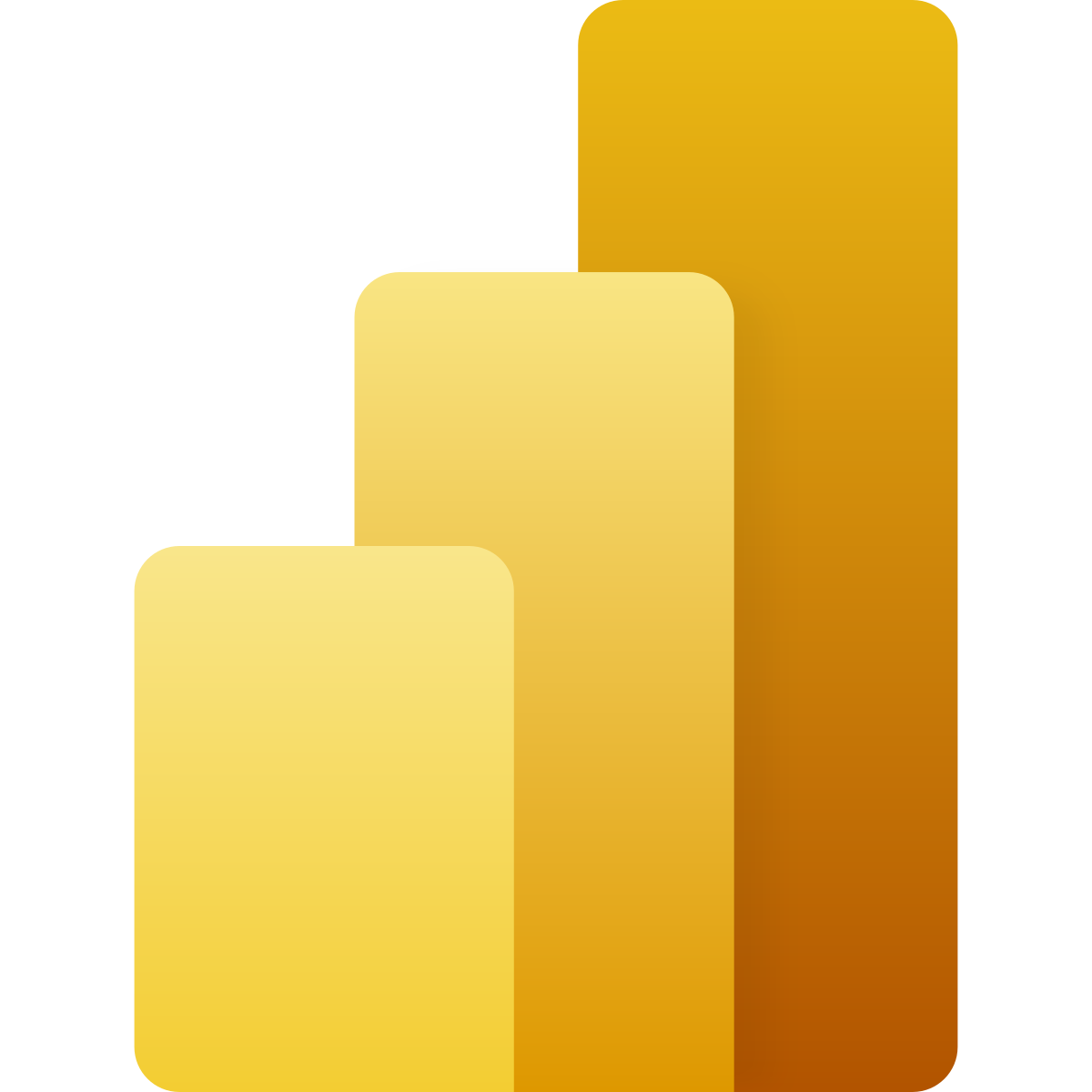
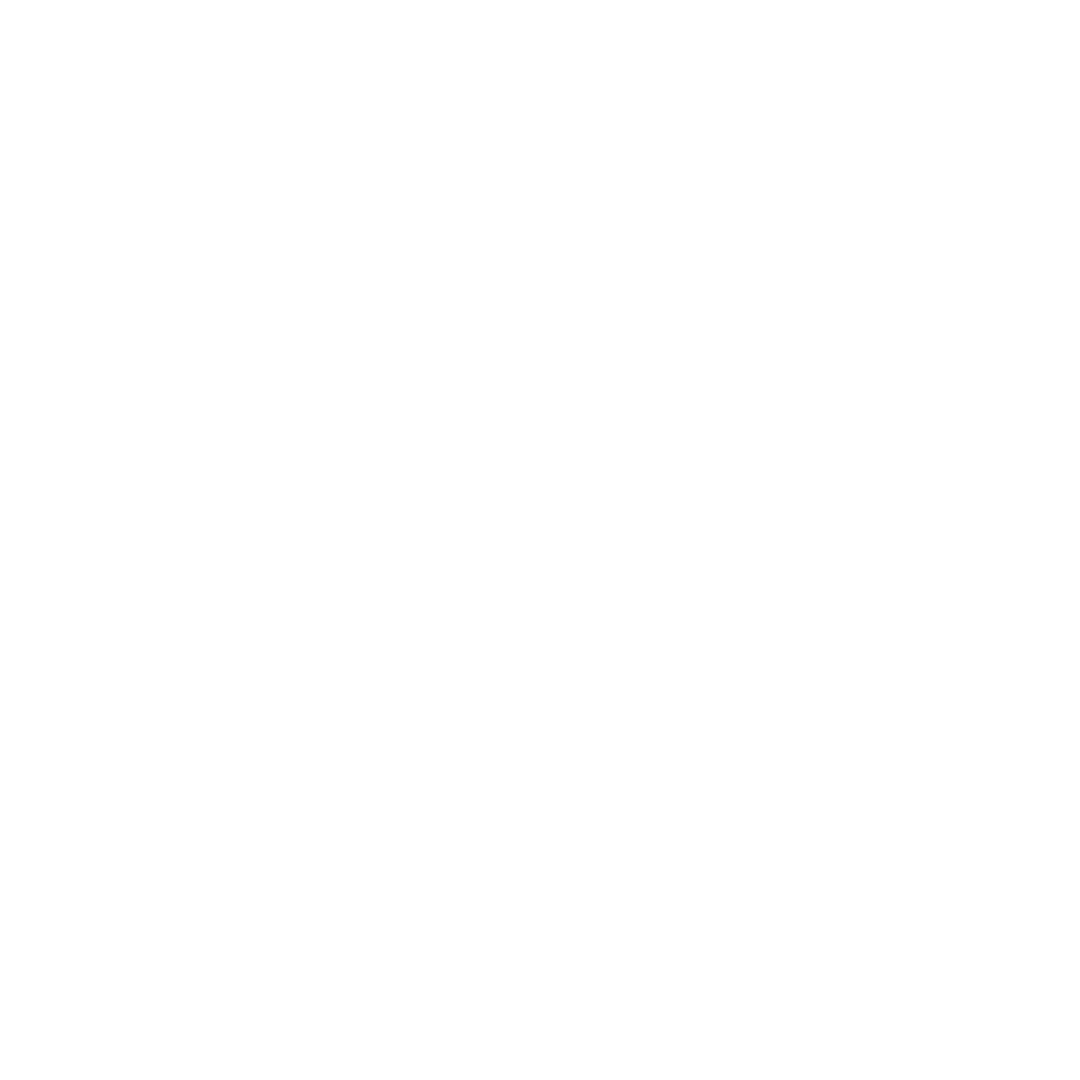
Insight Creation
Modern Analytics for Every Role and Data Stack
Business Intelligence
Empower a business analyst to create universal semantic models that work across BI tools, applications, and machine learning.
Generative AI
Eliminate the need for data wrangling while driving consistency and accuracy for building machine learning models and text-to-SQL applications.
Learn More
Analytical Applications
Deliver analytics-powered applications effortlessly with consistent metrics and ultra-fast queries.
Learn More
Data Modeling
Foster collaboration across data engineers and business analysts with a unified code and no-code experience.
Learn More
See AtScale In Action
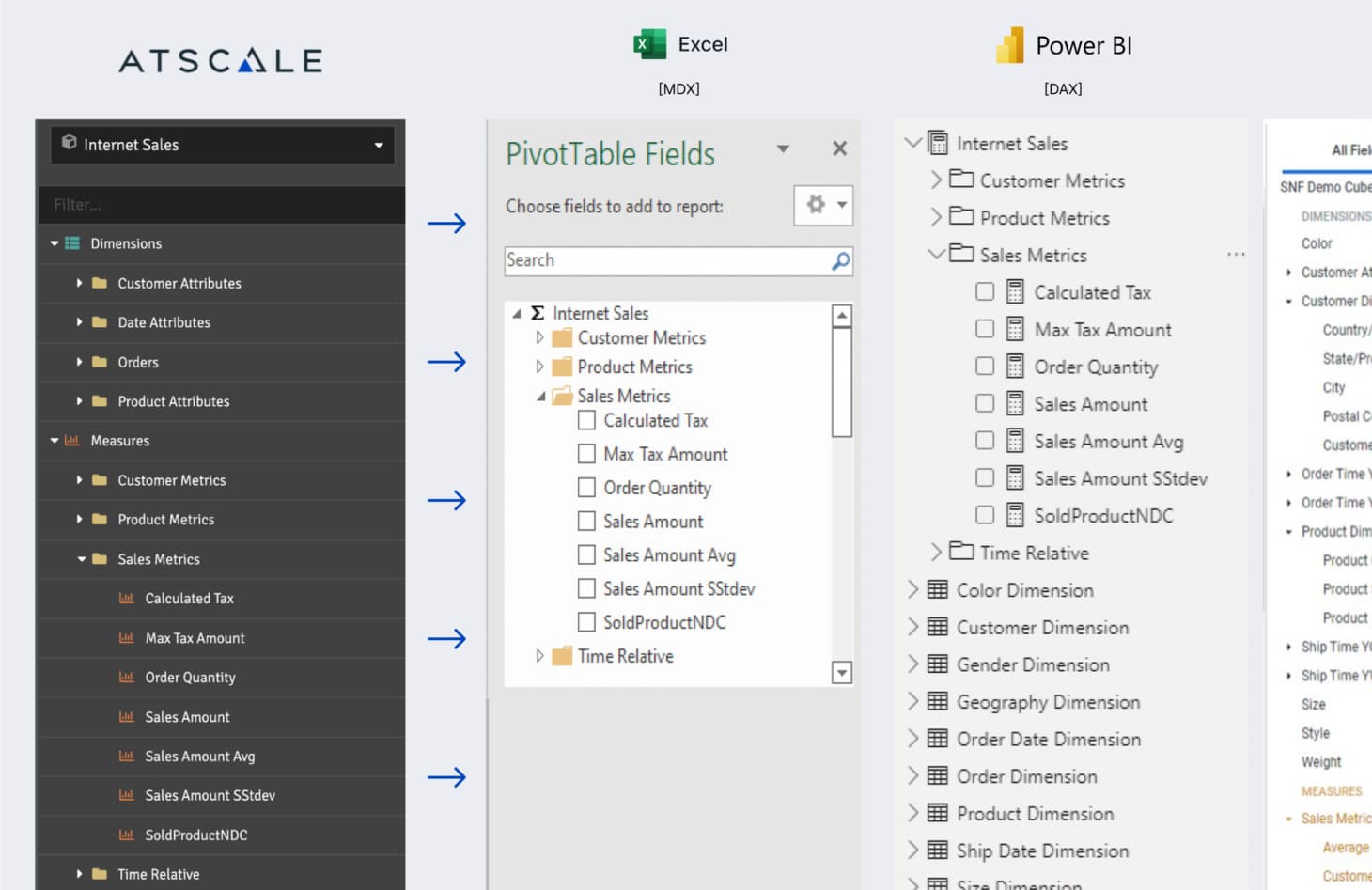
The Universal Semantic Layer for Enterprises
Independent, flexible, and scalable: Empower teams with secure, live data access, robust data modeling, and unmatched compatibility across tools and protocols.

Independent and Tool-Agnostic
Serves as an independent layer not tied to a single consumption style or analytics tool.
Learn More

Live Data Access Without Movement
Supports direct access to live data without data movement or preprocessing
Learn More

Support Multiple Modeling Personas
Fosters collaboration between business analysts and data engineers with code or no-code solutions.
Learn More

Multi-Dimensional Business Logic
Supports multi-dimensional (OLAP) expressions for encoding complex business concepts and functions.
Learn More

Speed-of-Thought Query Performance
Delivers “speed of thought” queries using automated query performance tuning and caching.
Learn More

Supports Text-to-SQL Natural Language Query
Integrates with LLMs to deliver business context for GenAI
Learn More
Unify Your Data Ecosystem
Multi-protocol deep integrations that support SQL, MDX, DAX, Python, and REST protocols, integrating seamlessly with various BI tools or visualization platforms. Learn More -->
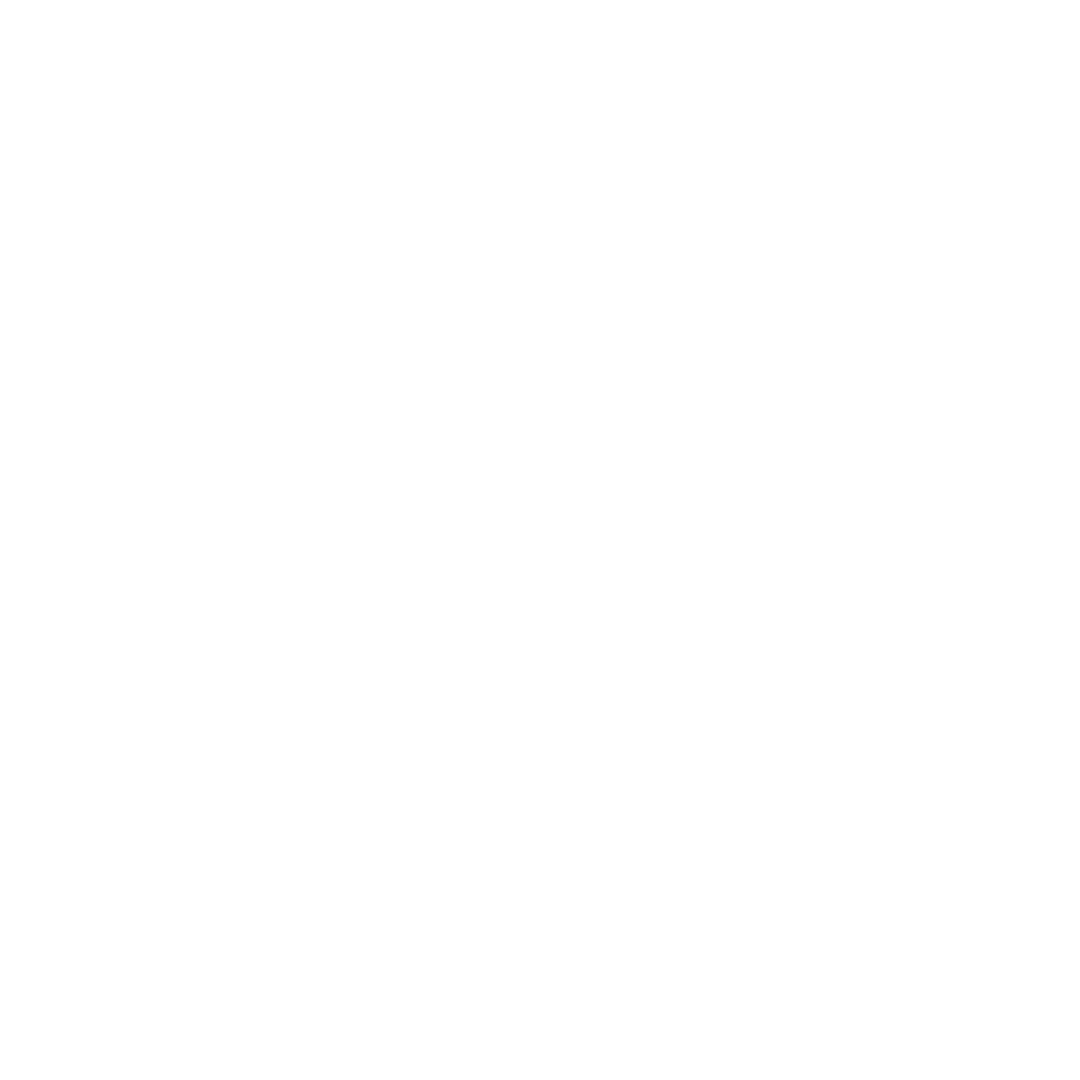
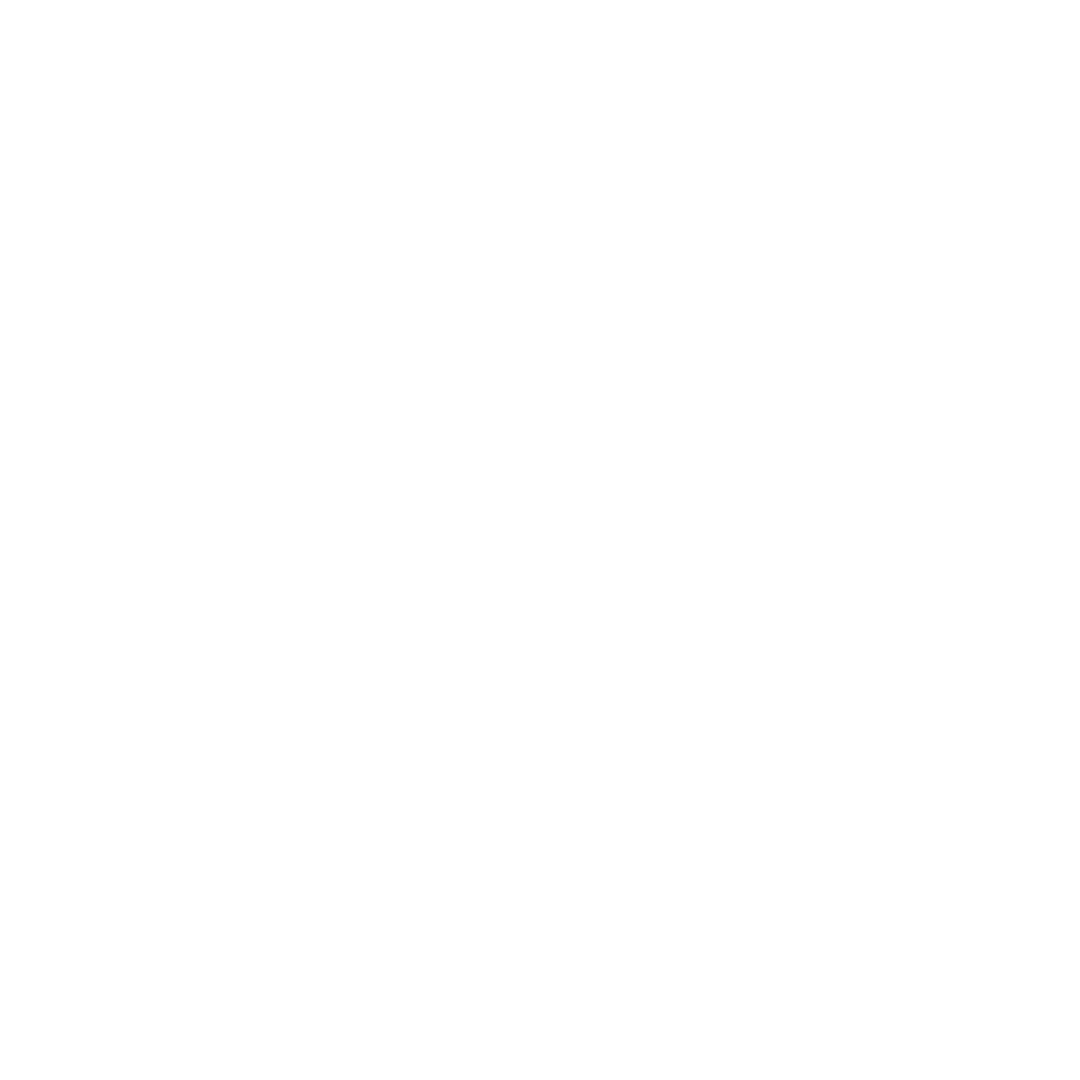
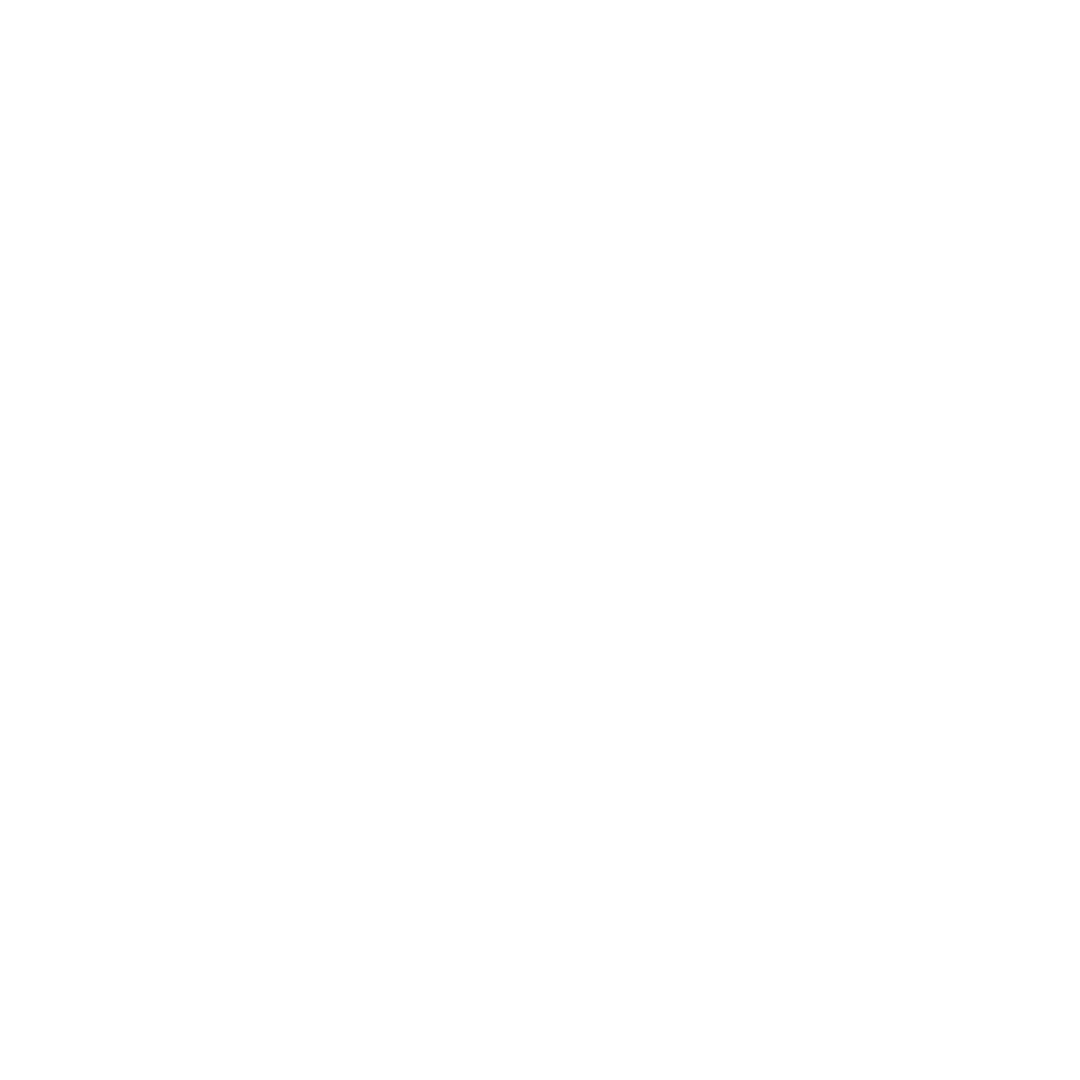
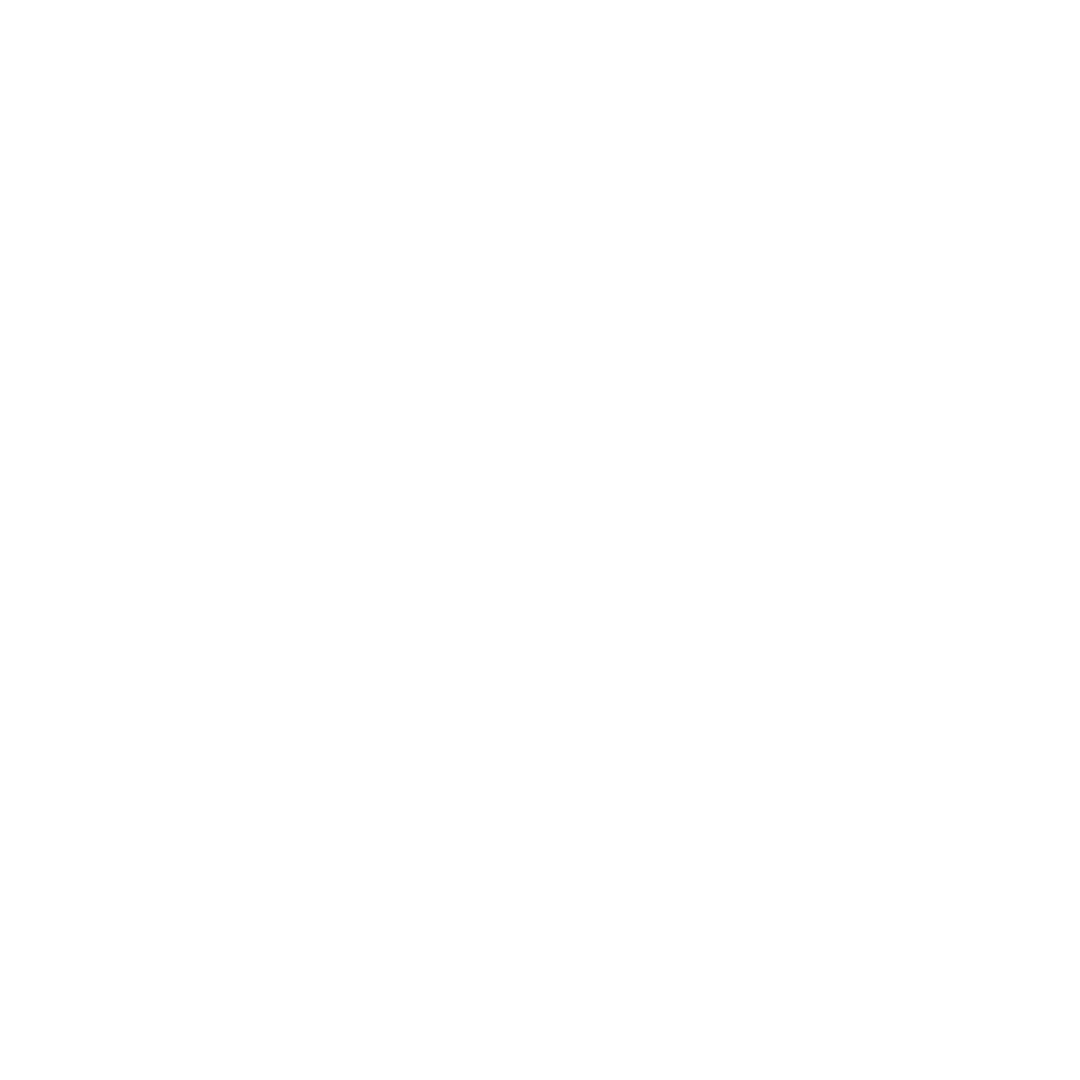
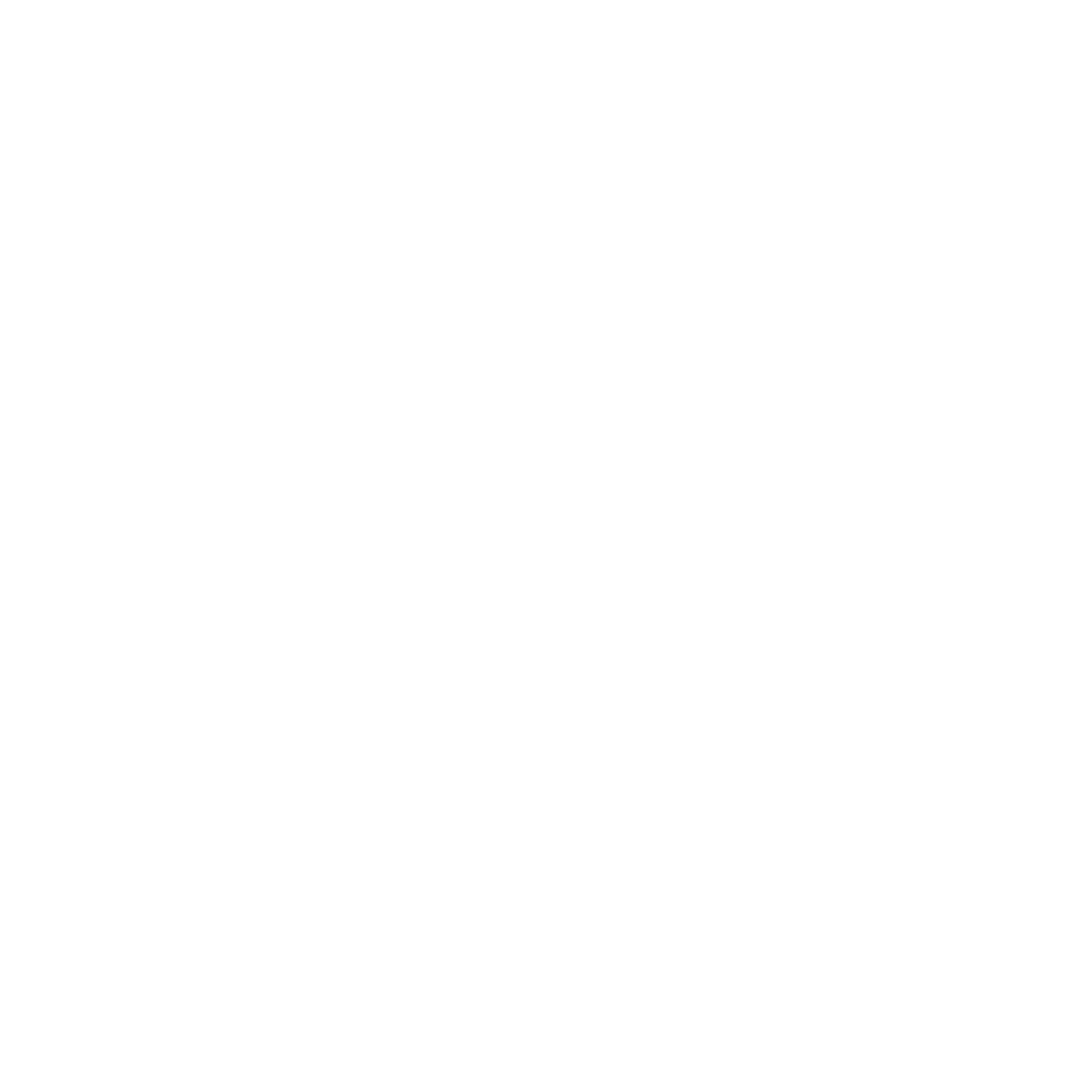
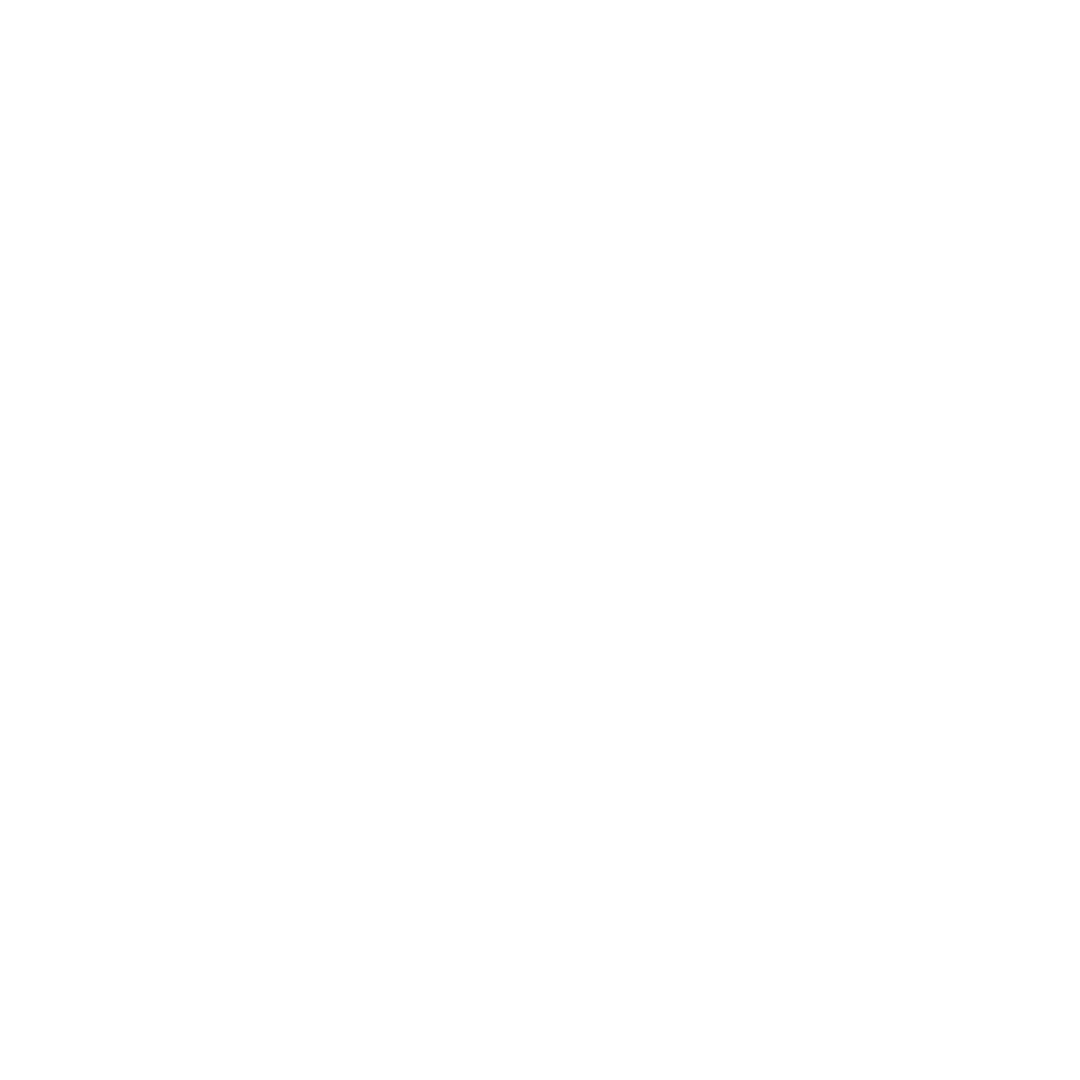
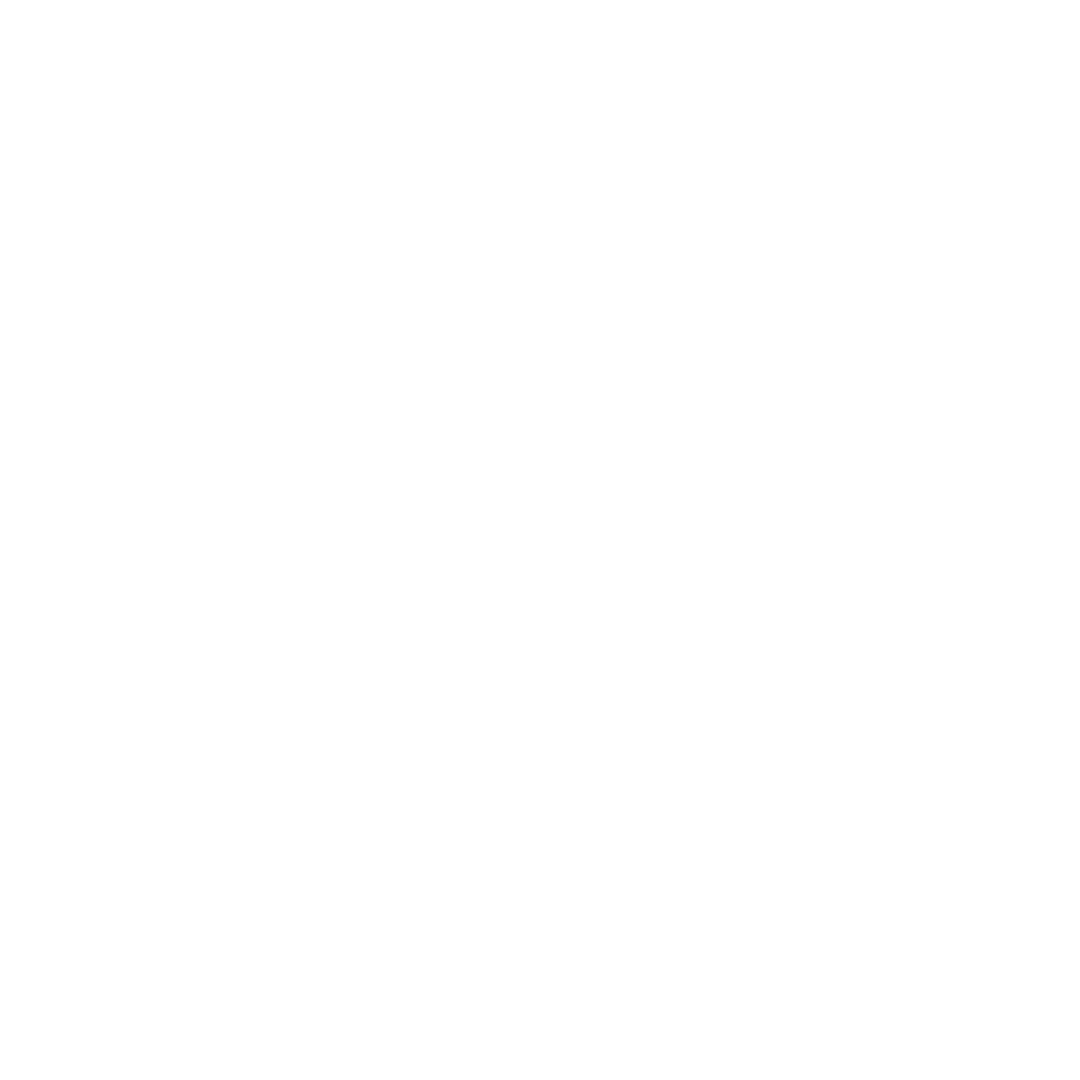
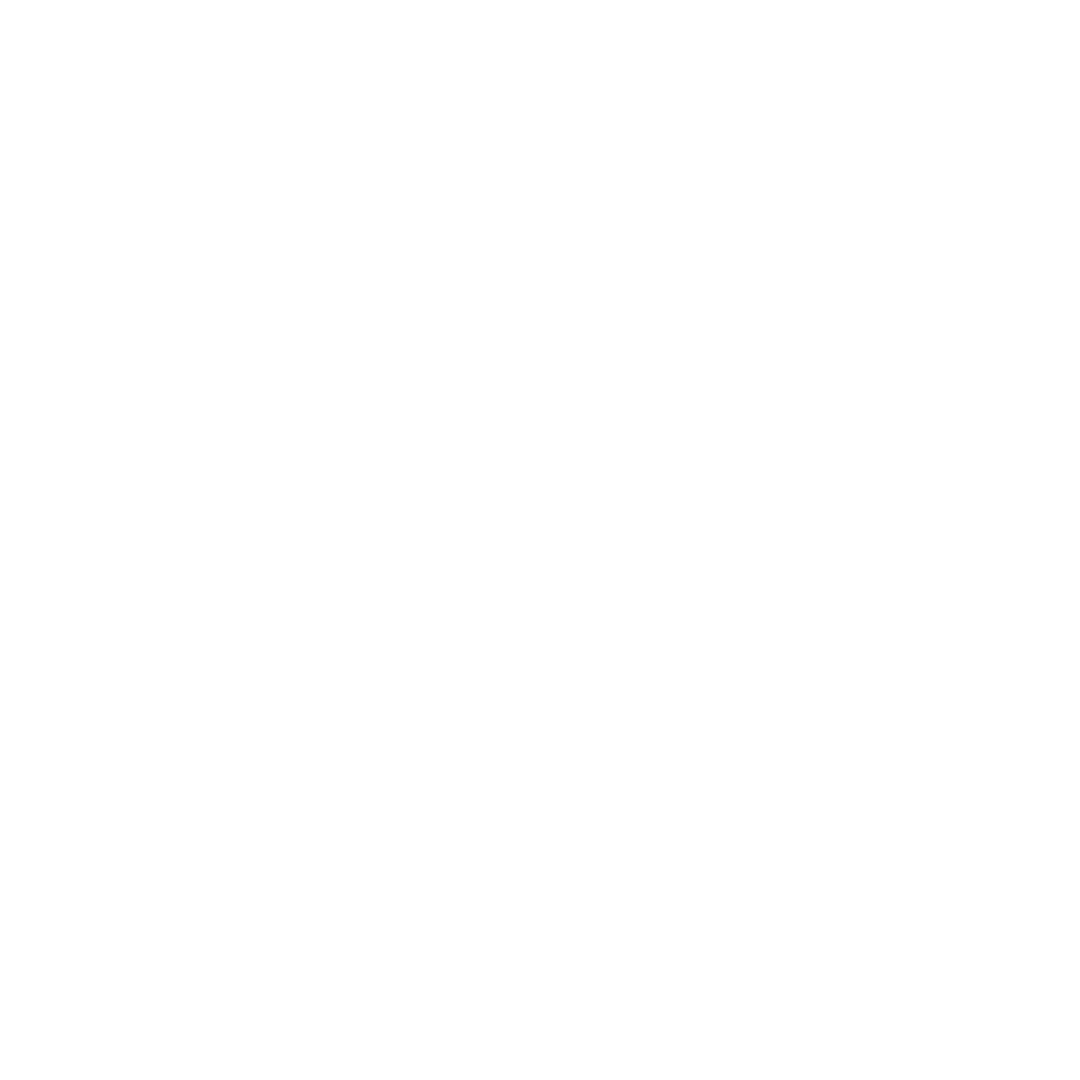
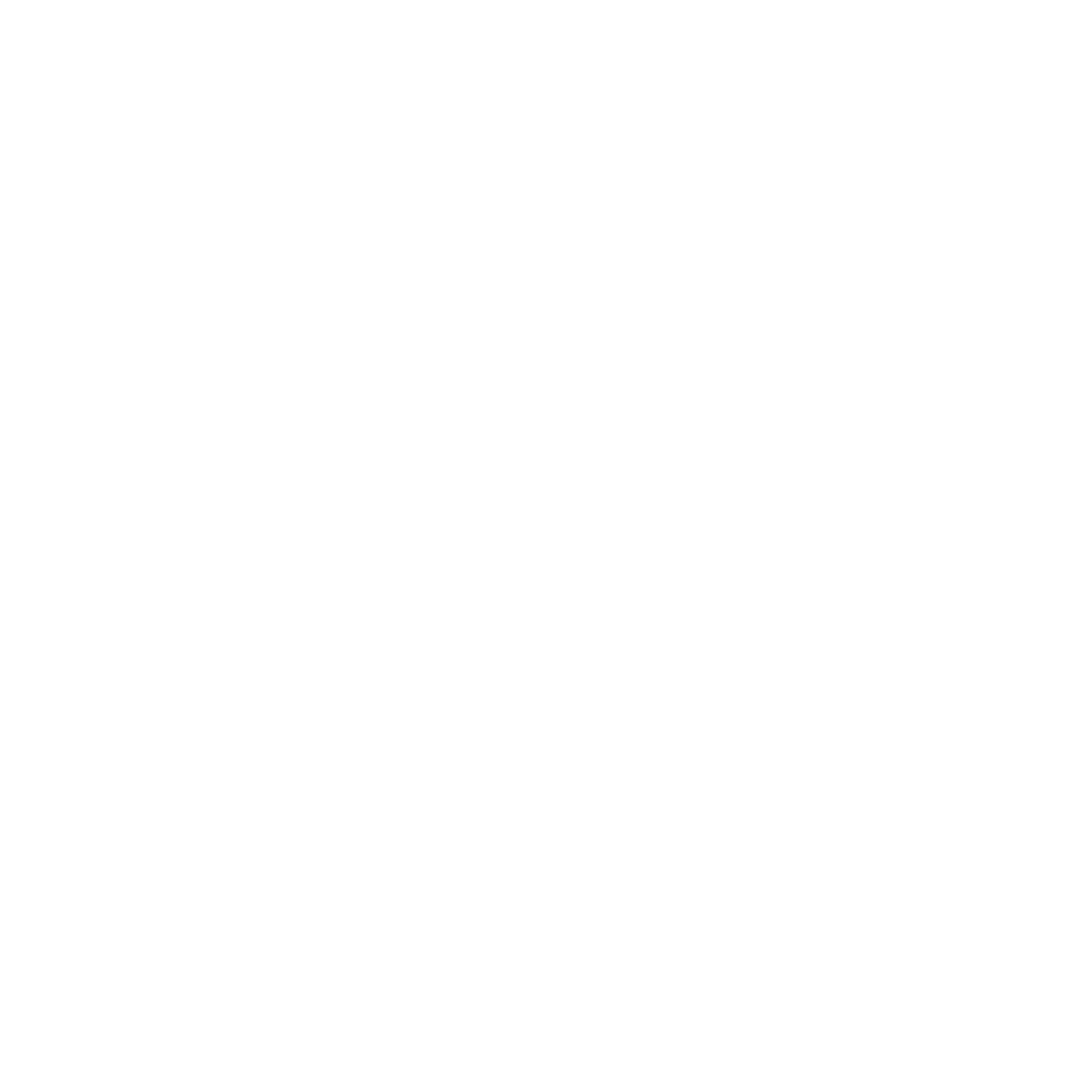
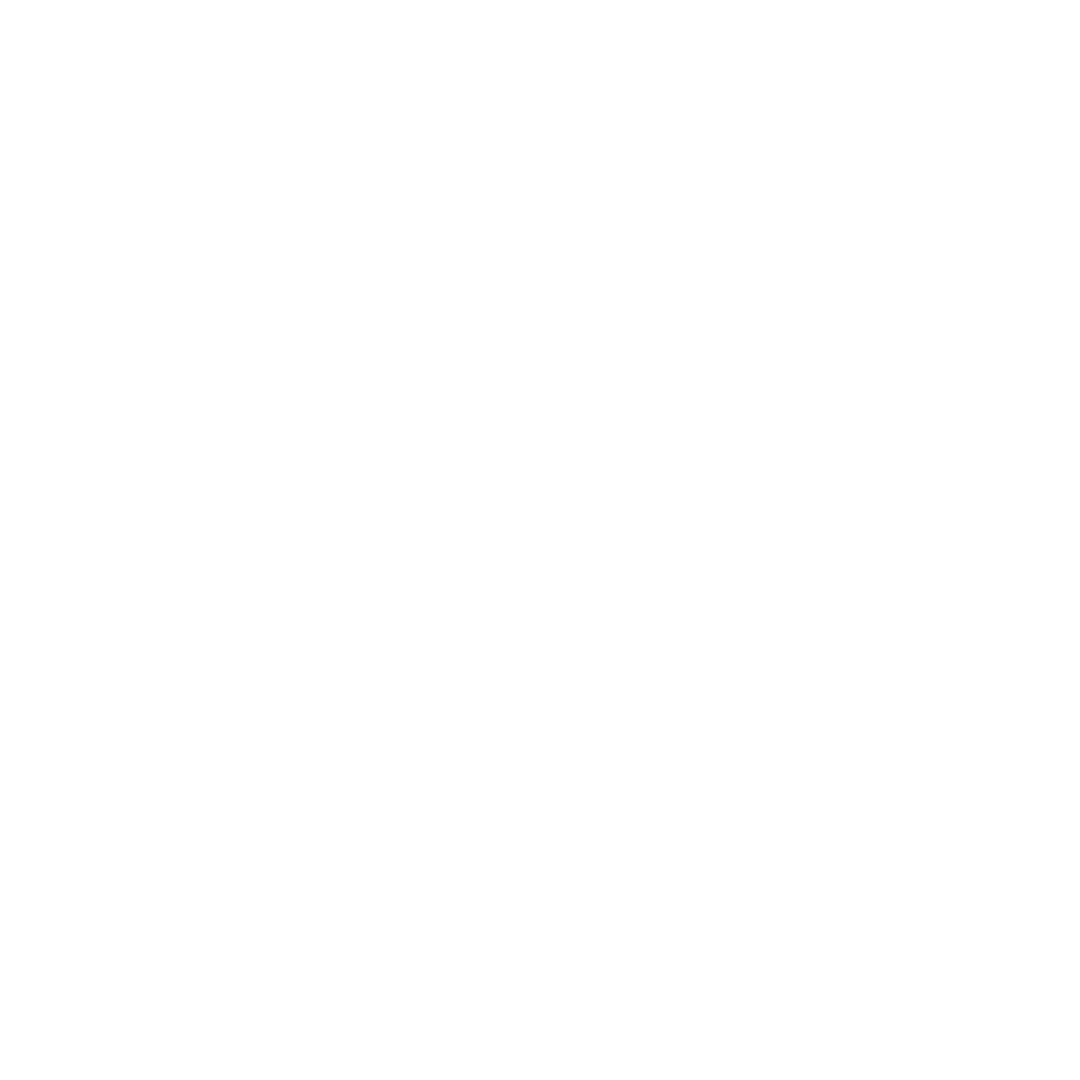
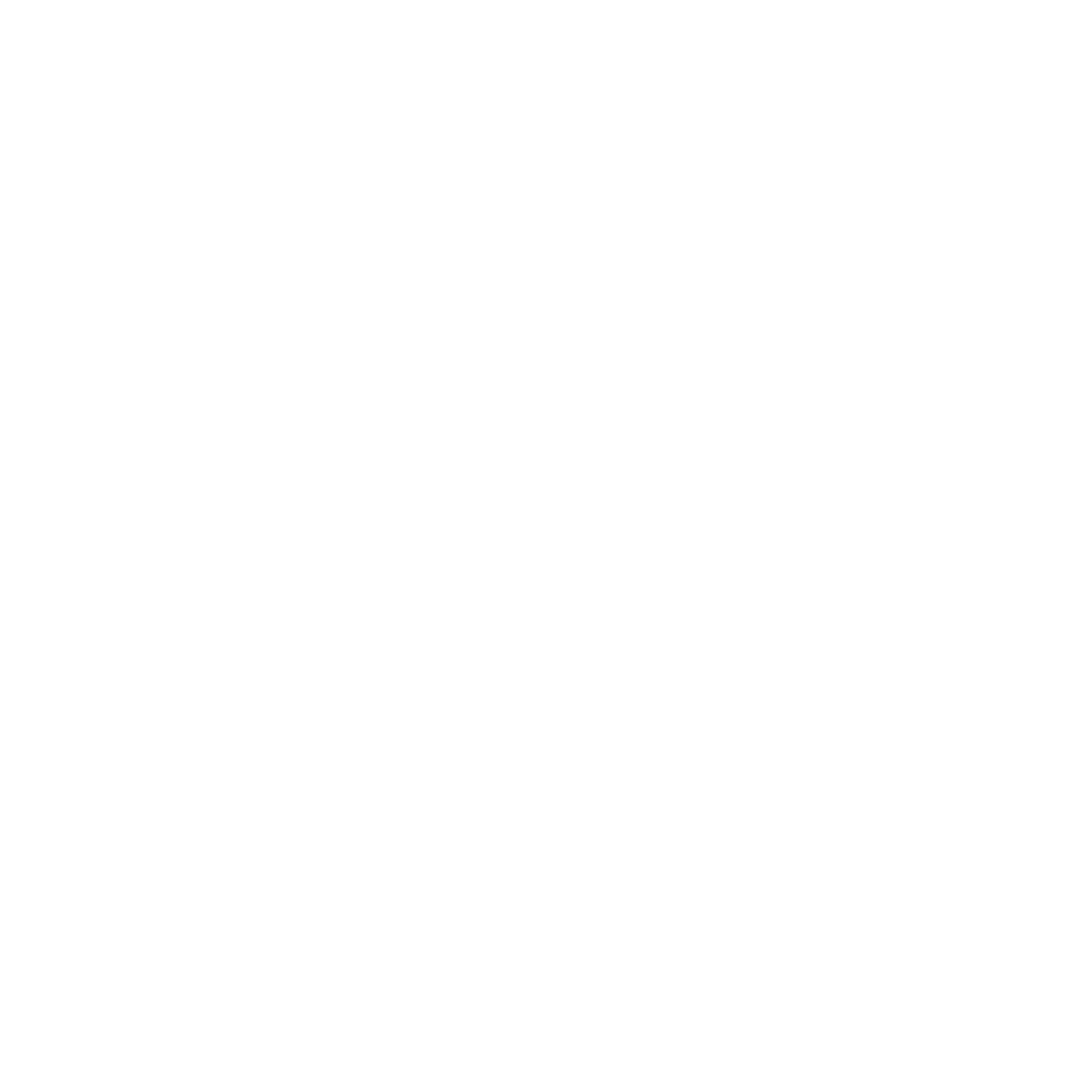
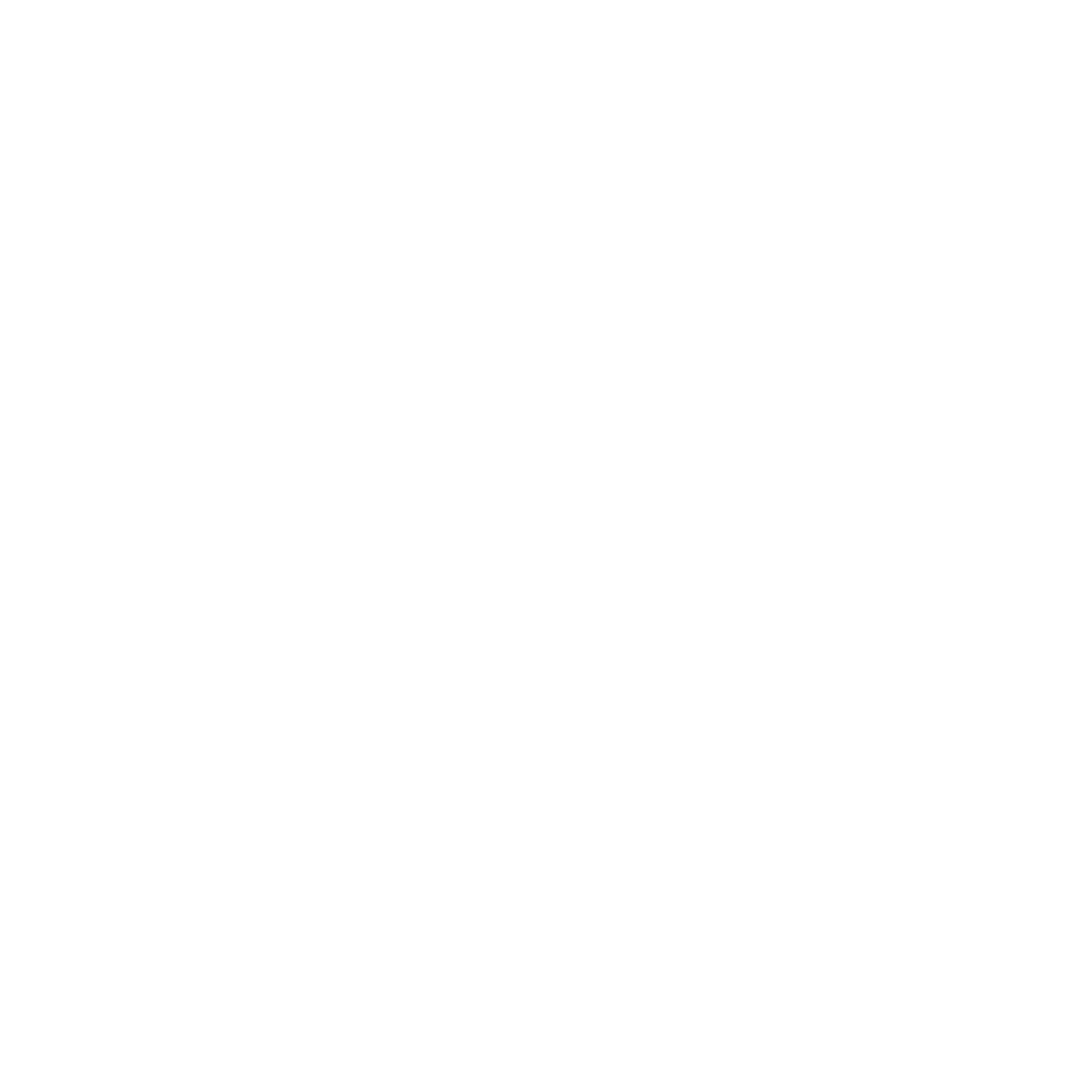
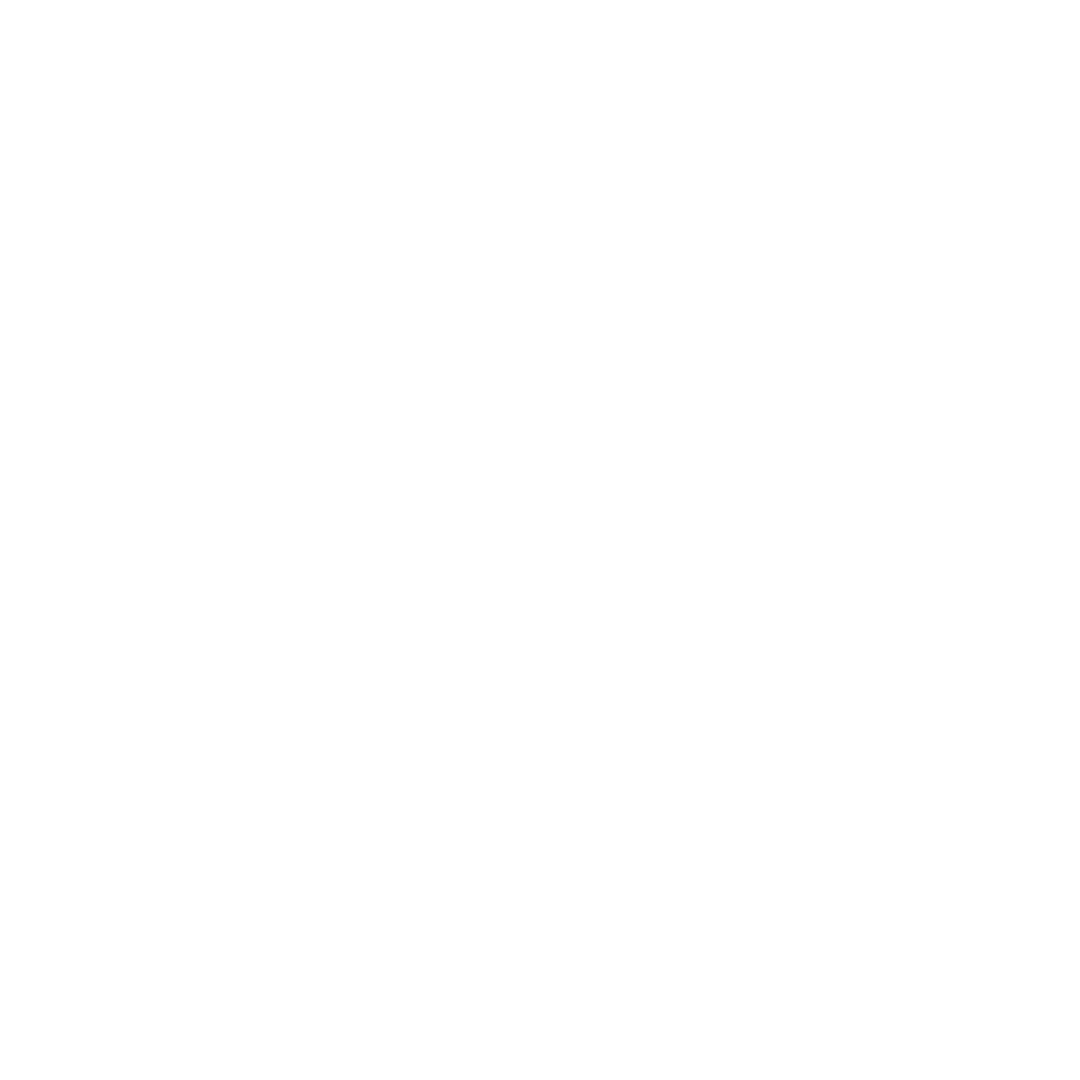
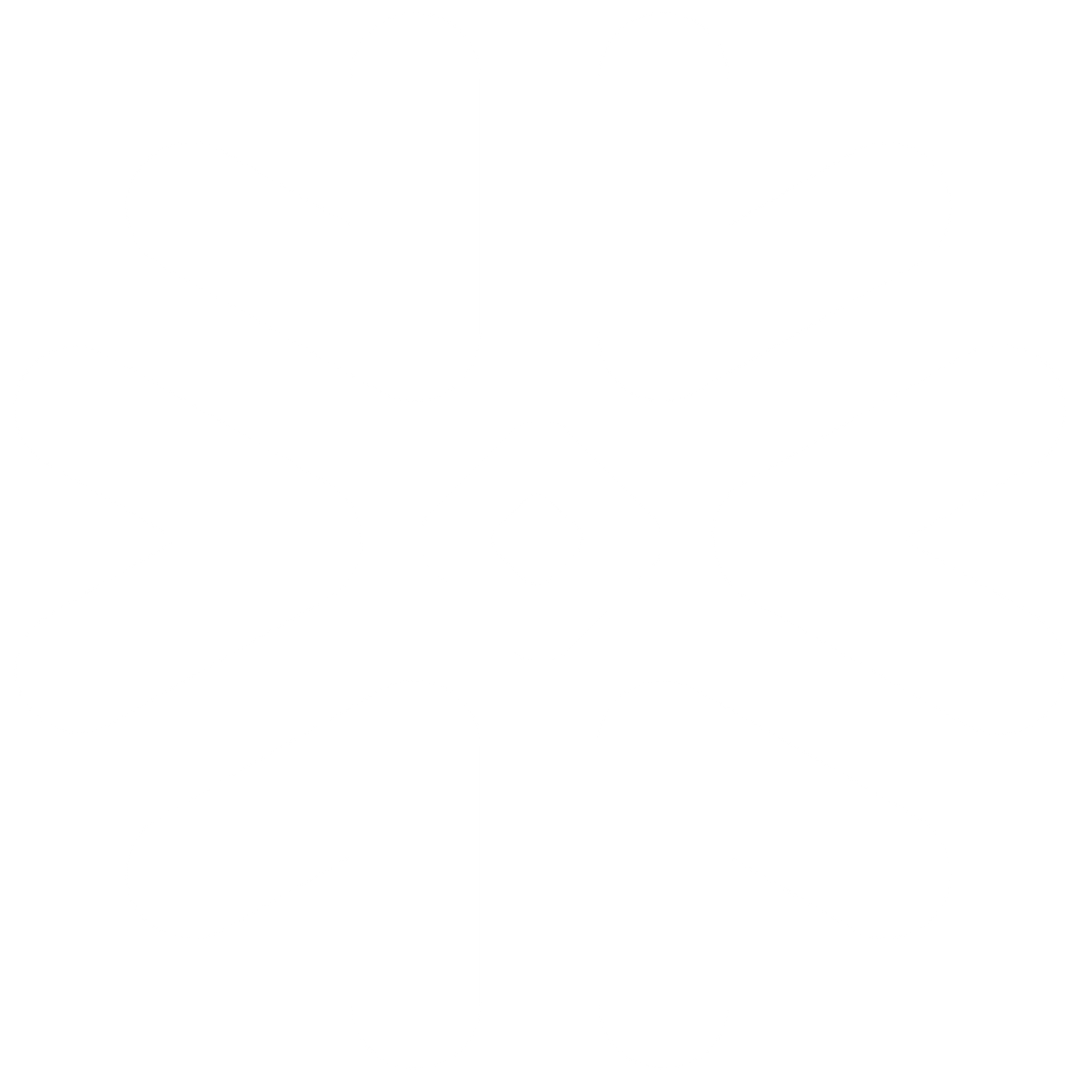
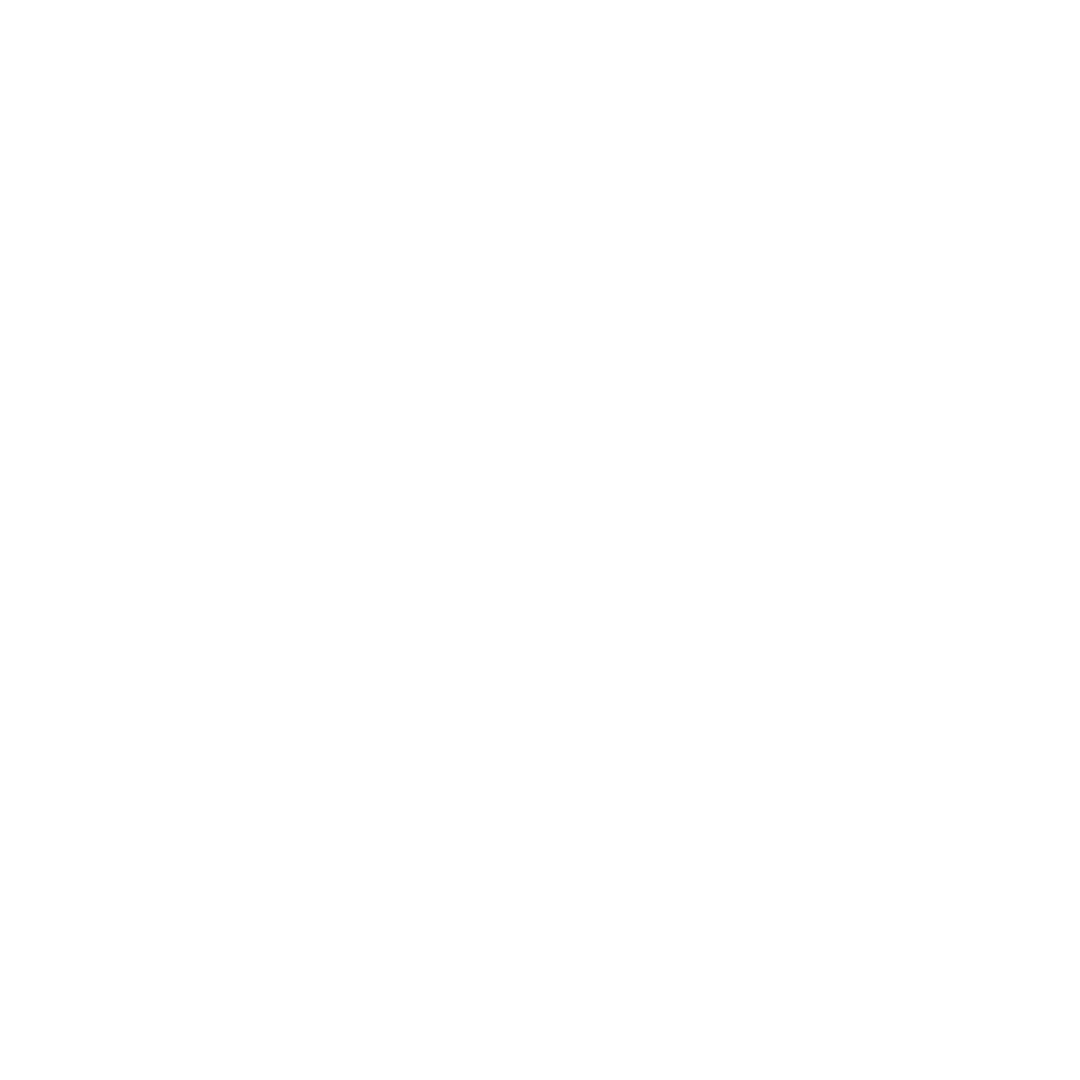
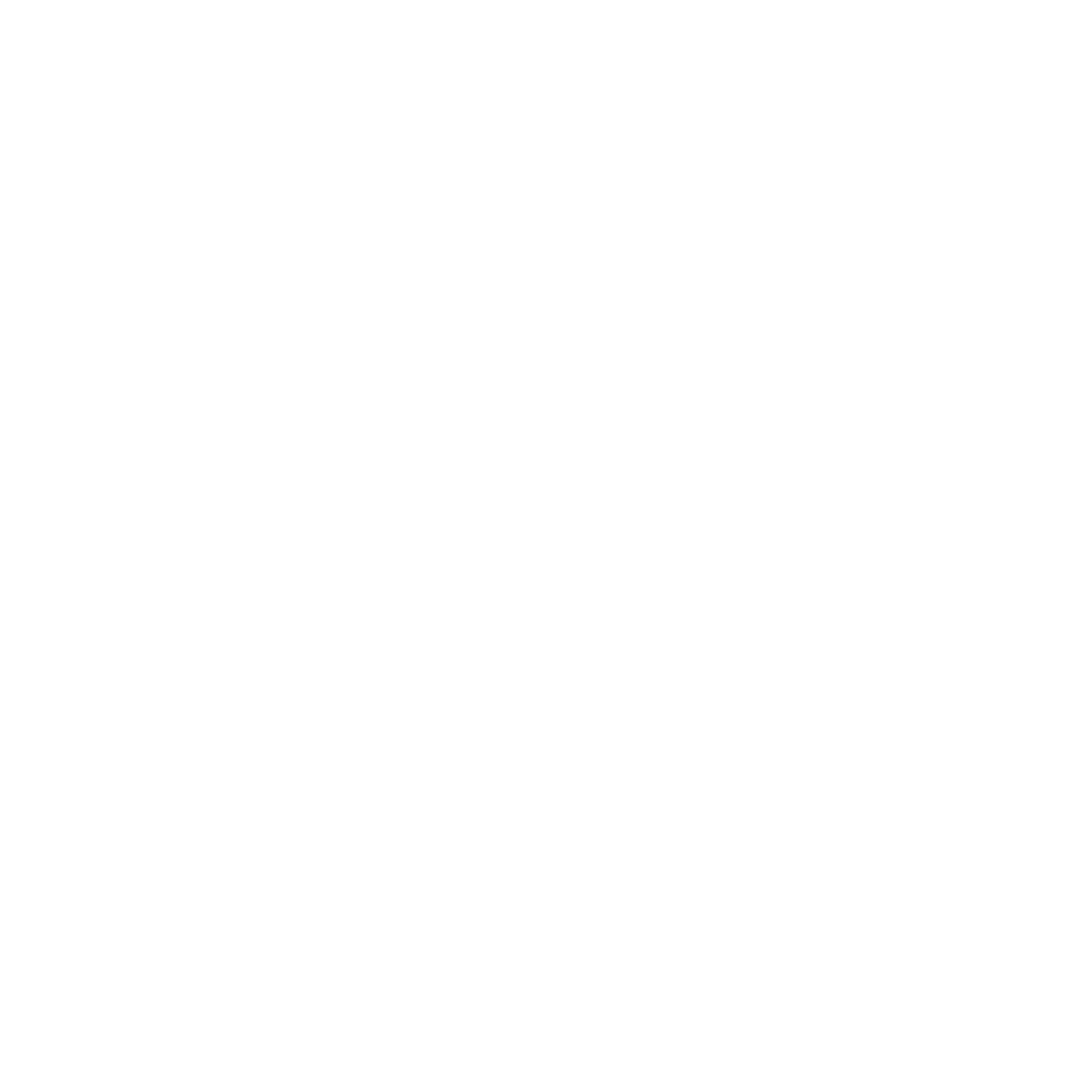
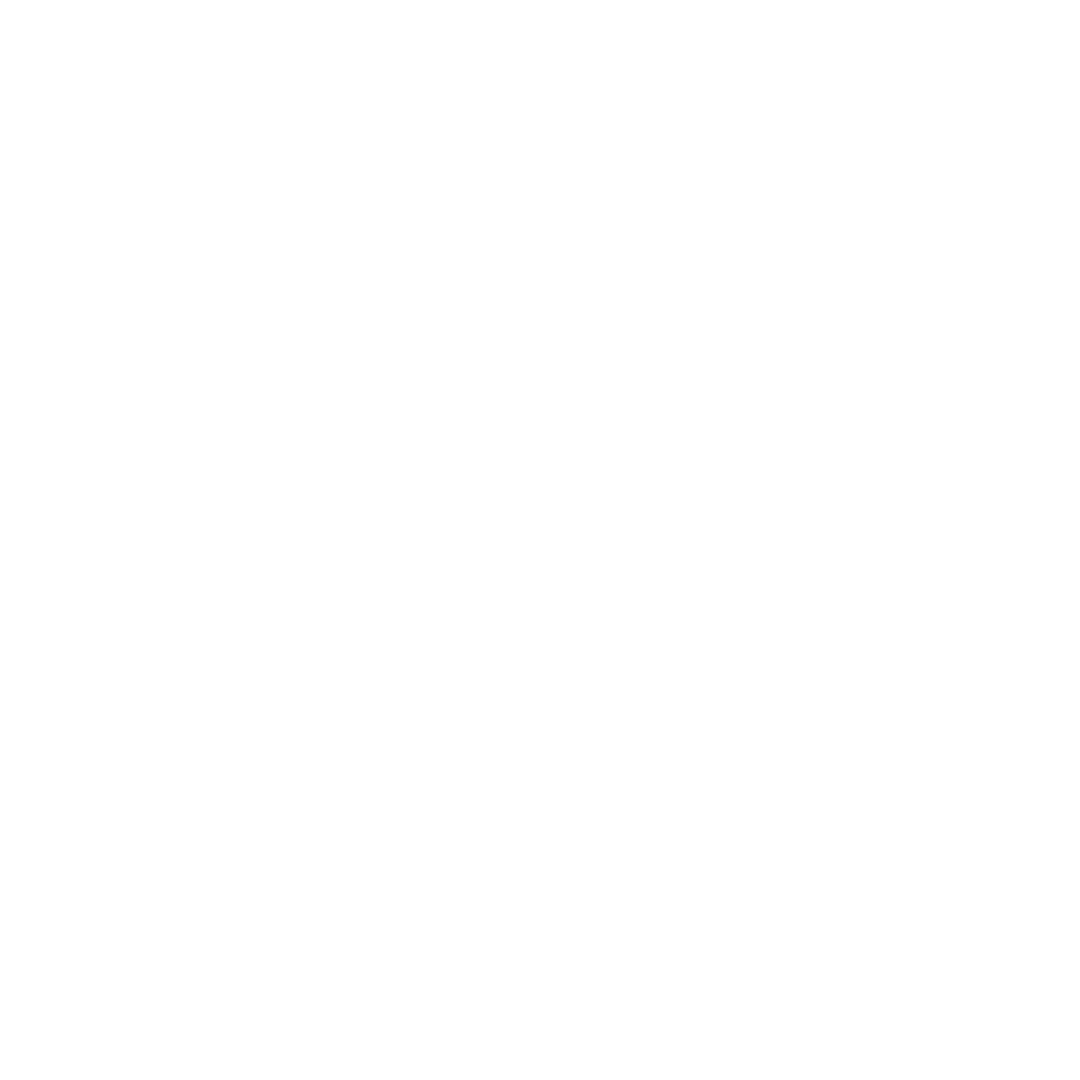
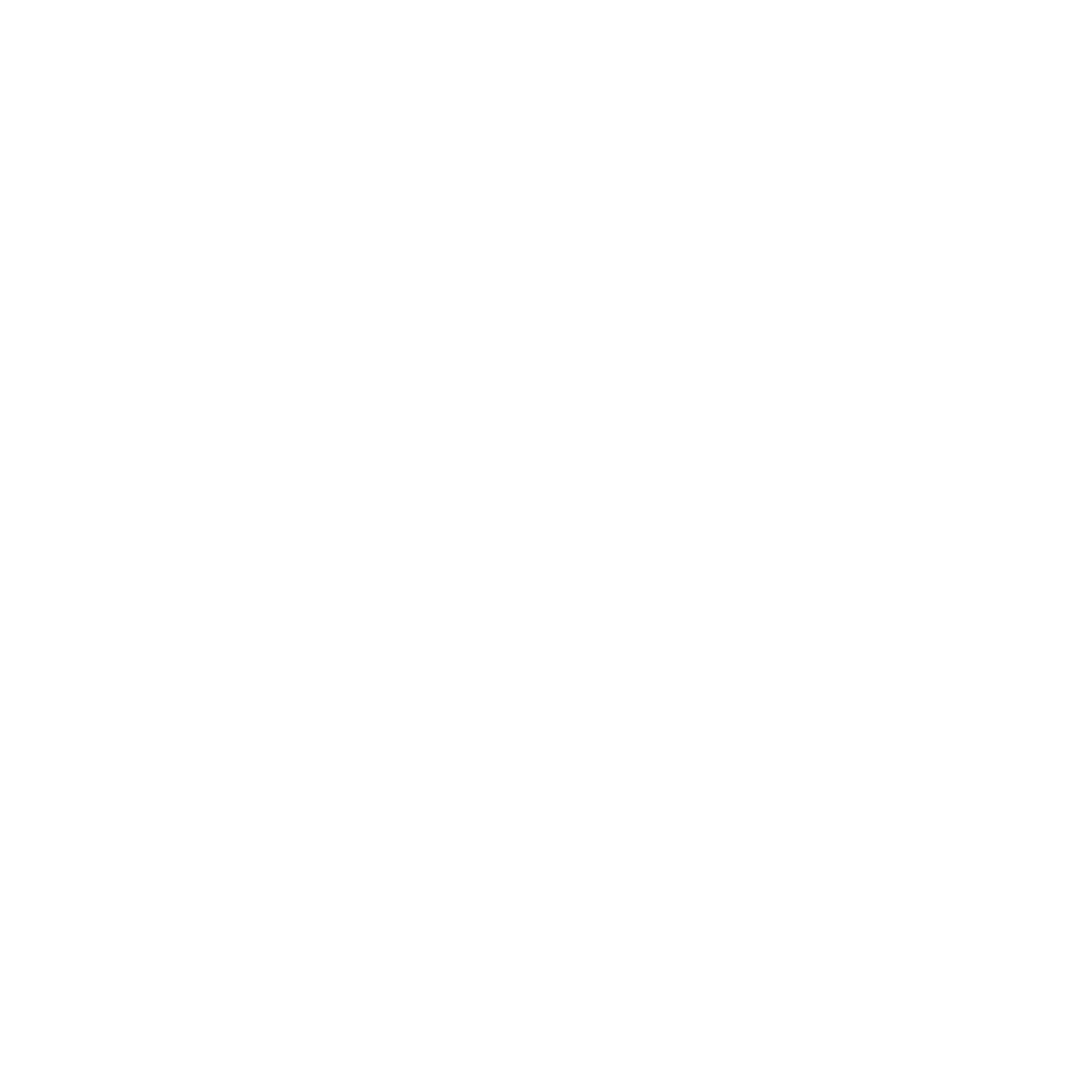
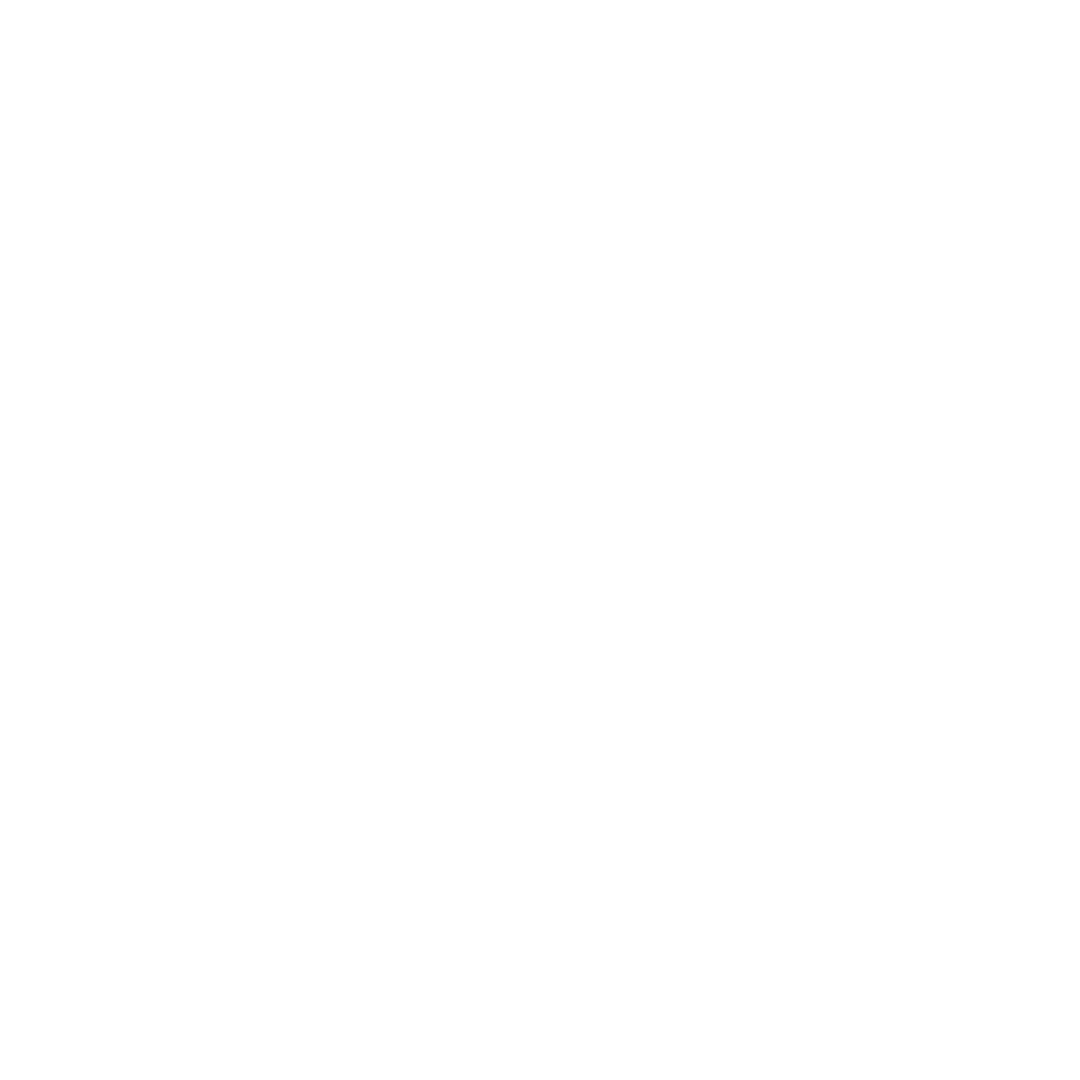
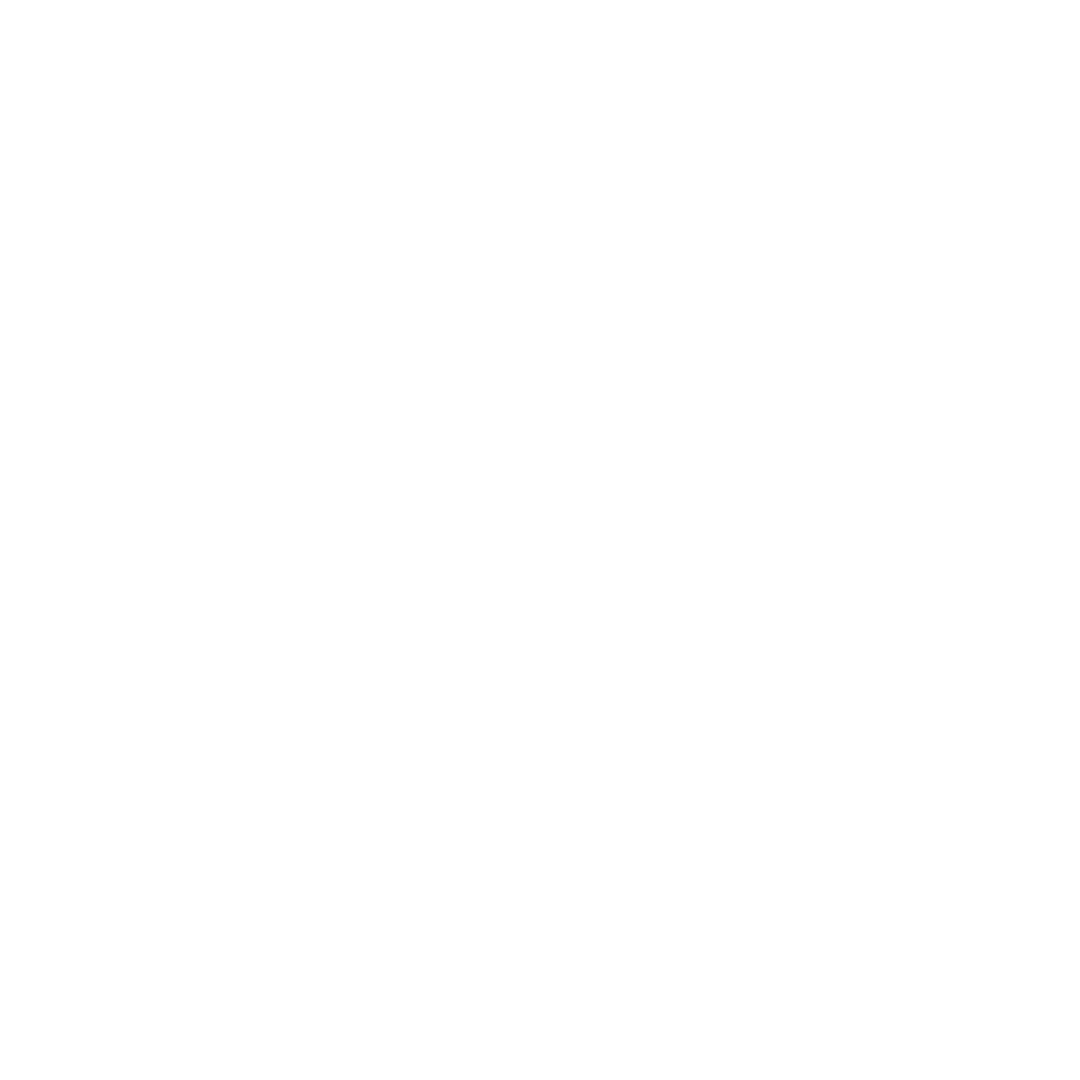
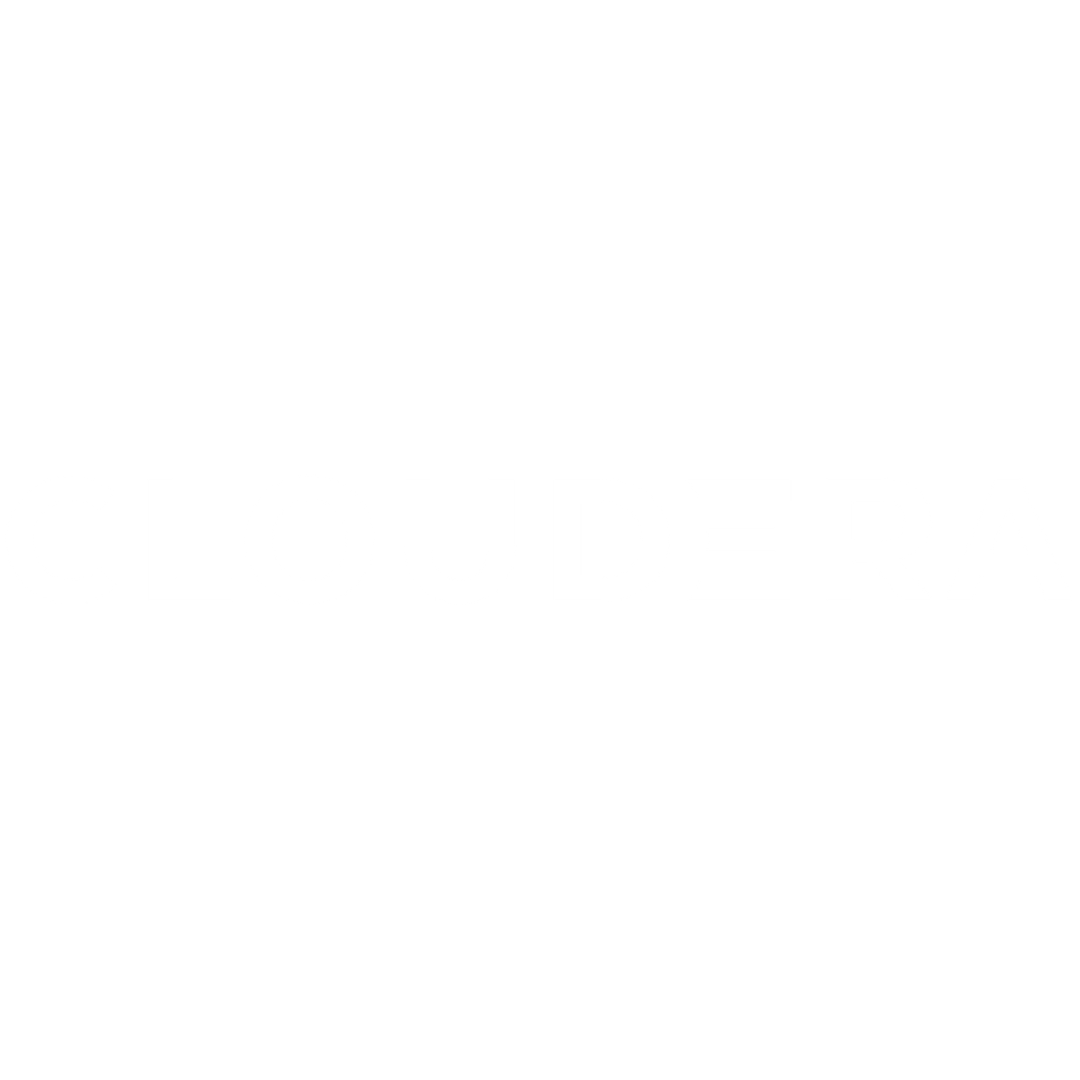
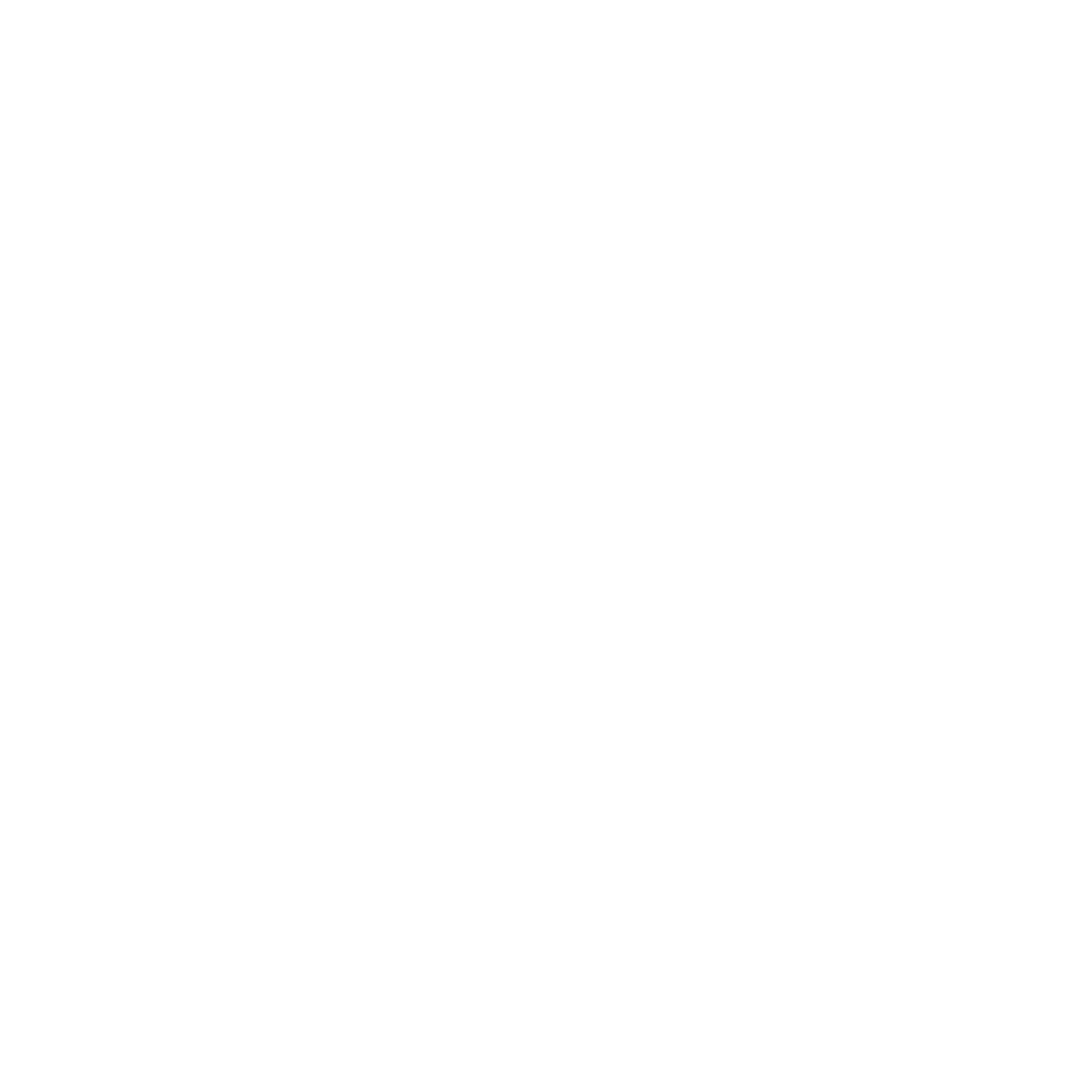
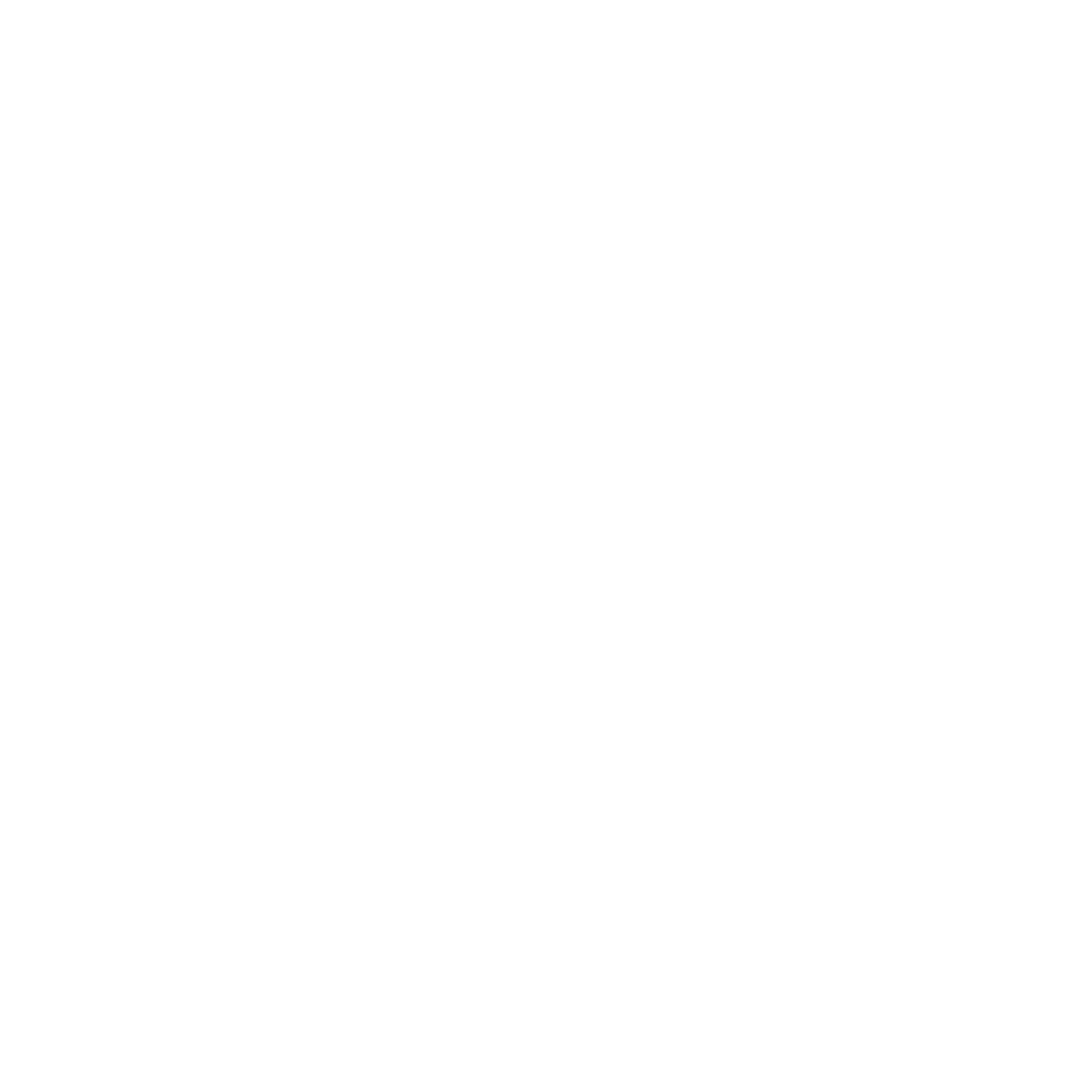
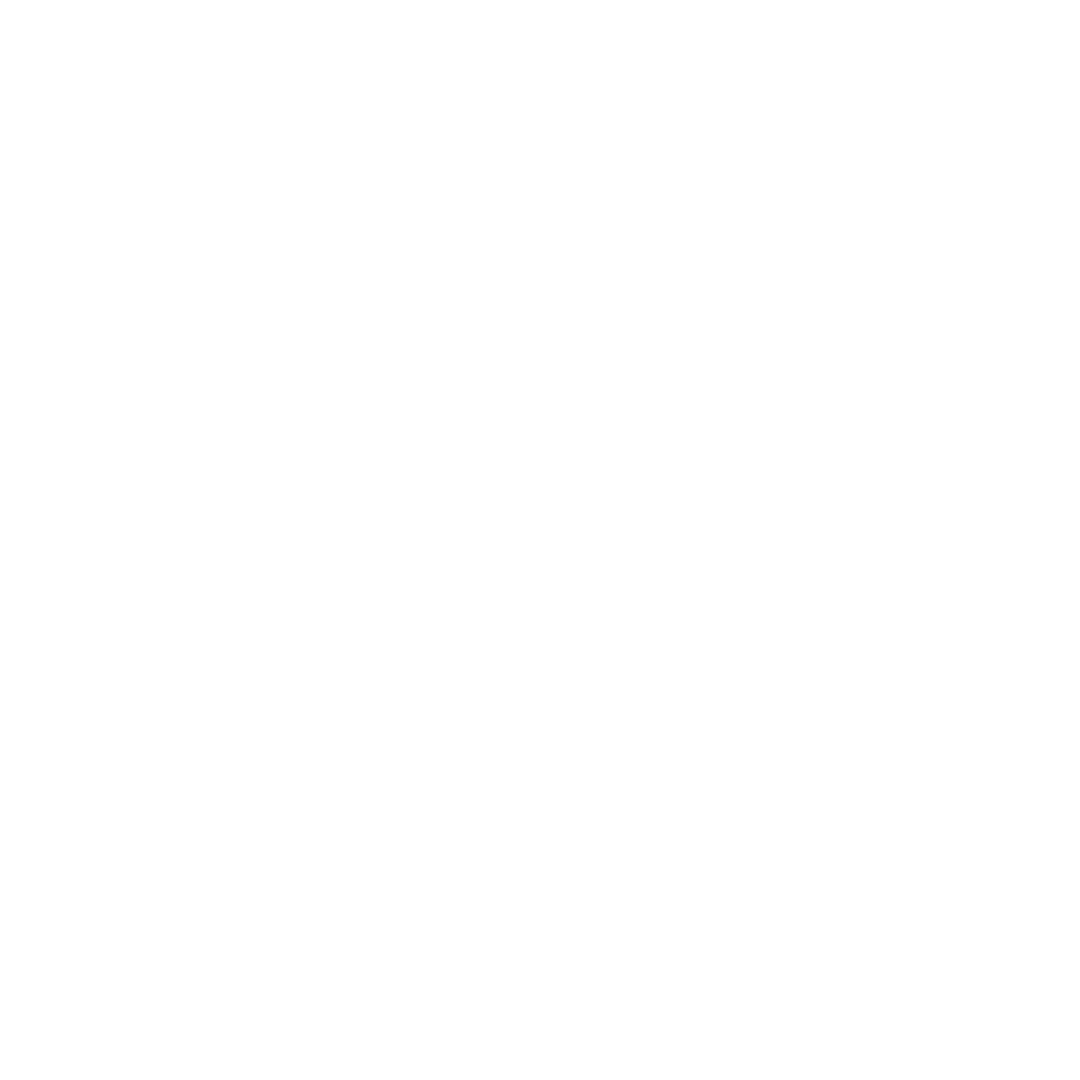
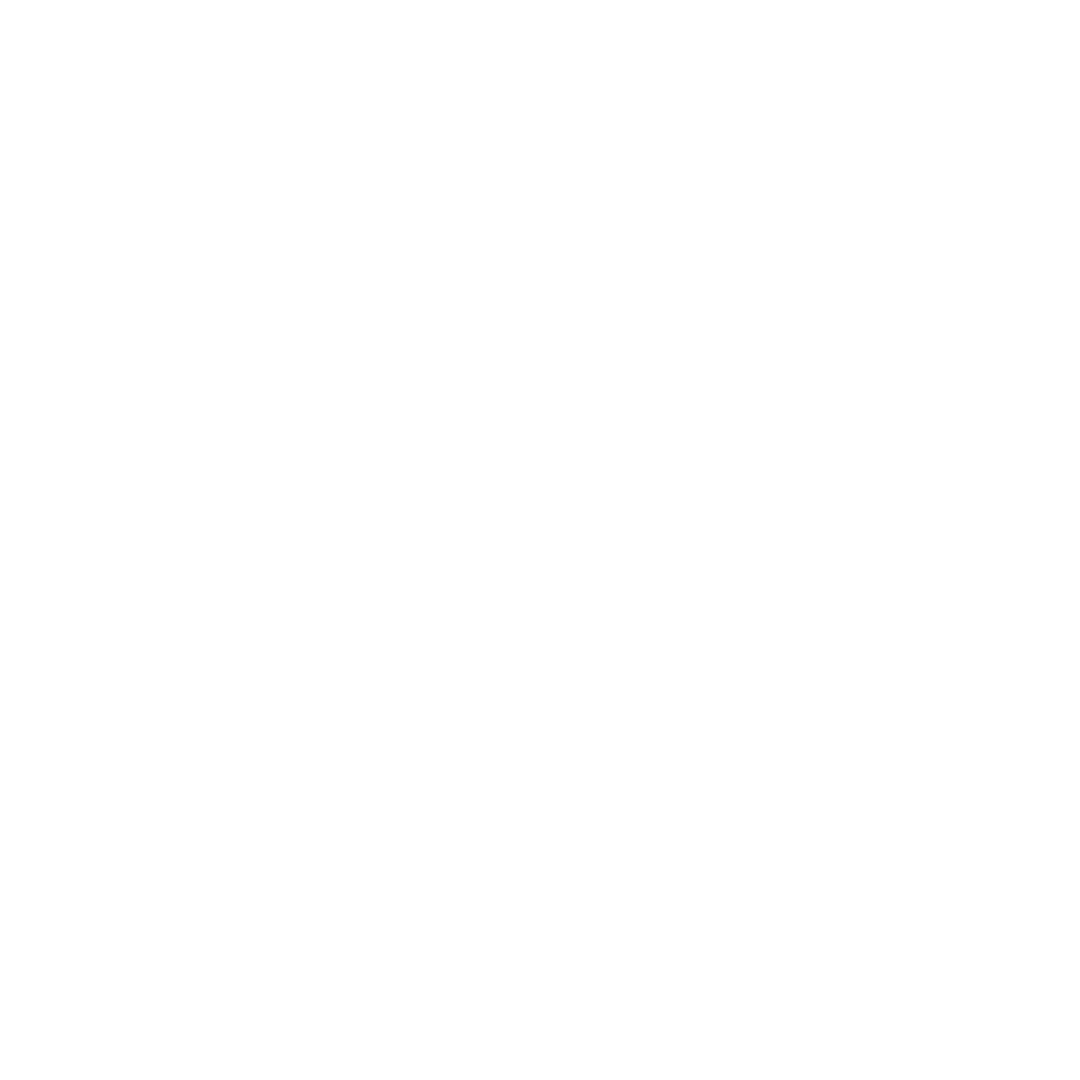
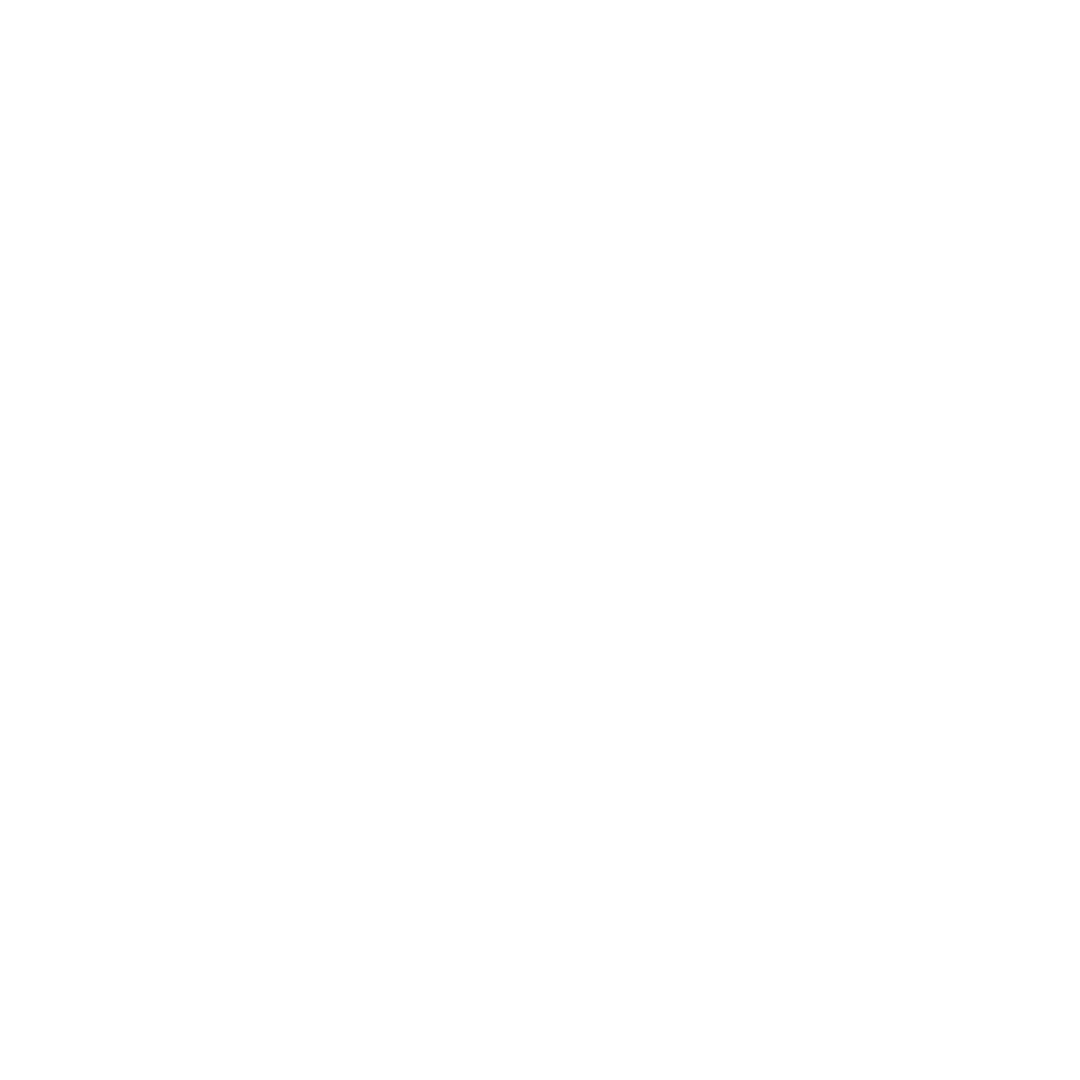
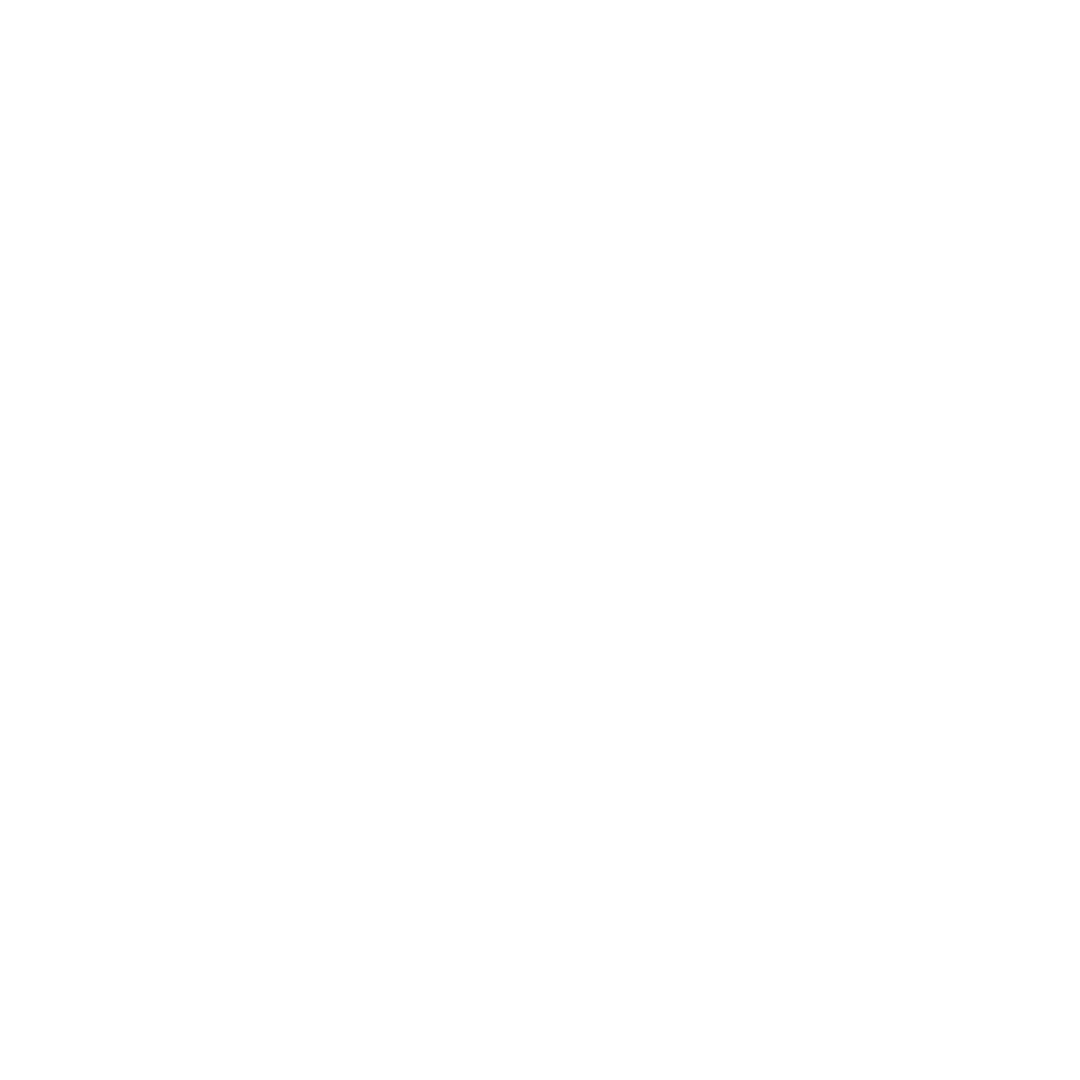
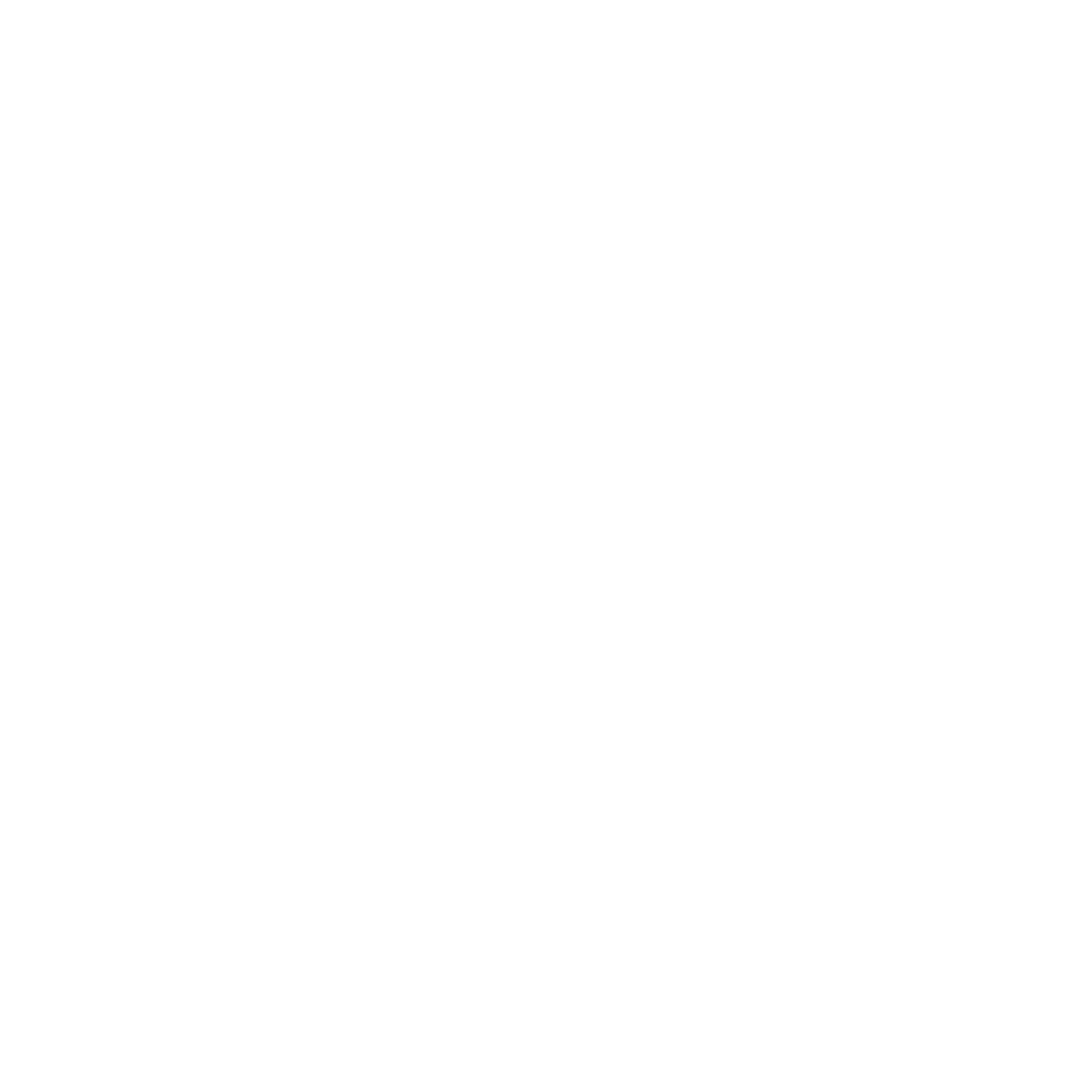
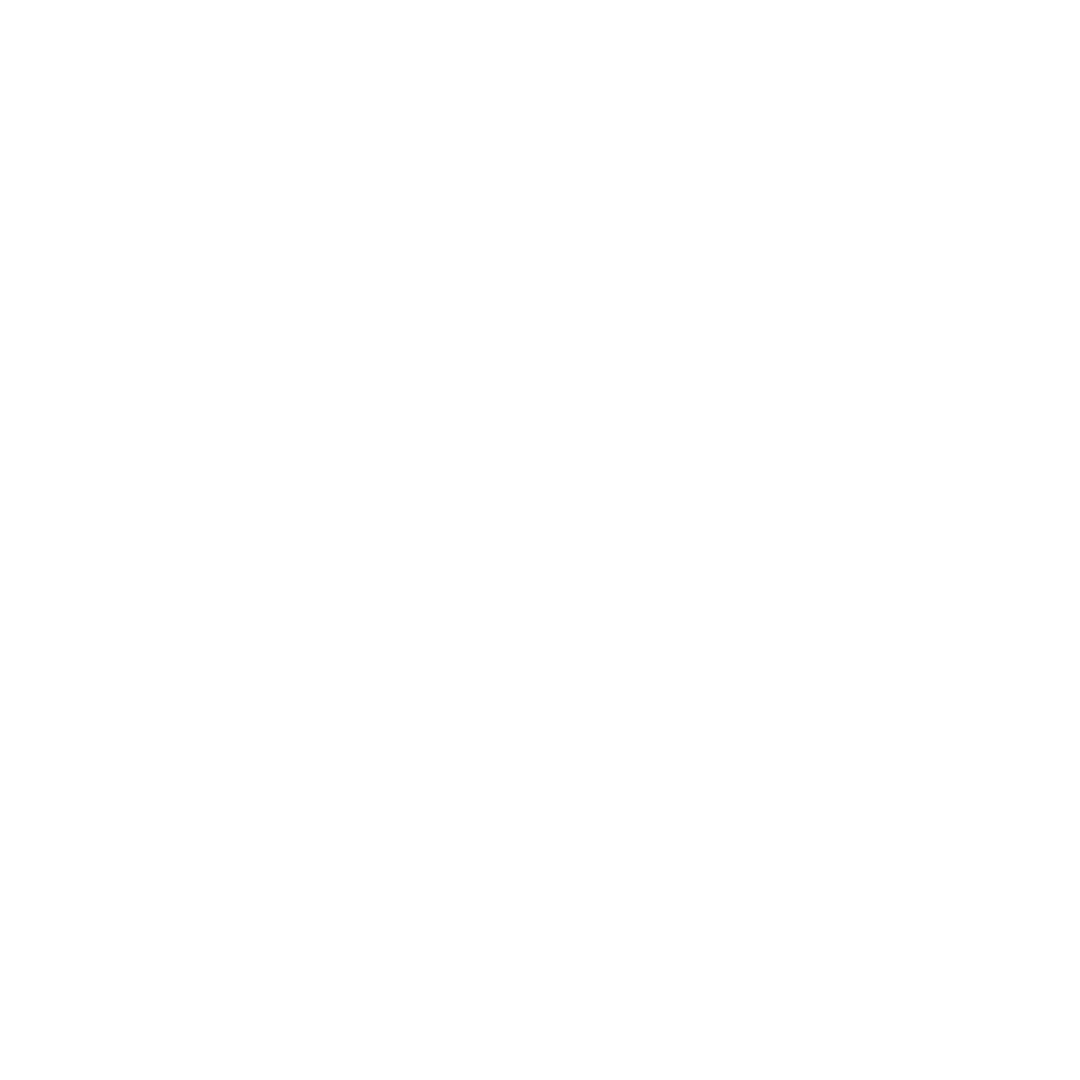
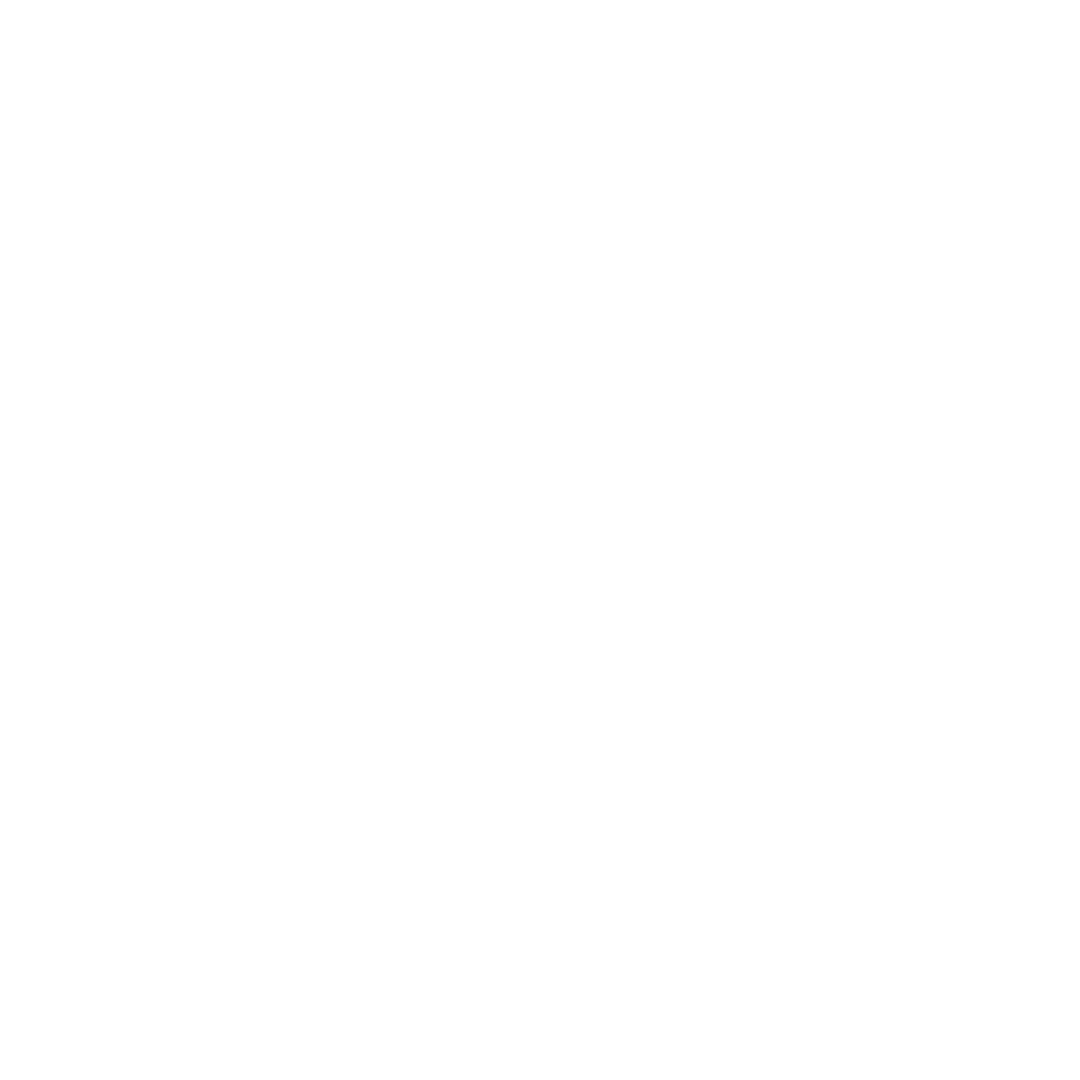
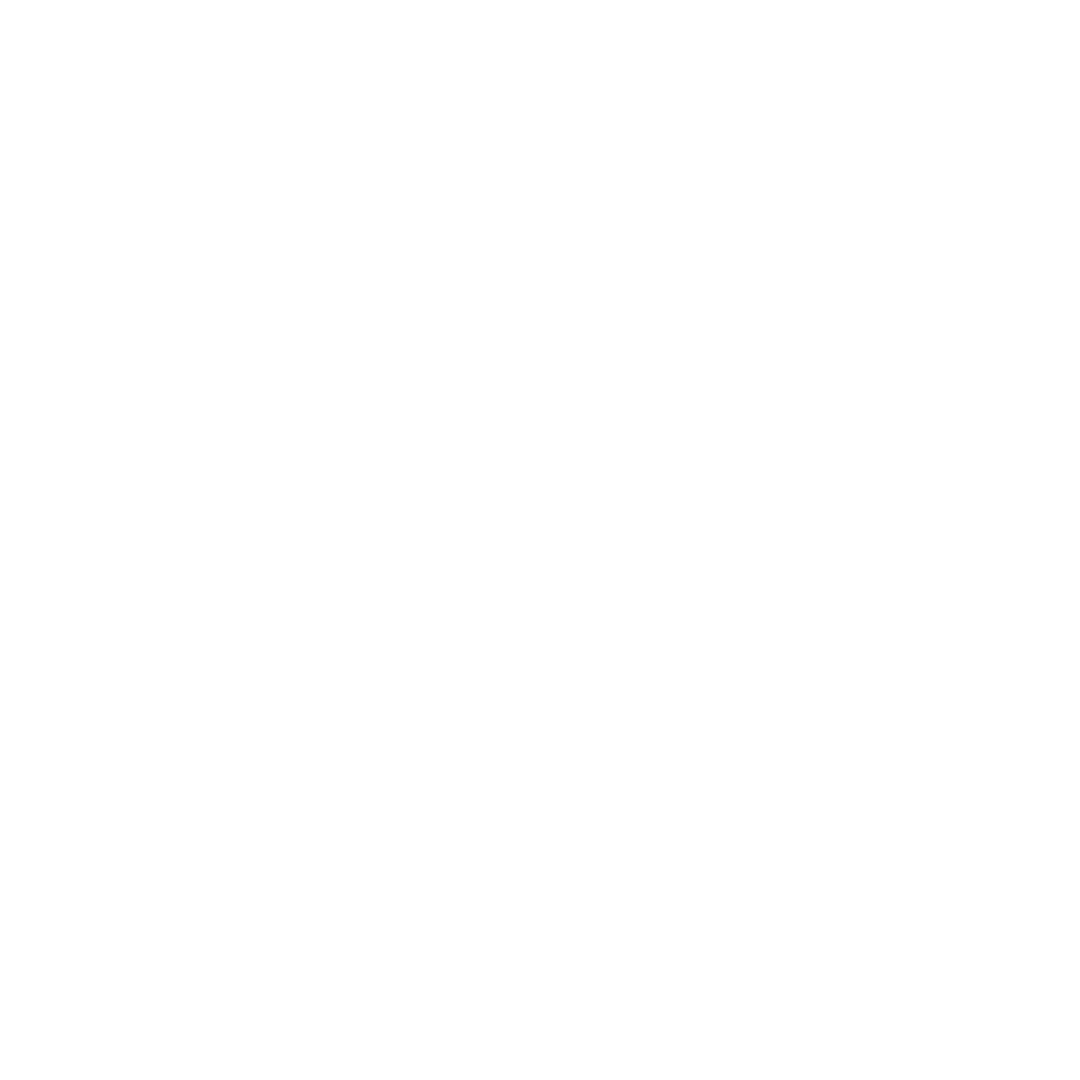
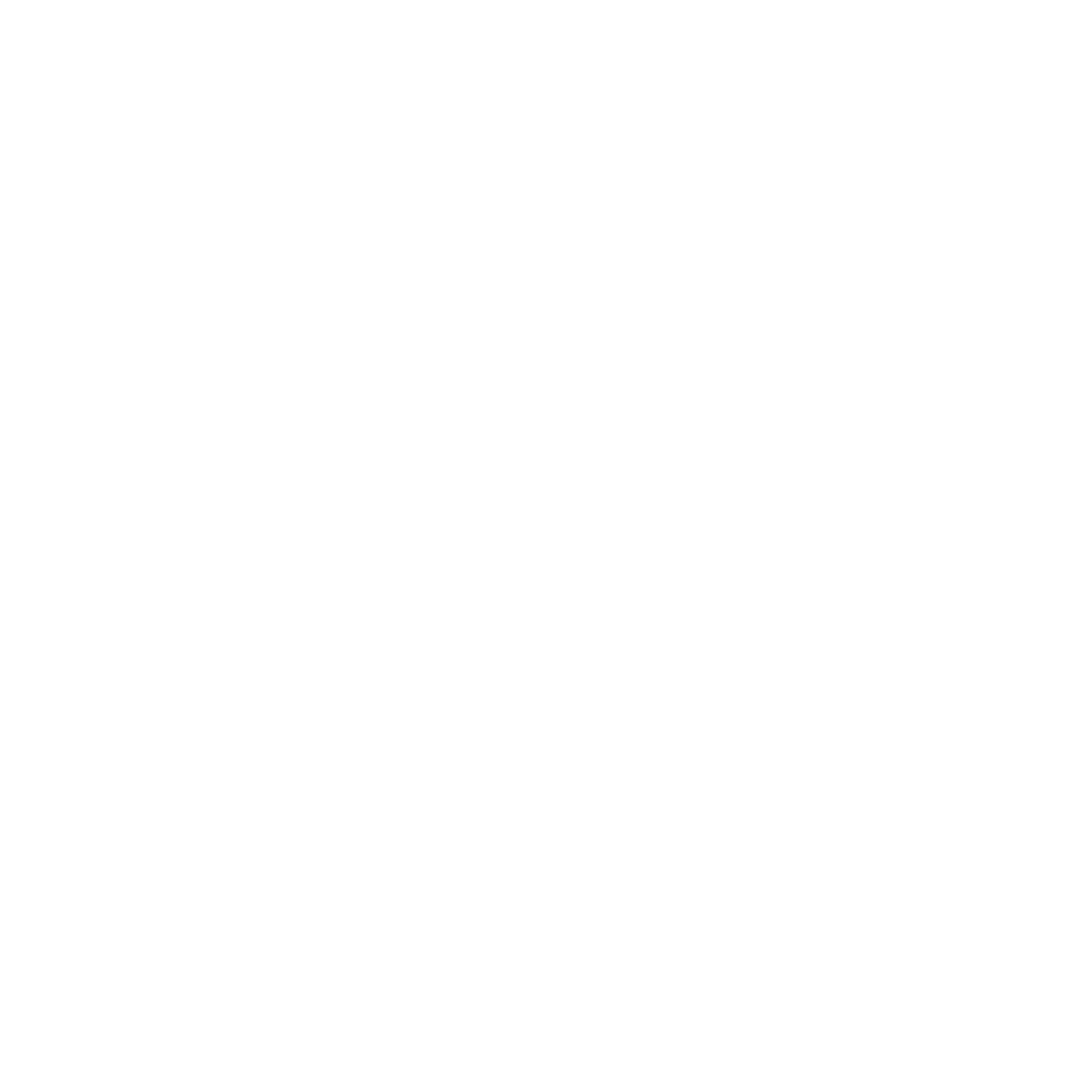
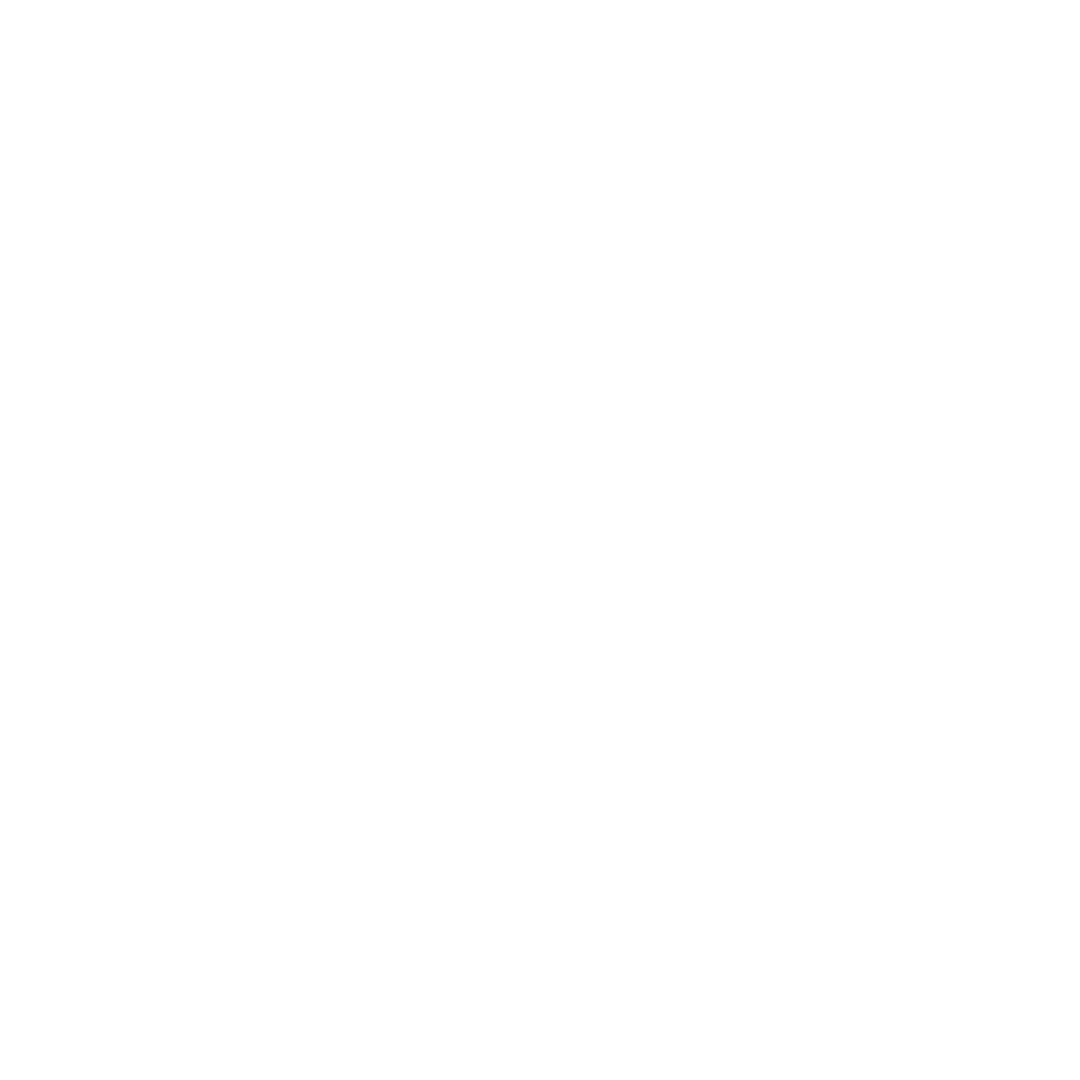
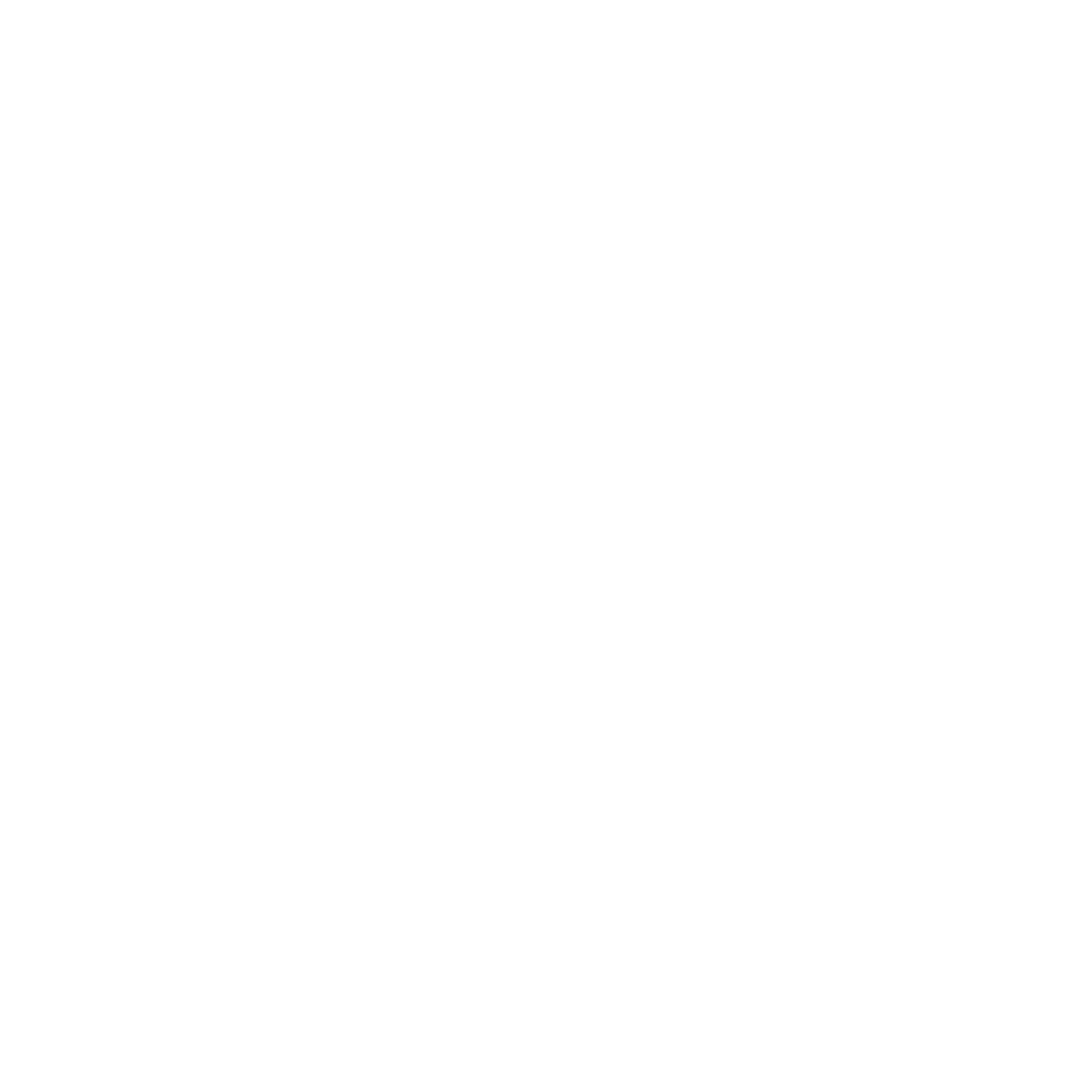
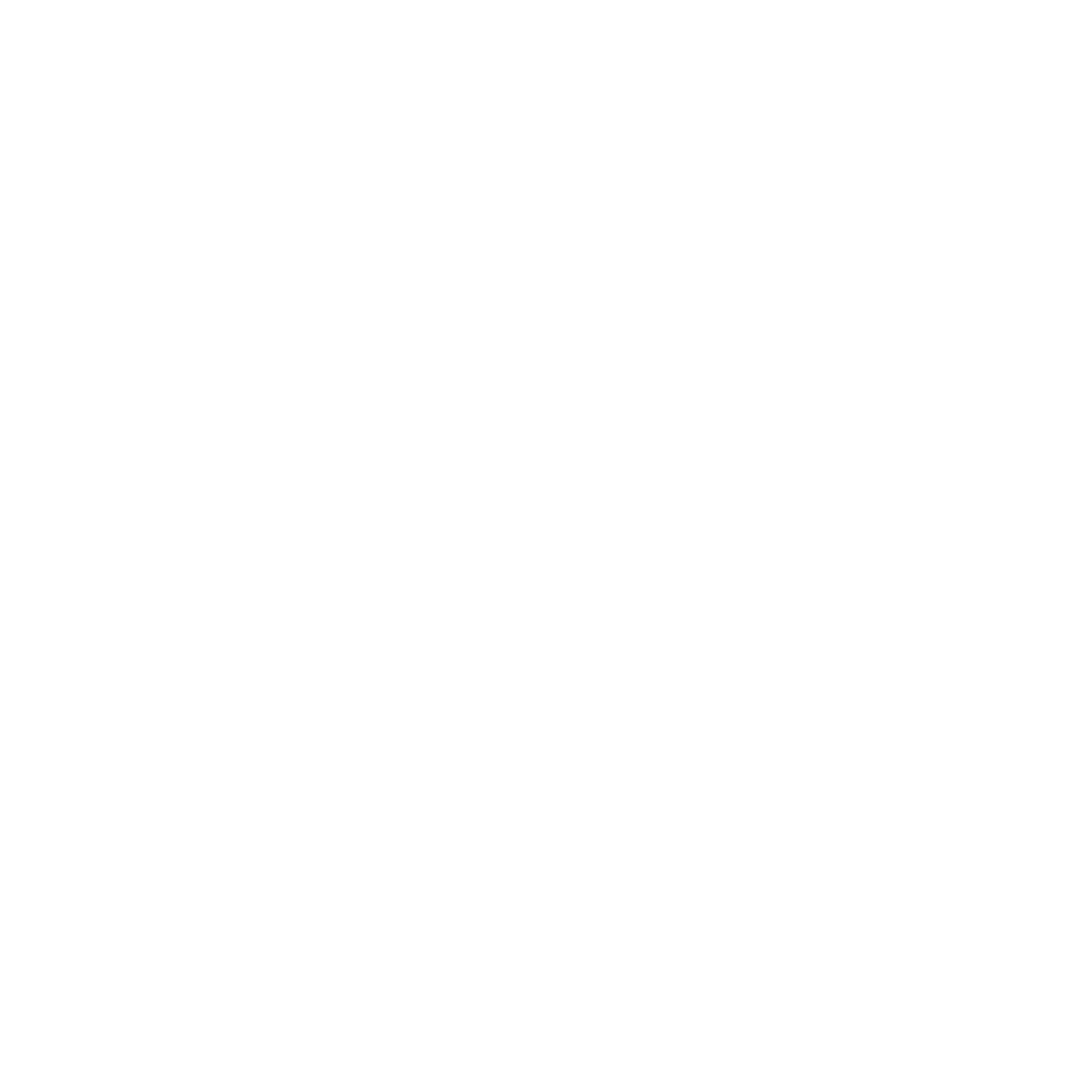
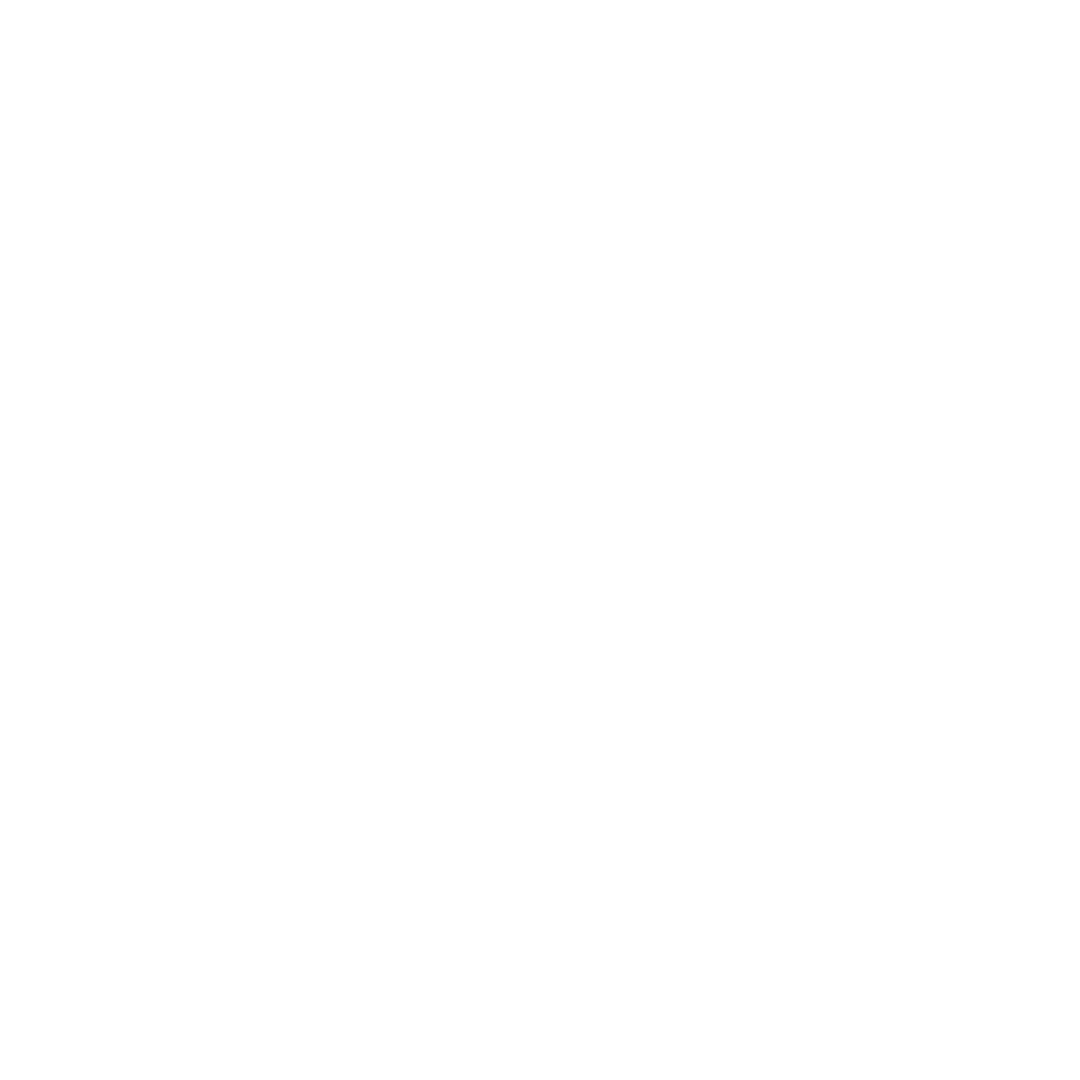
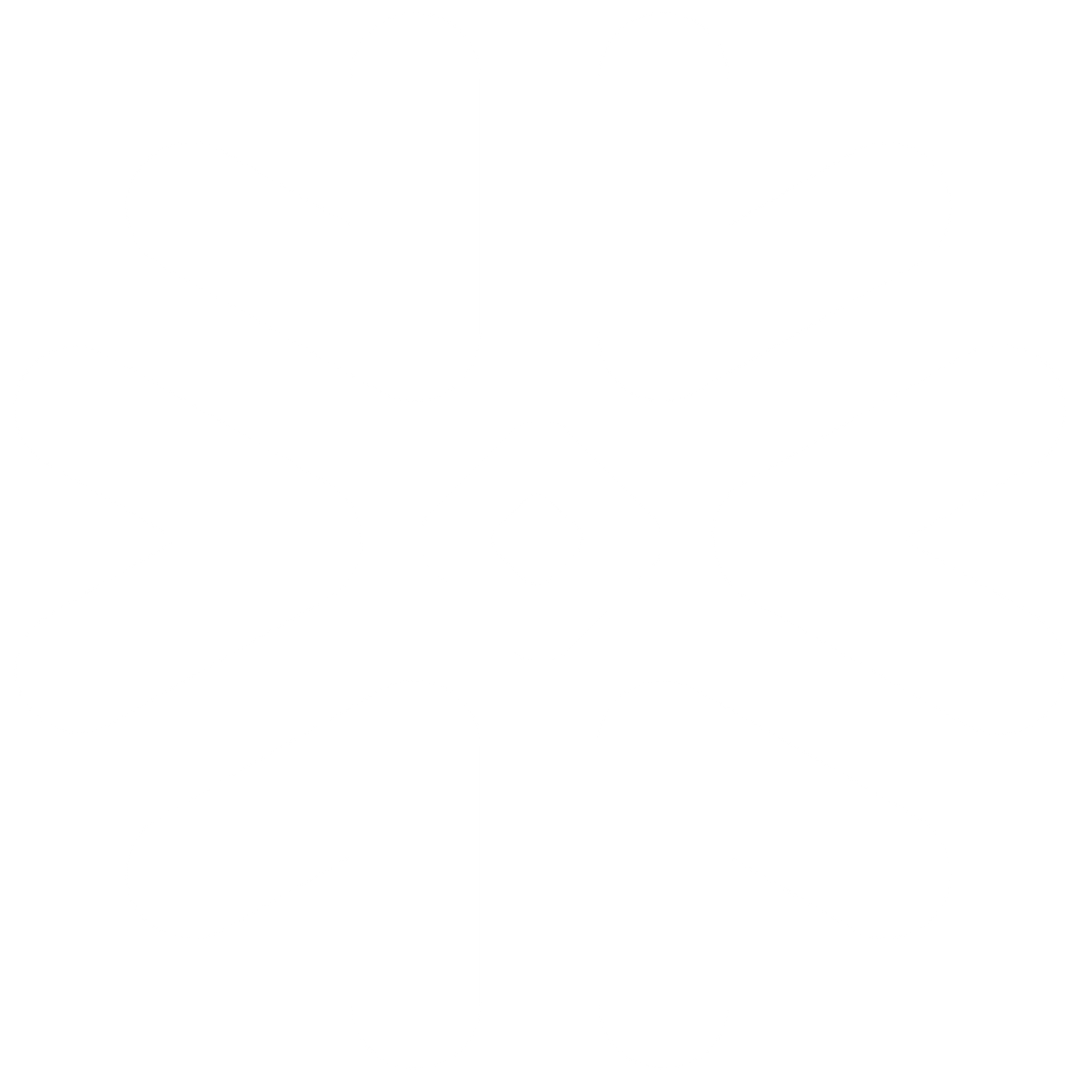
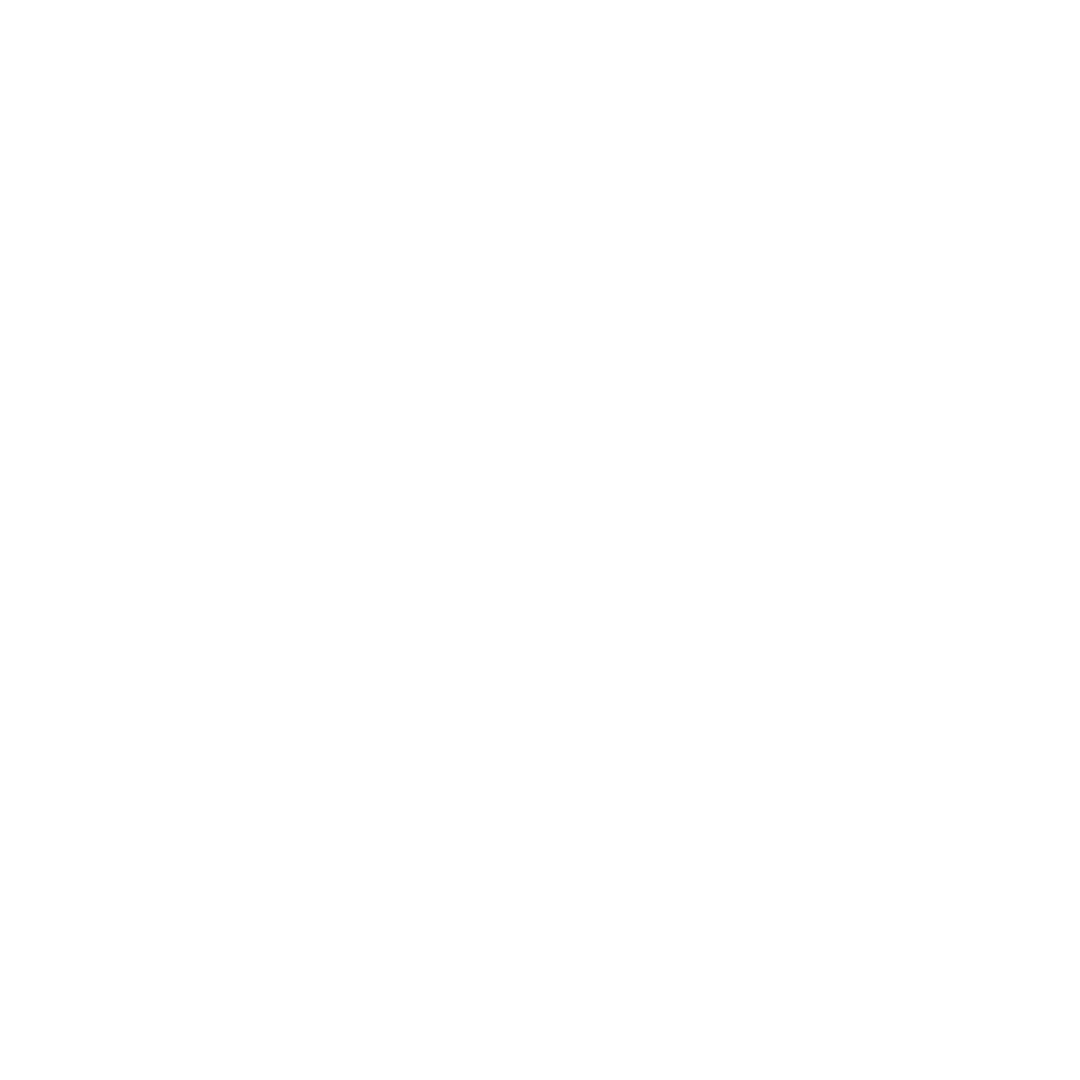
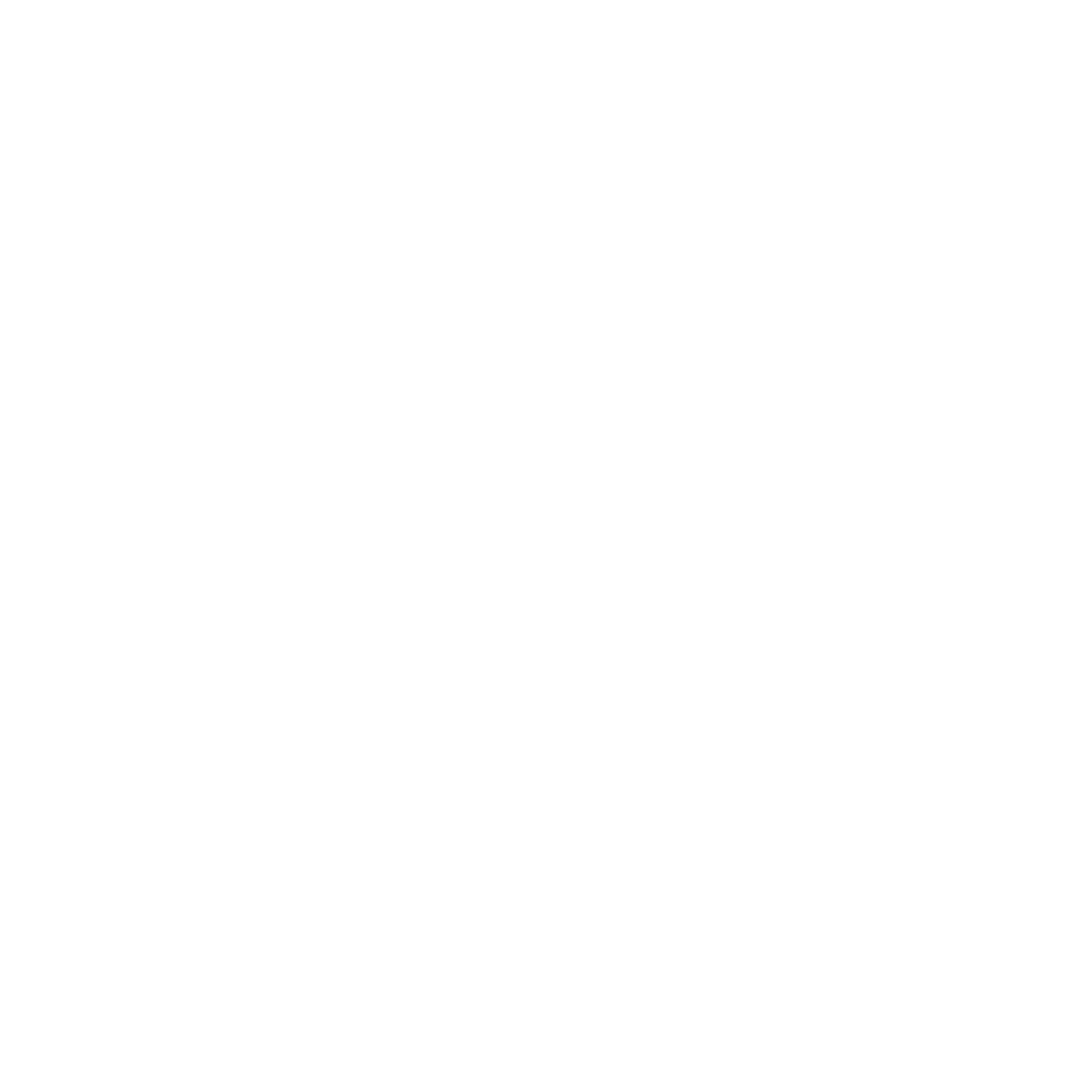
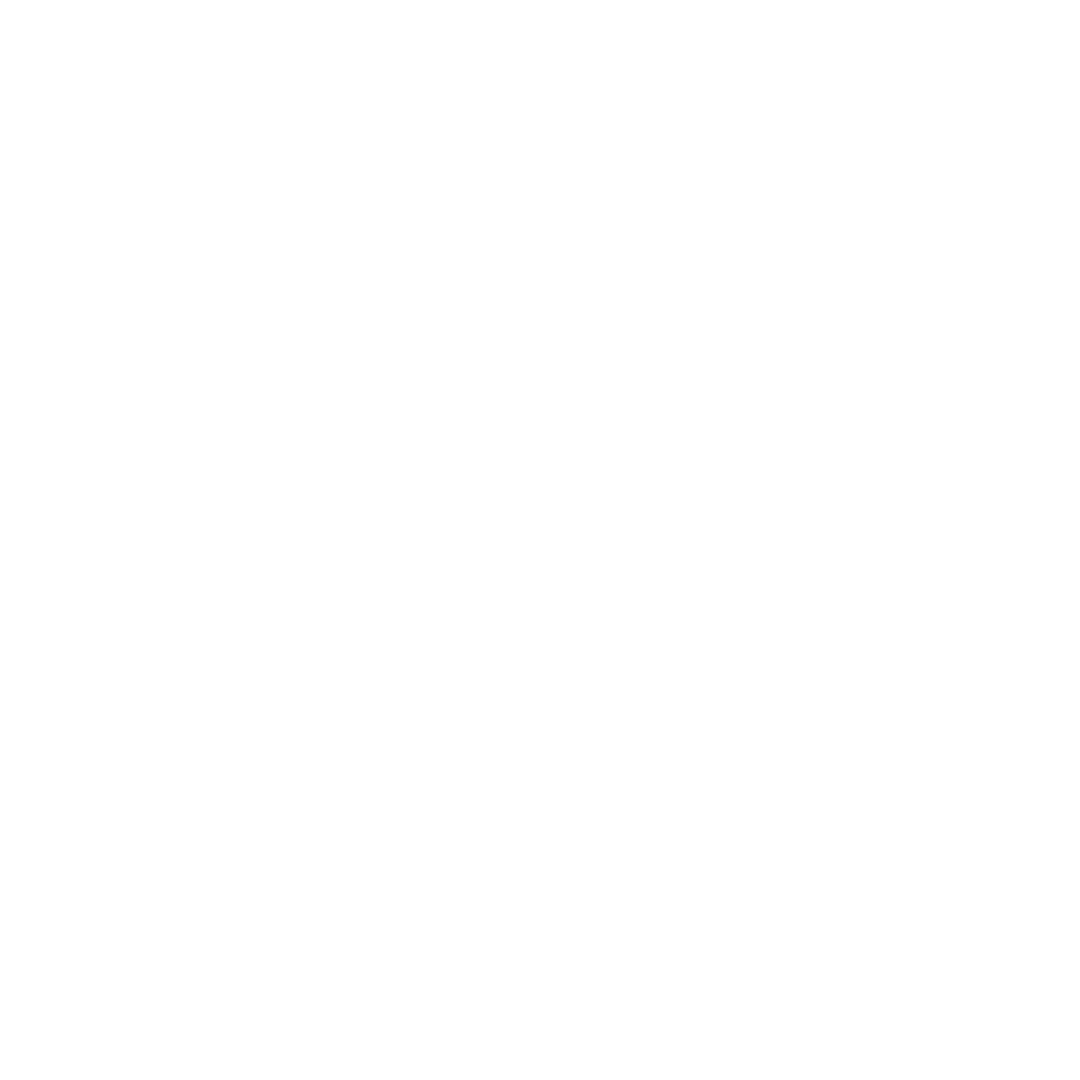
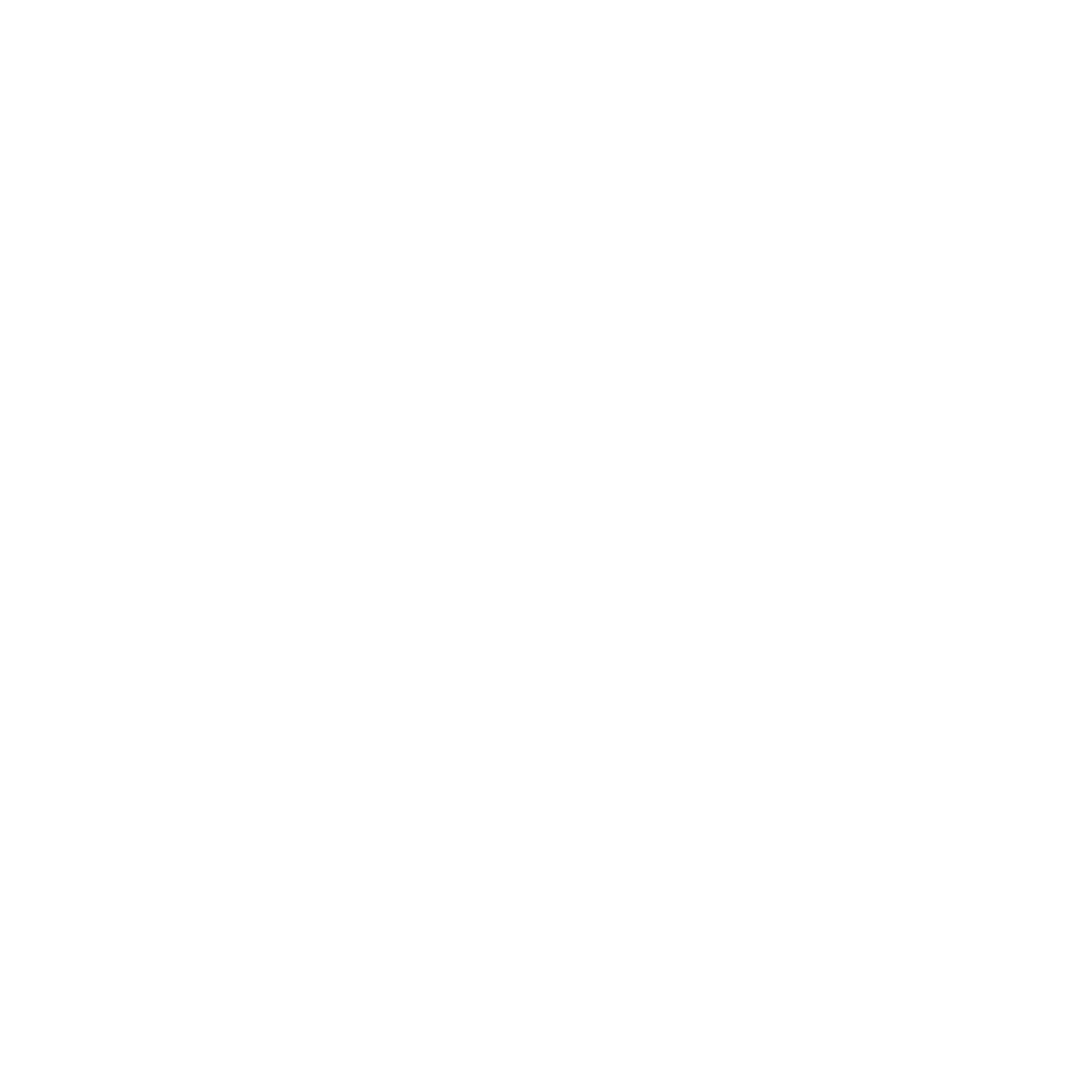
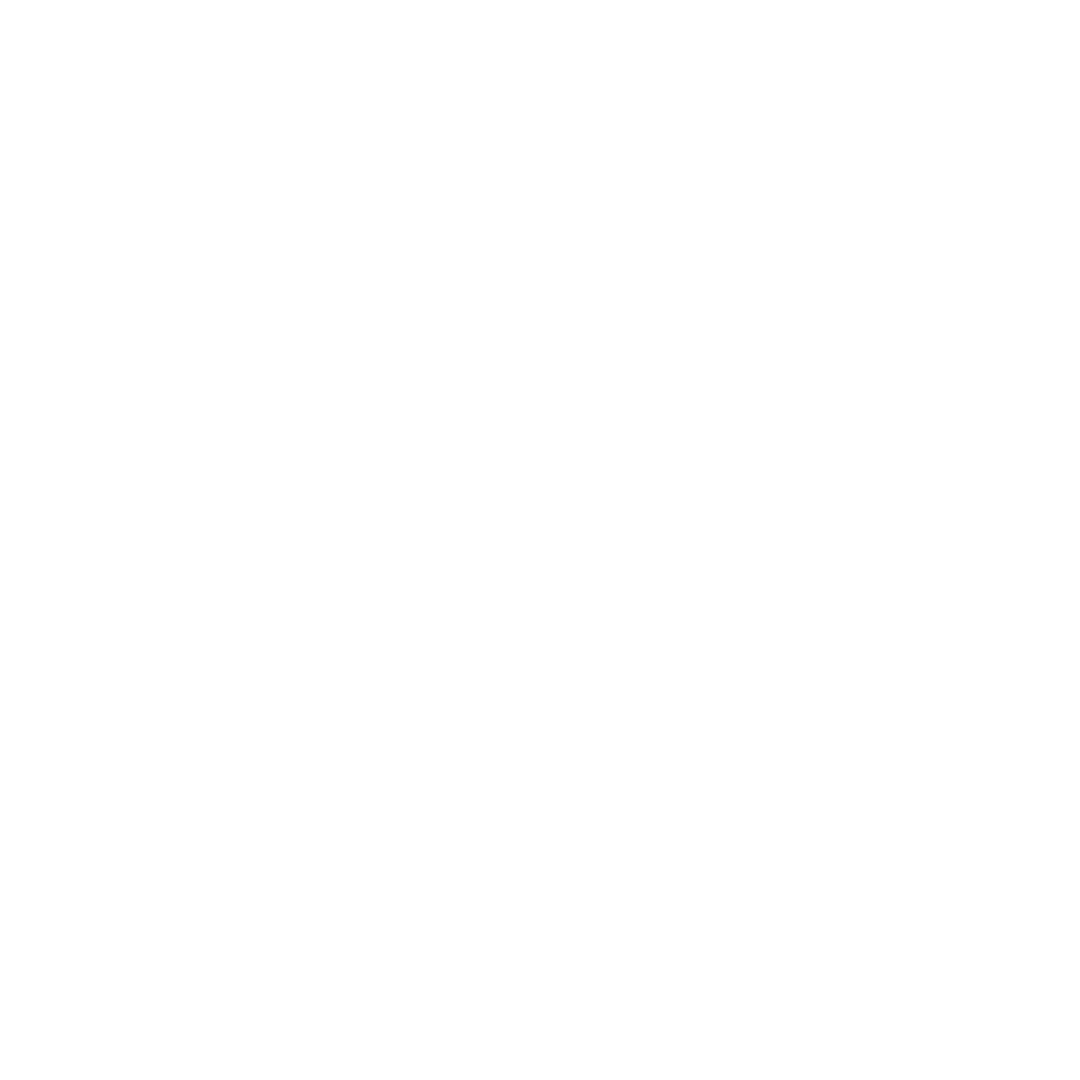
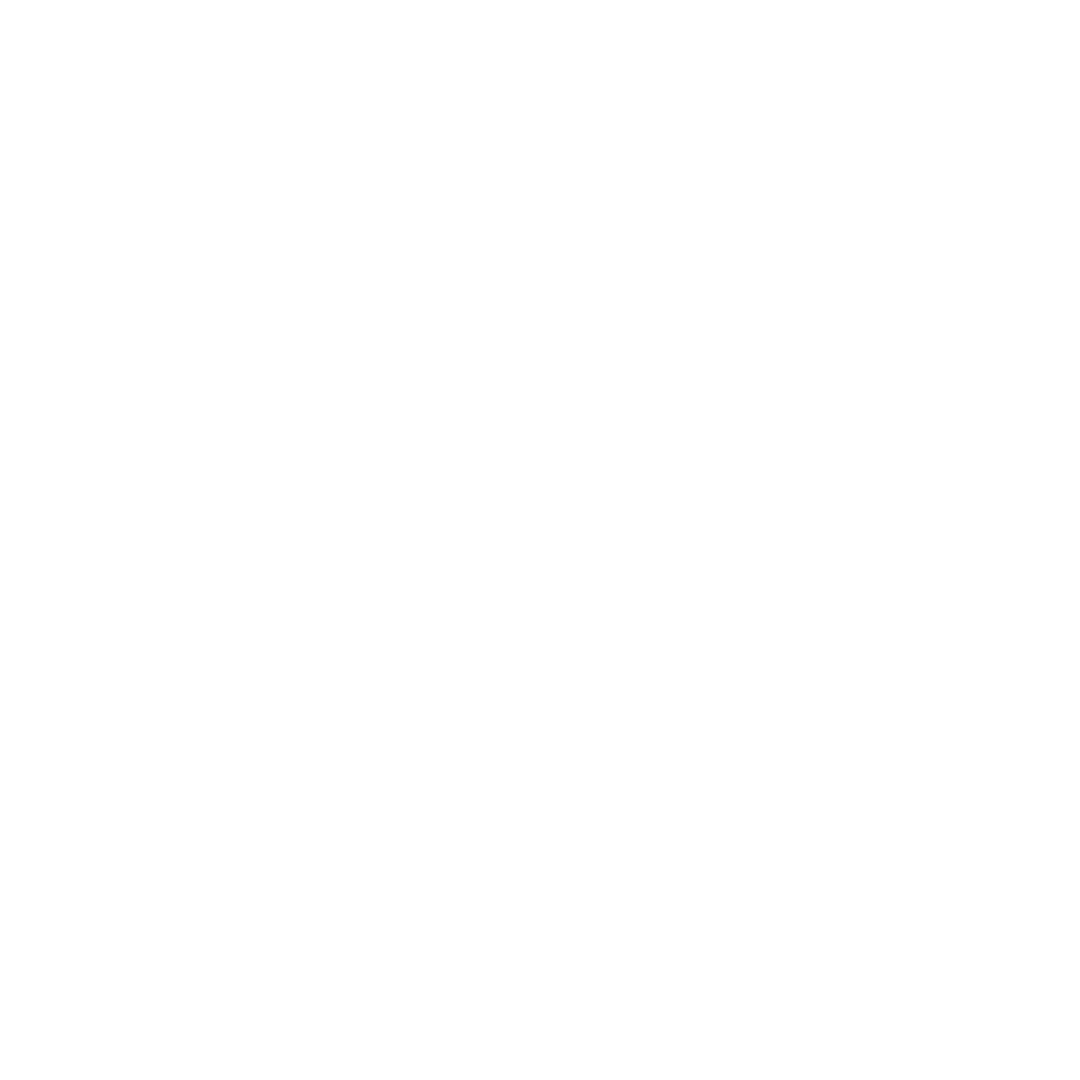
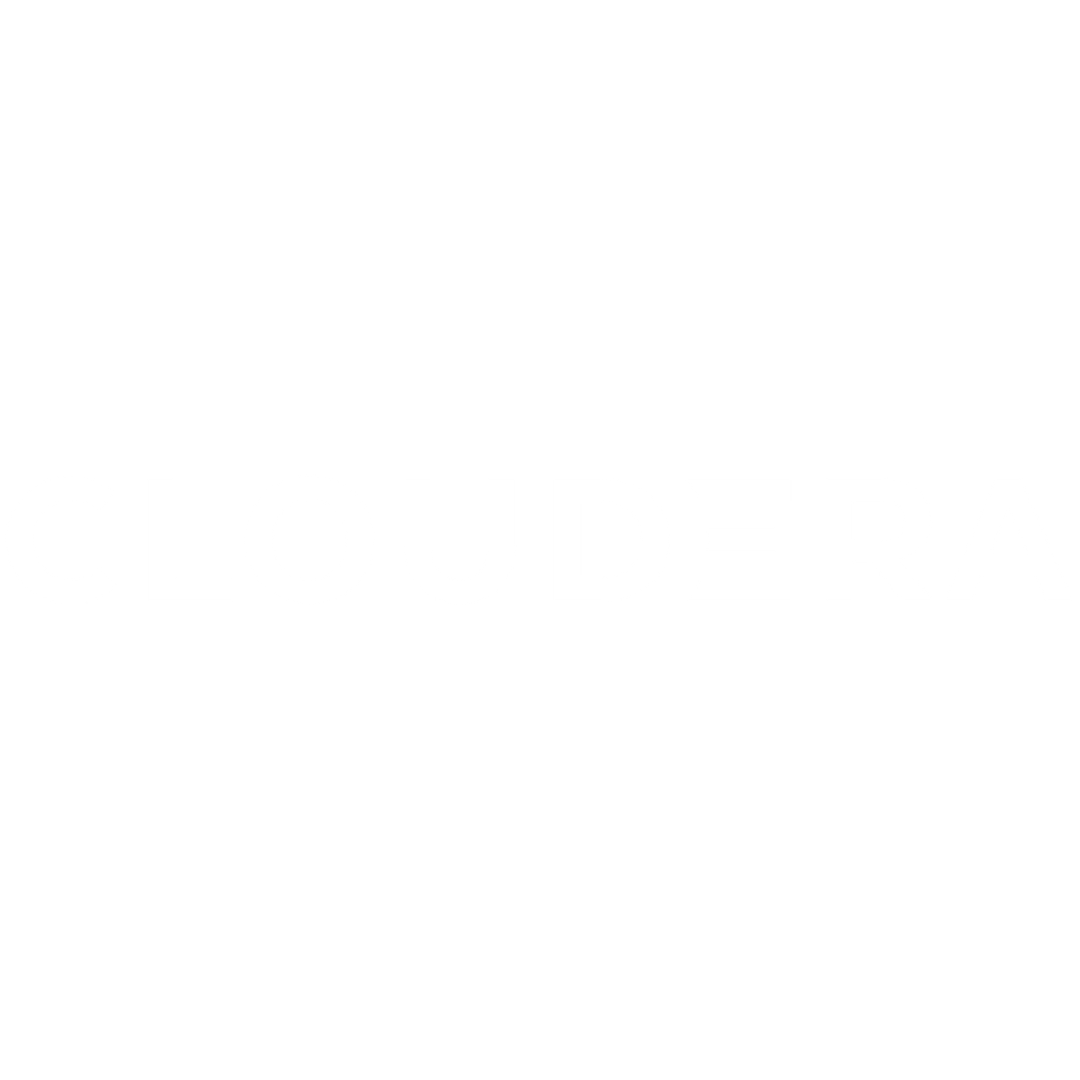
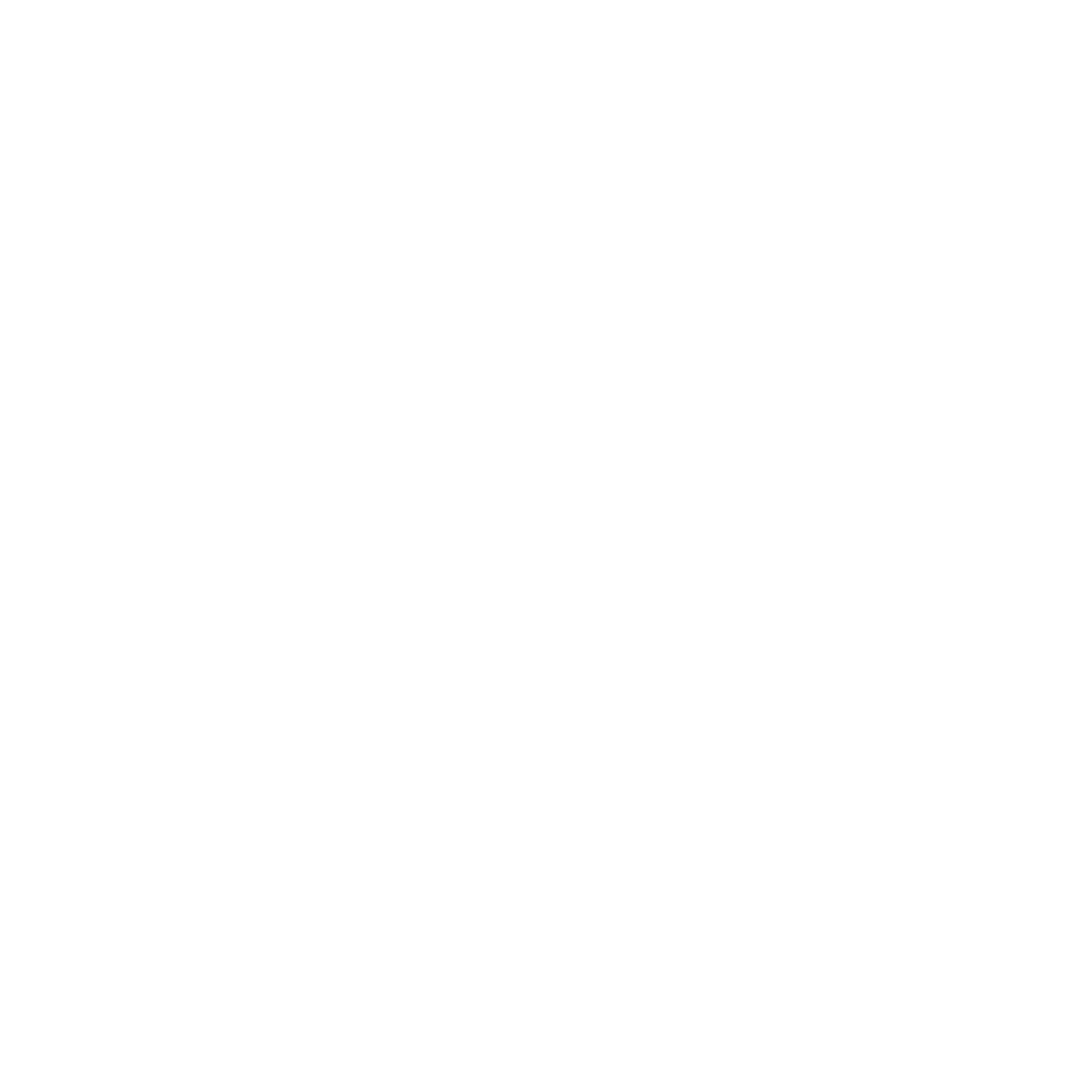
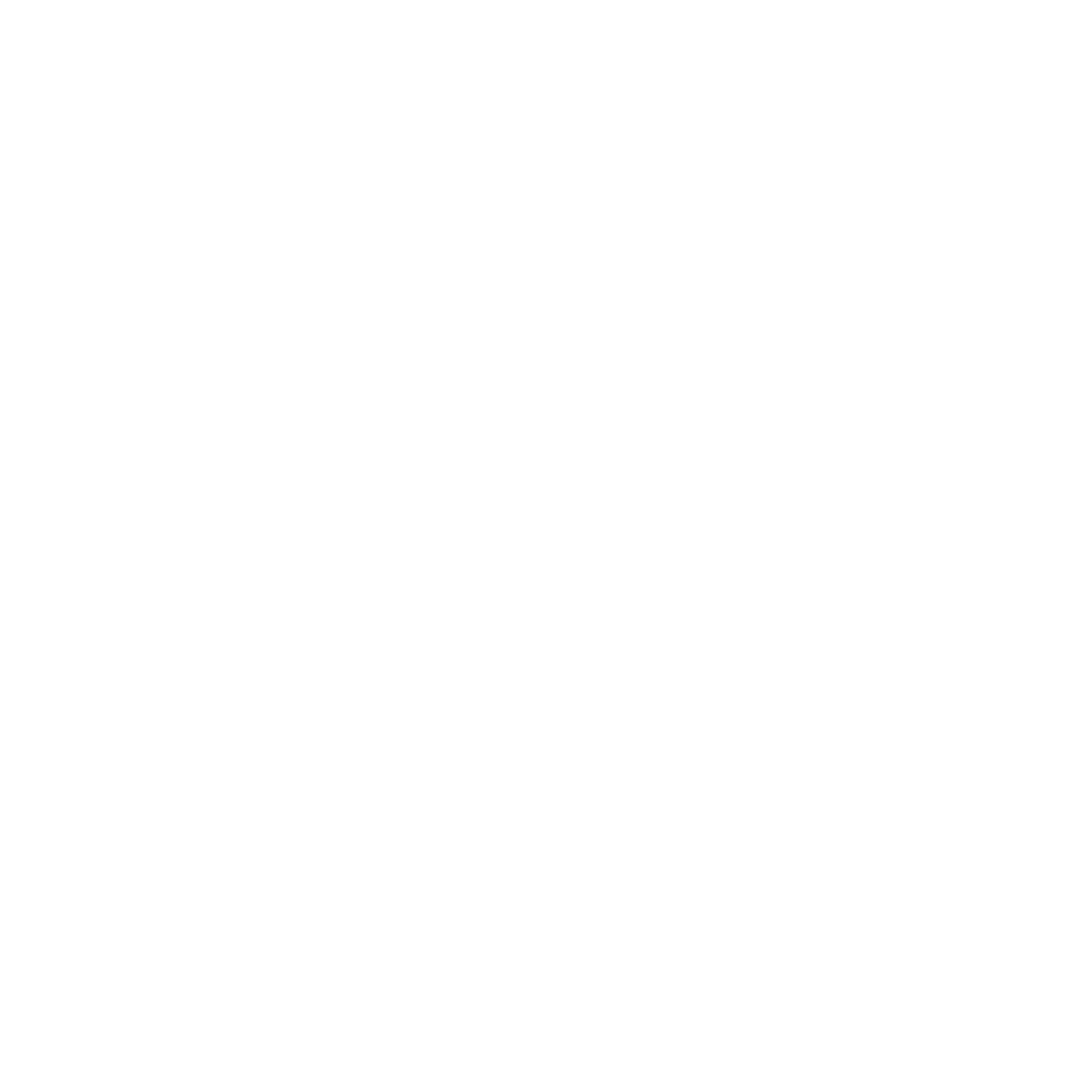
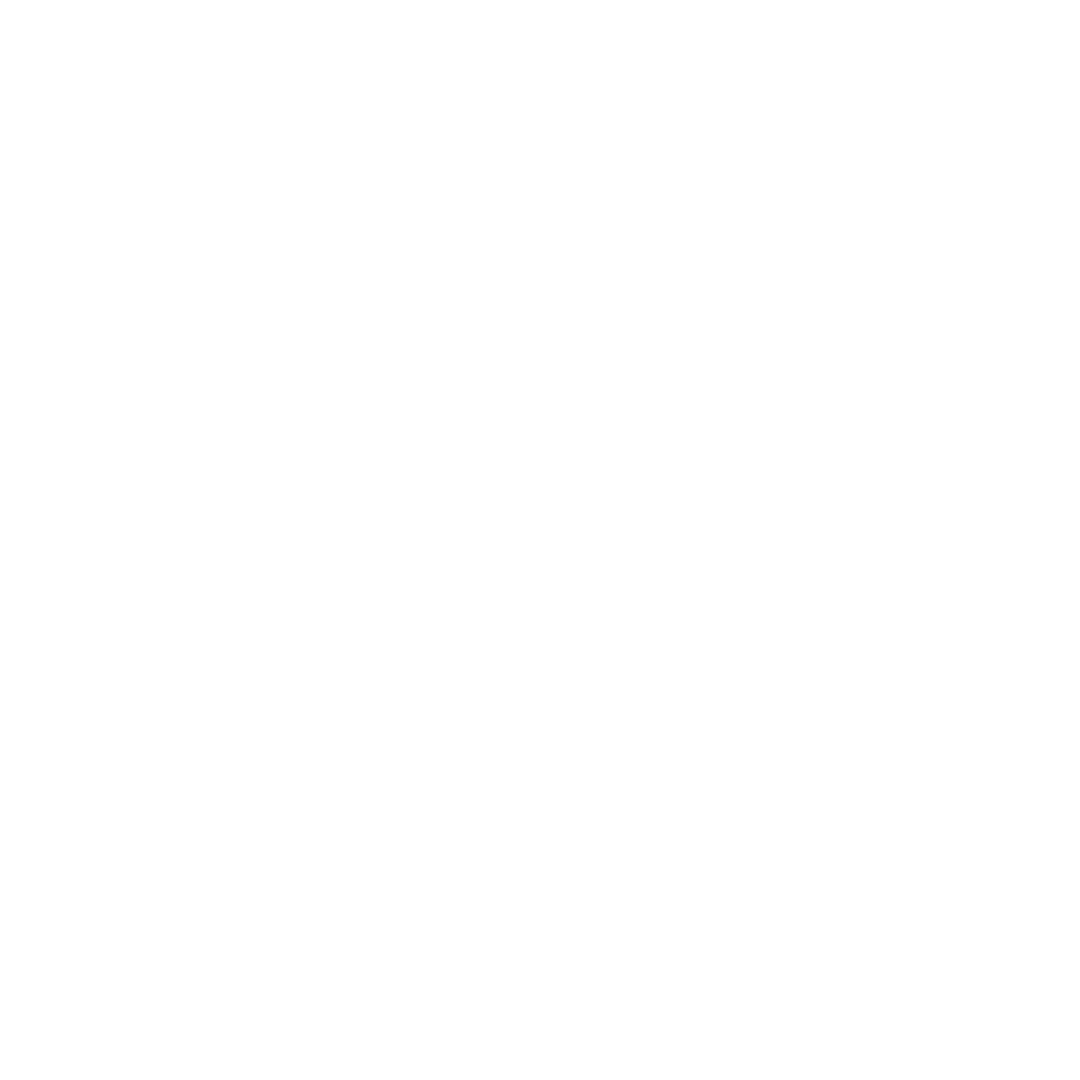
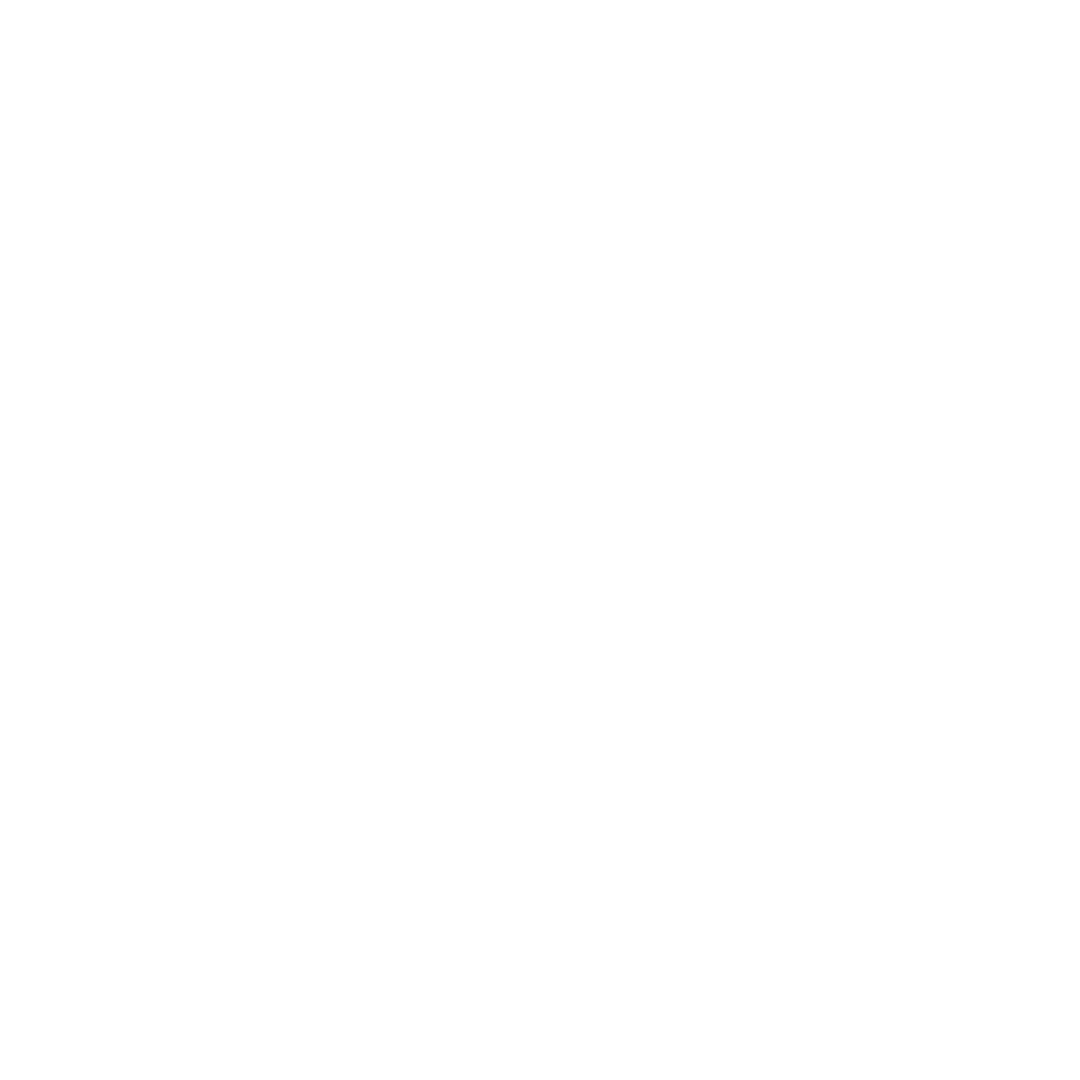
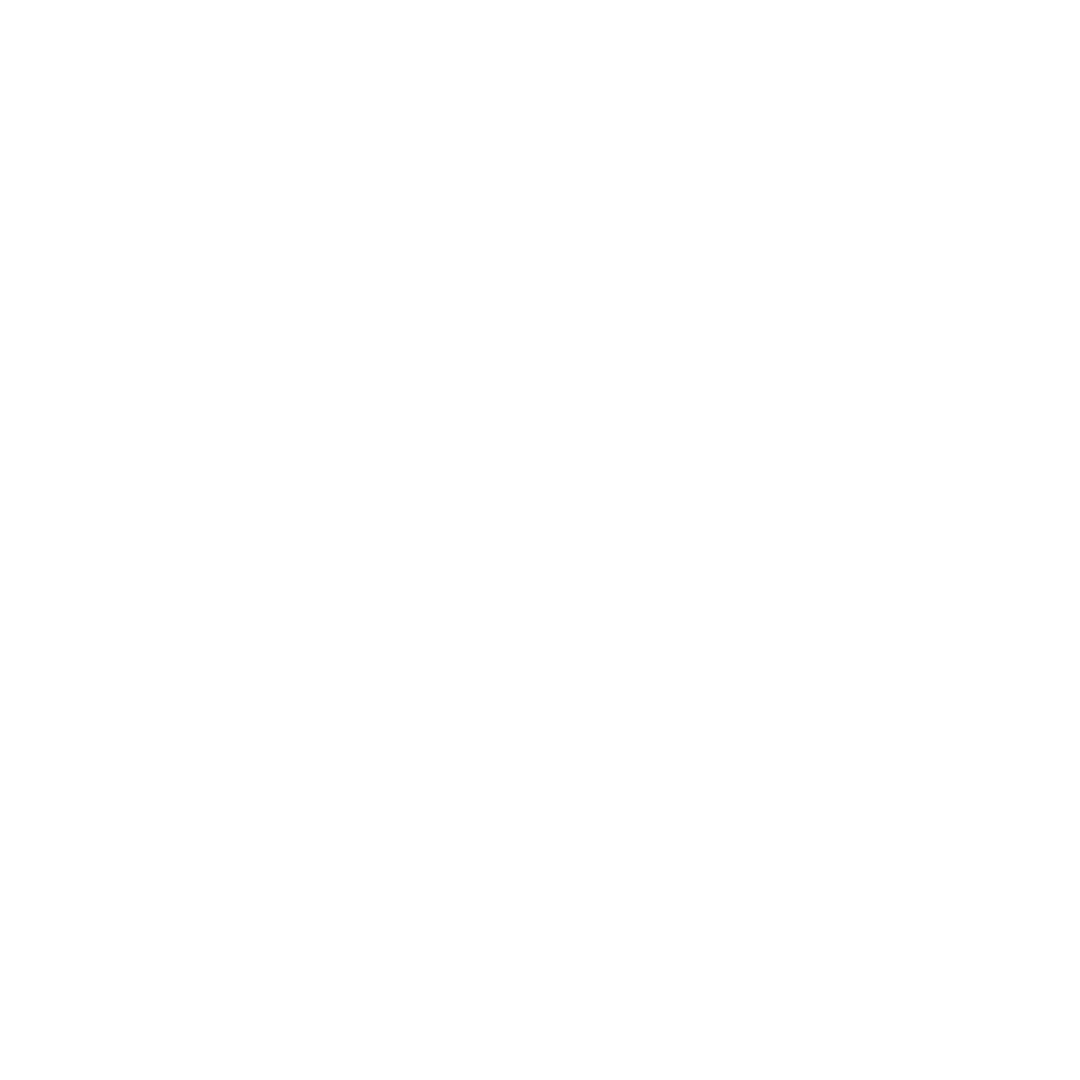
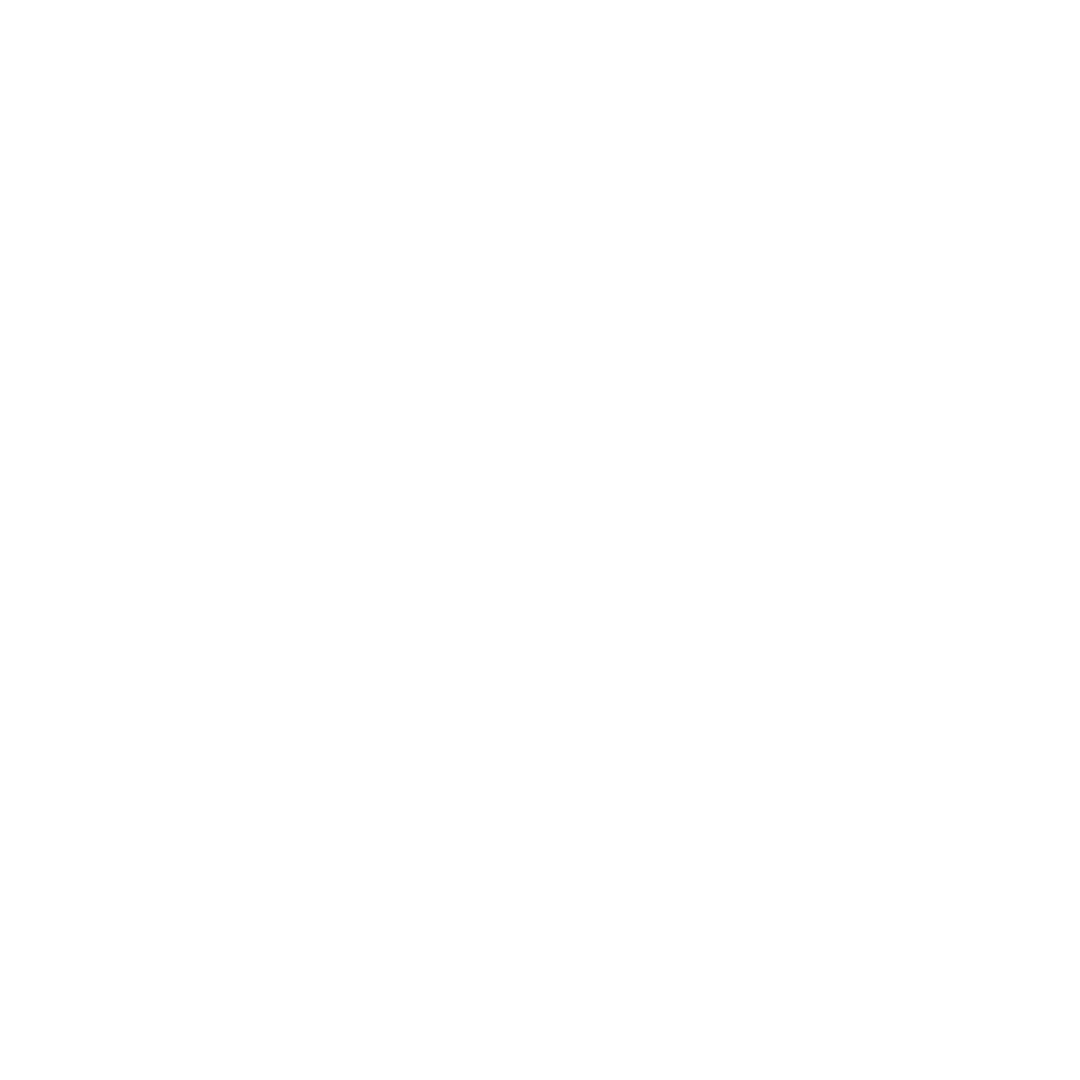
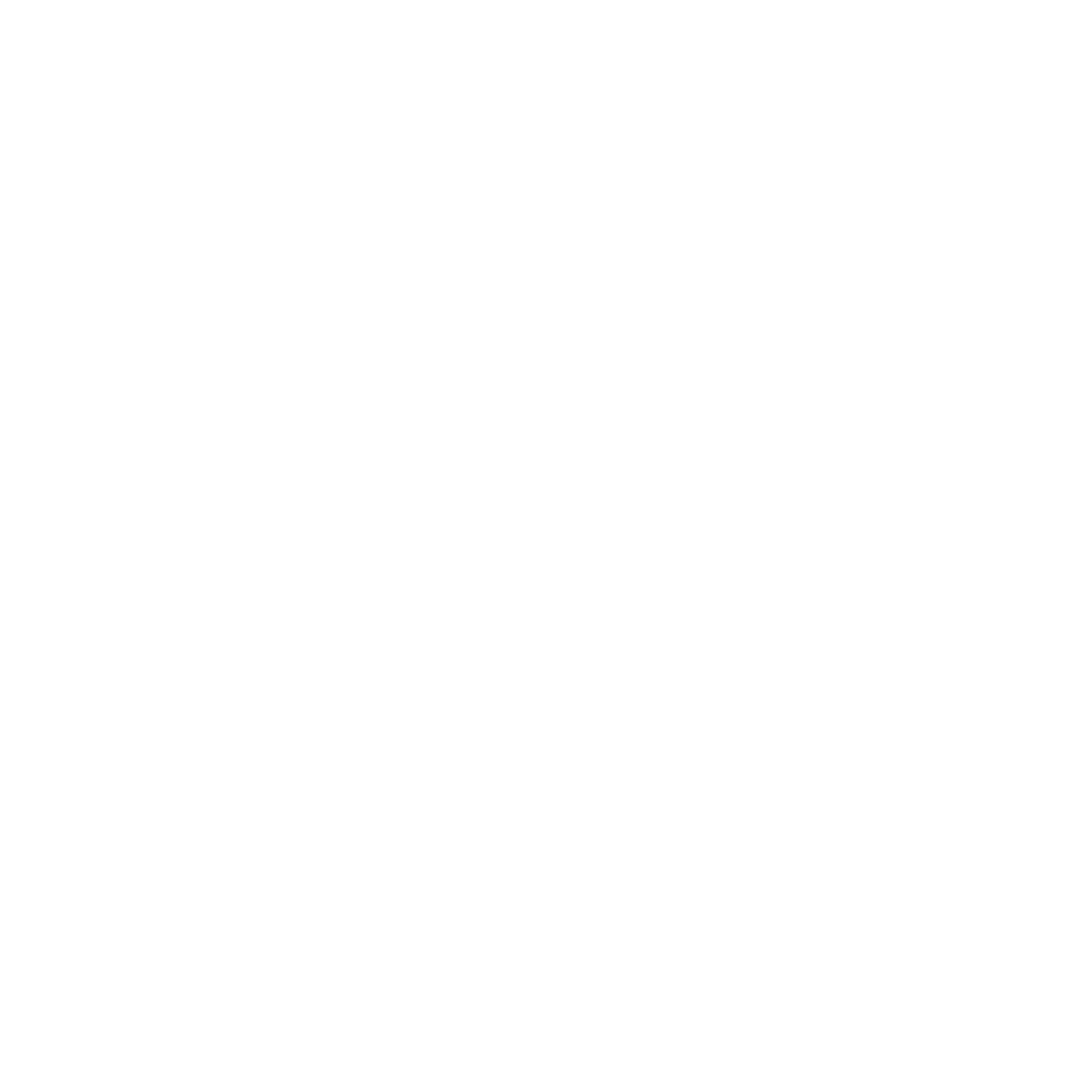
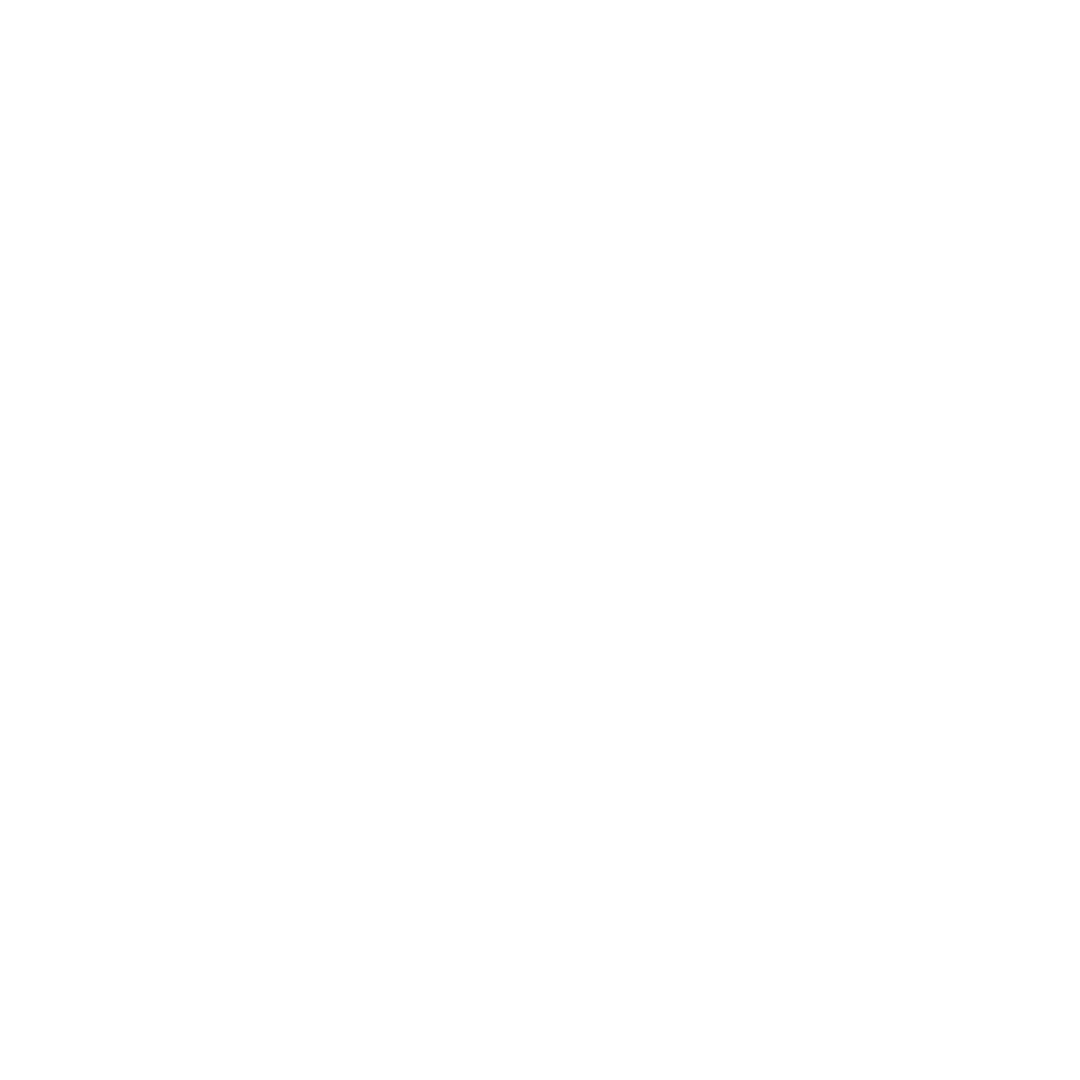
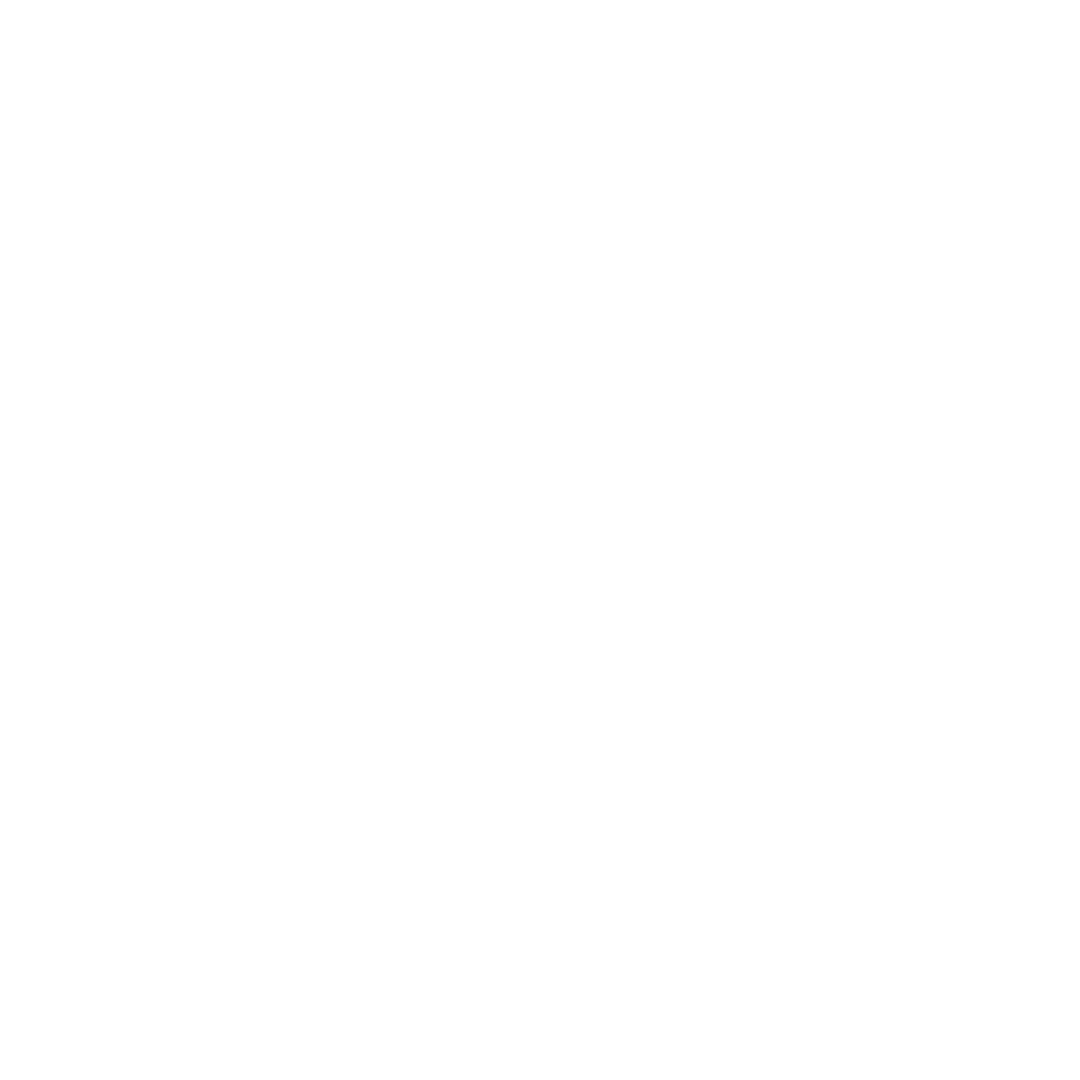
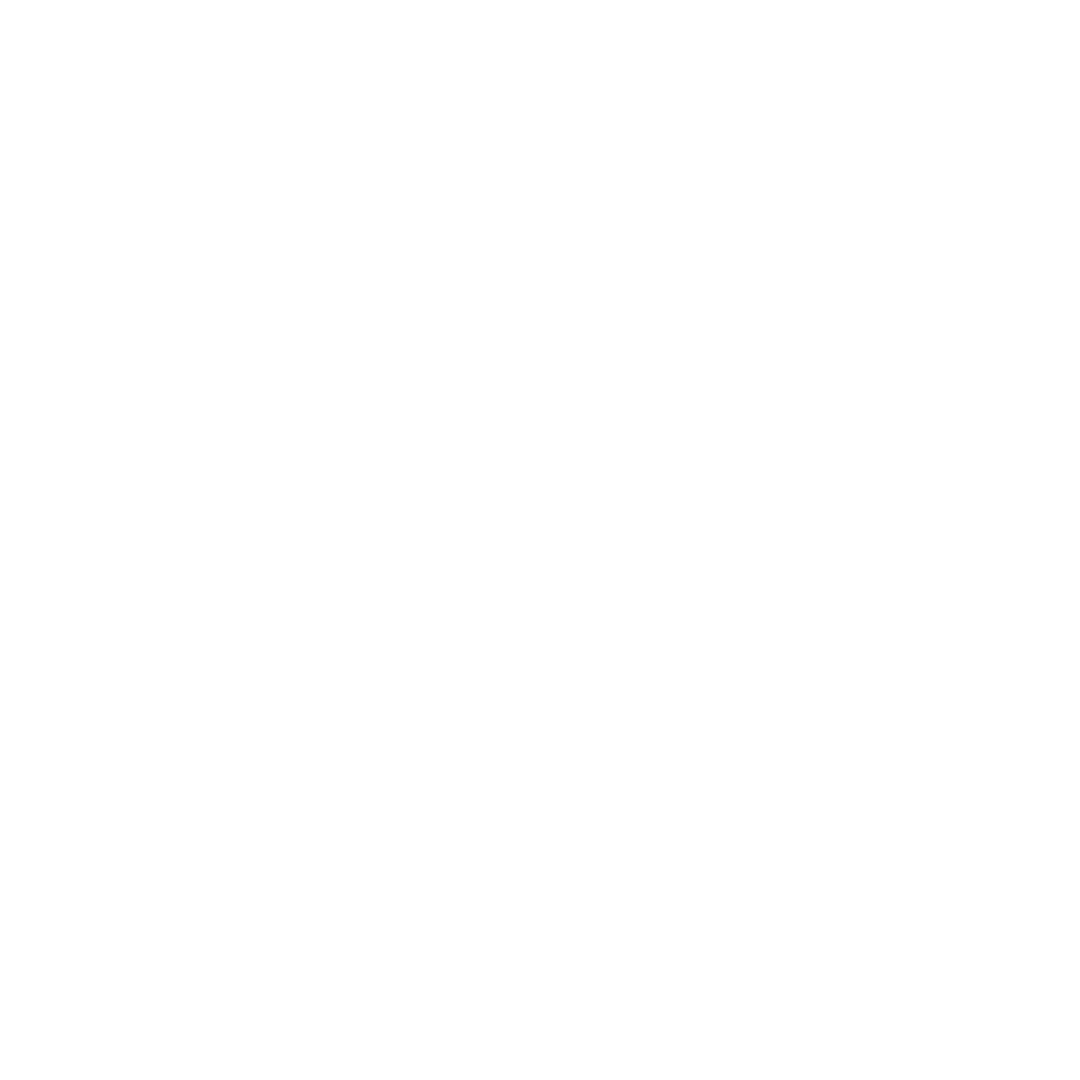
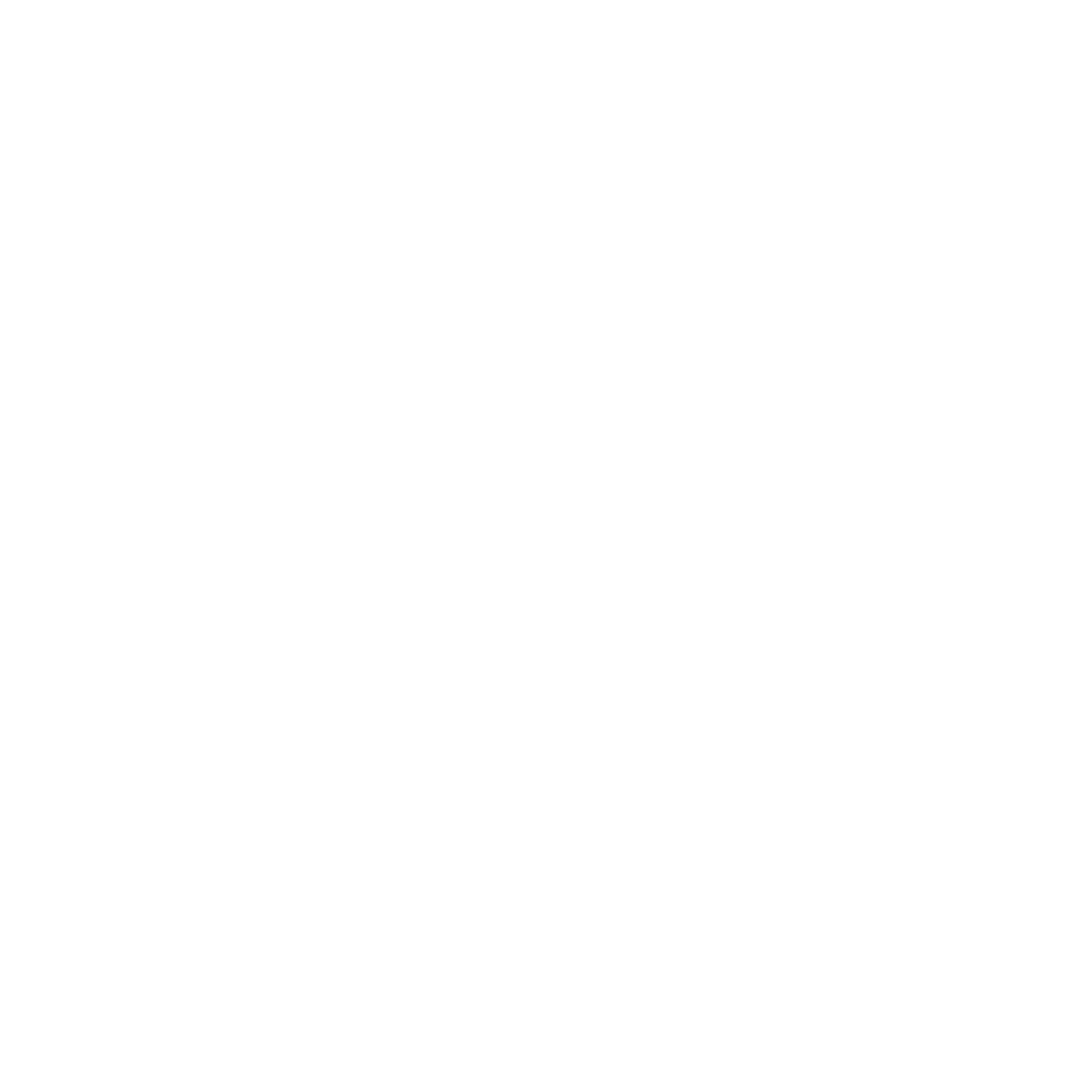
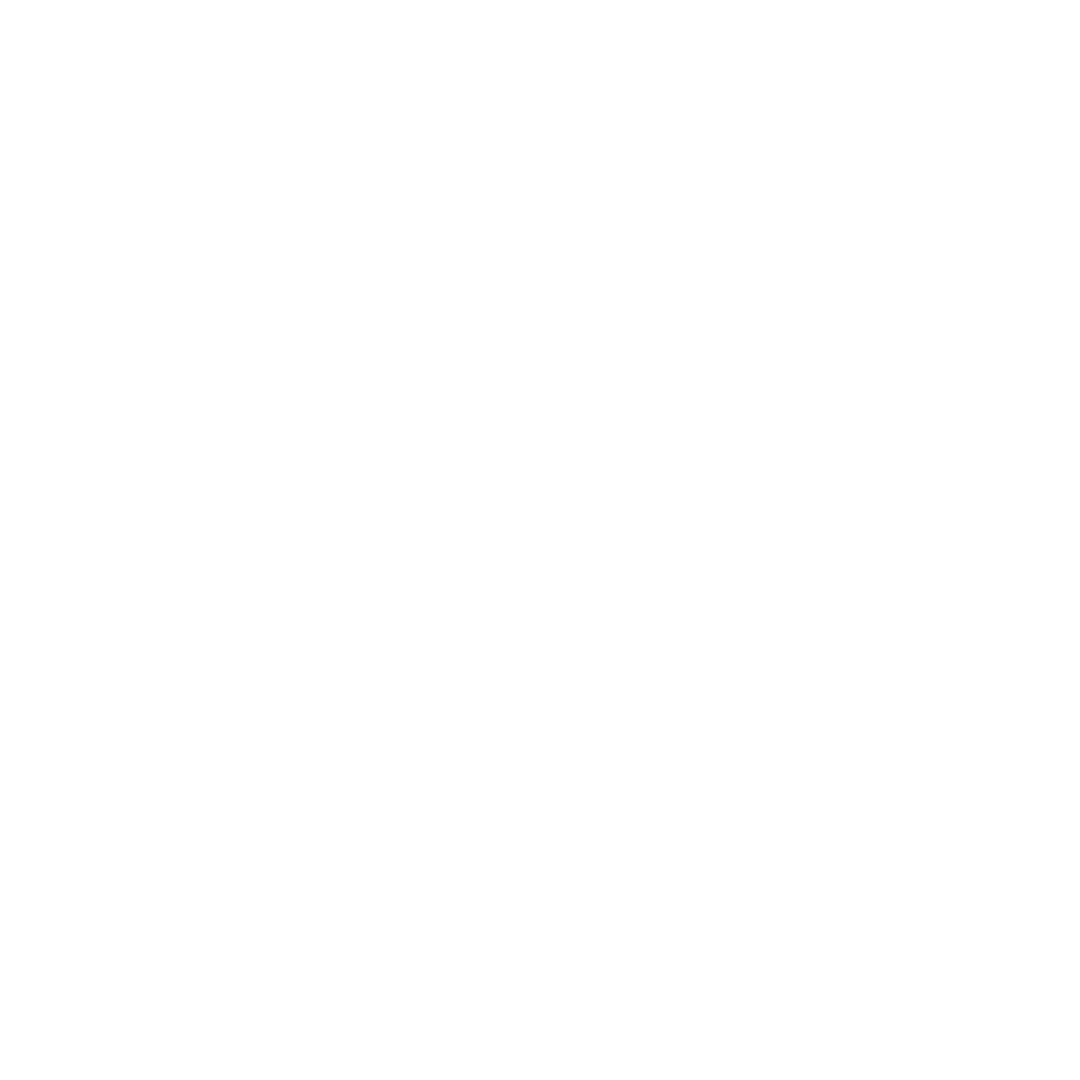
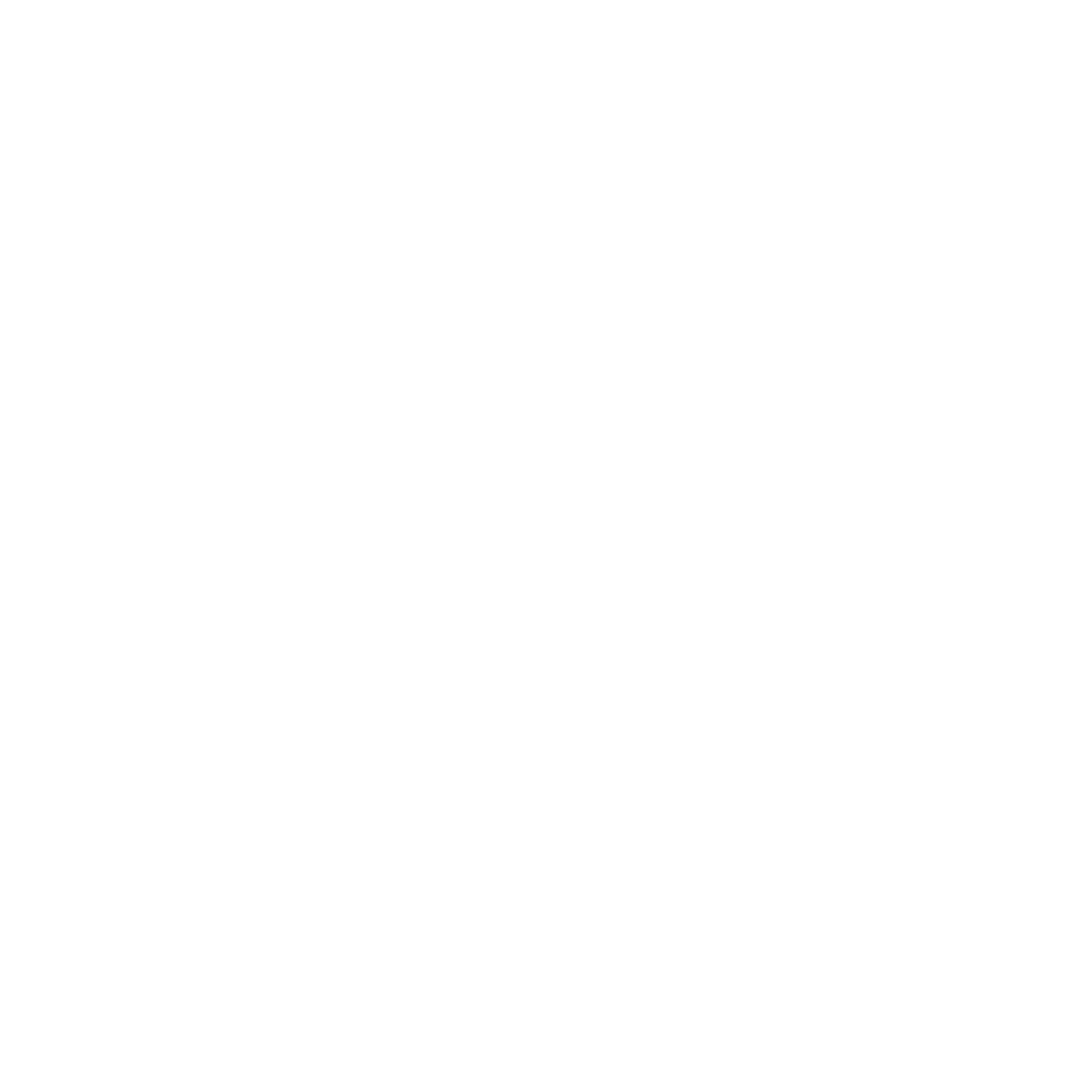
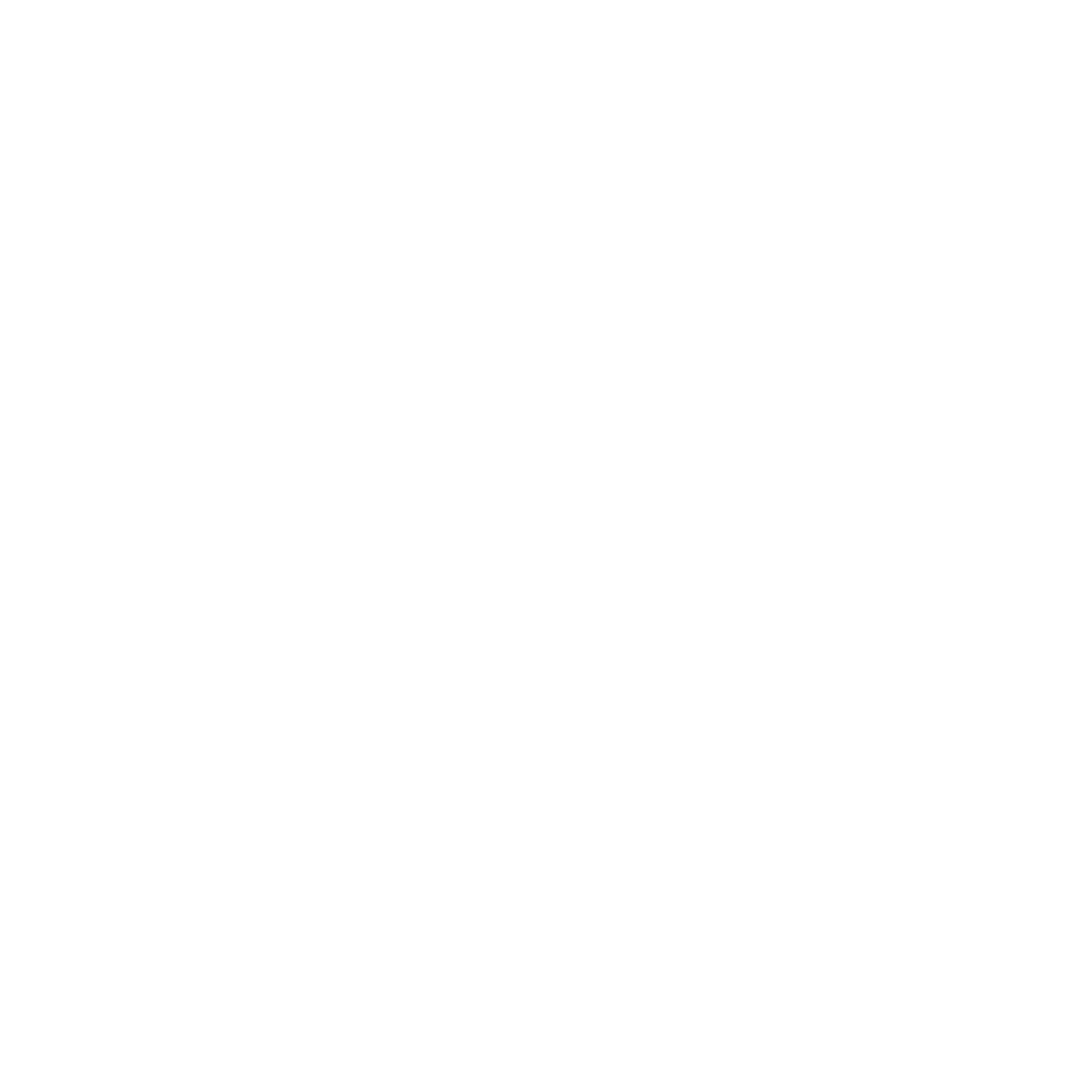
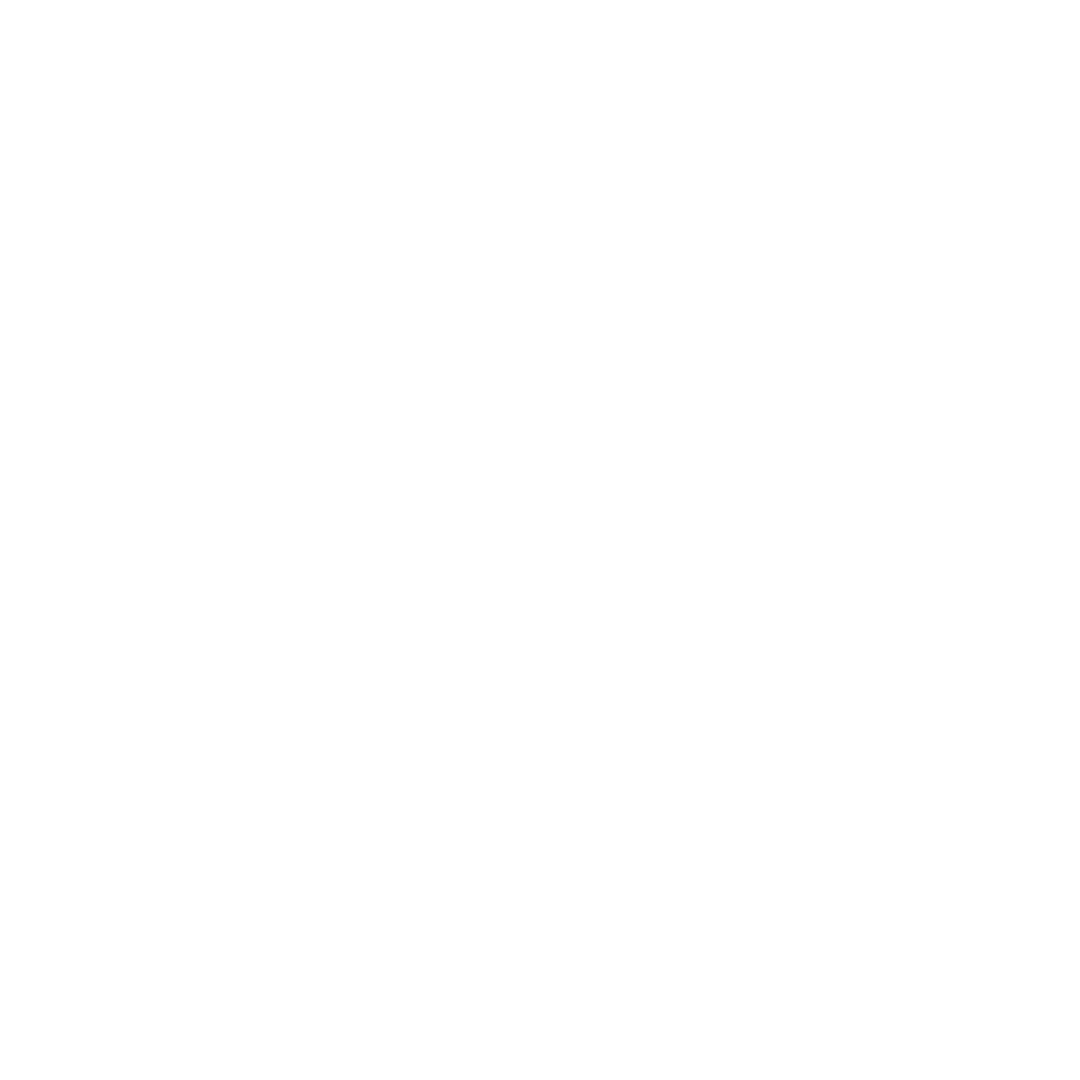
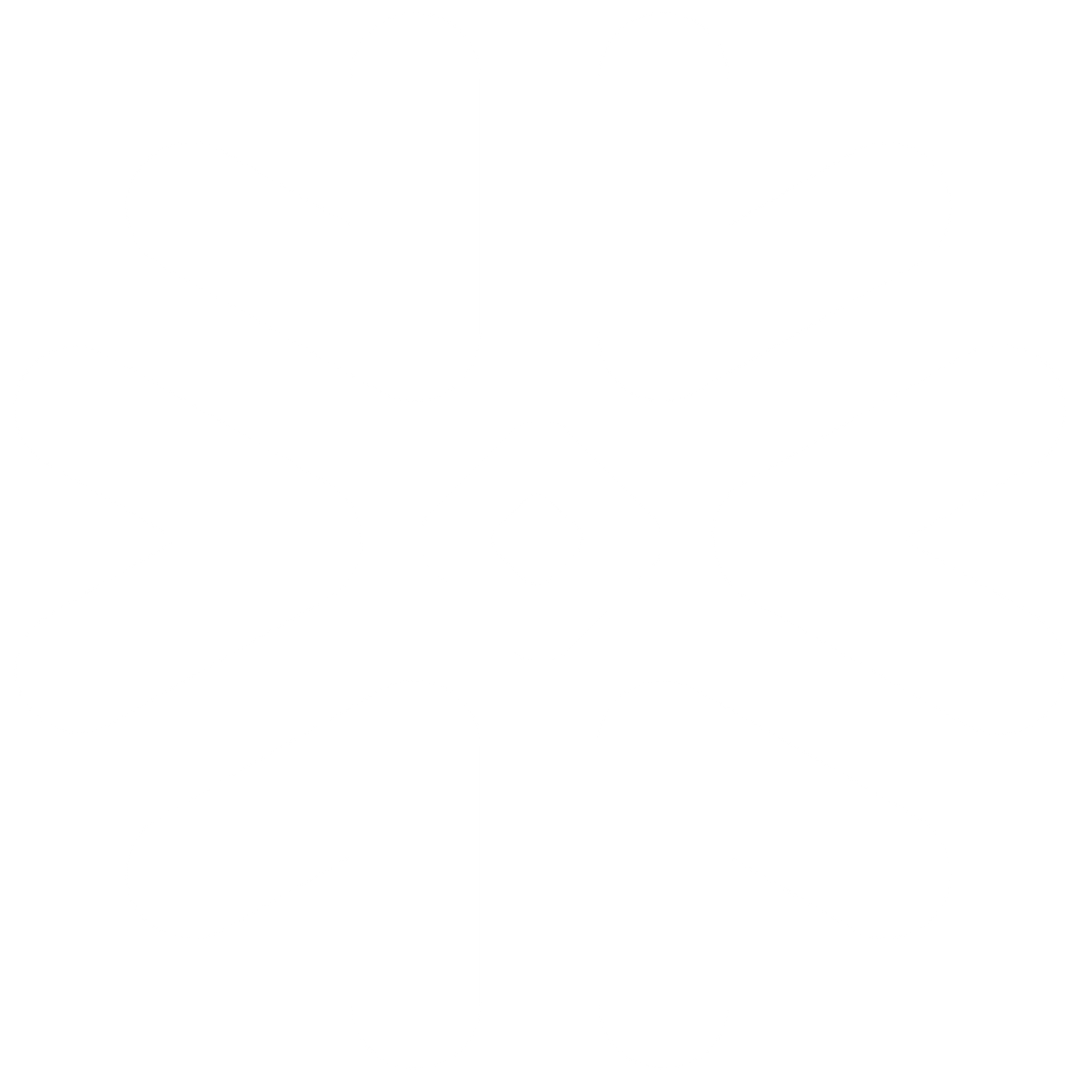
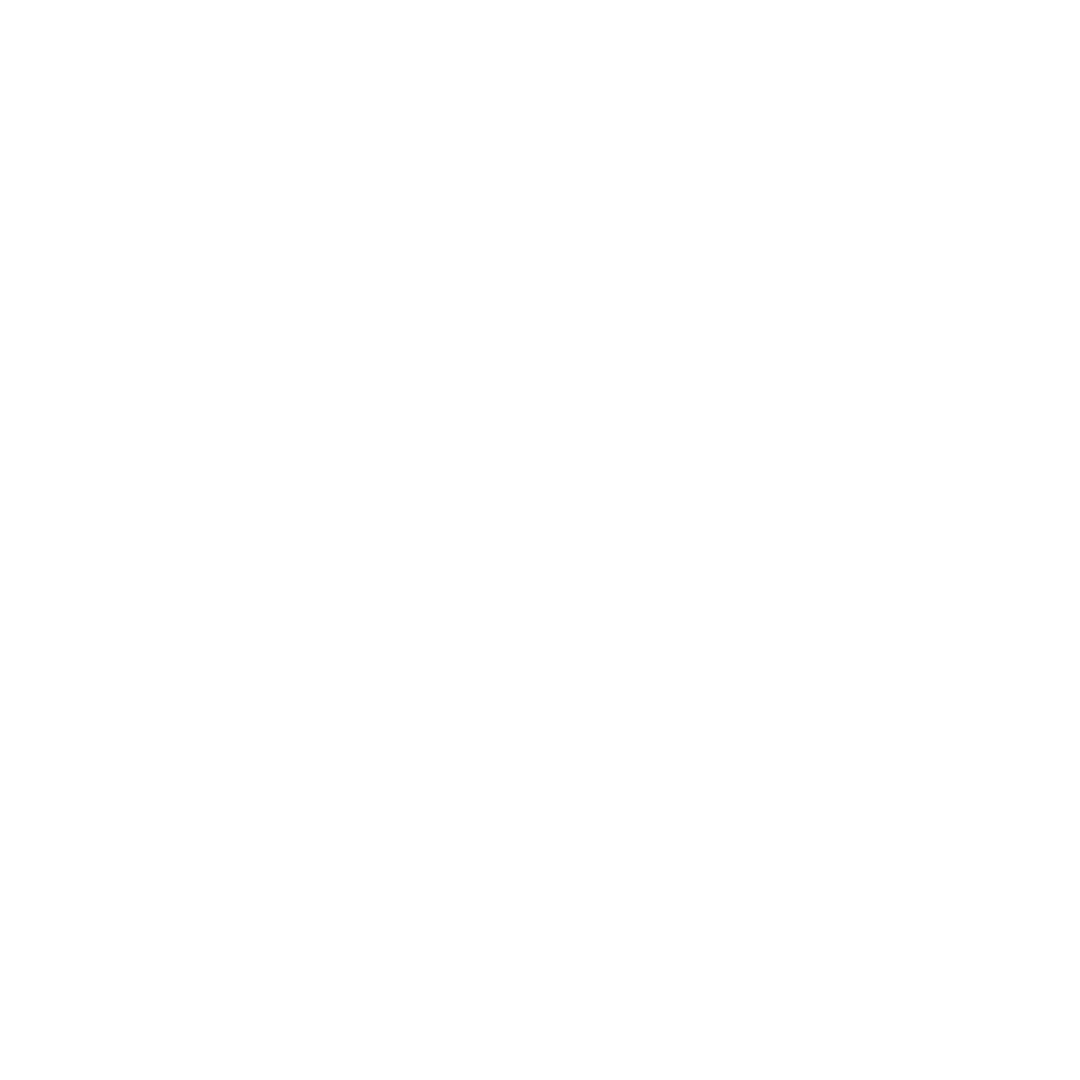
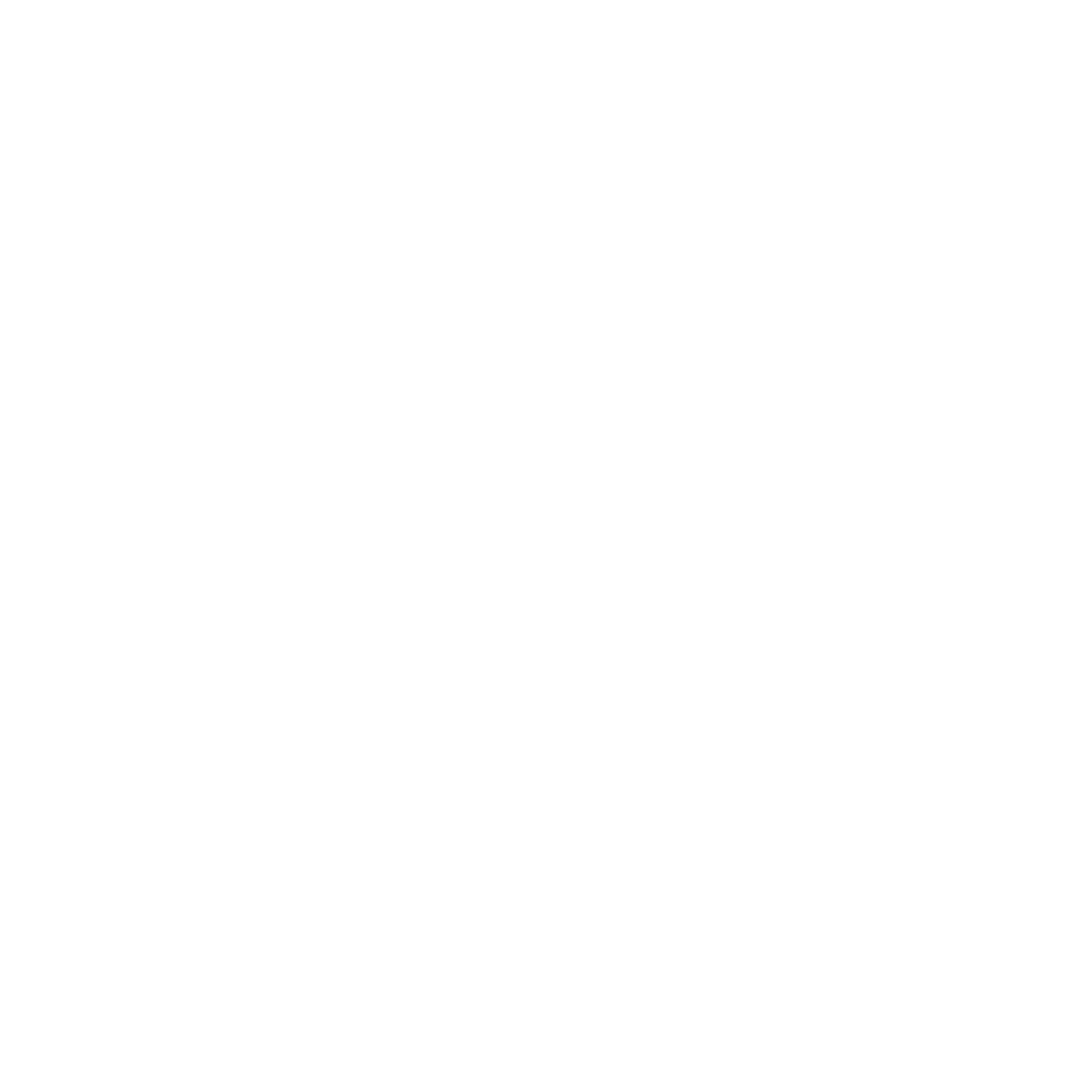
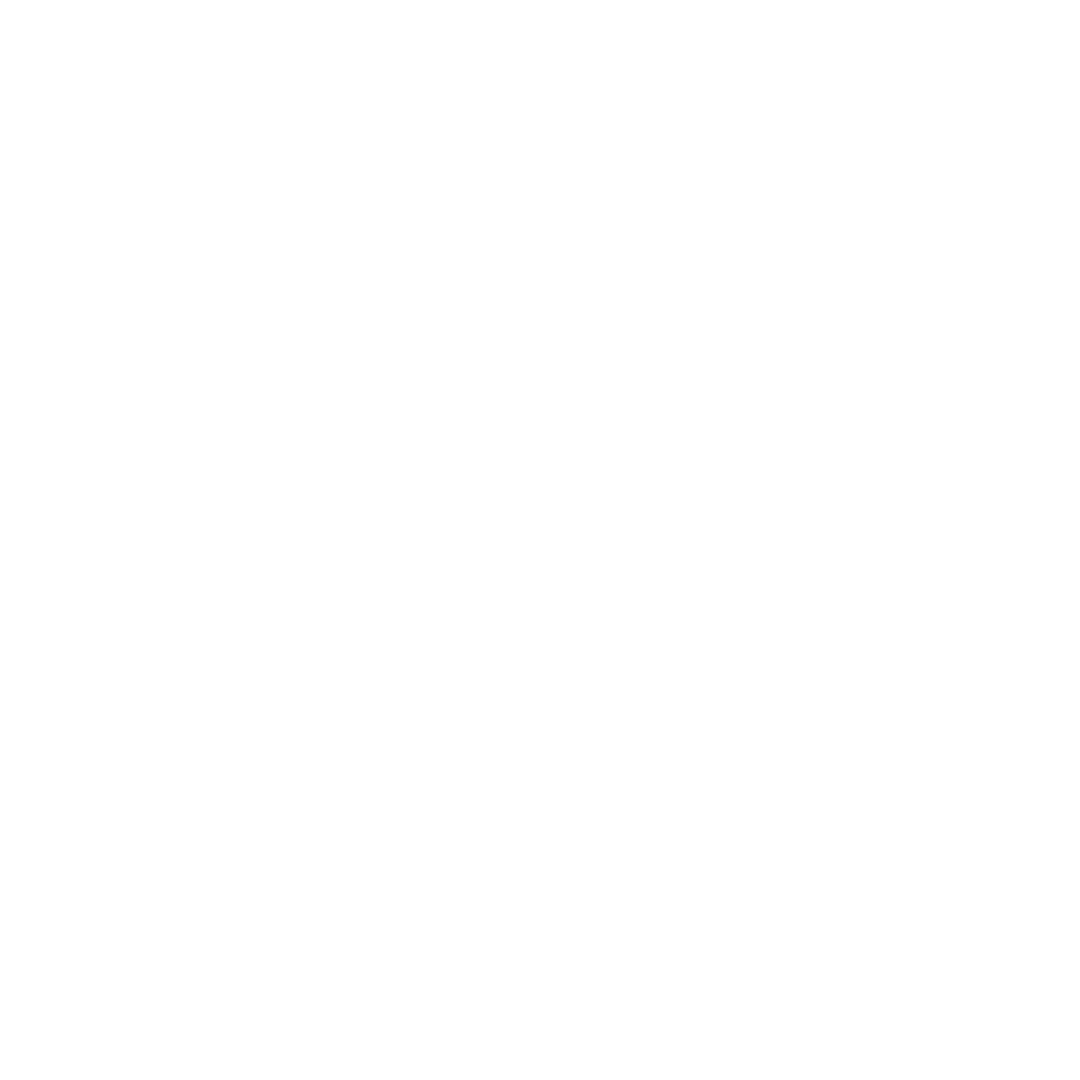
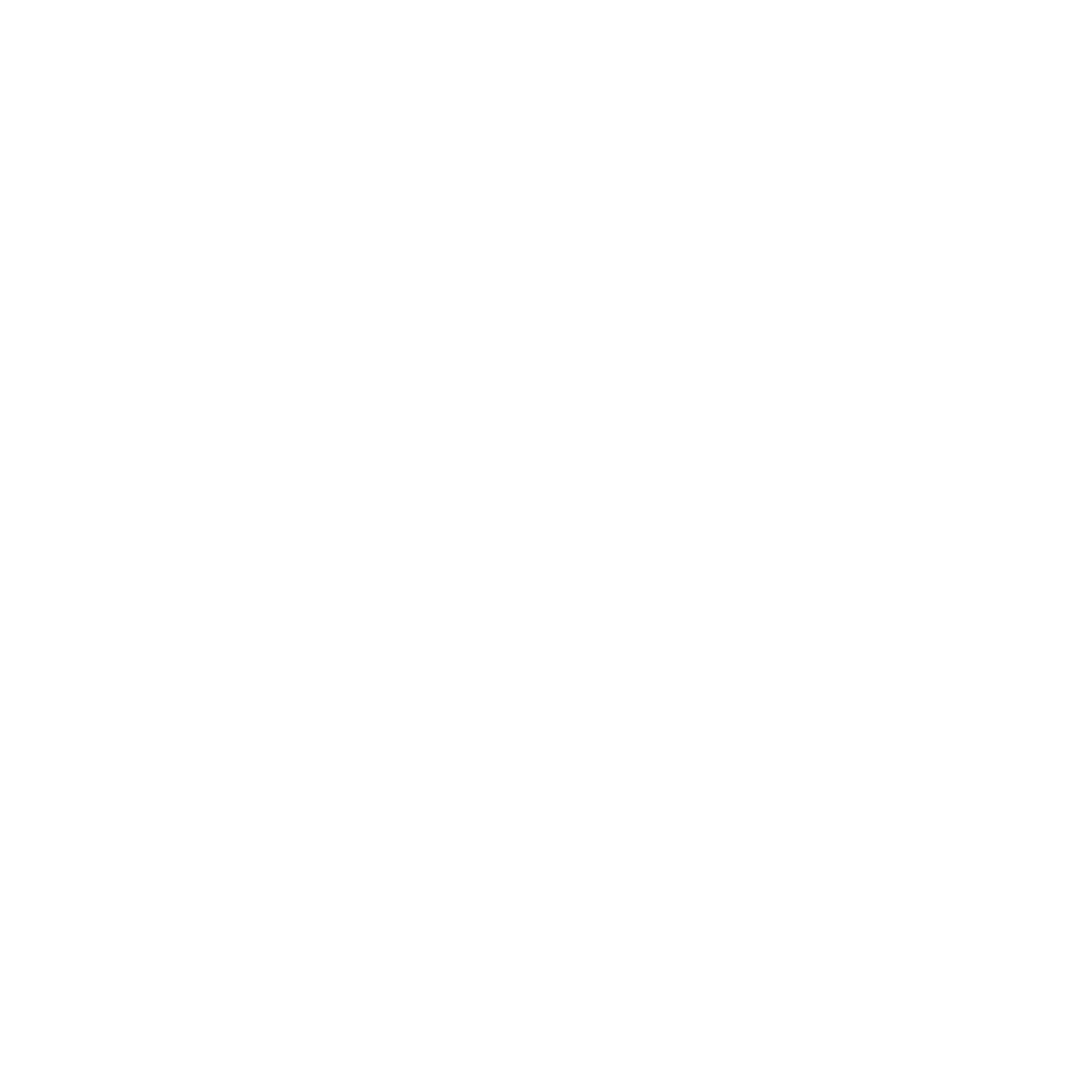
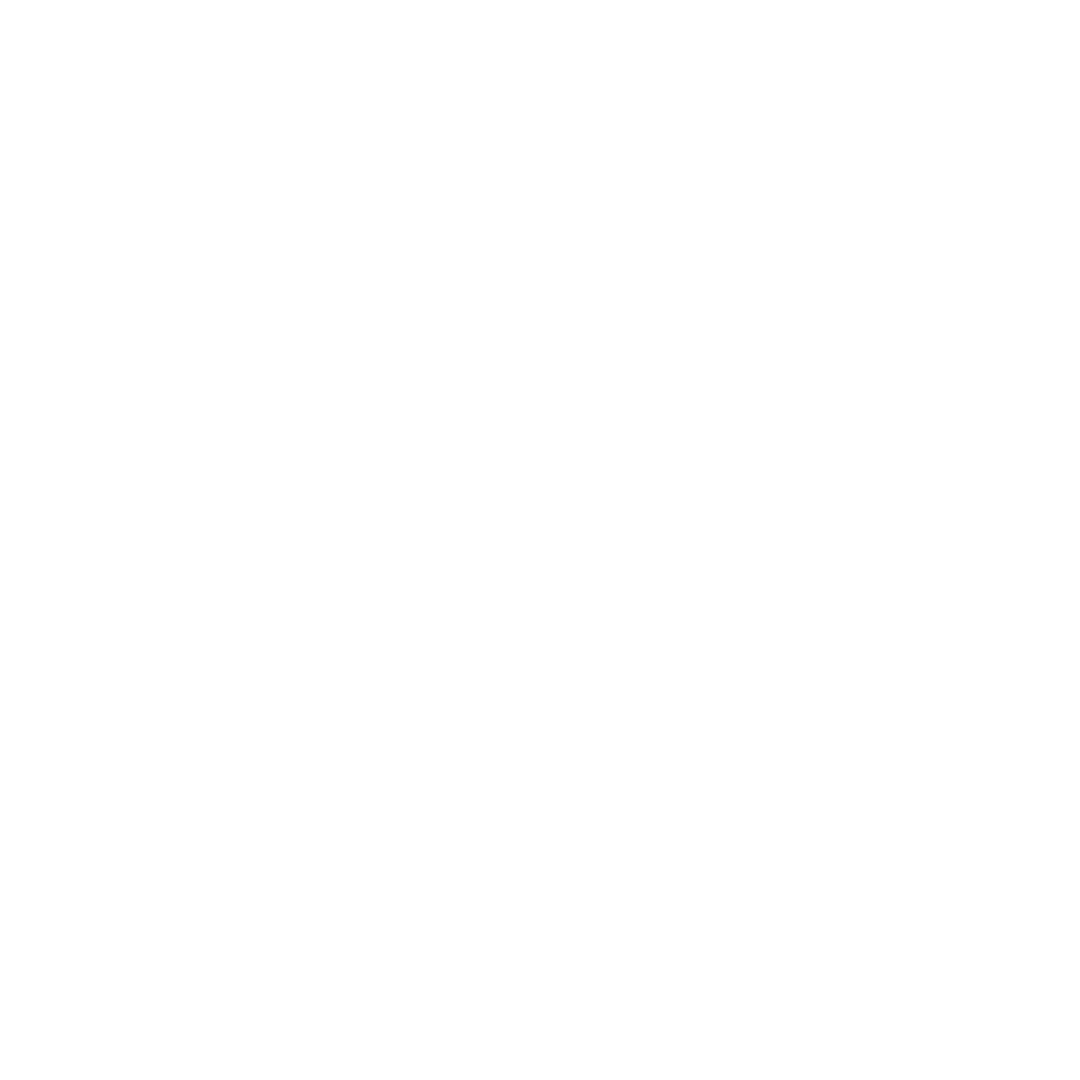
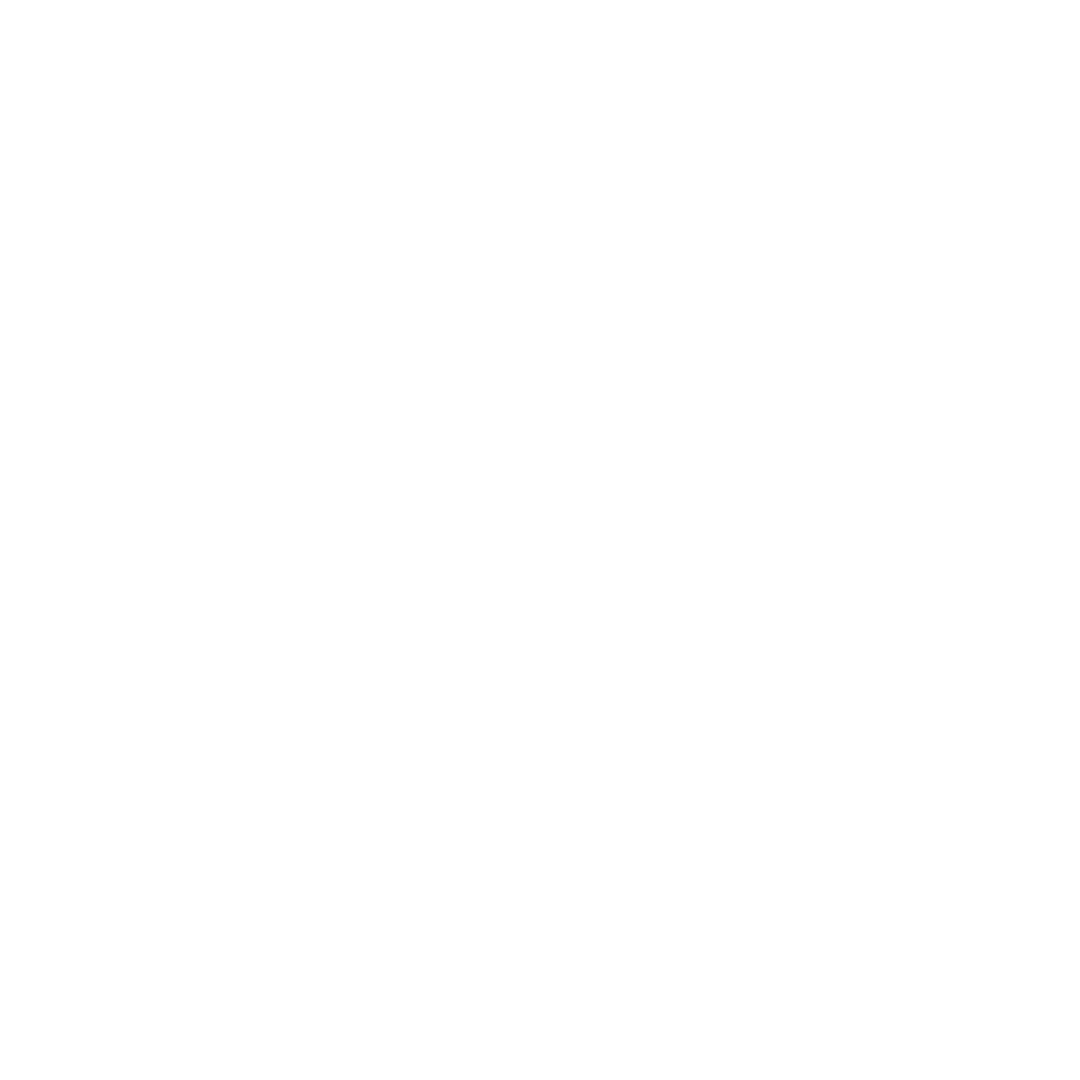
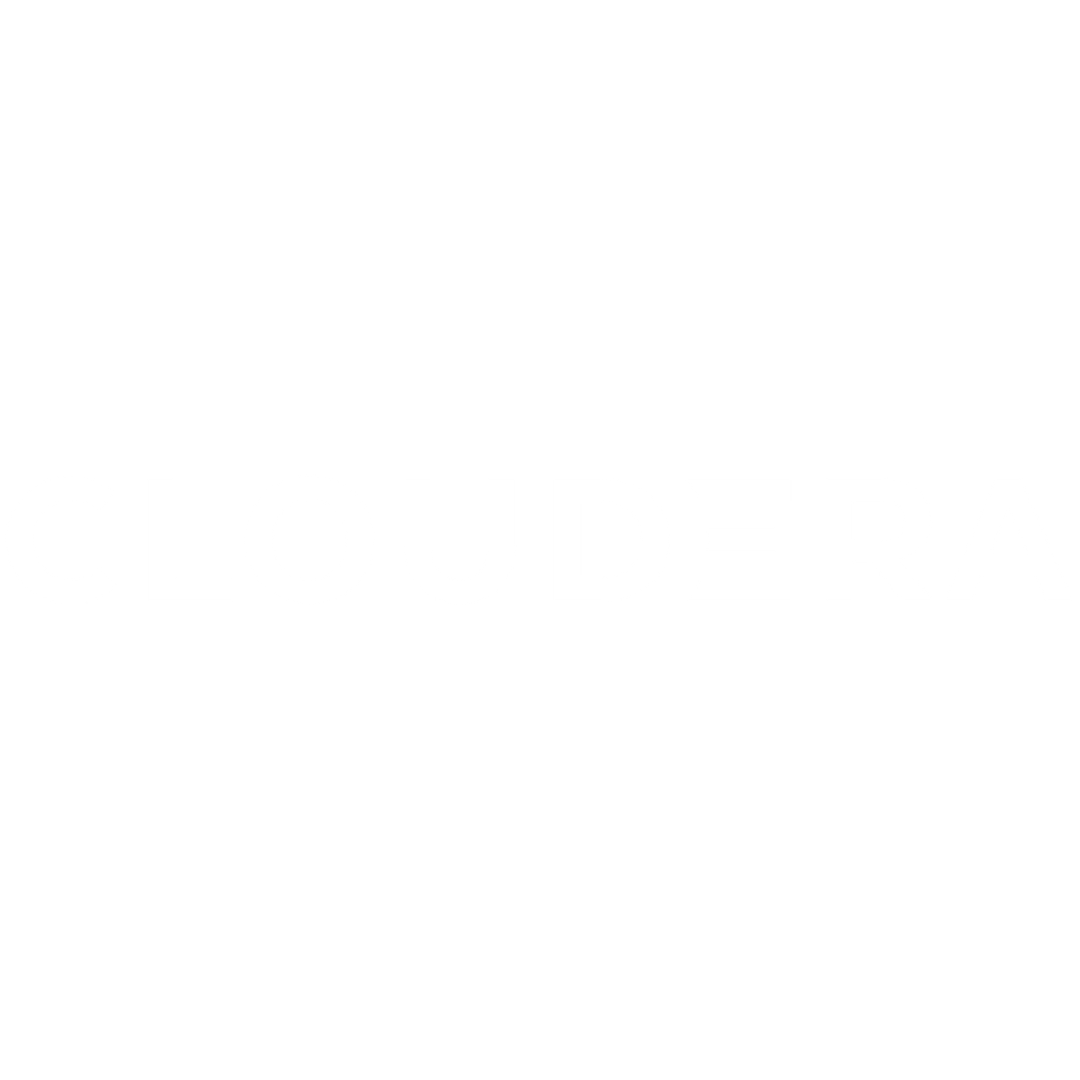
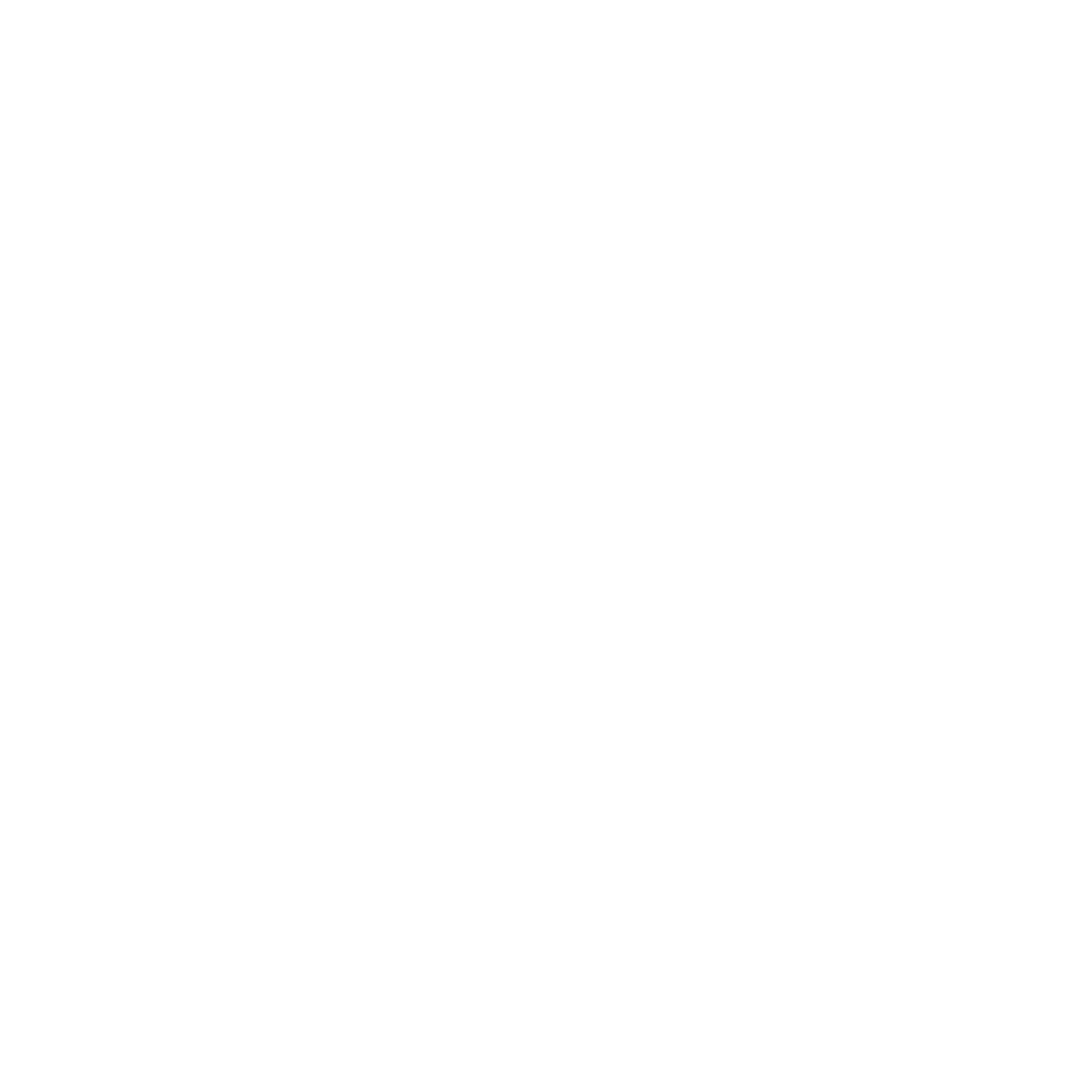
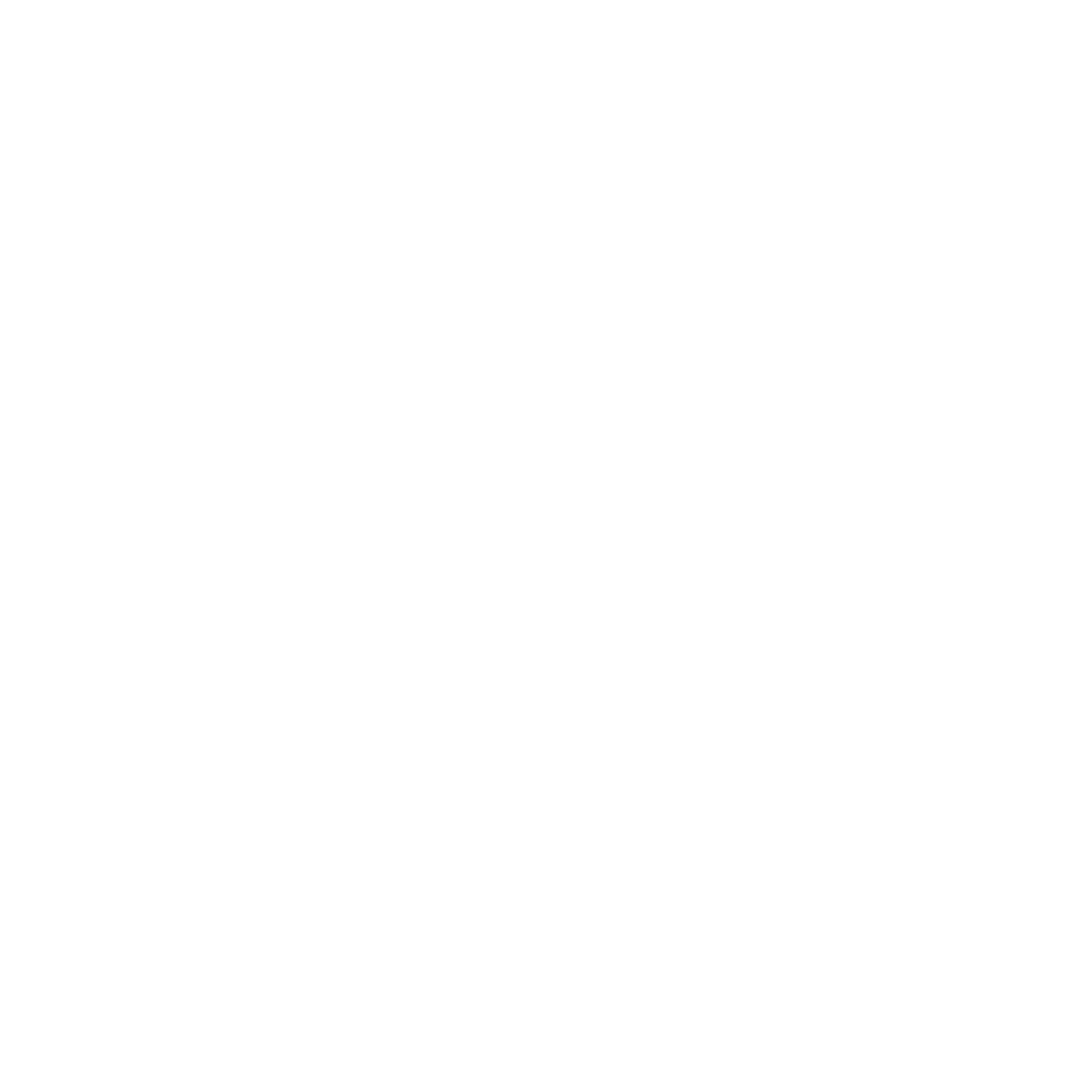
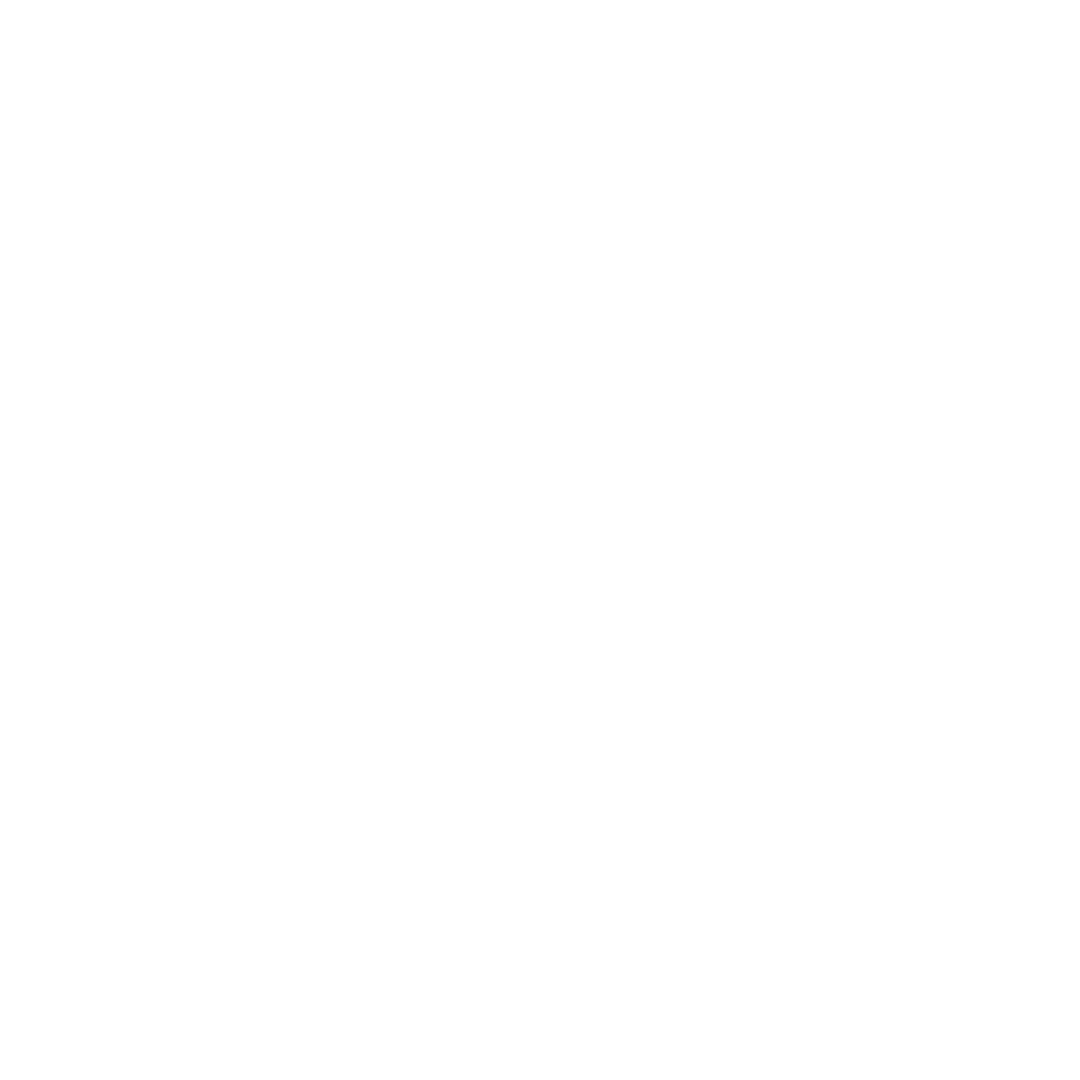
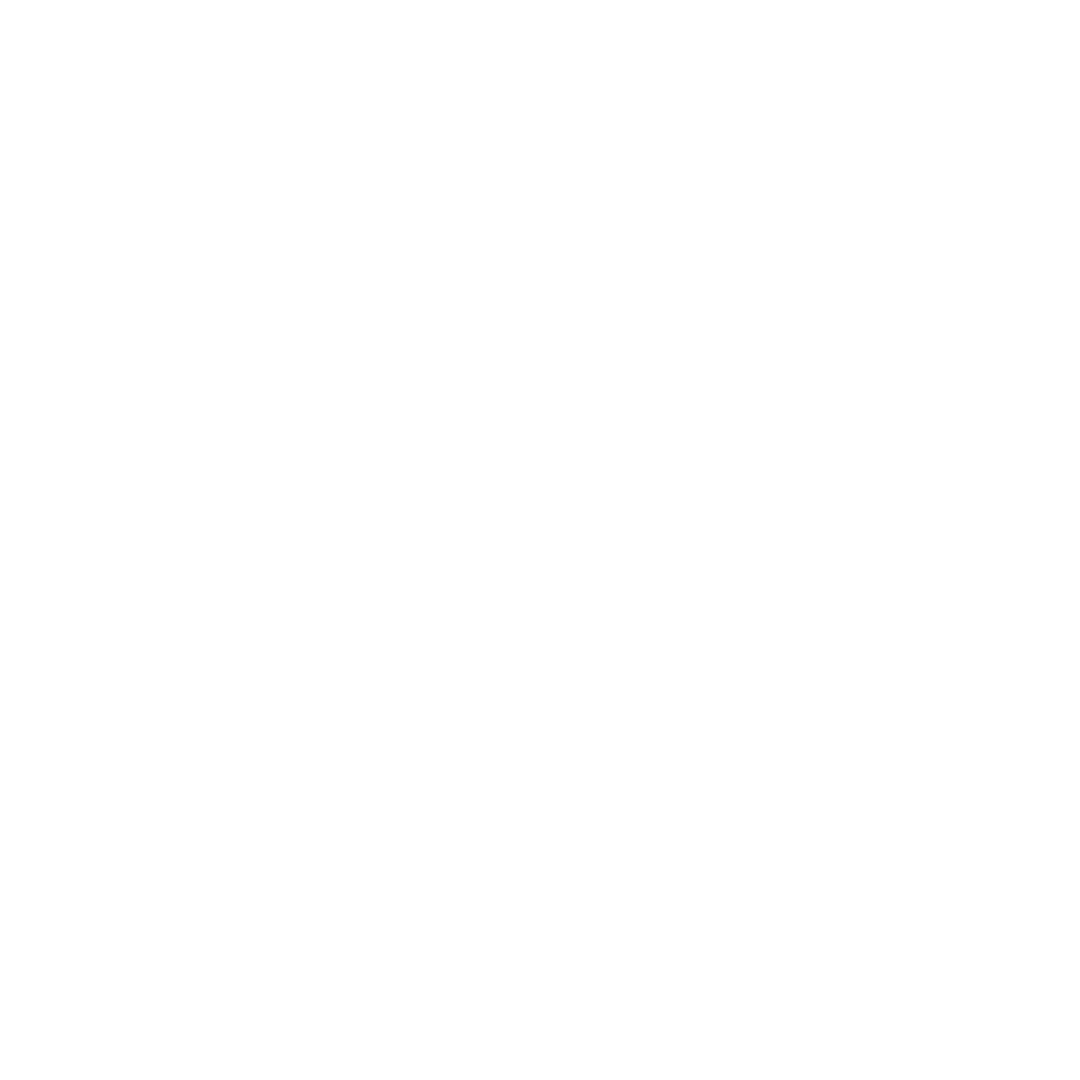
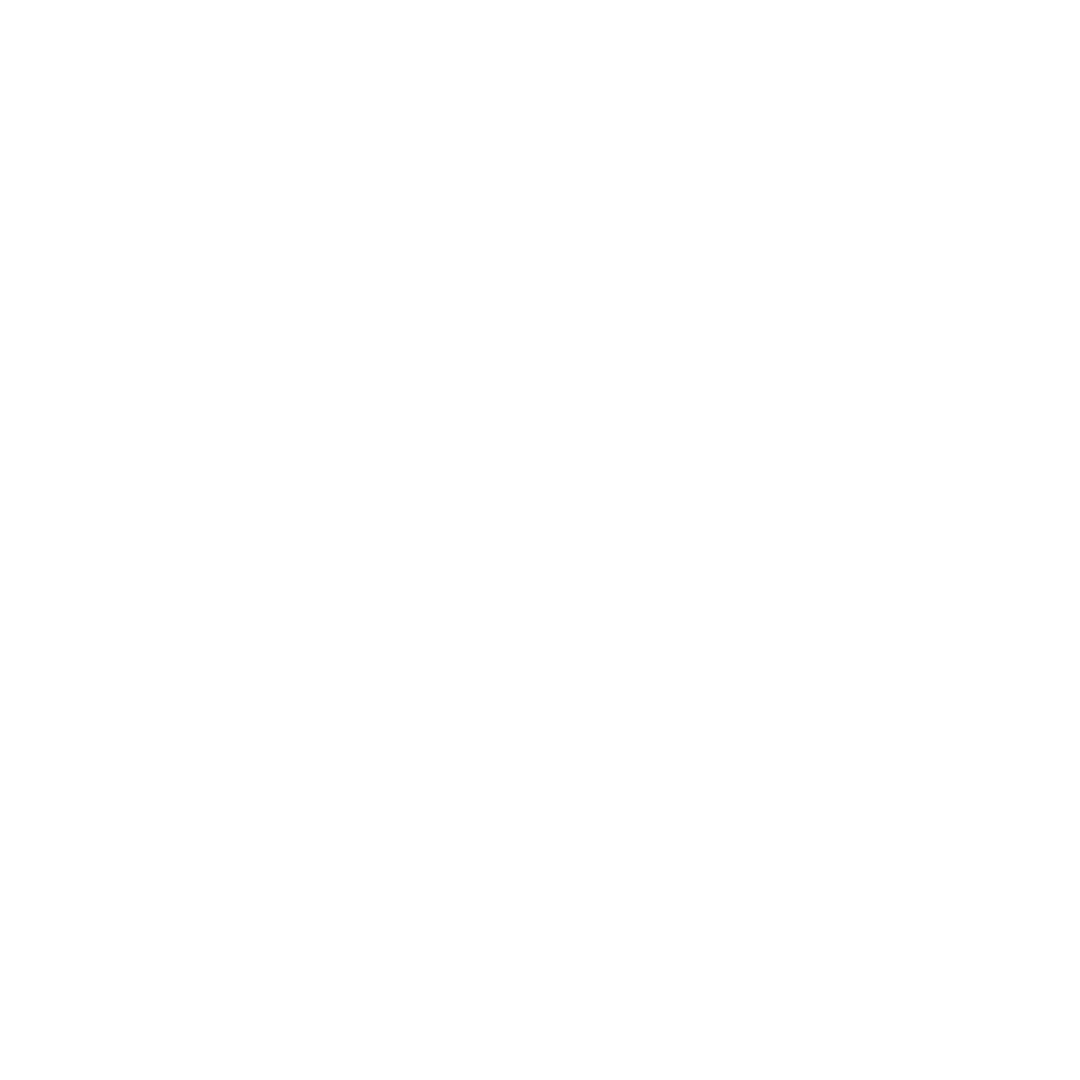
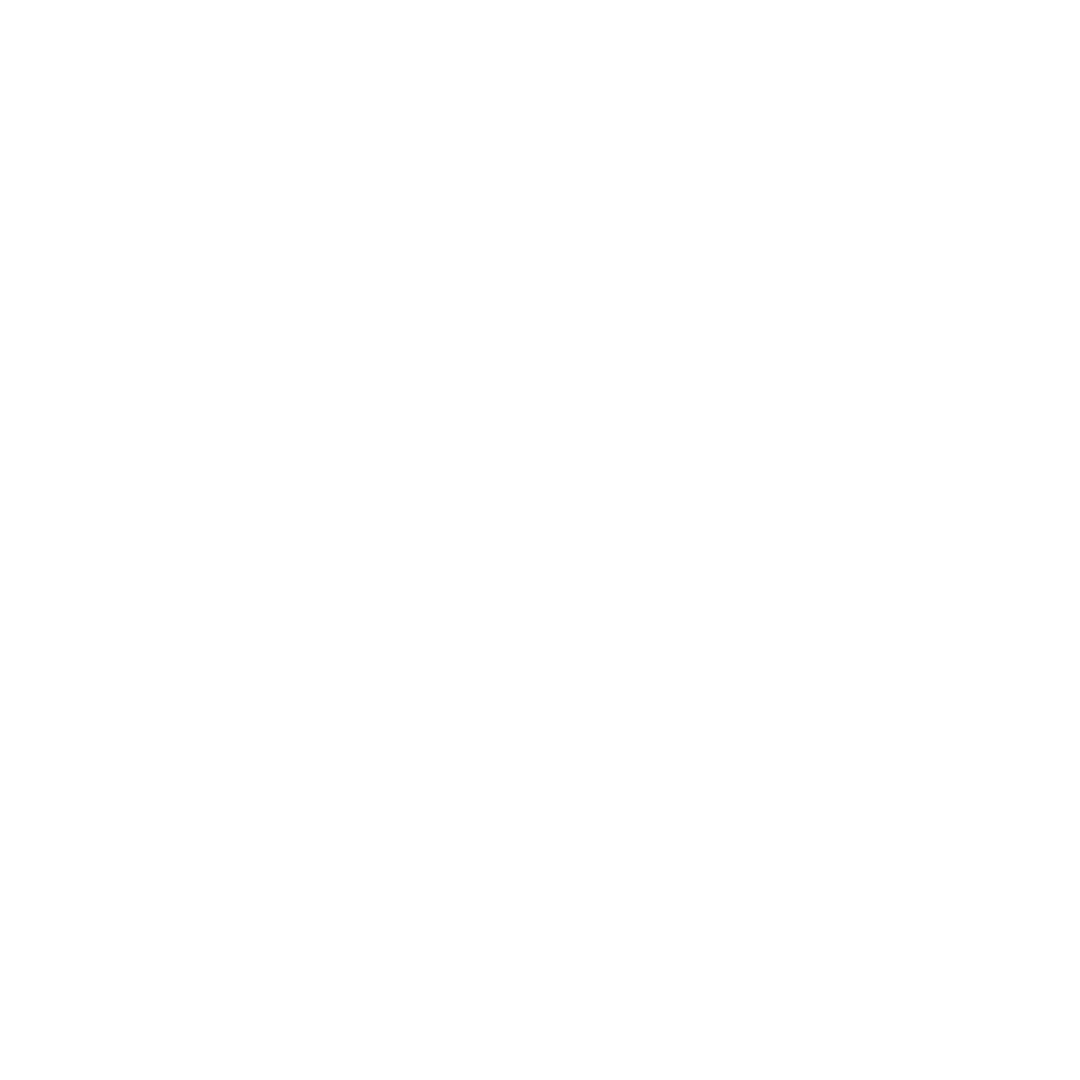
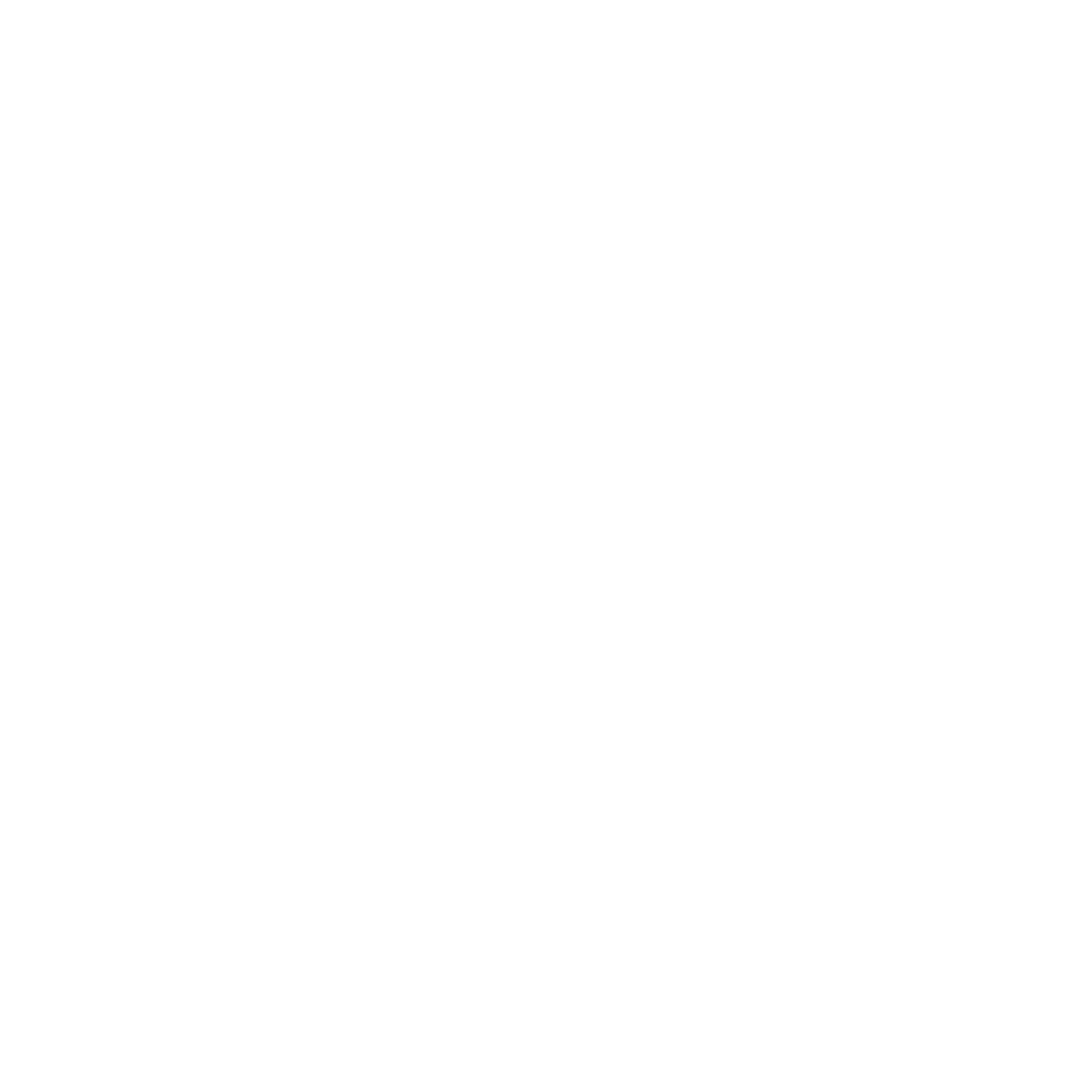
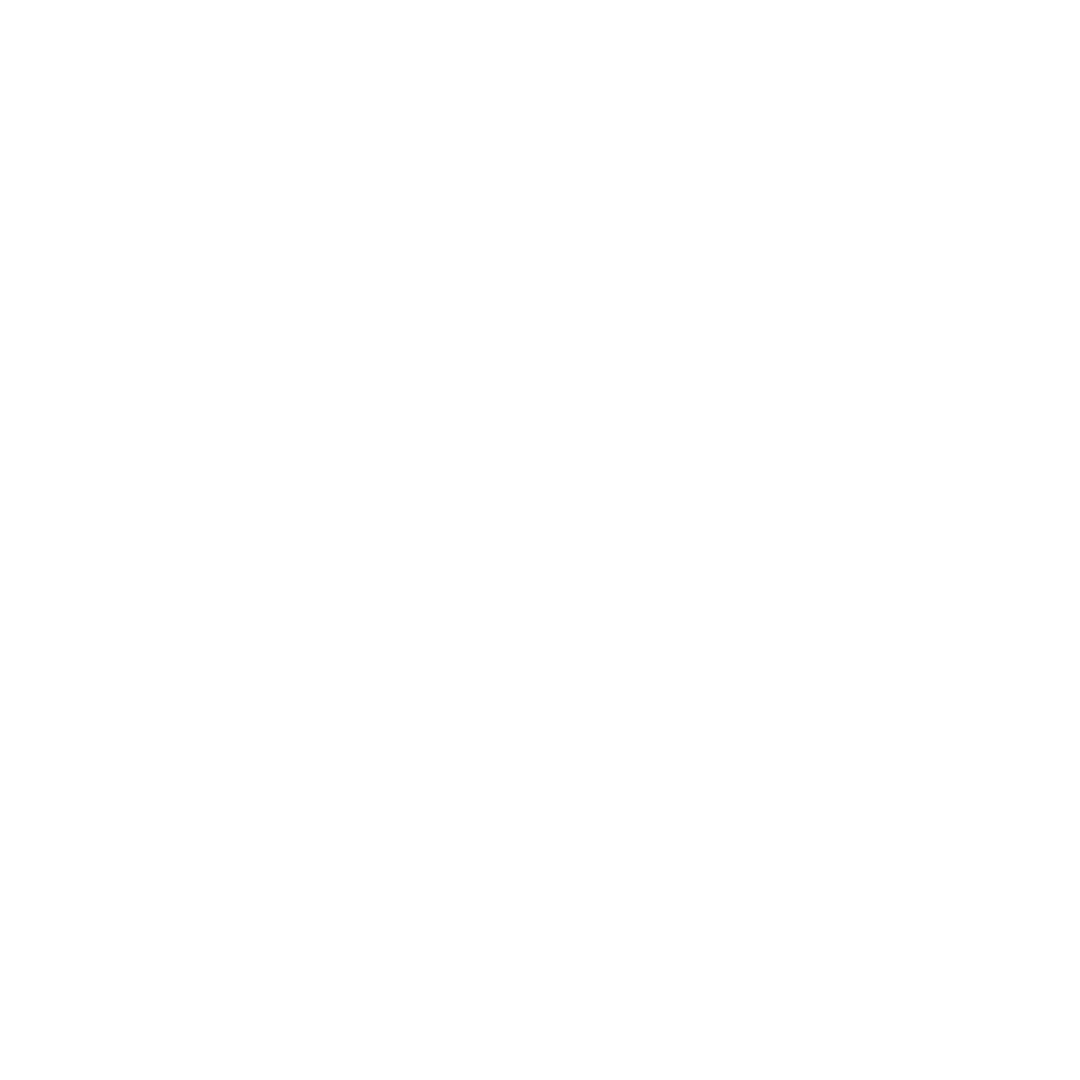
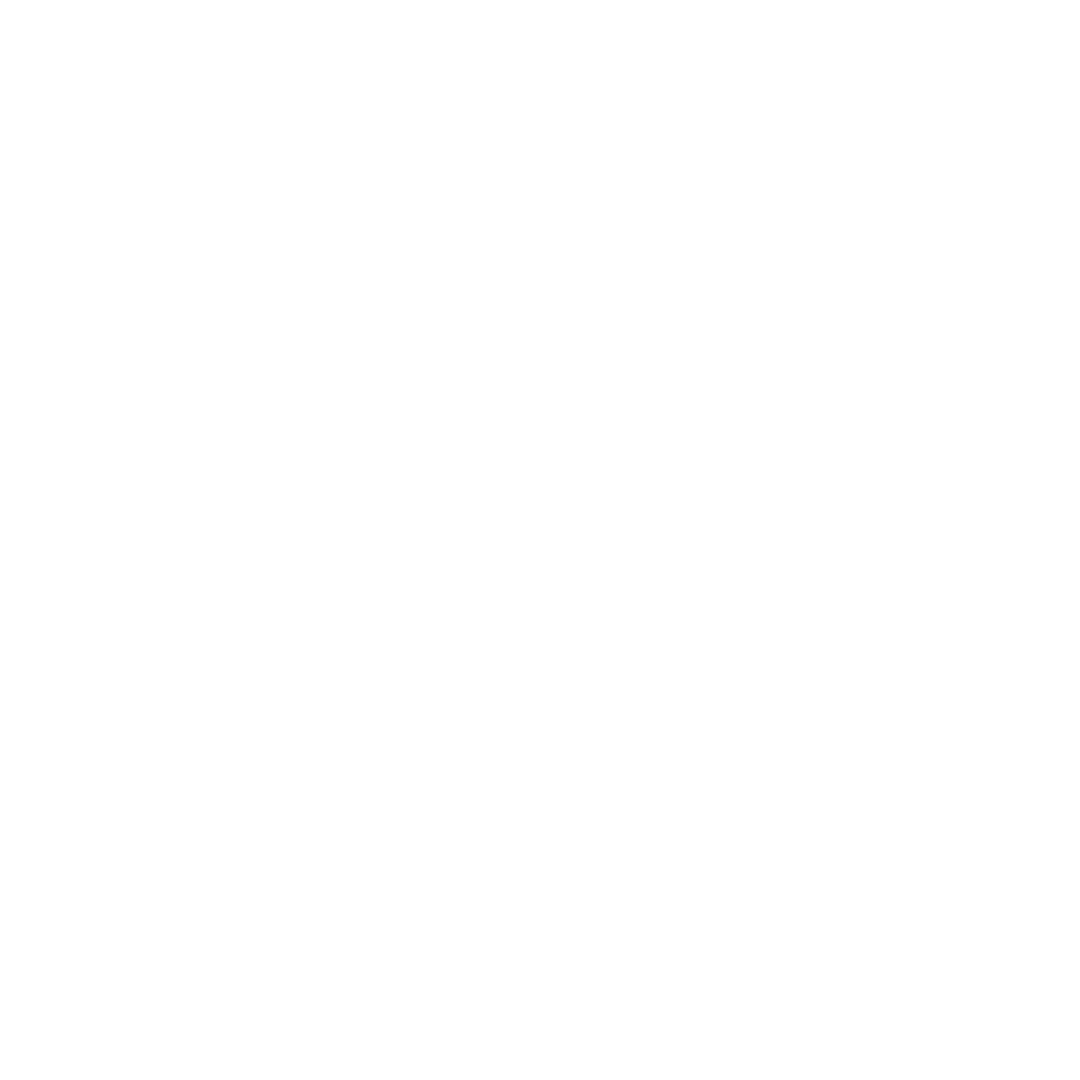
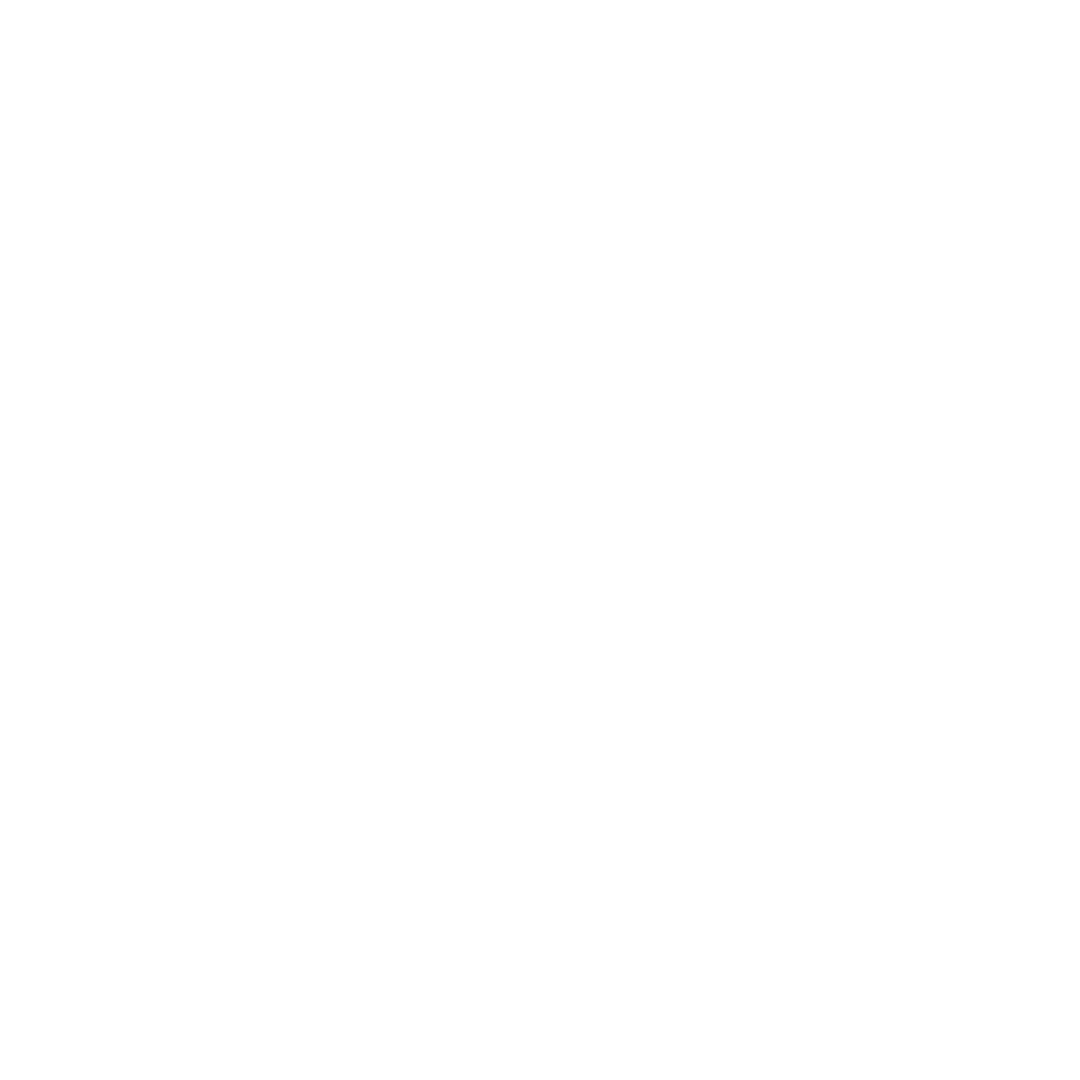
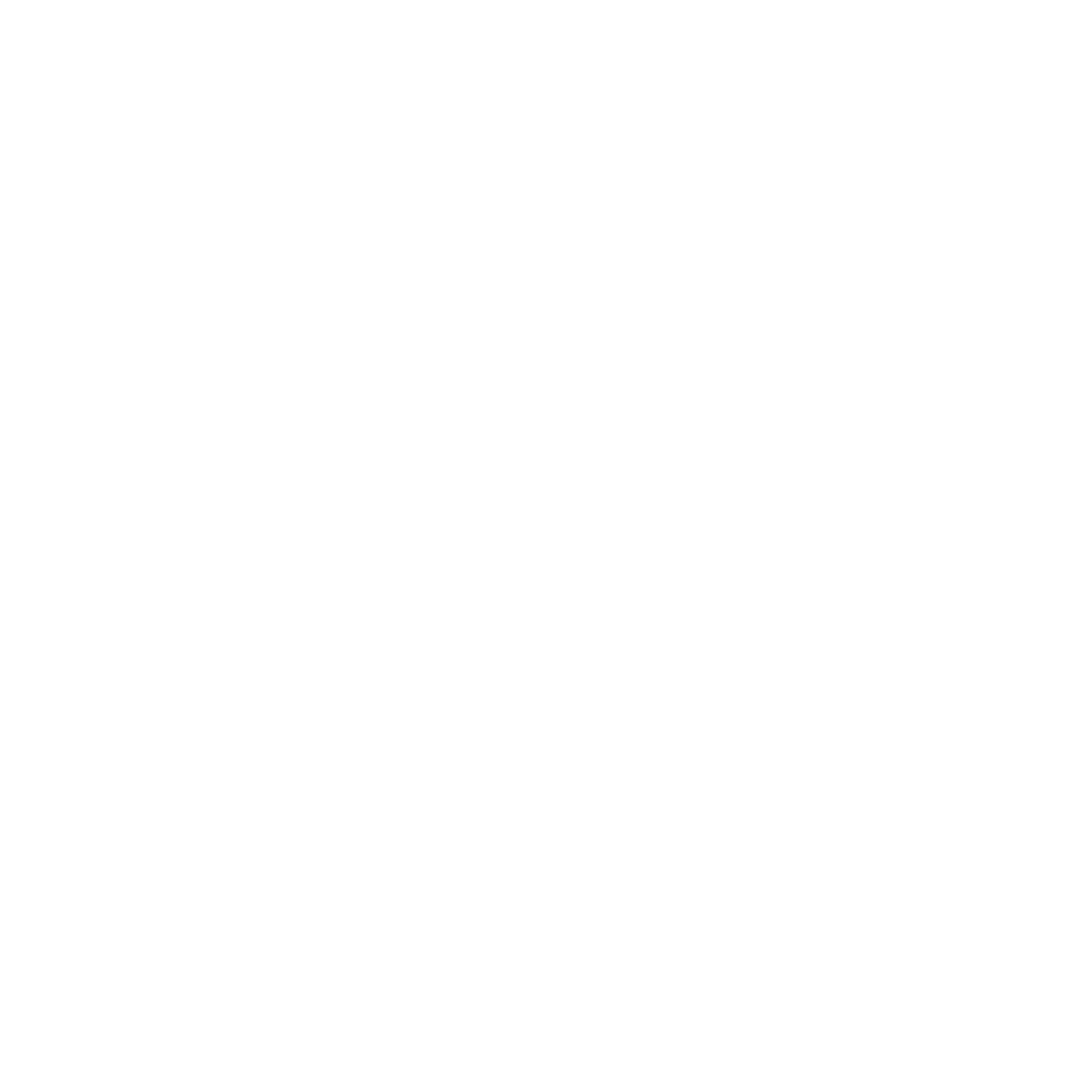
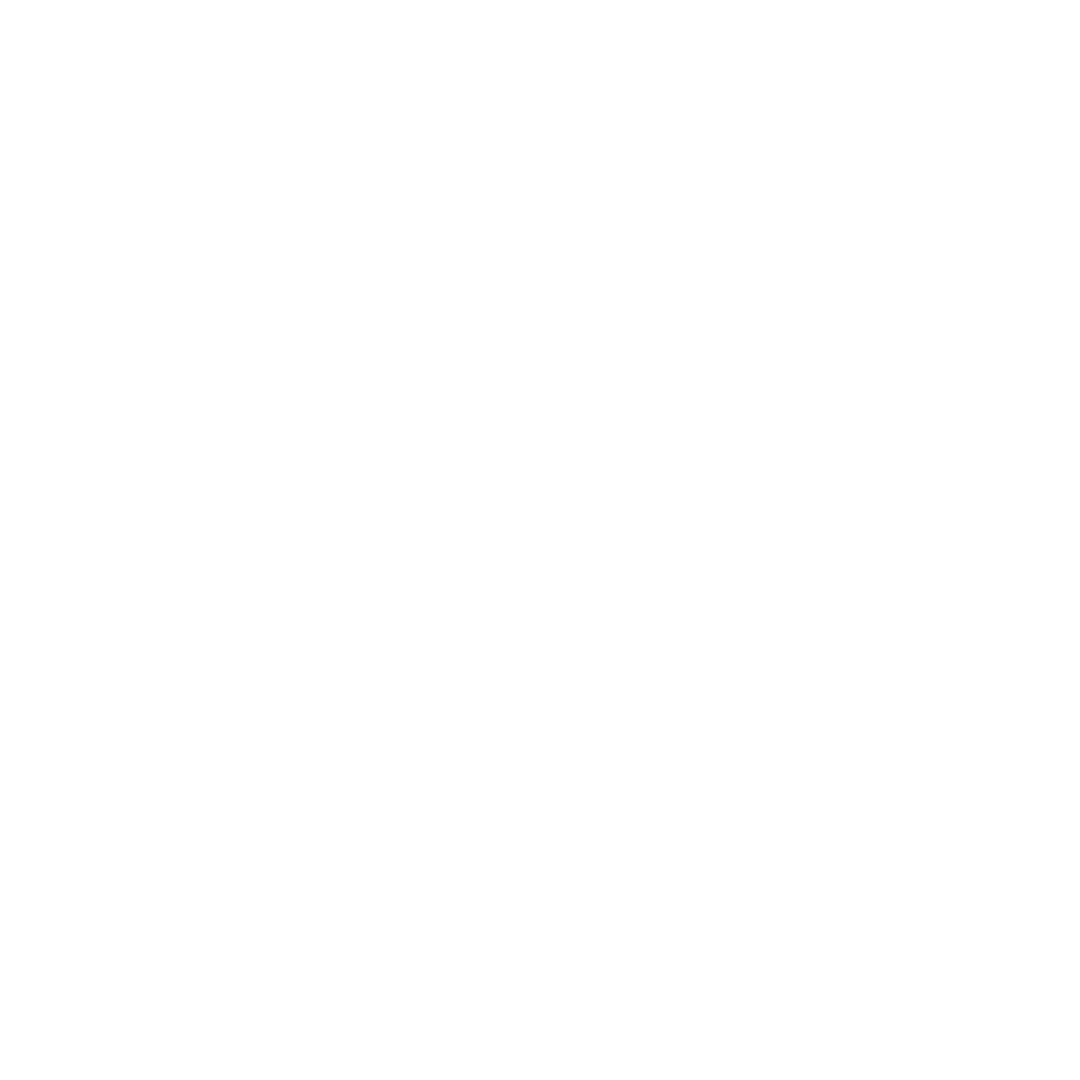
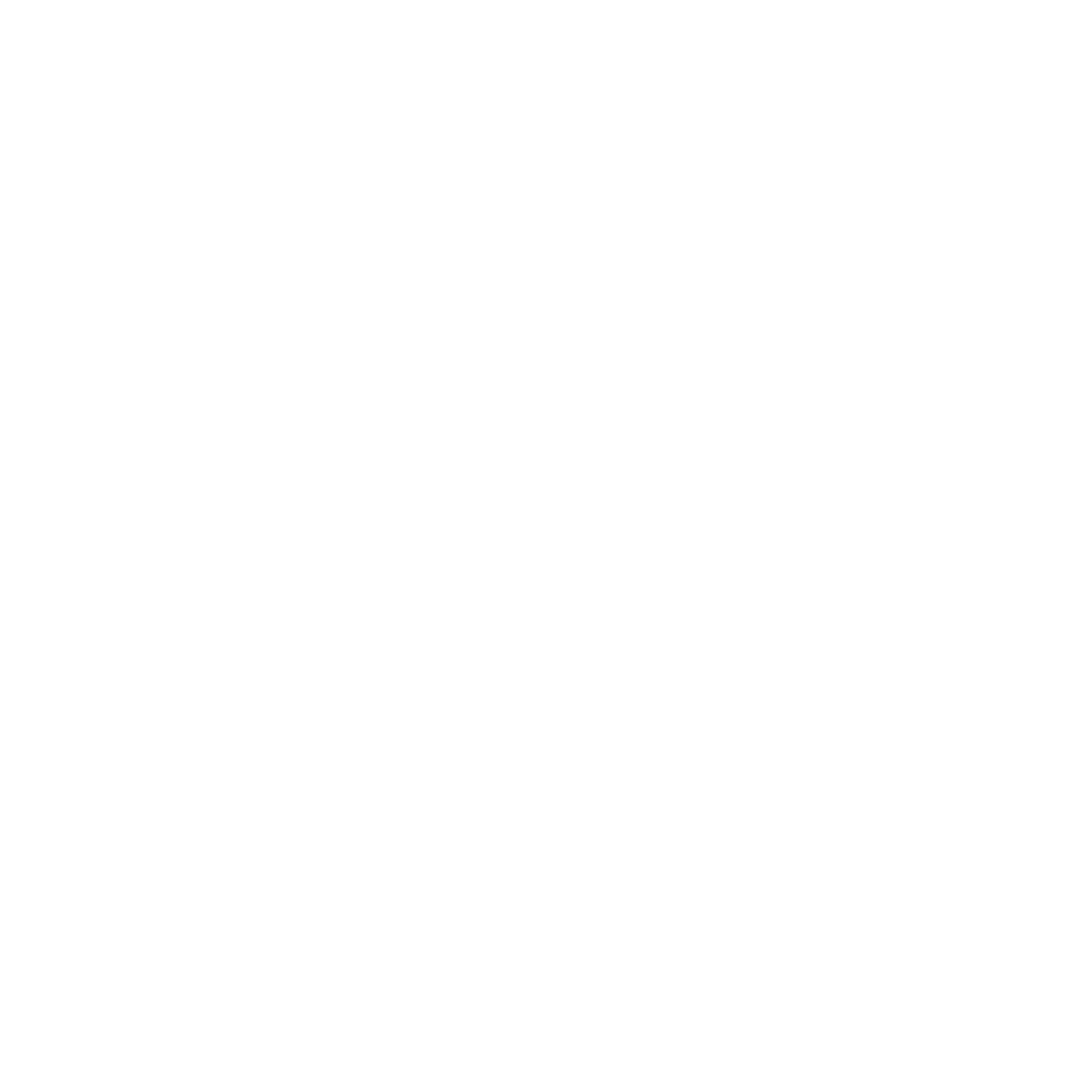
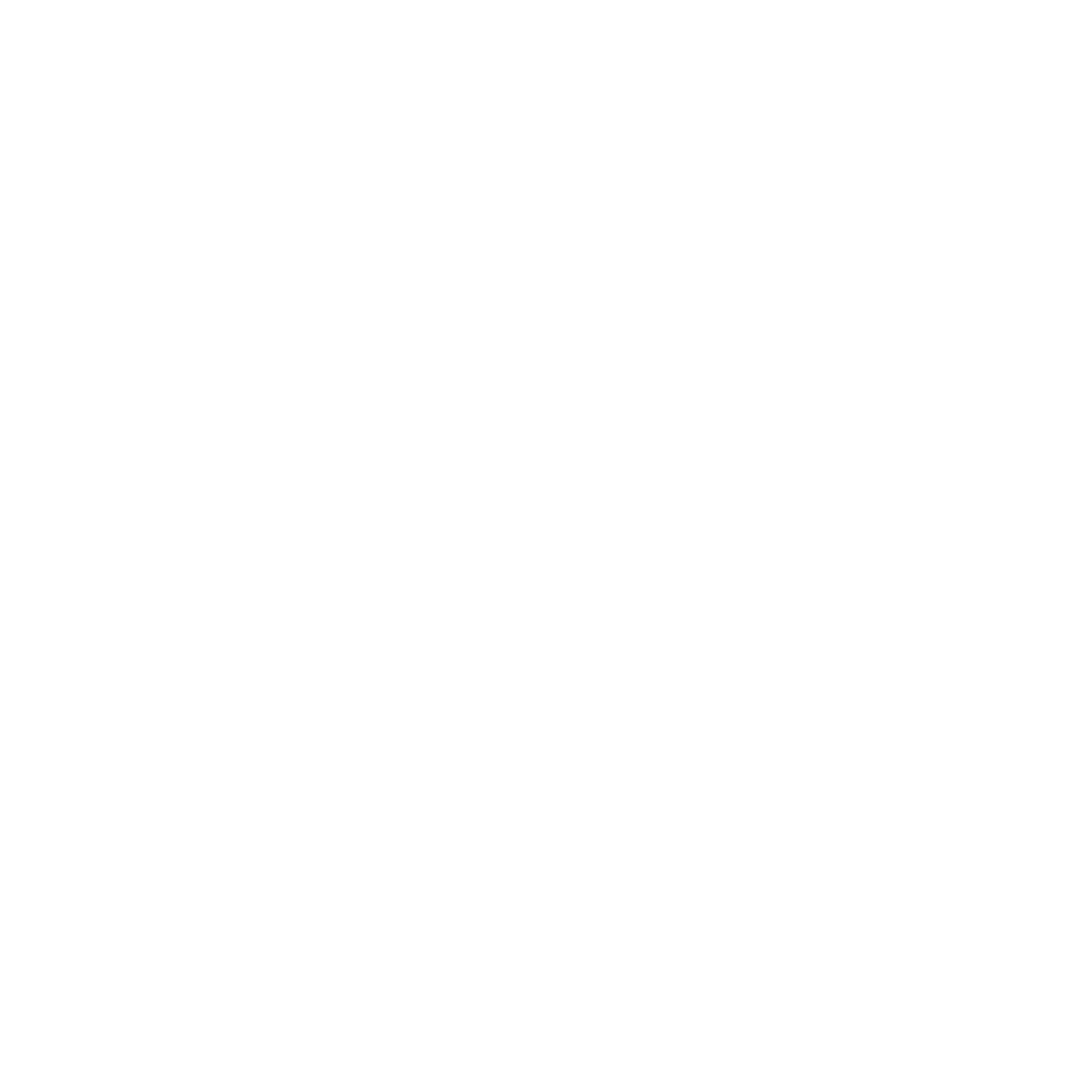
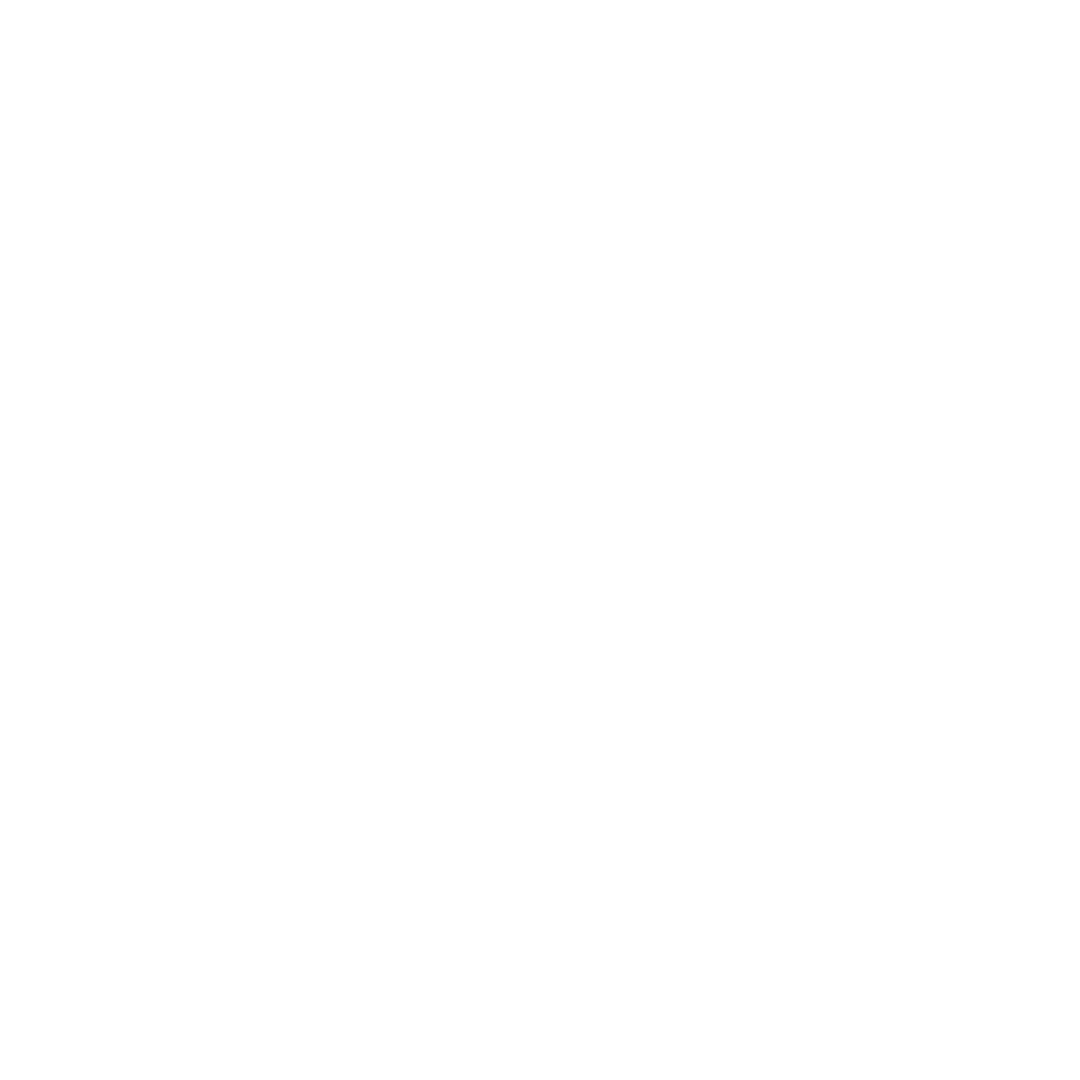
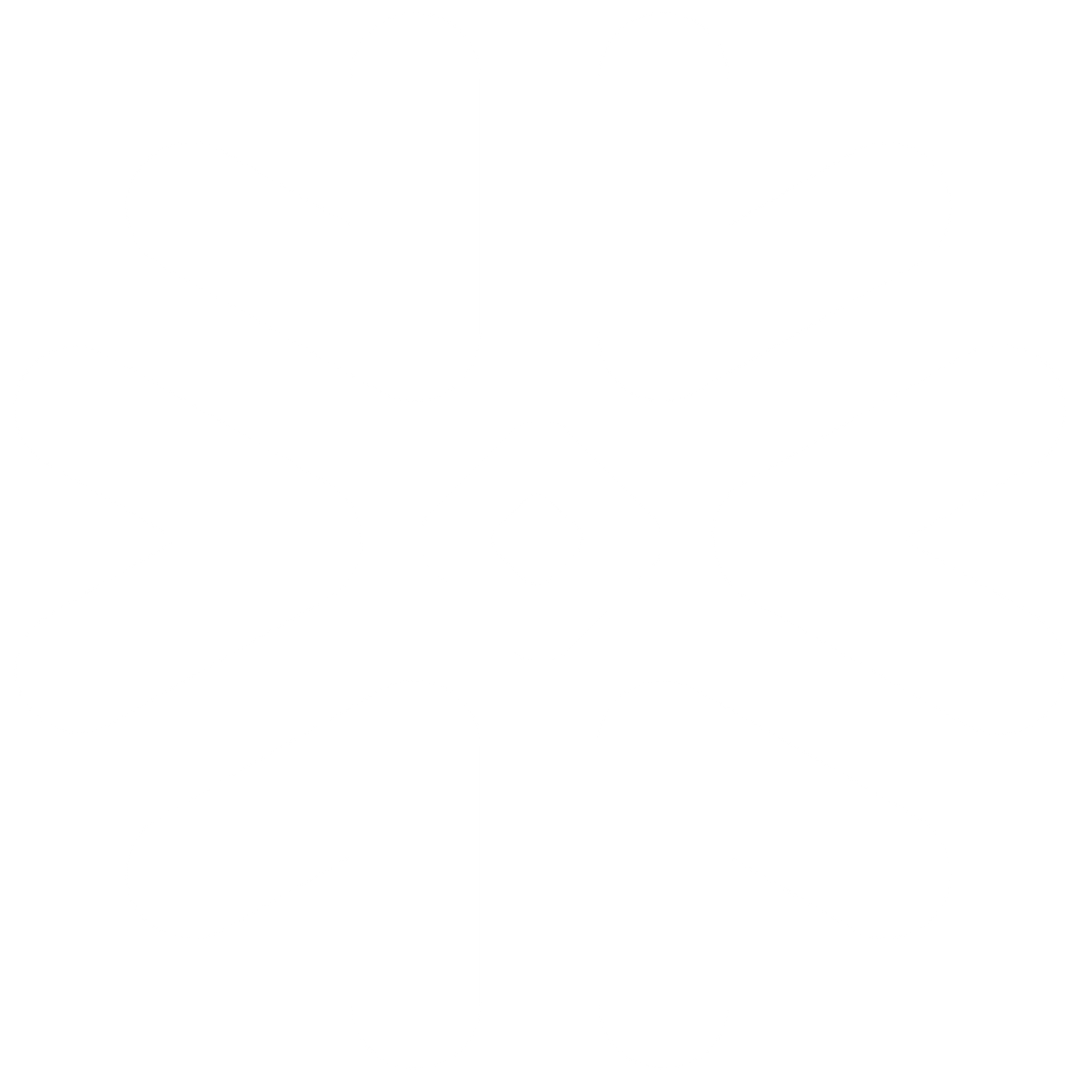
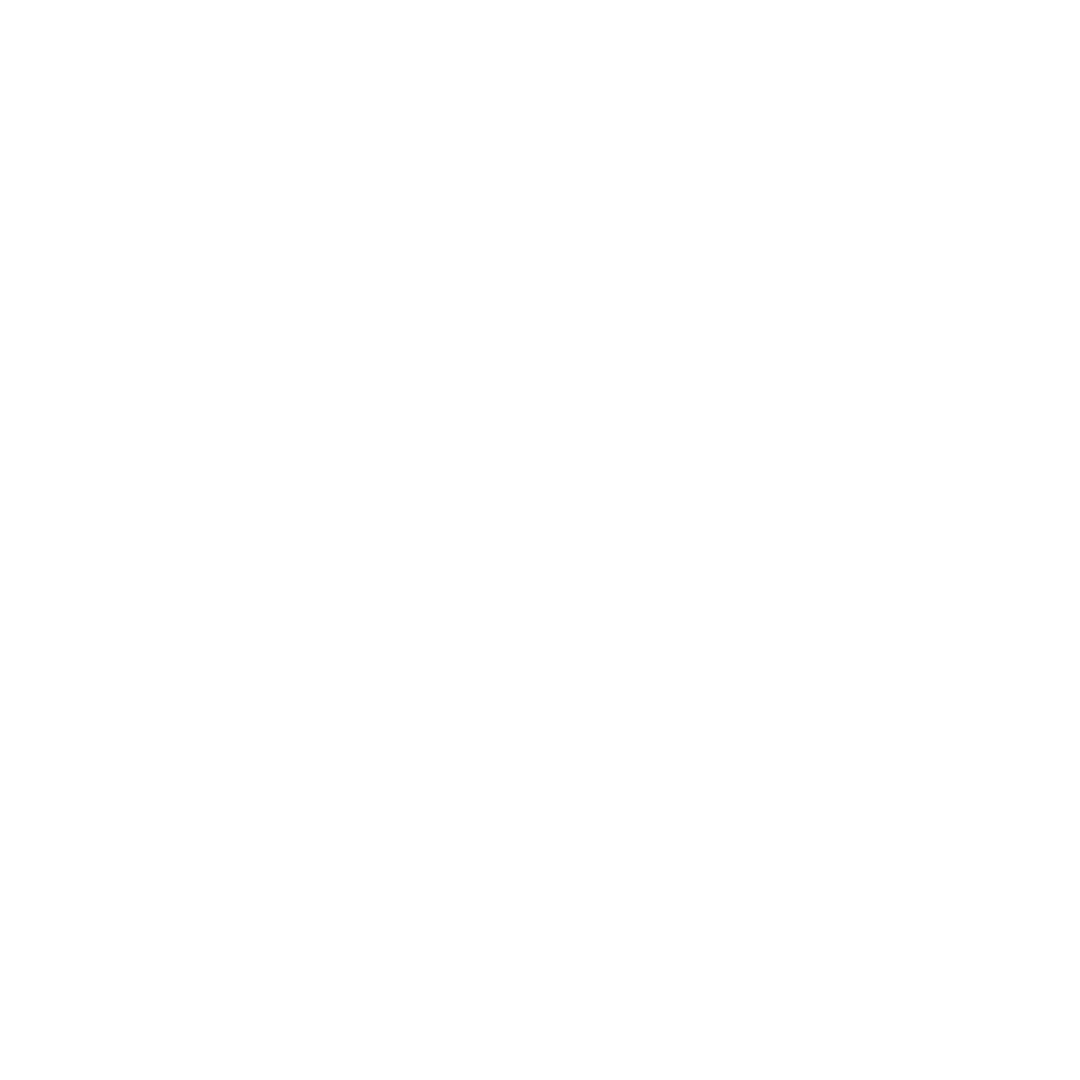
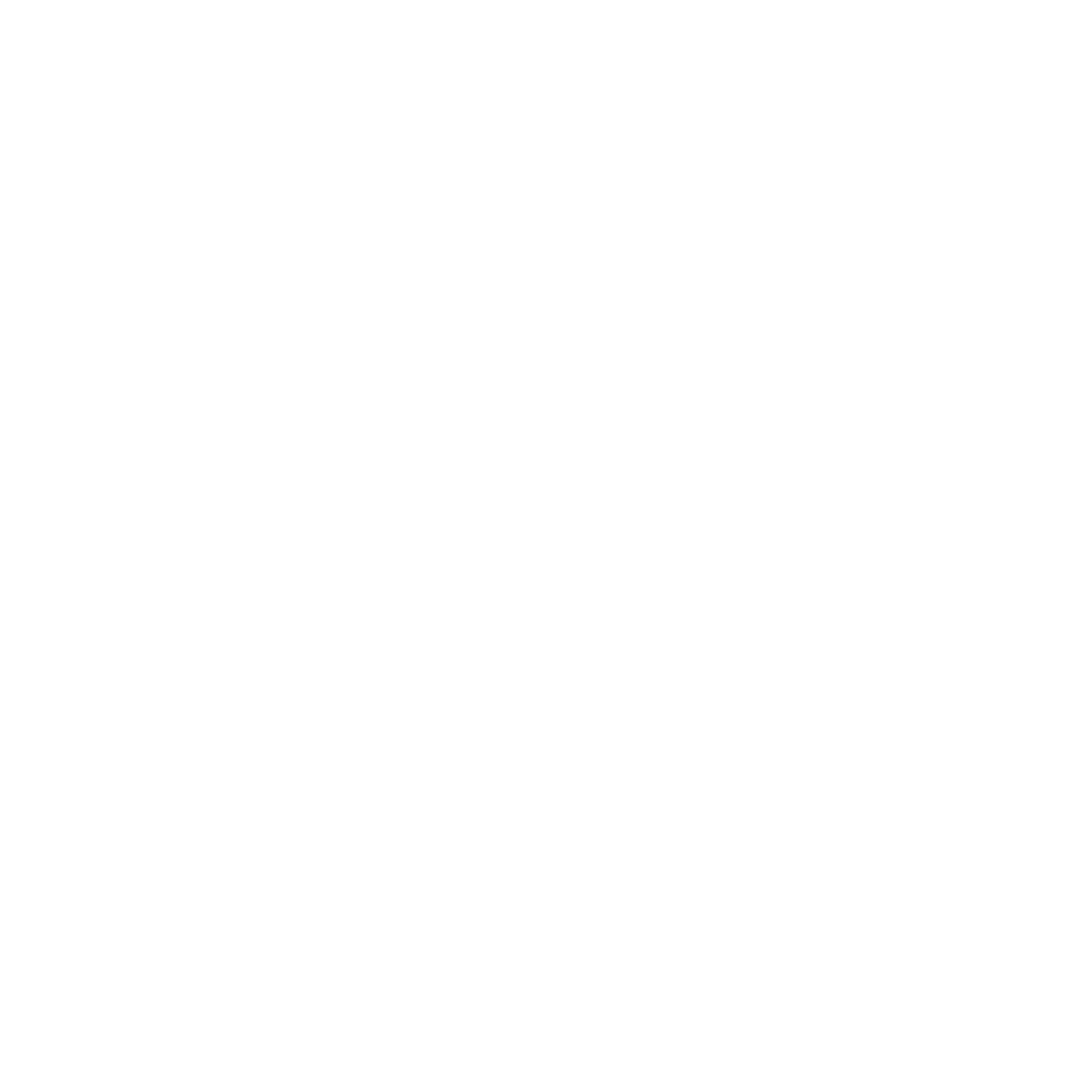
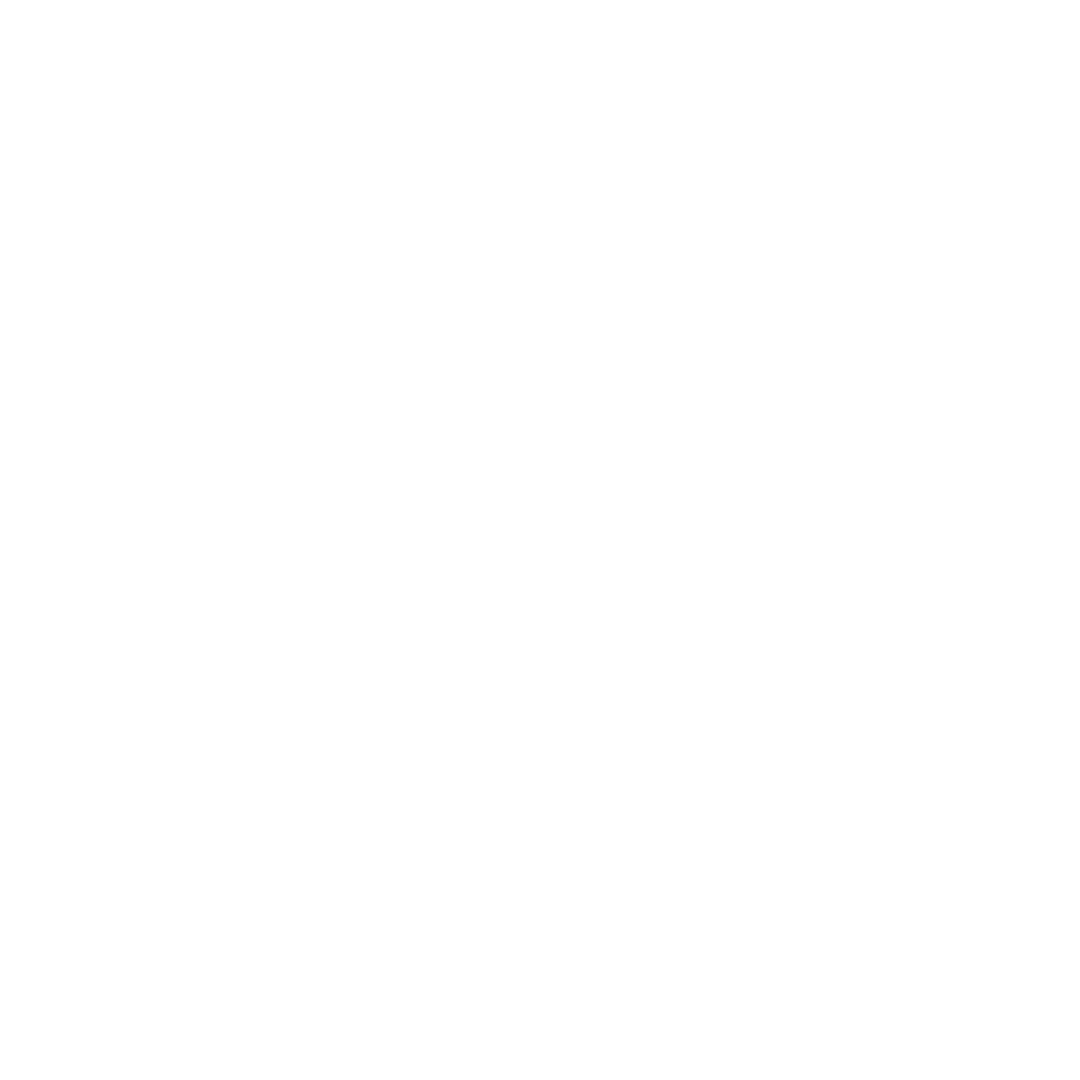
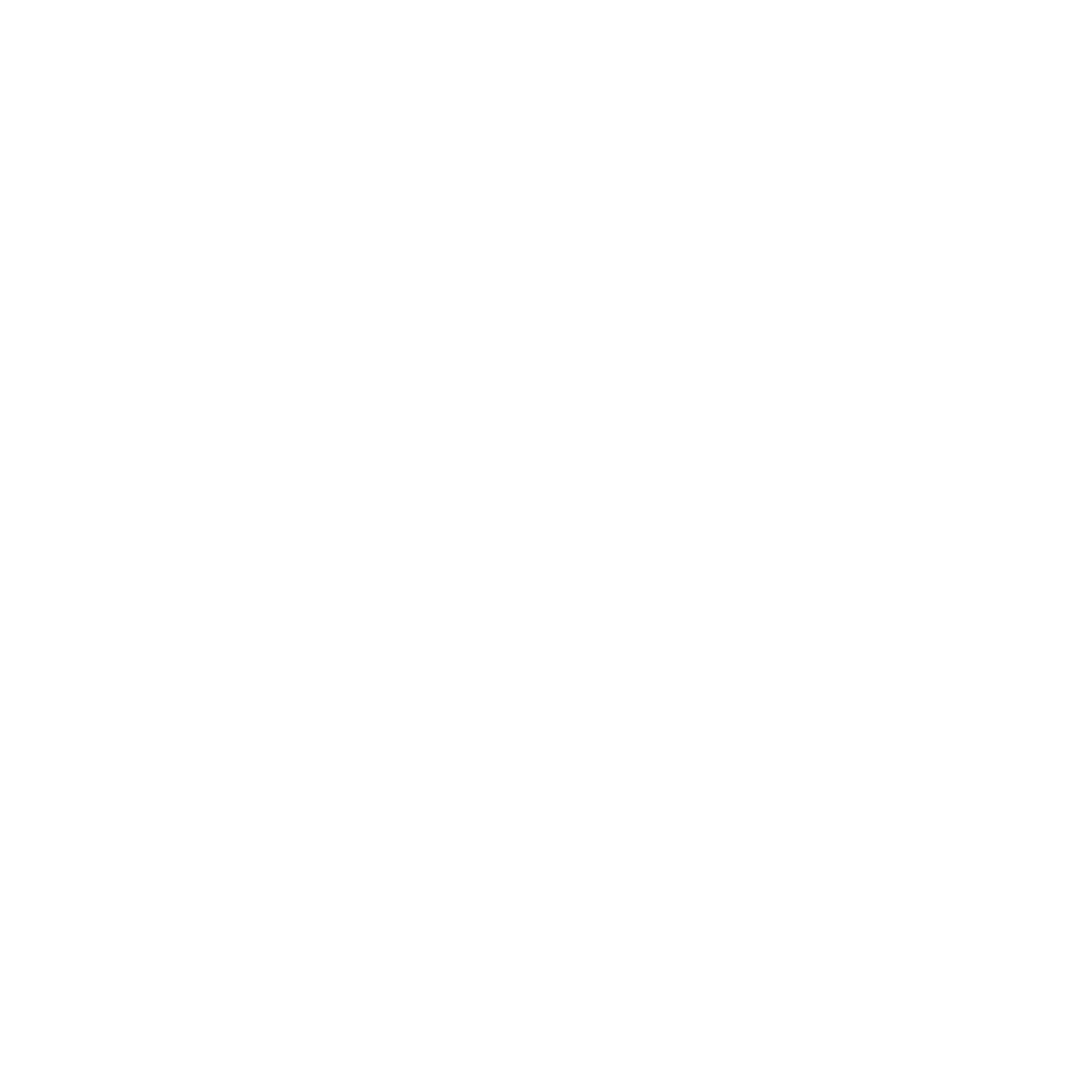
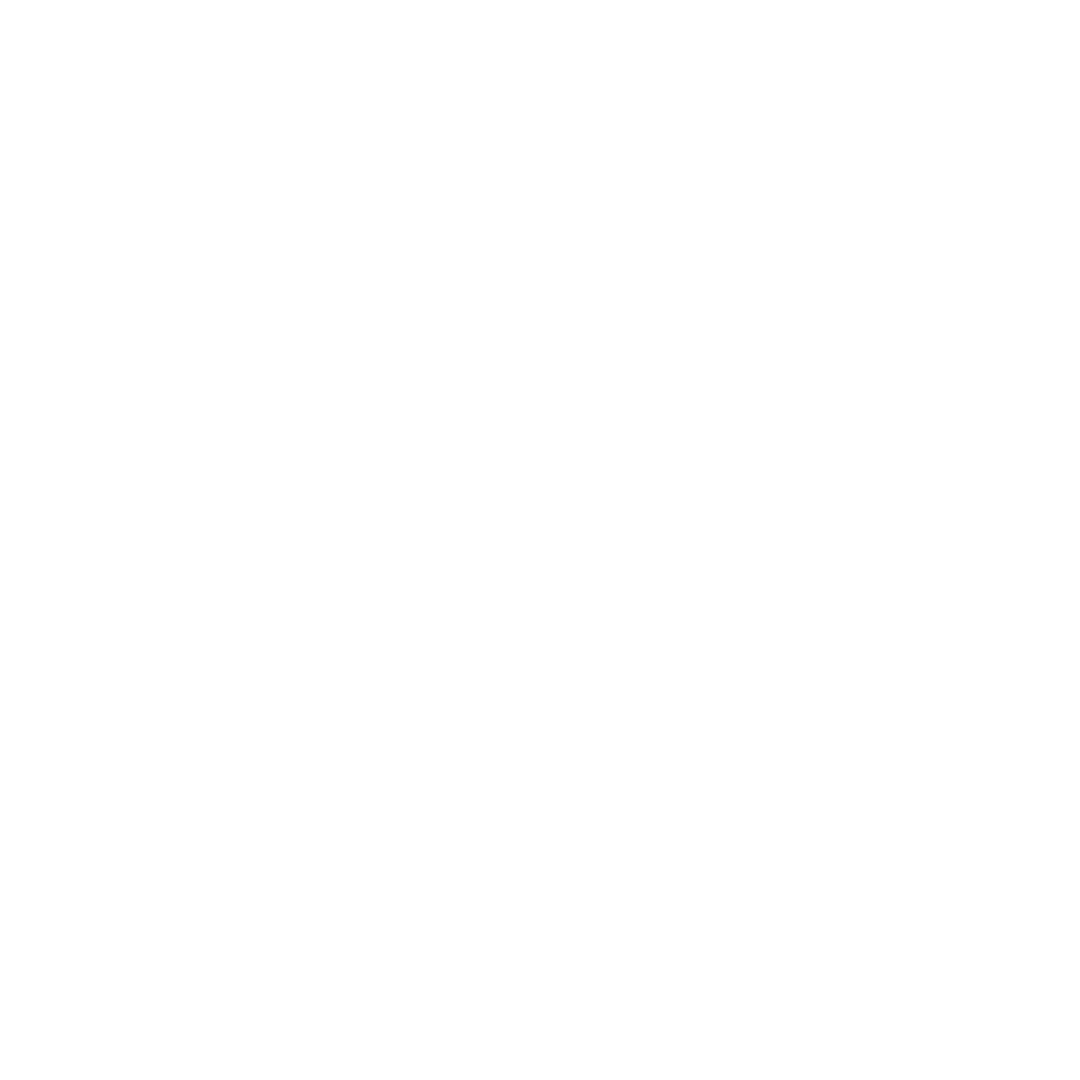
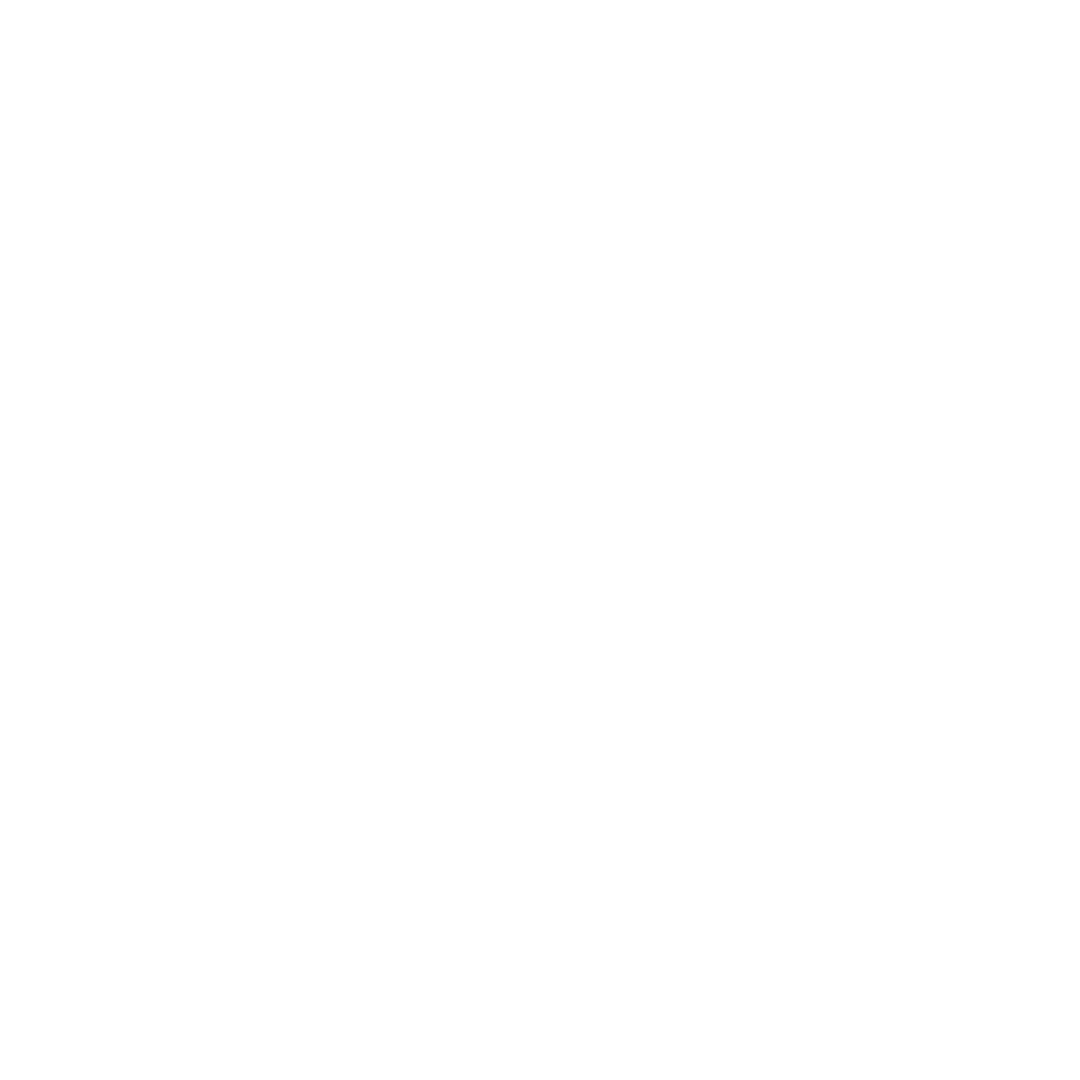
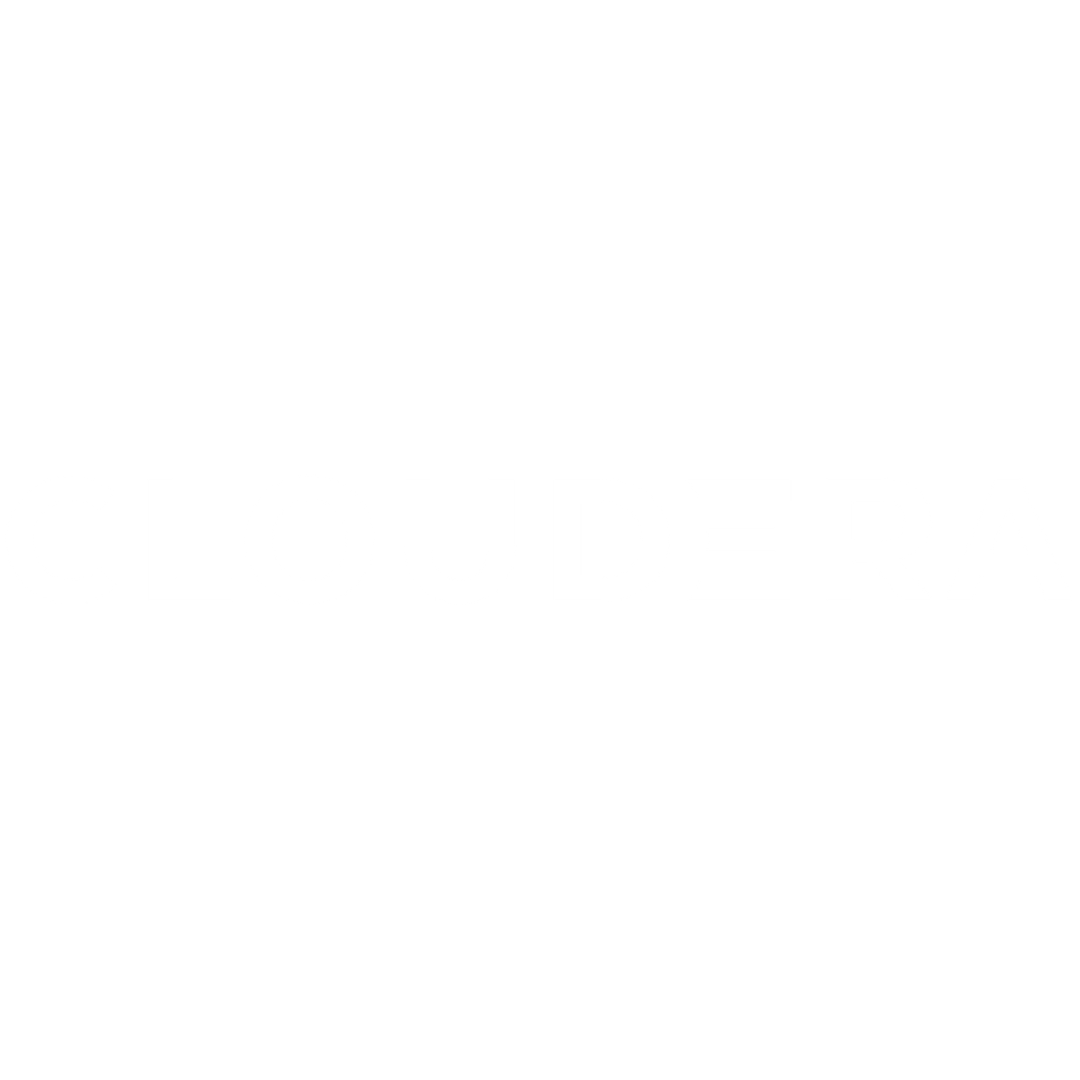
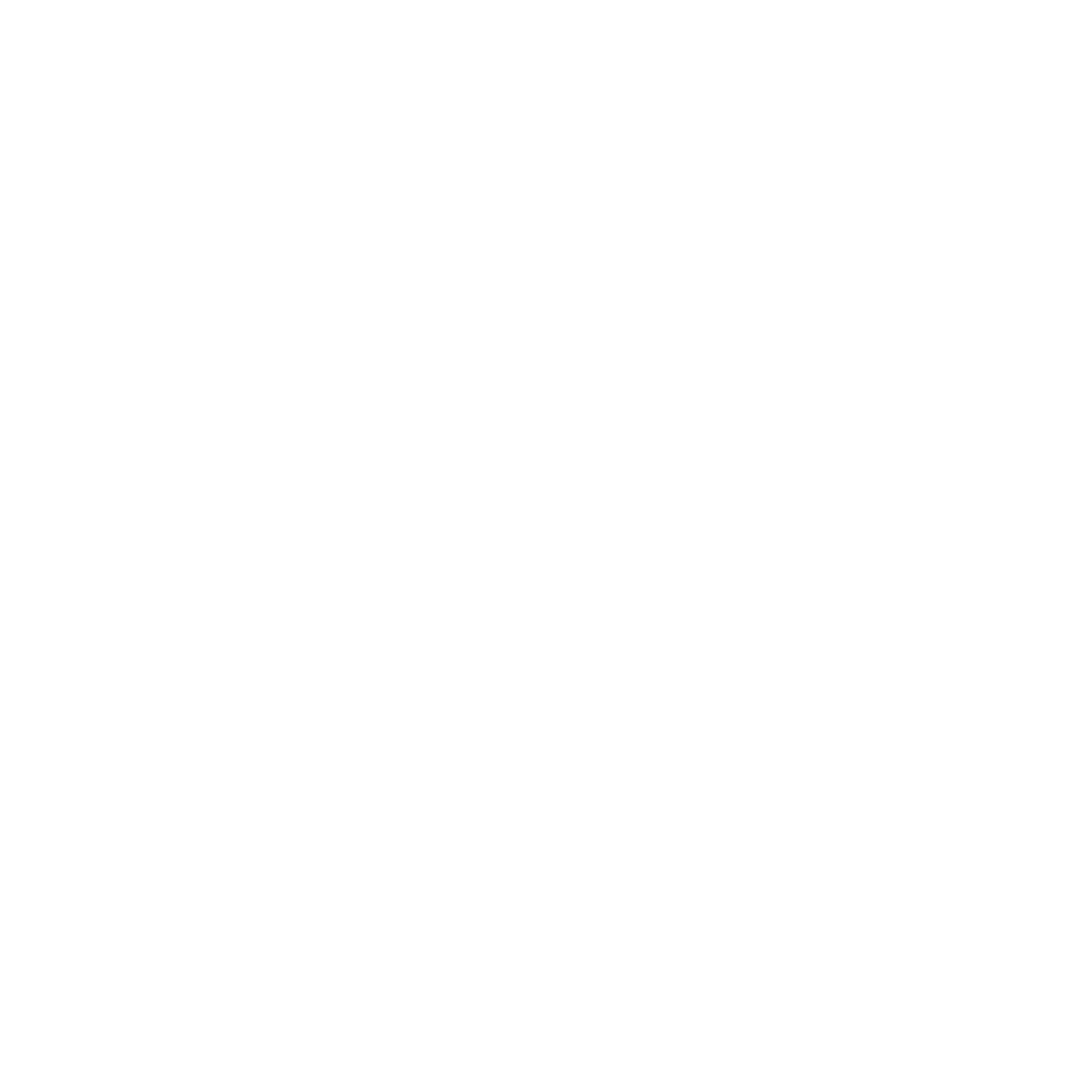
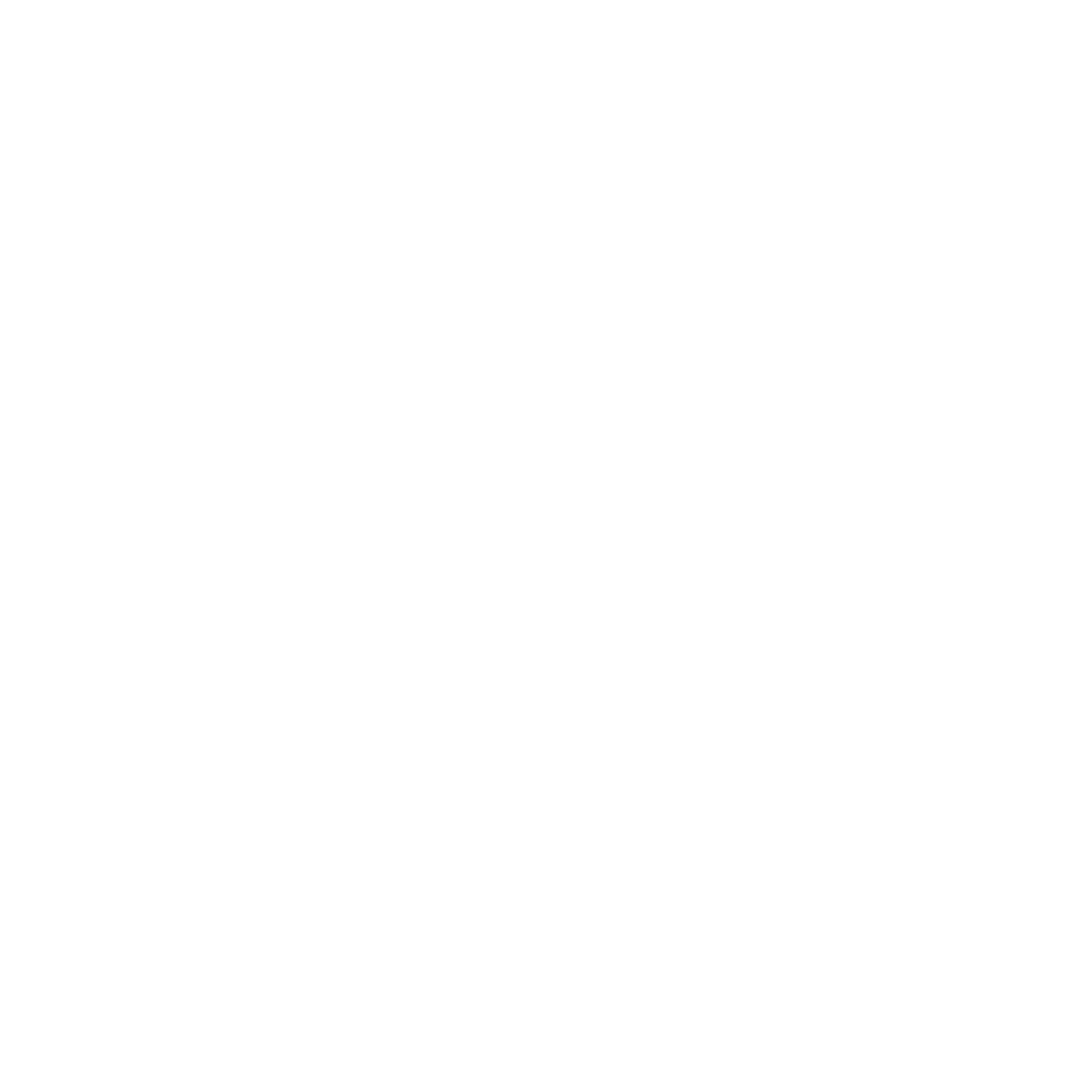
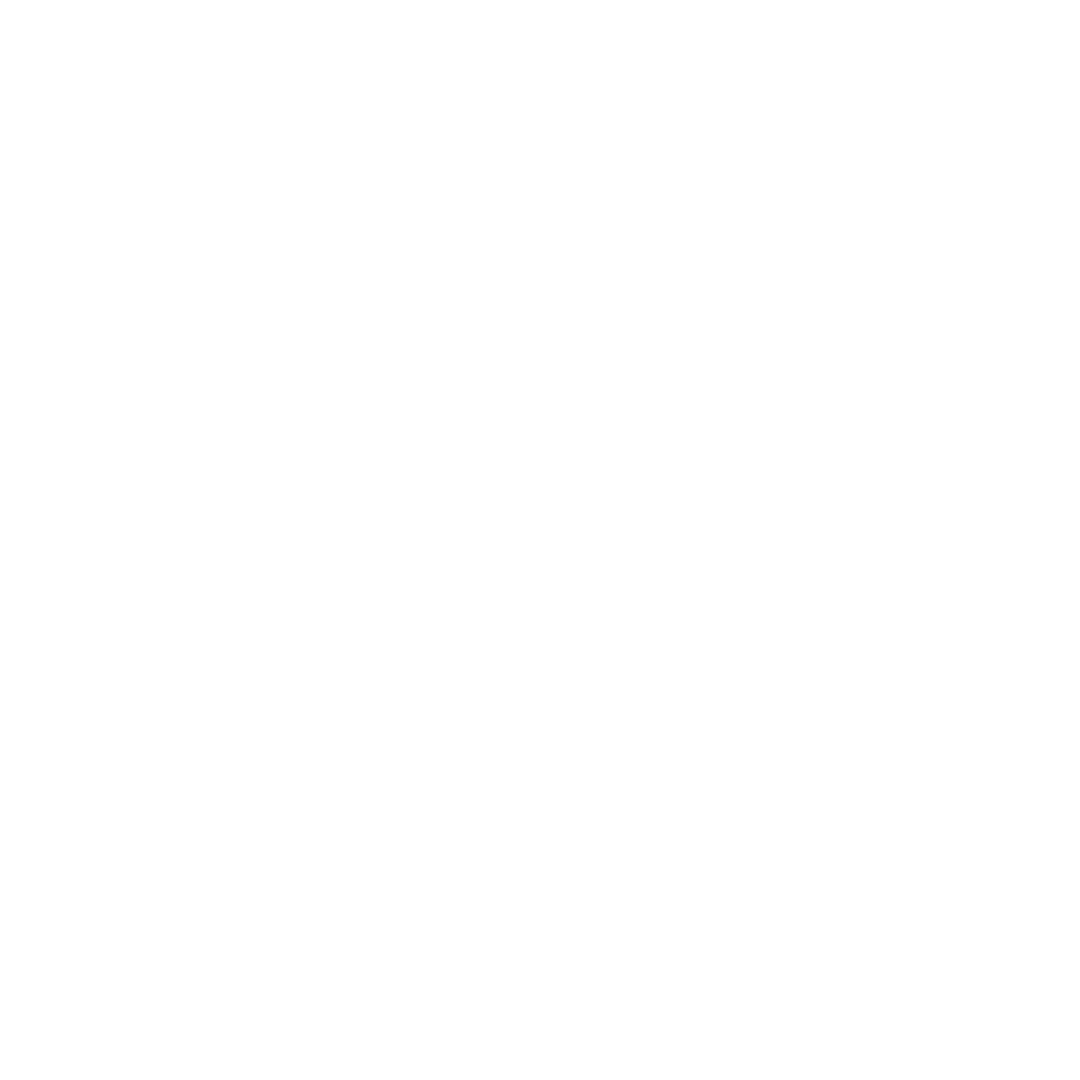
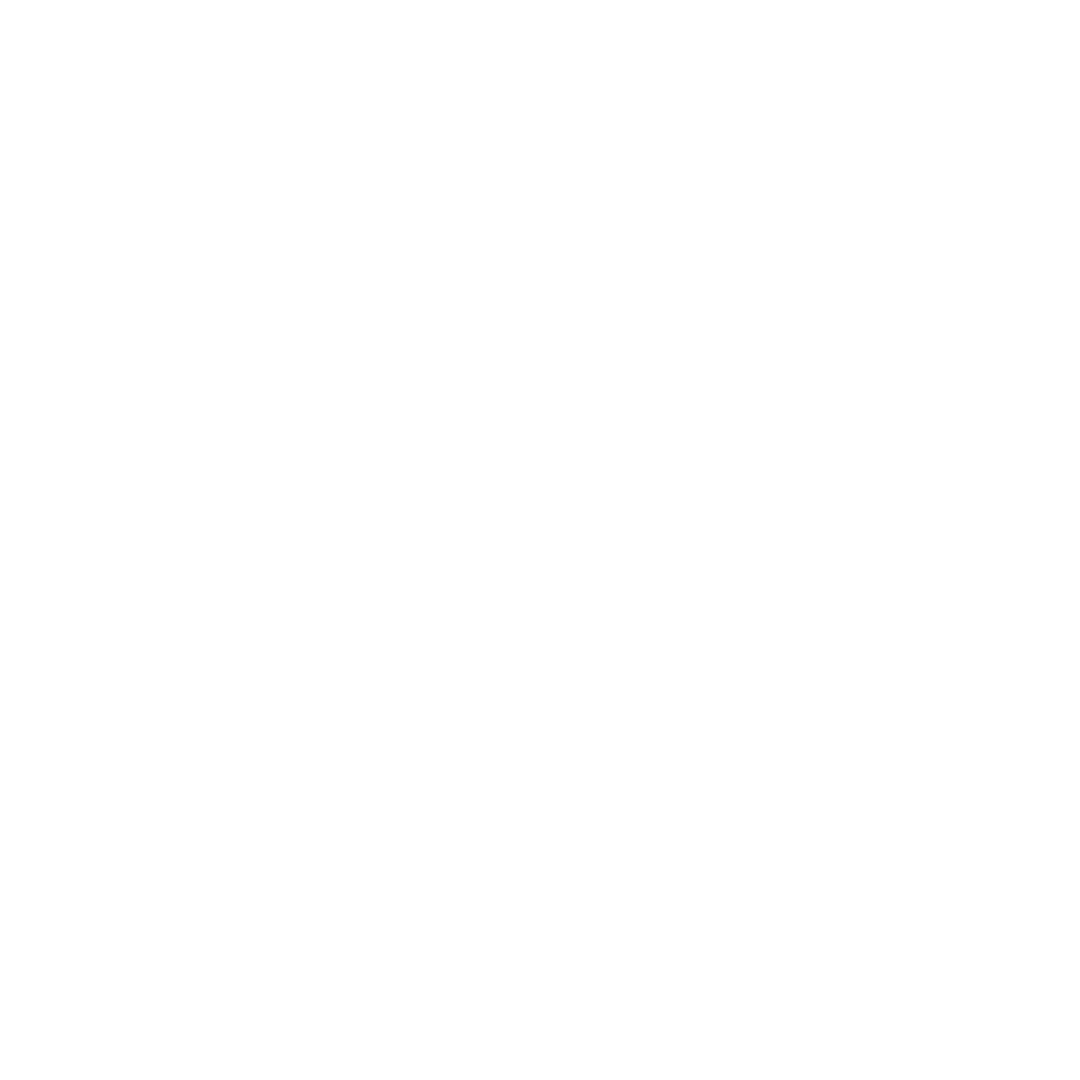
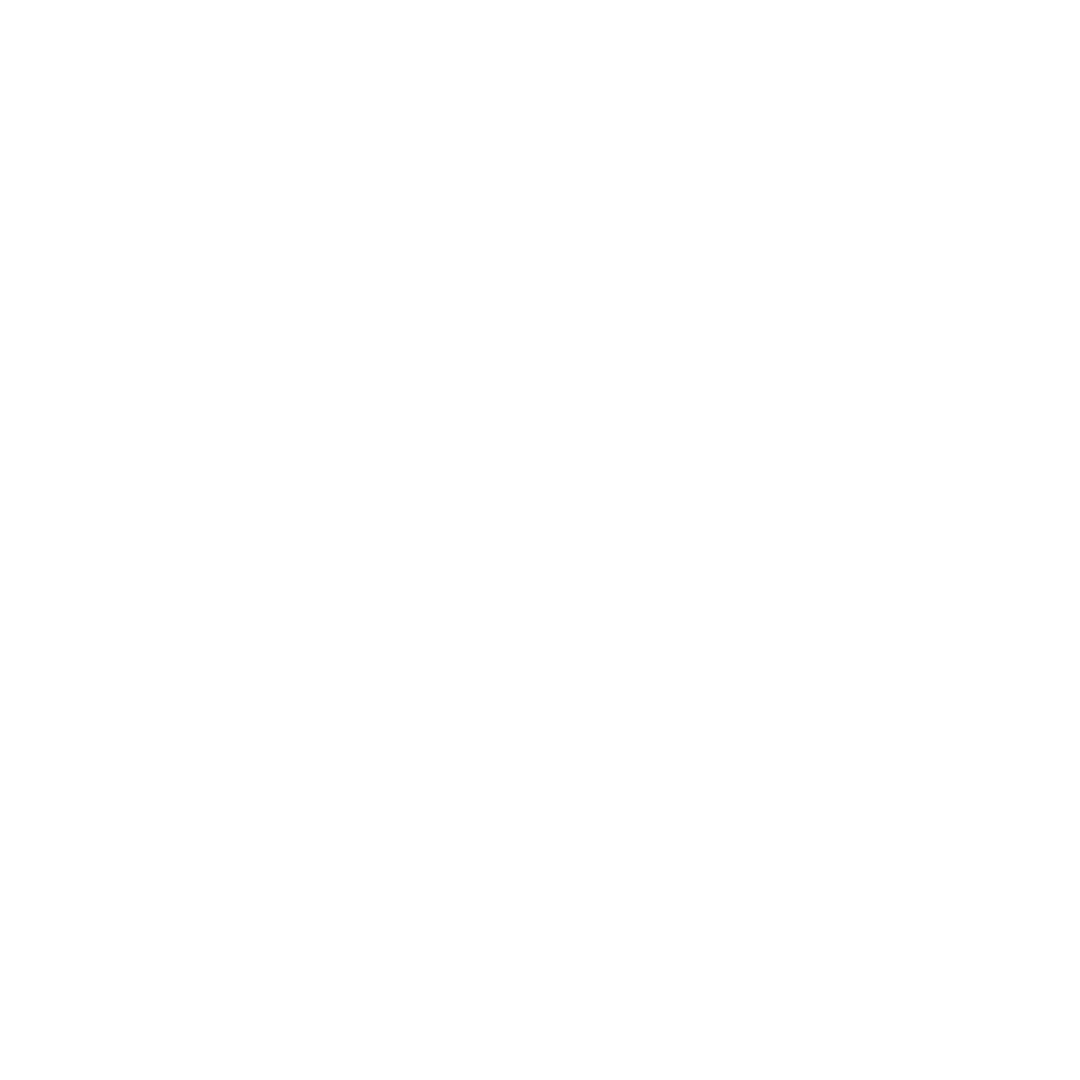
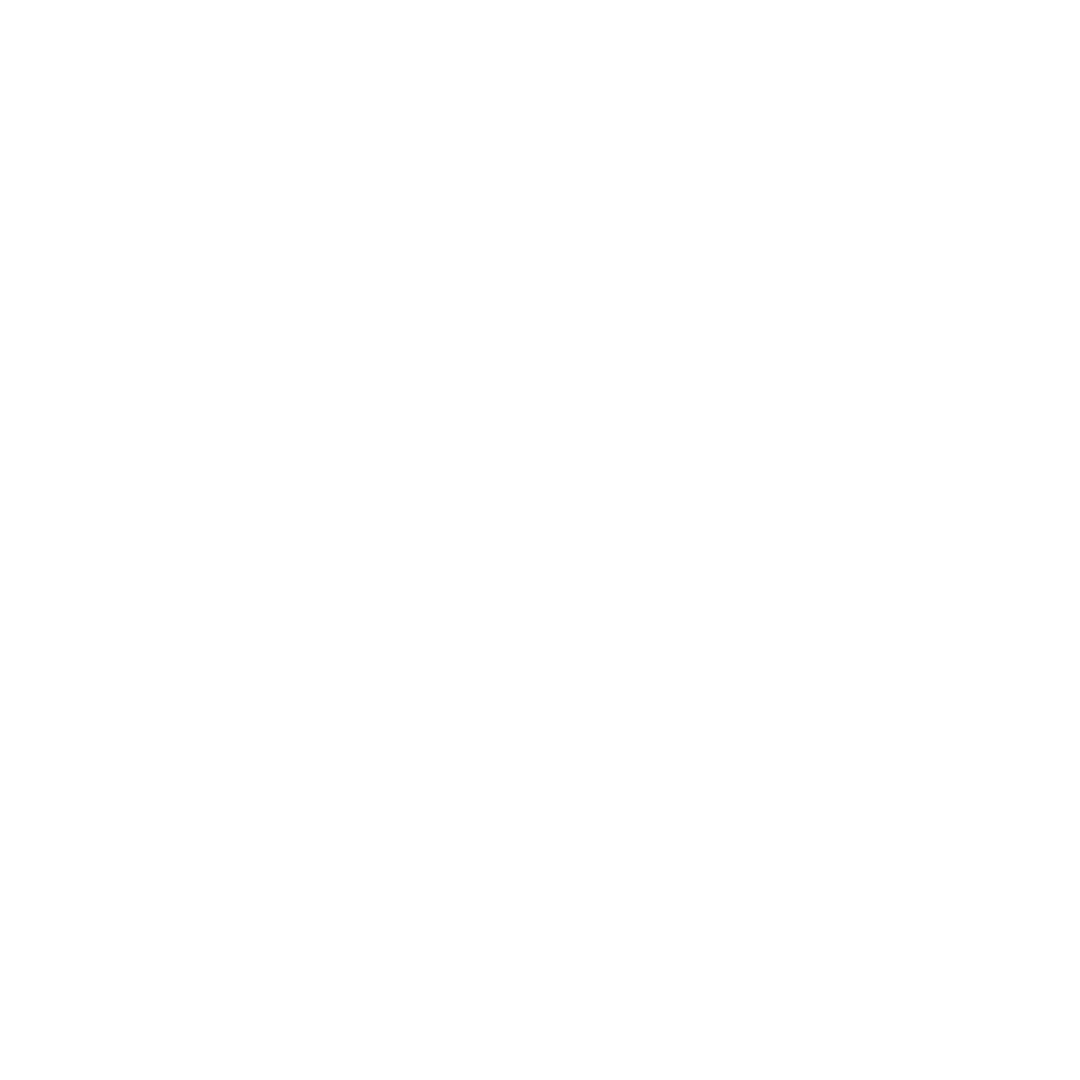
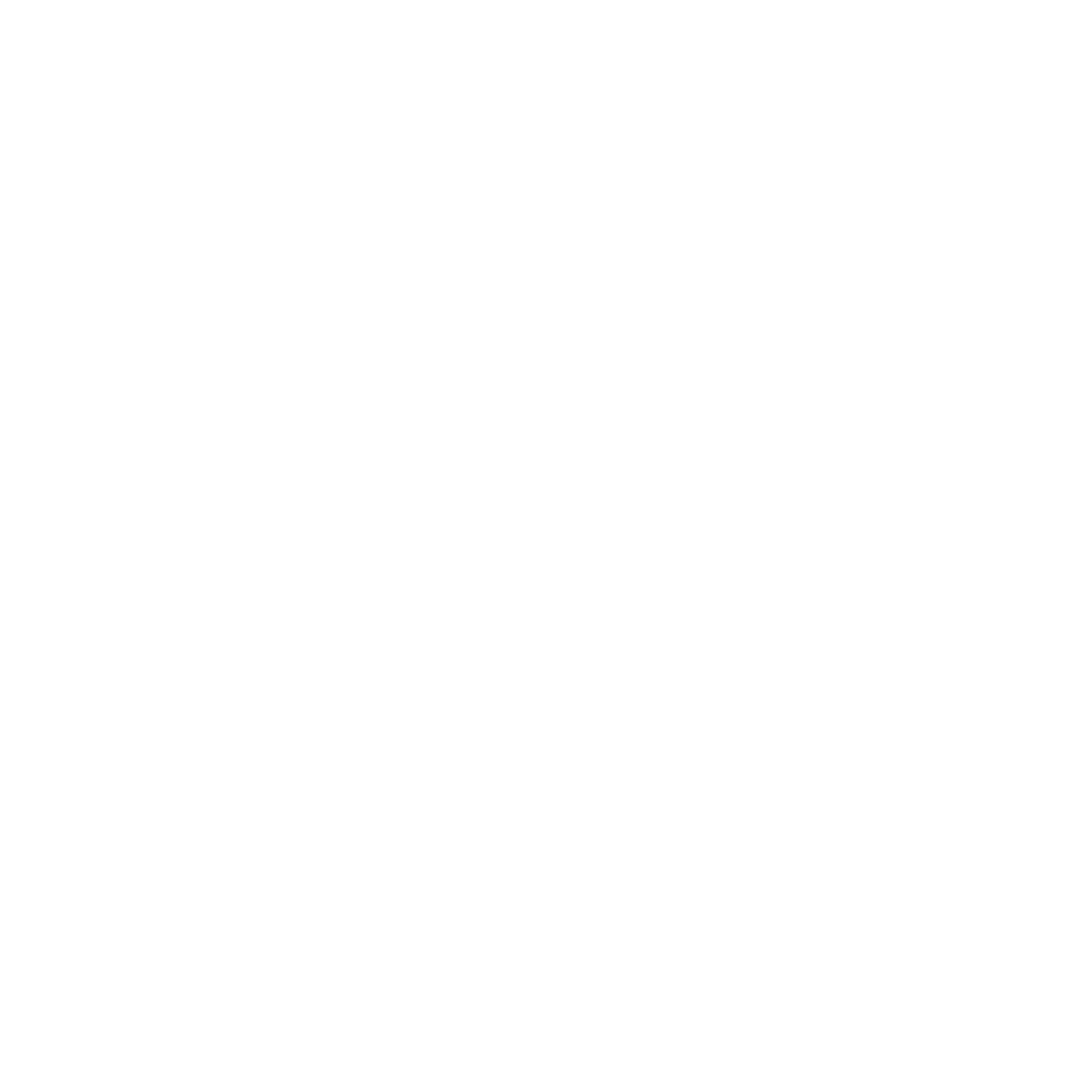
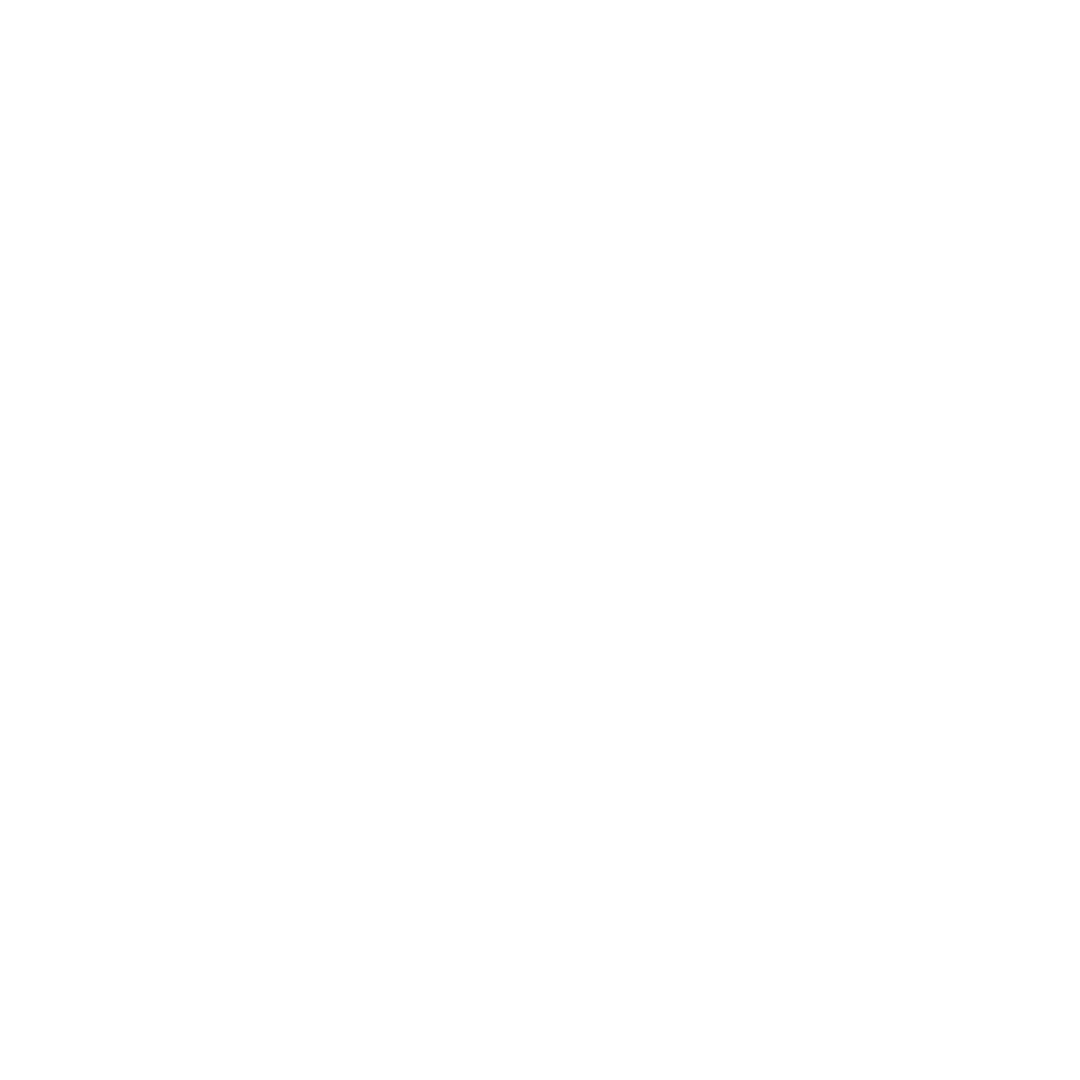
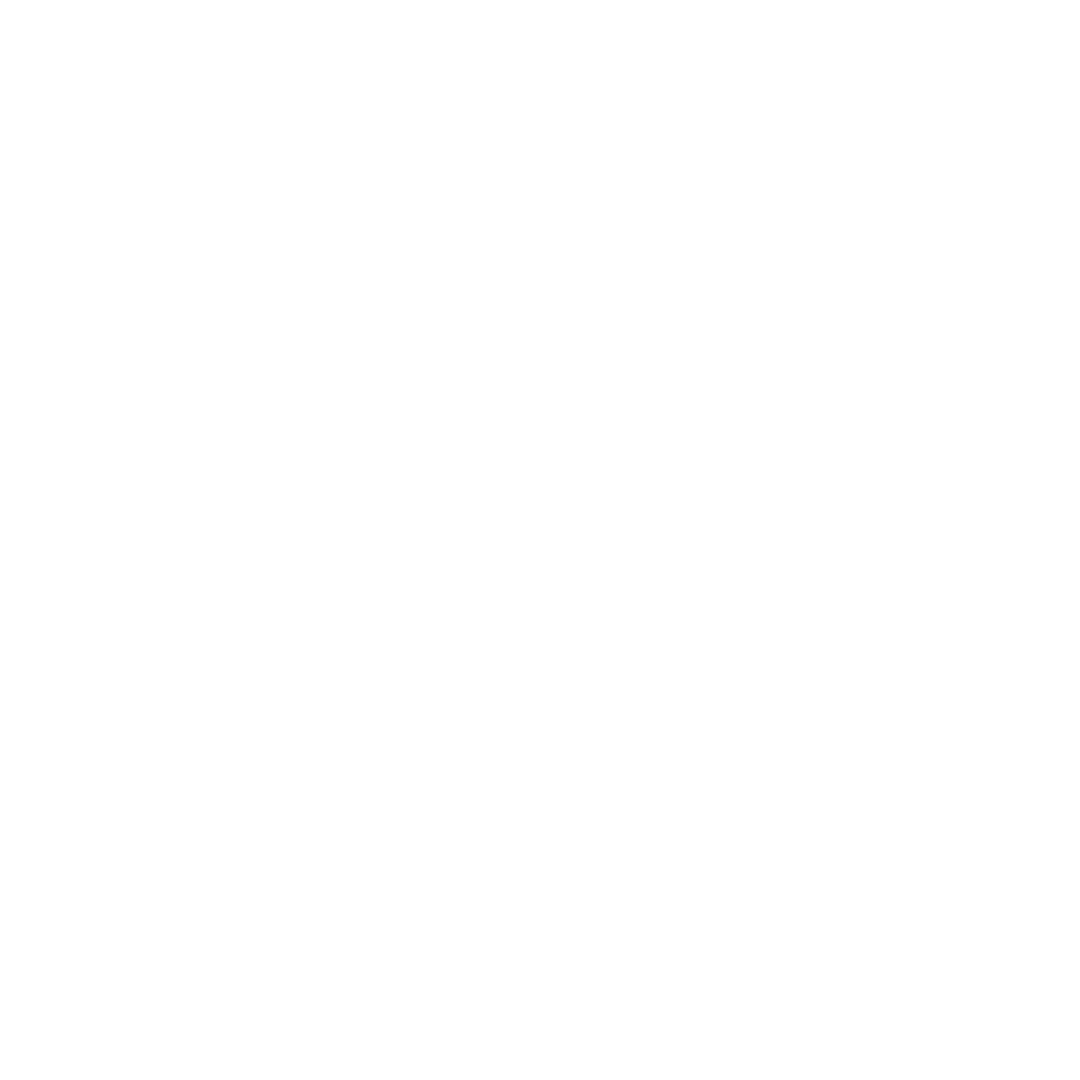
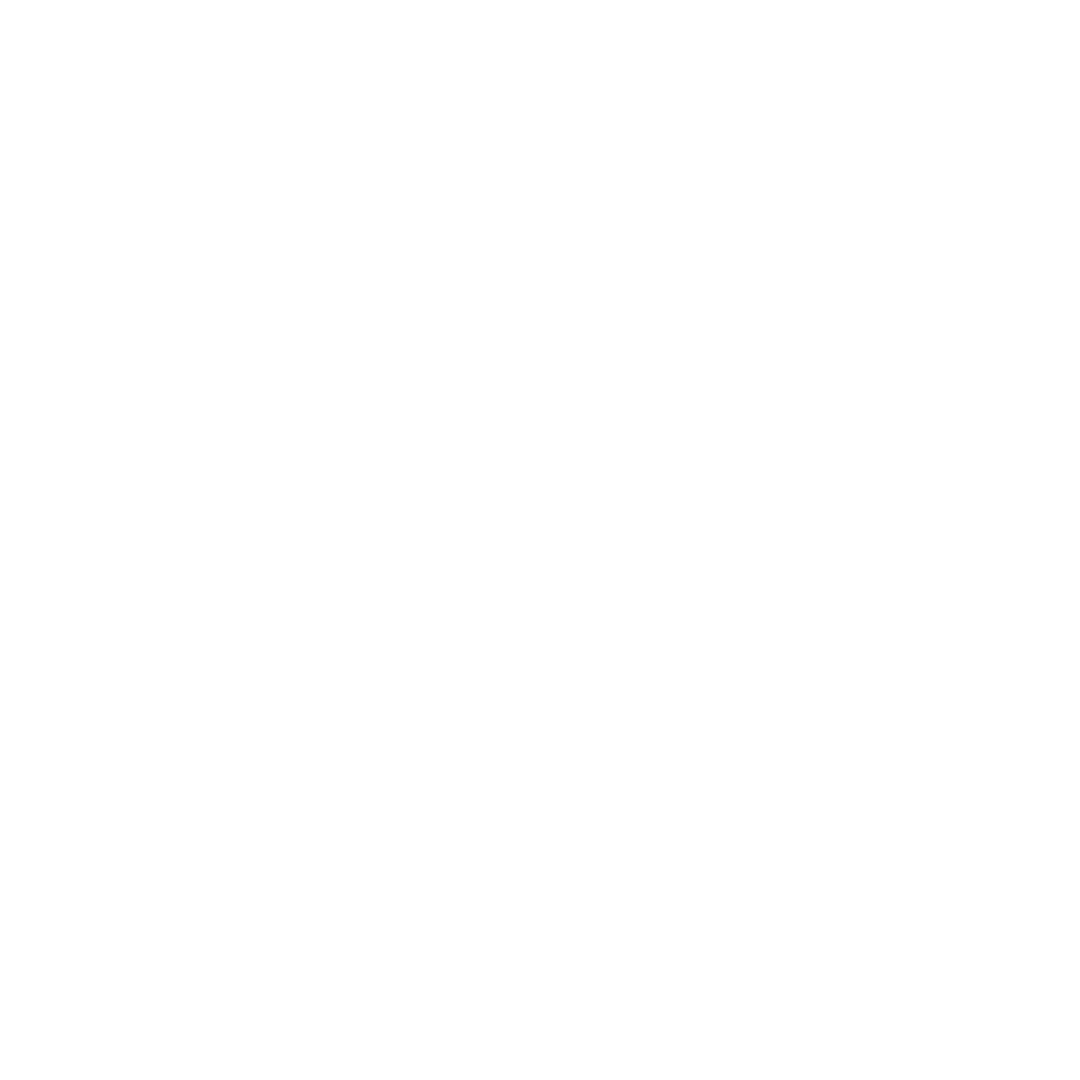
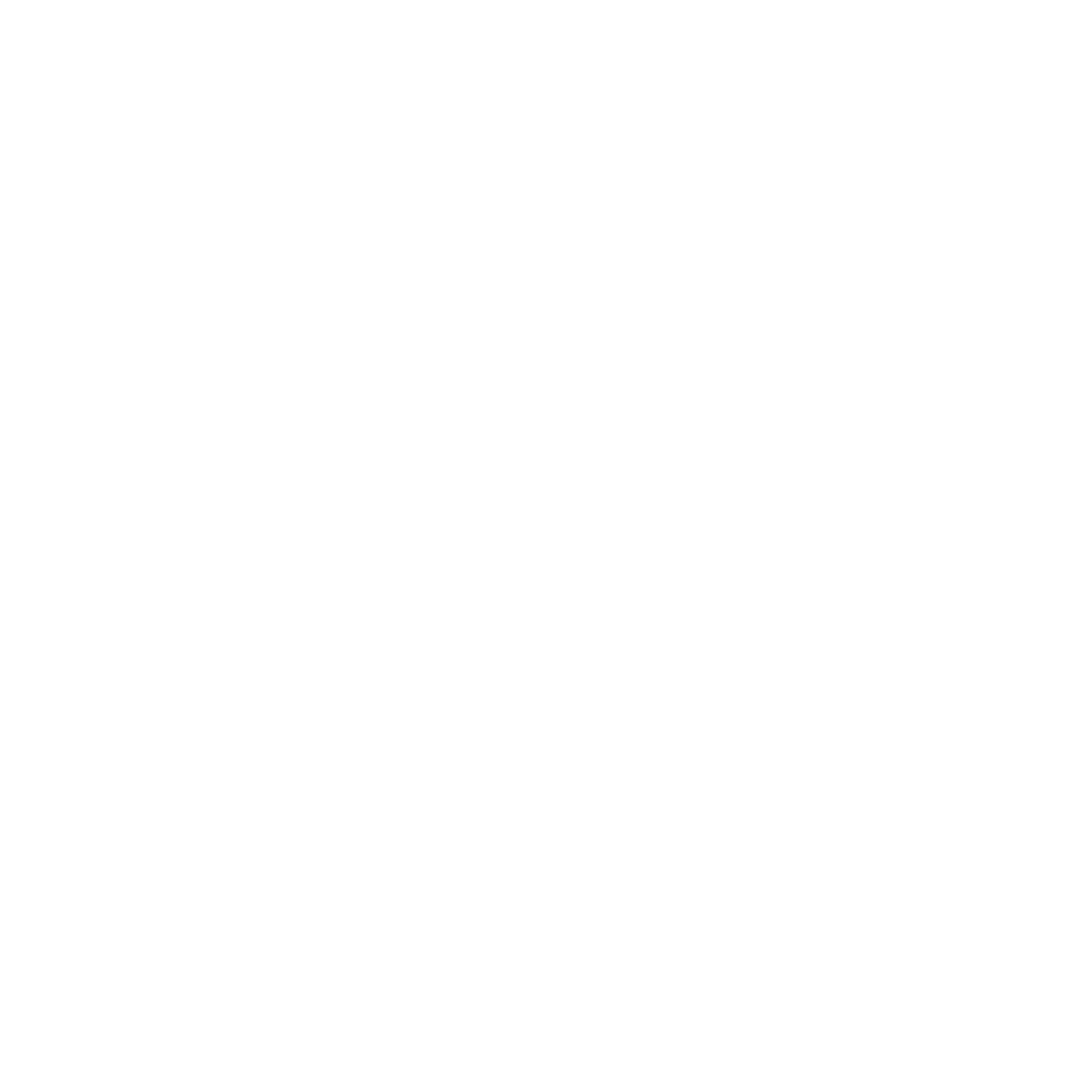
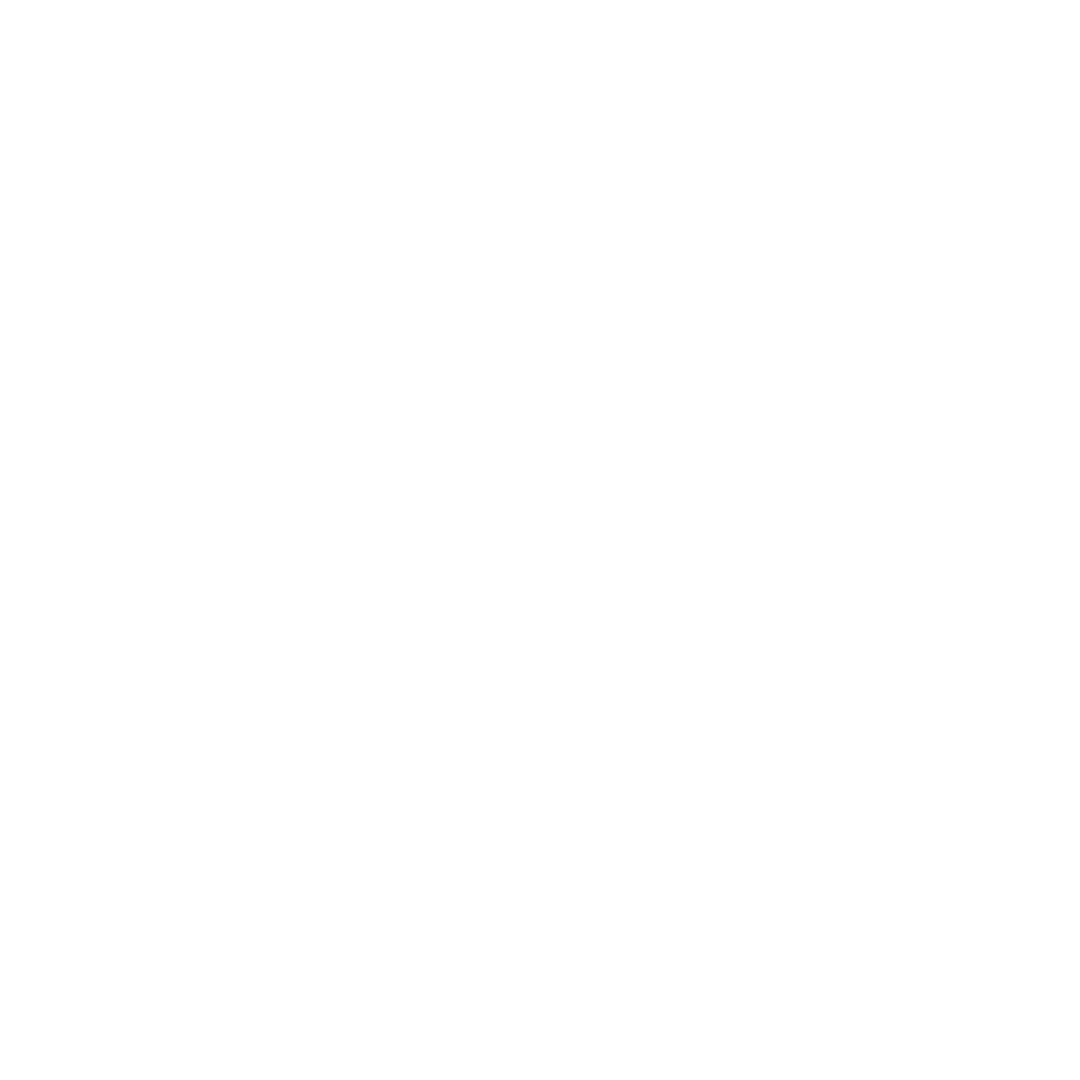
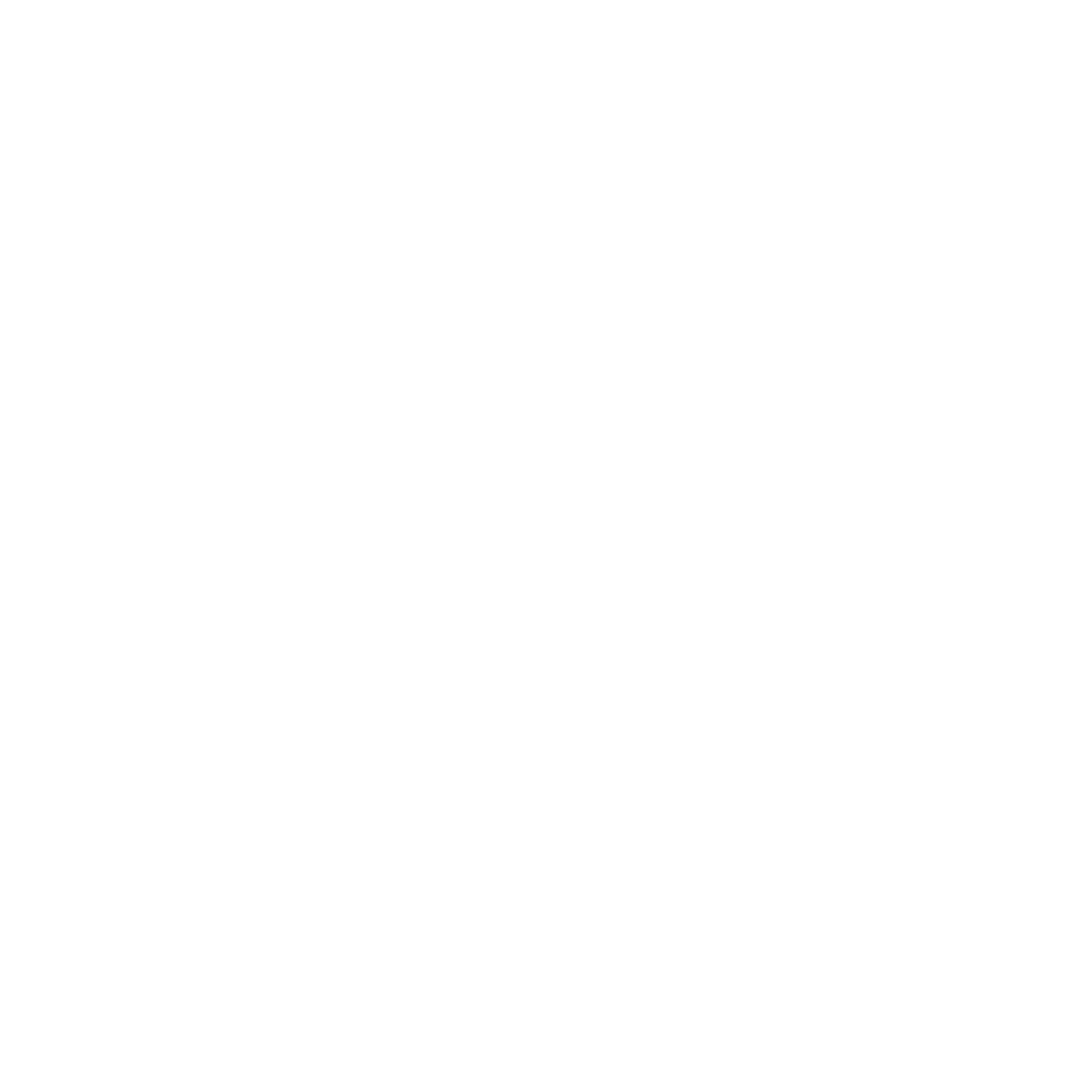
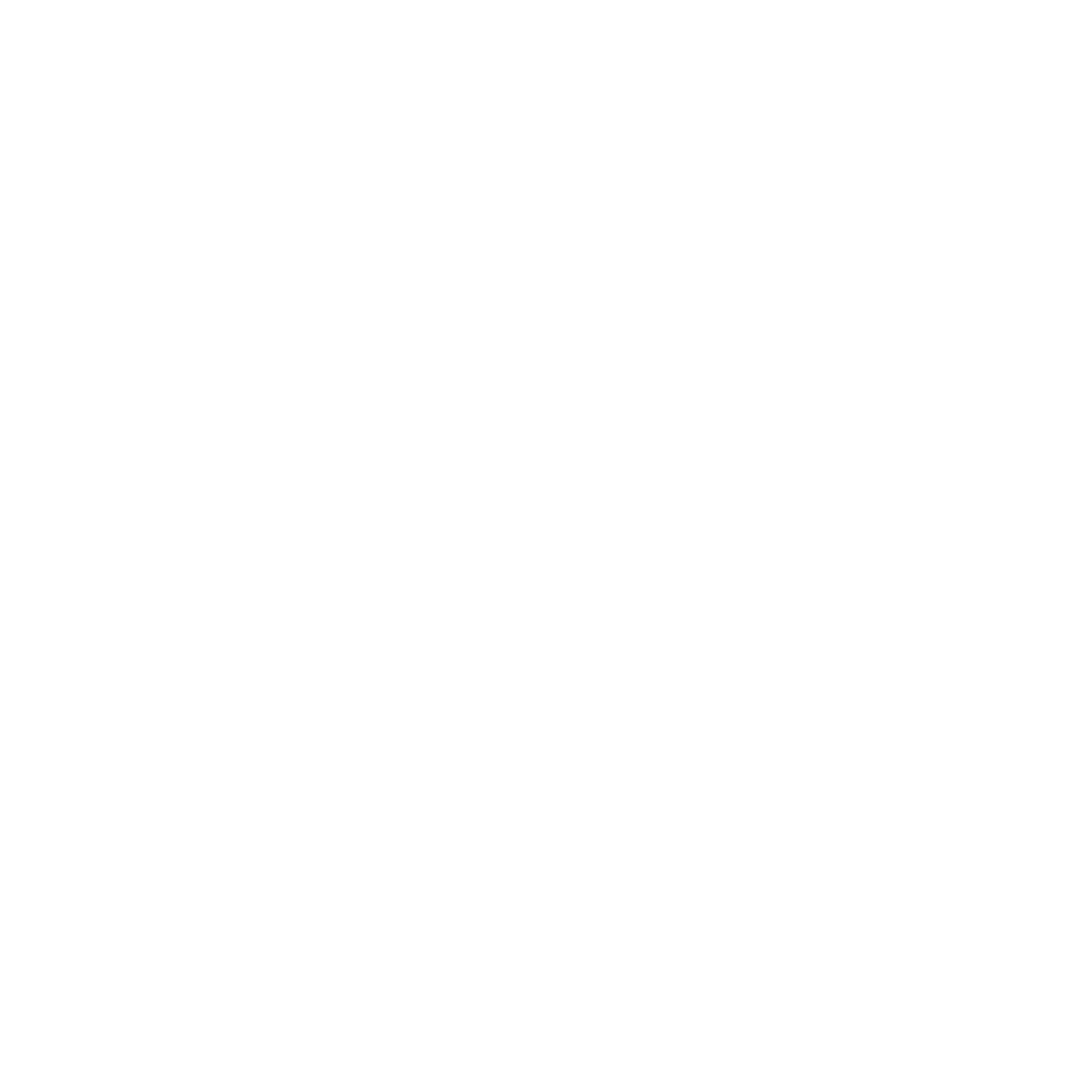
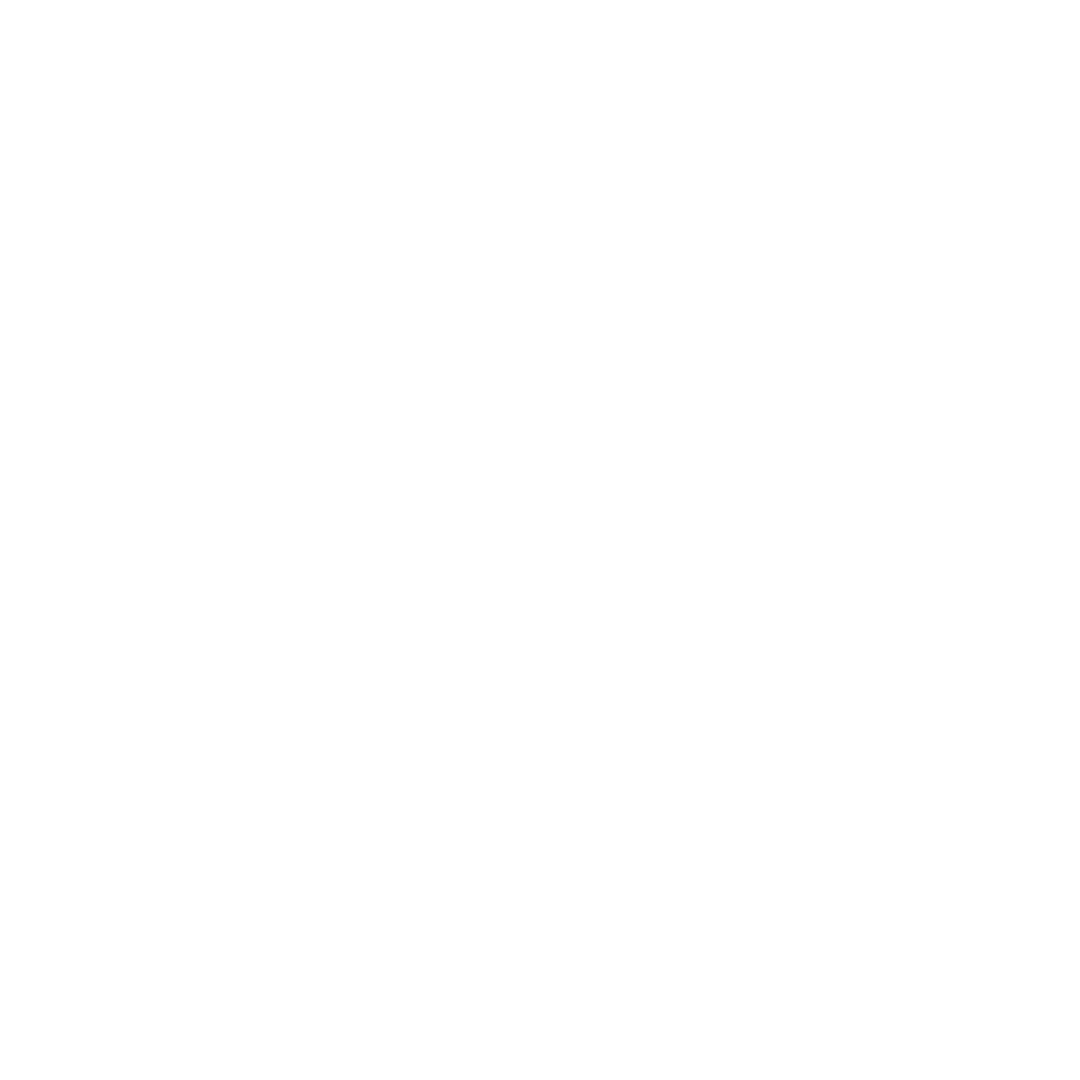
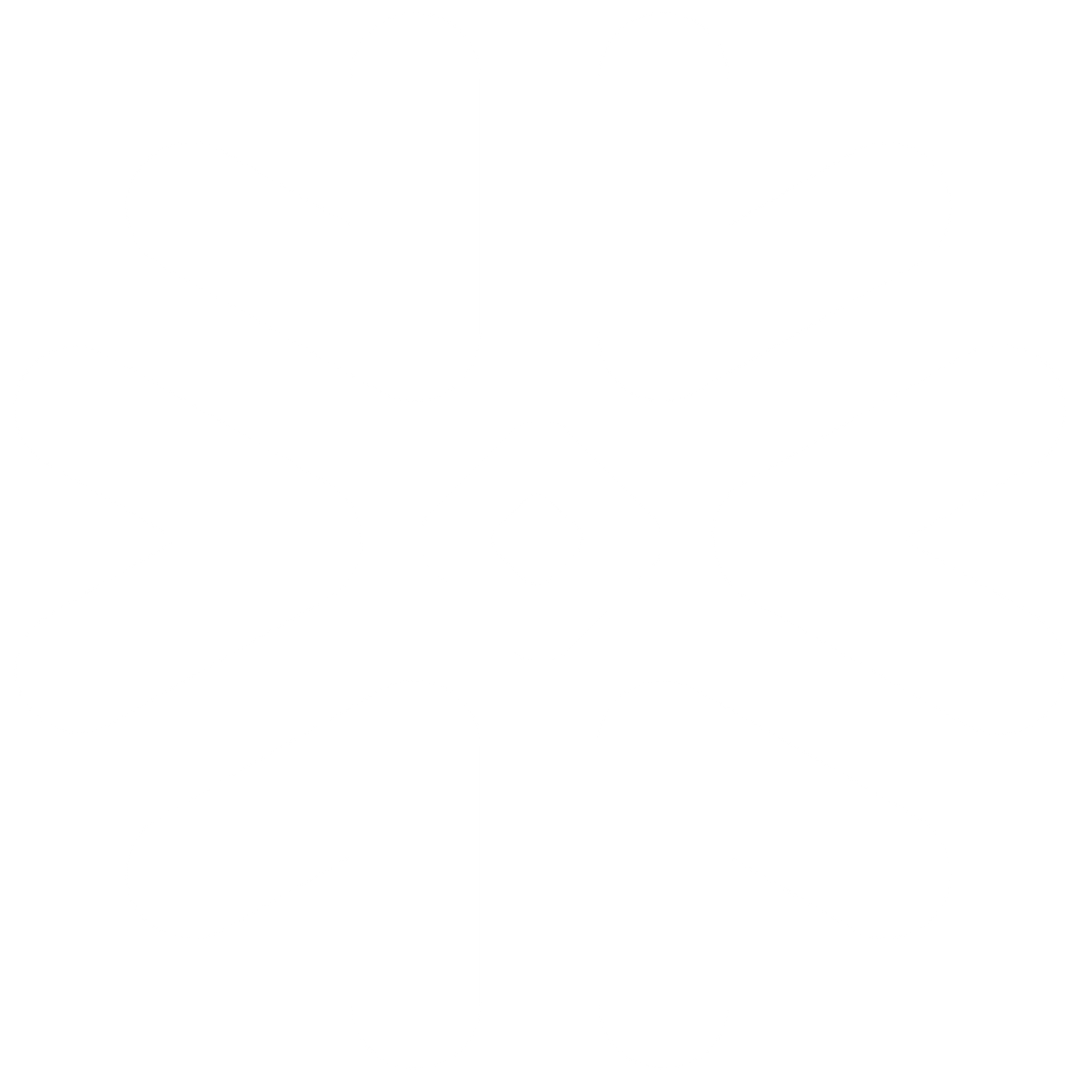
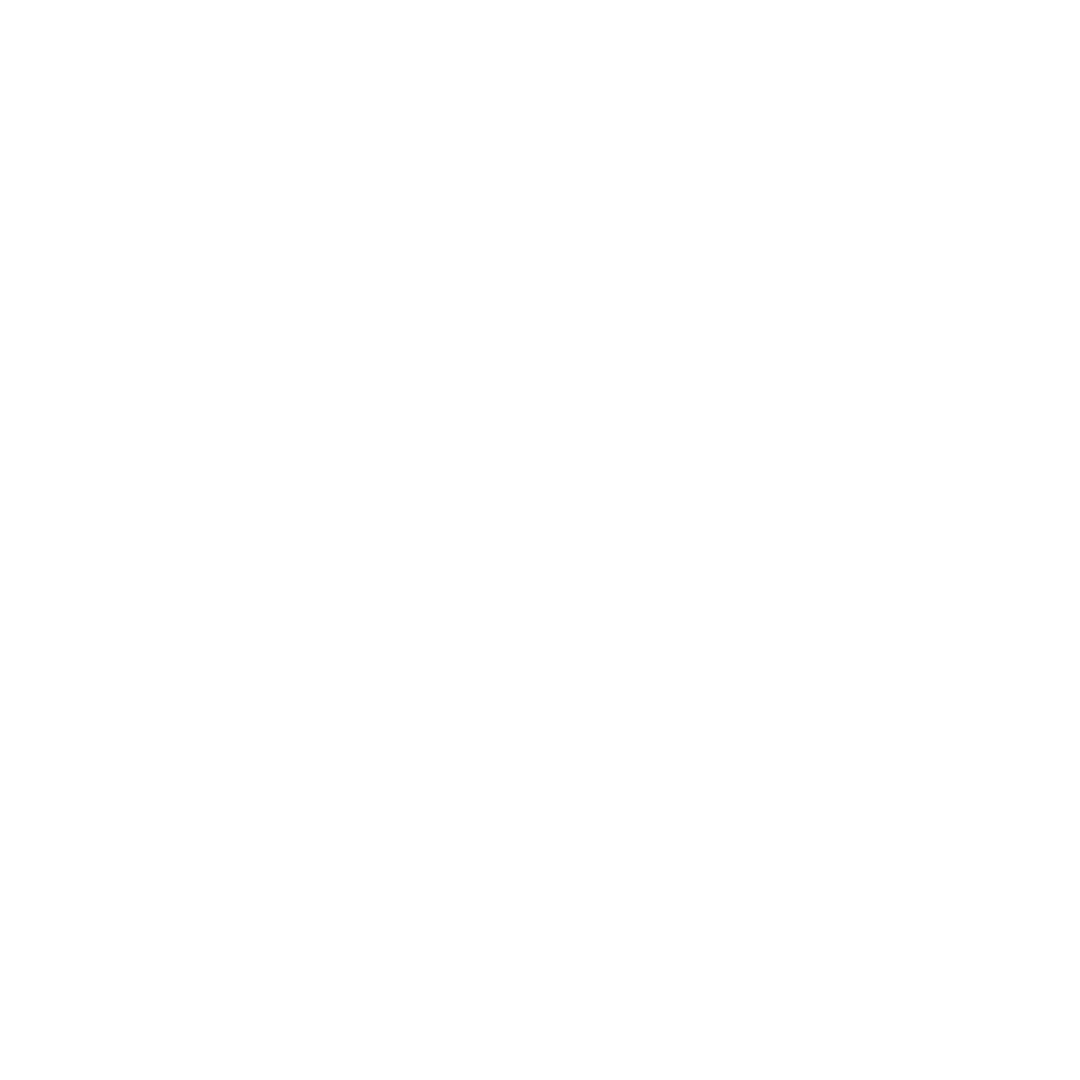
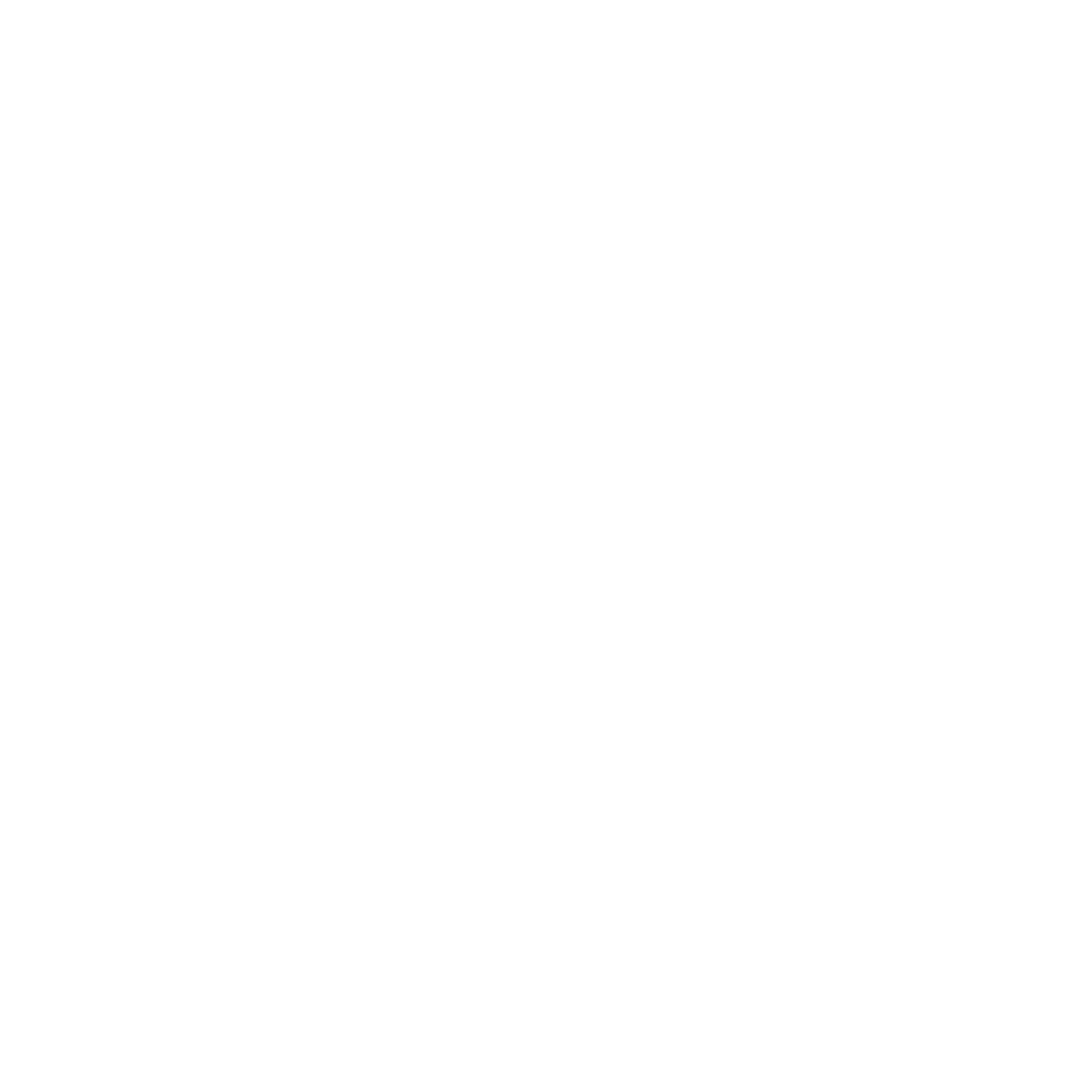
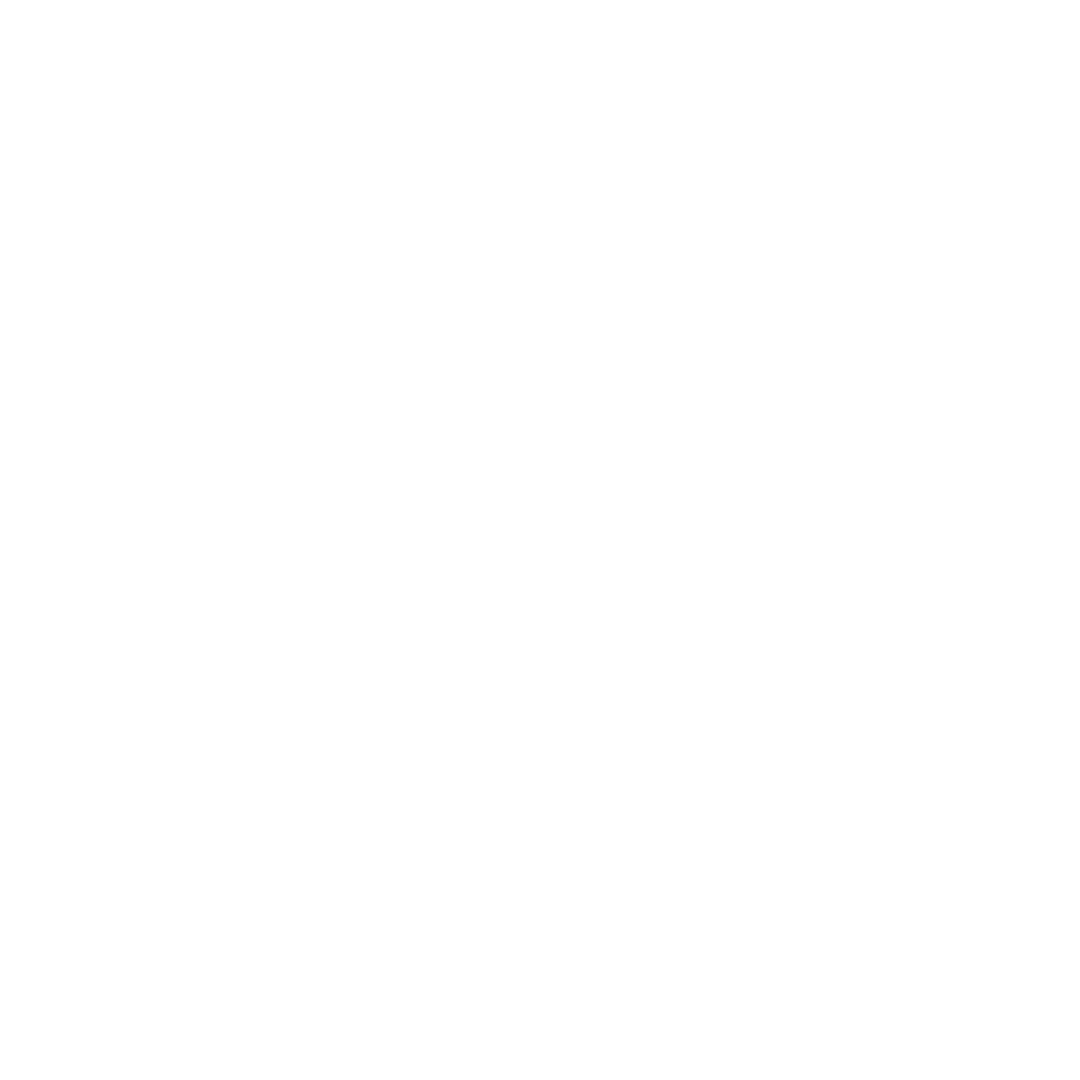
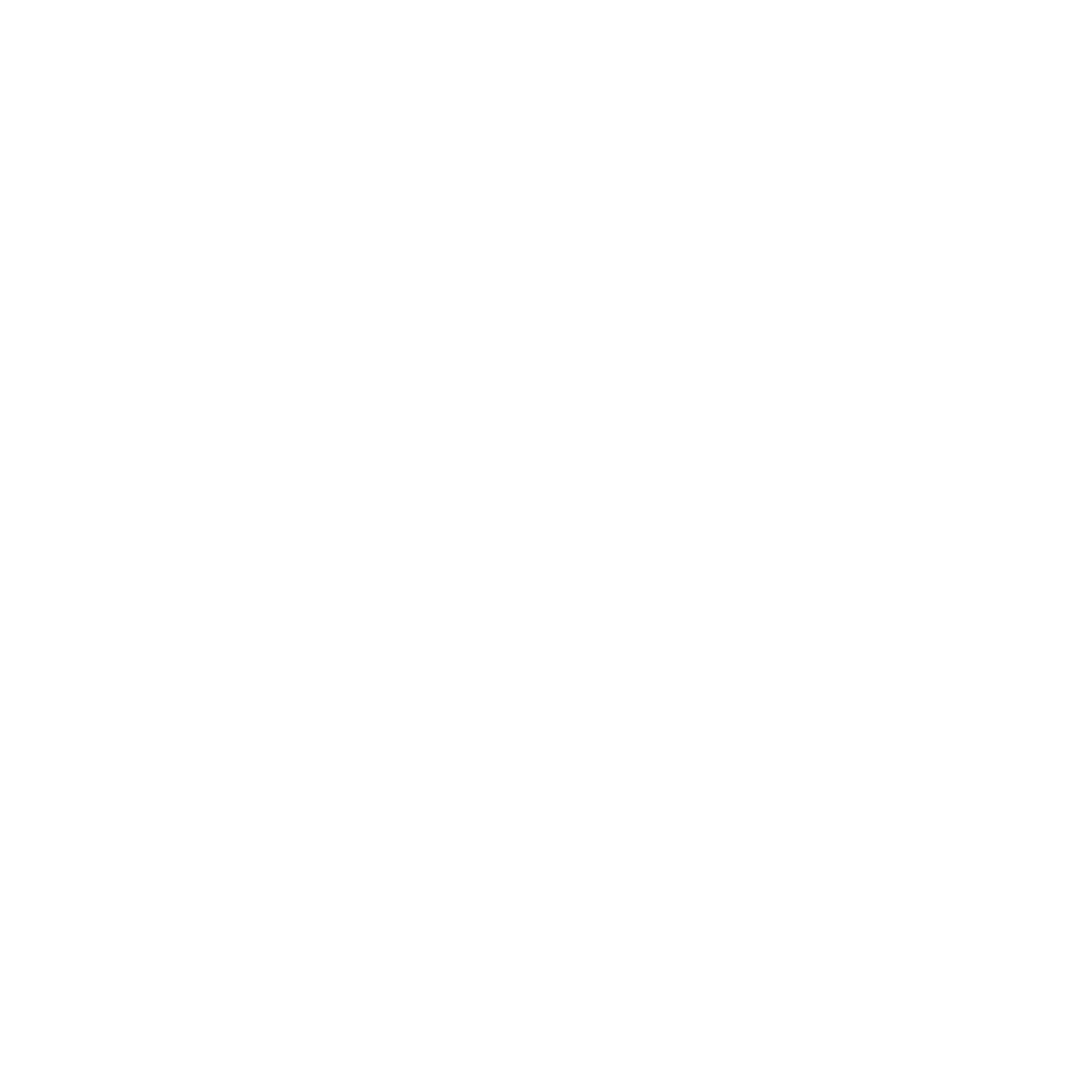
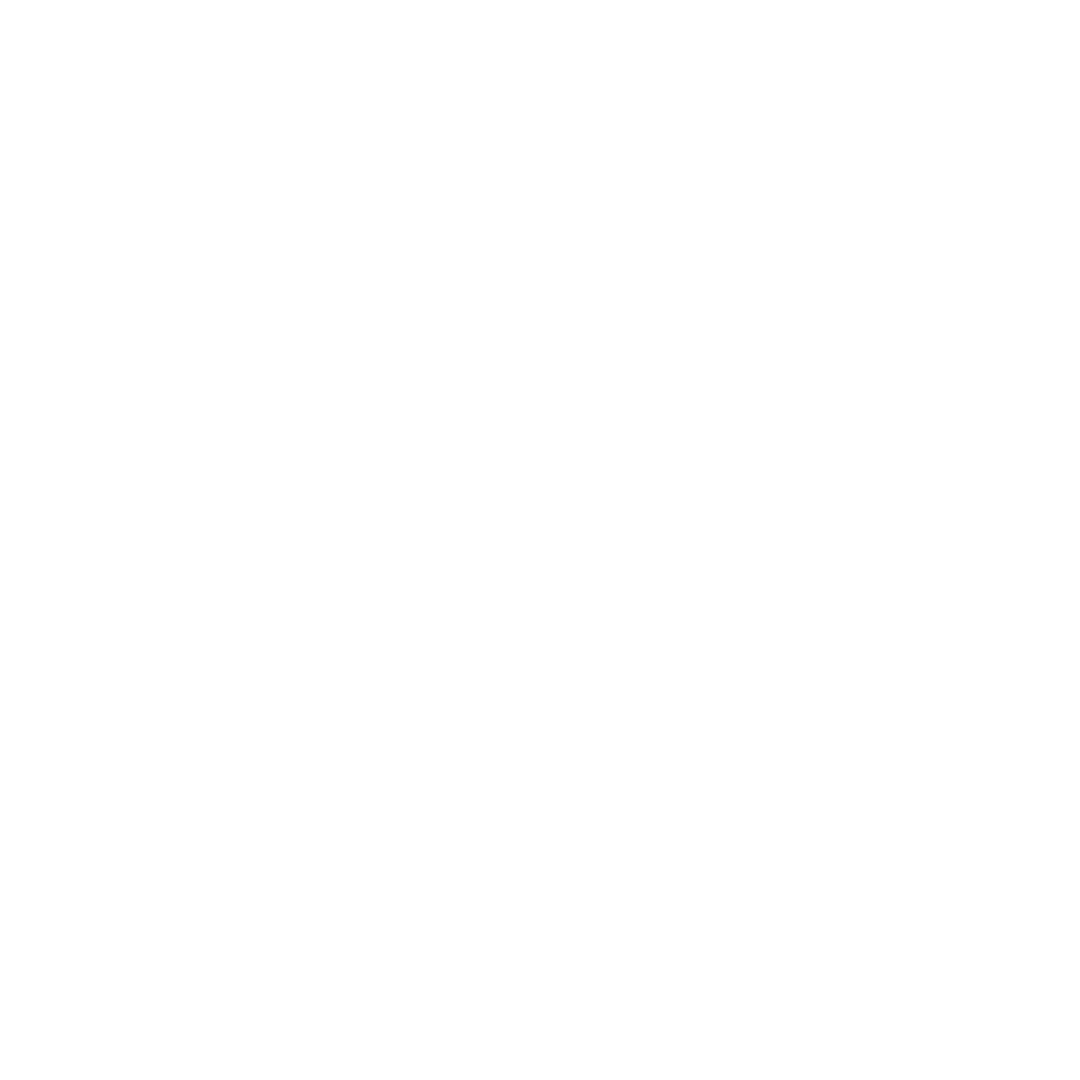
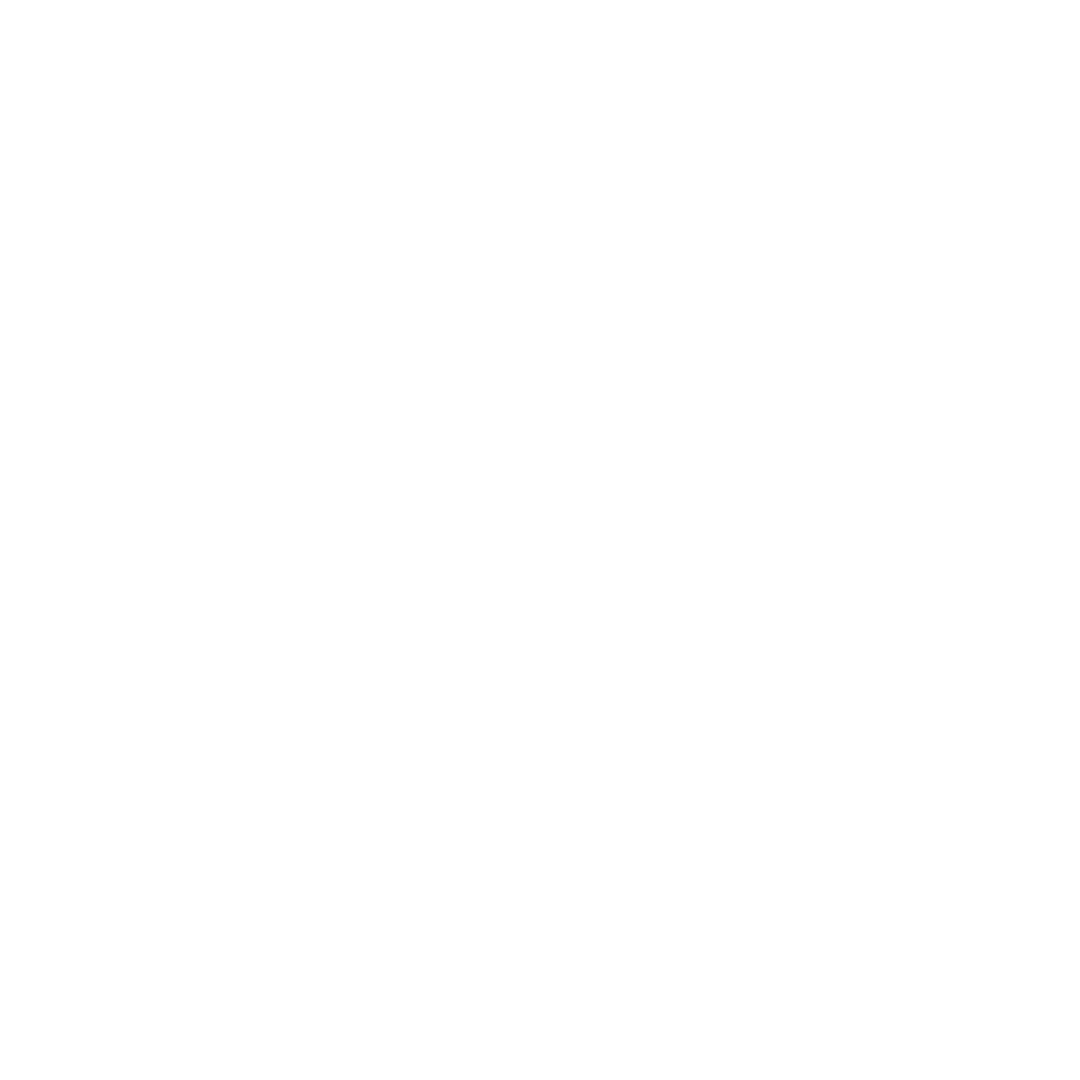
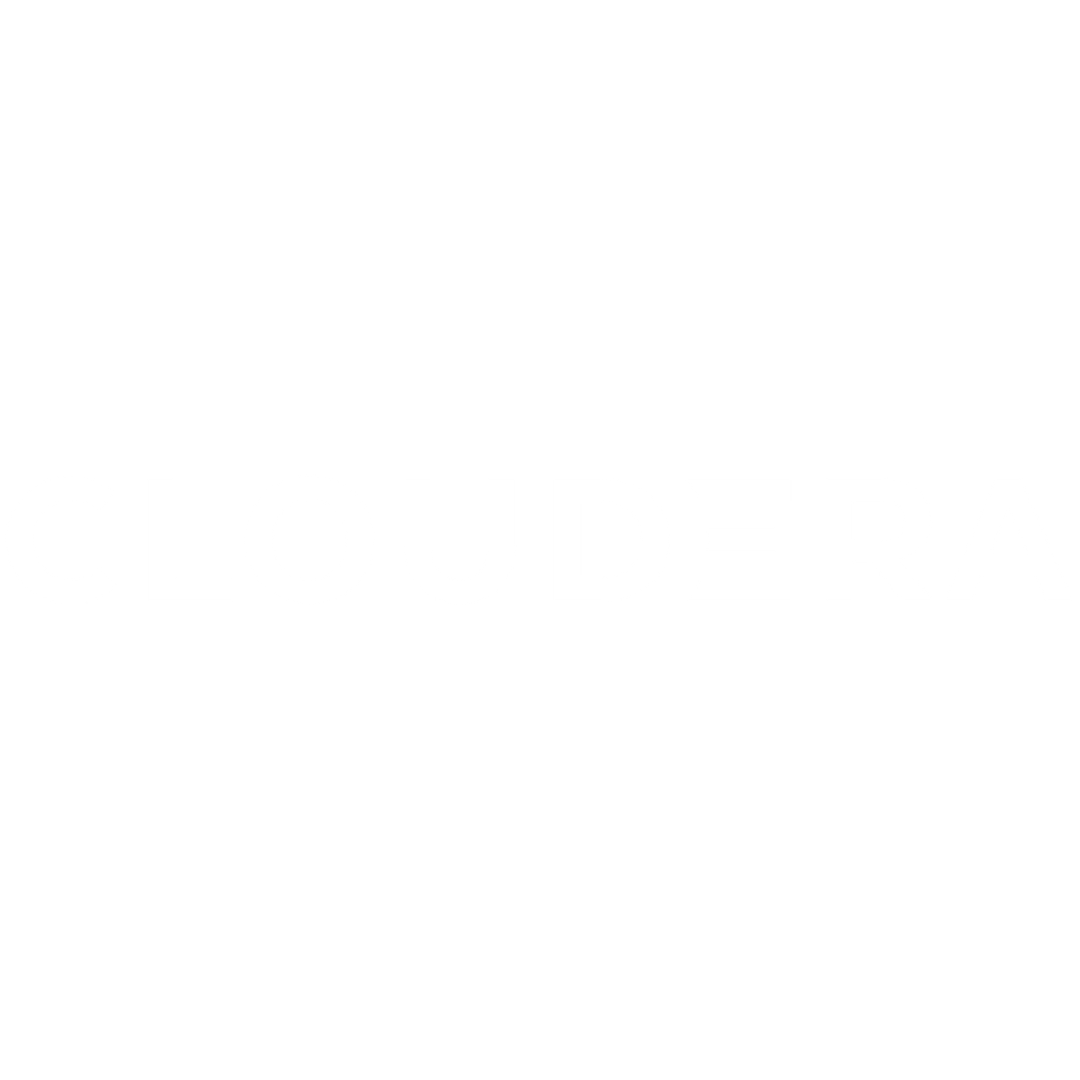
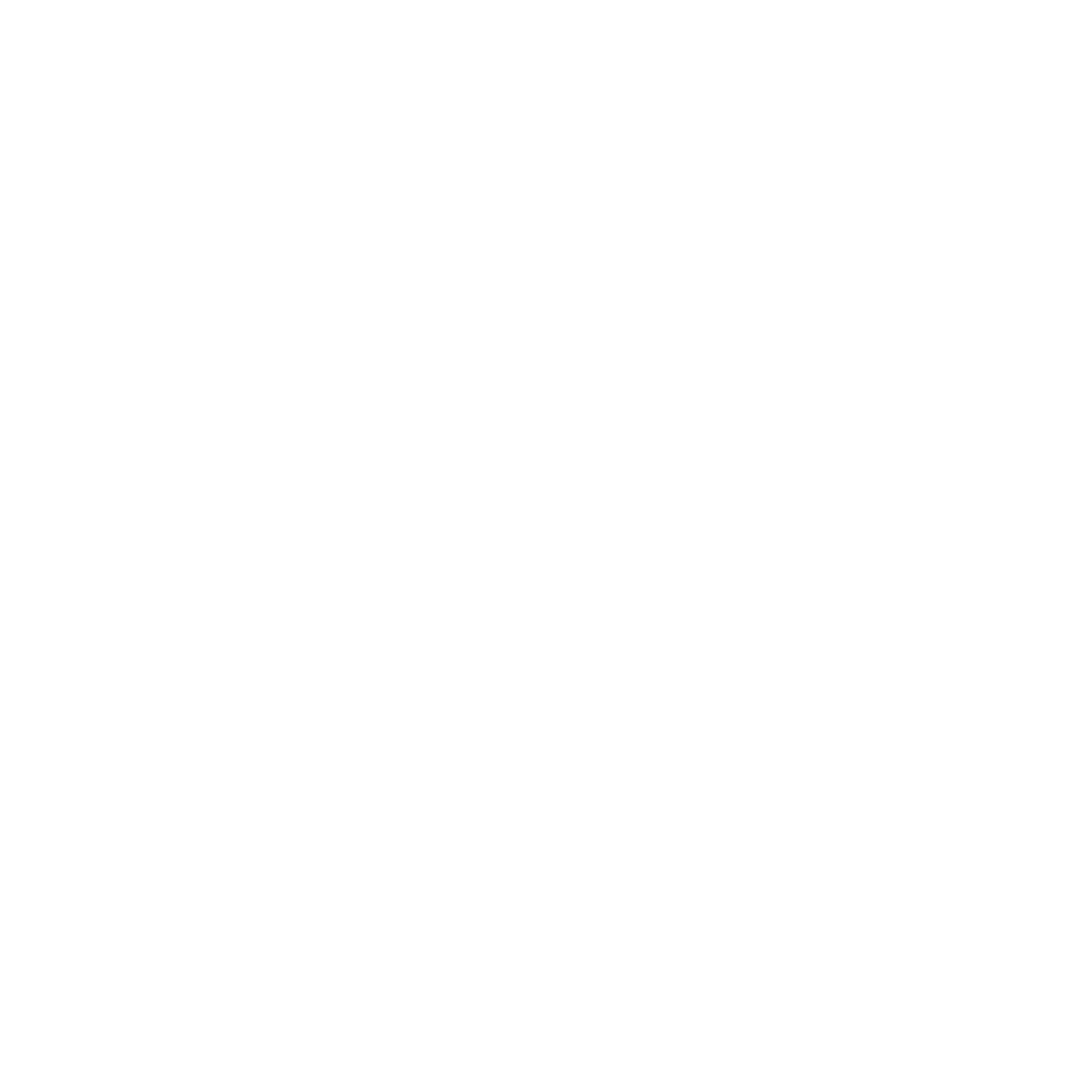
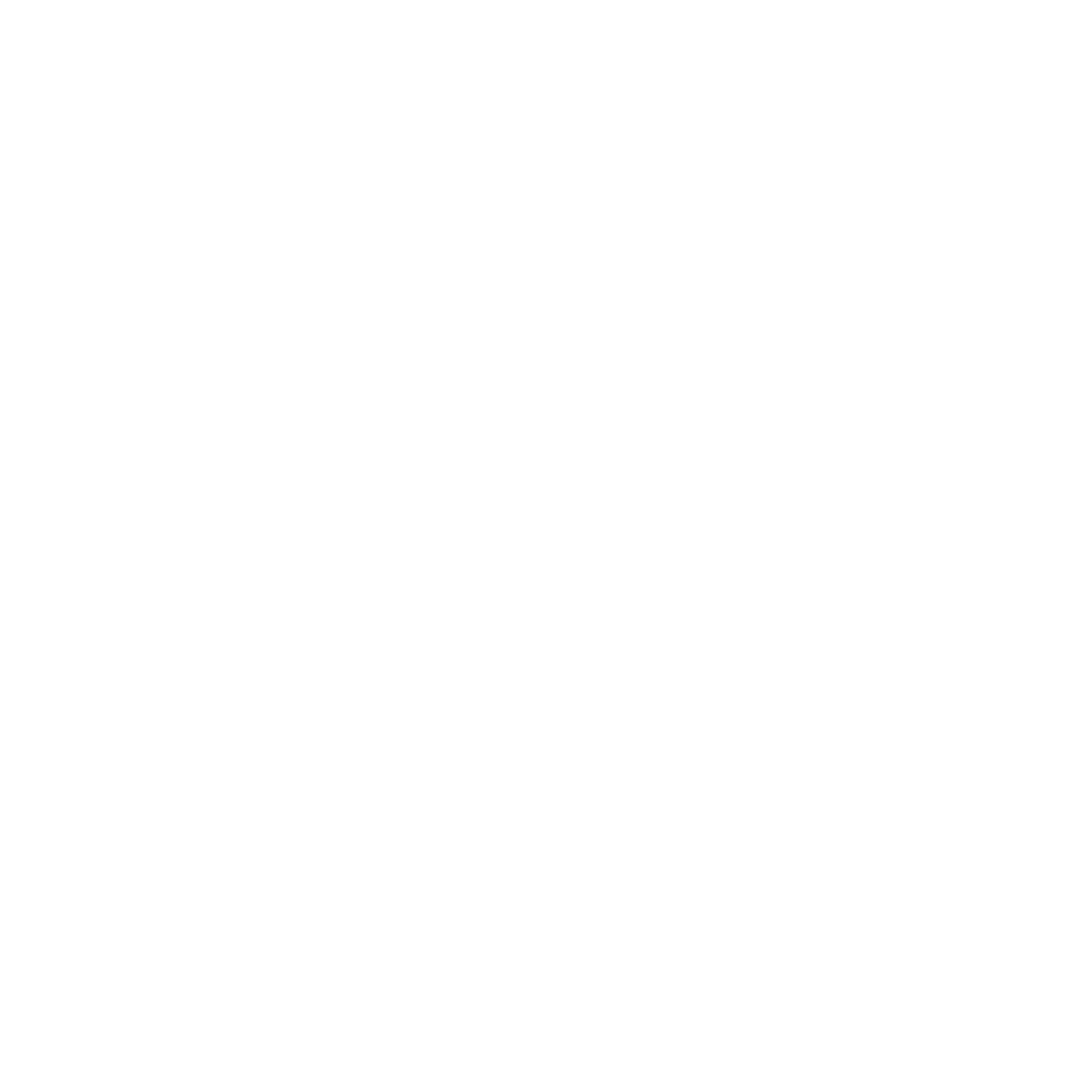
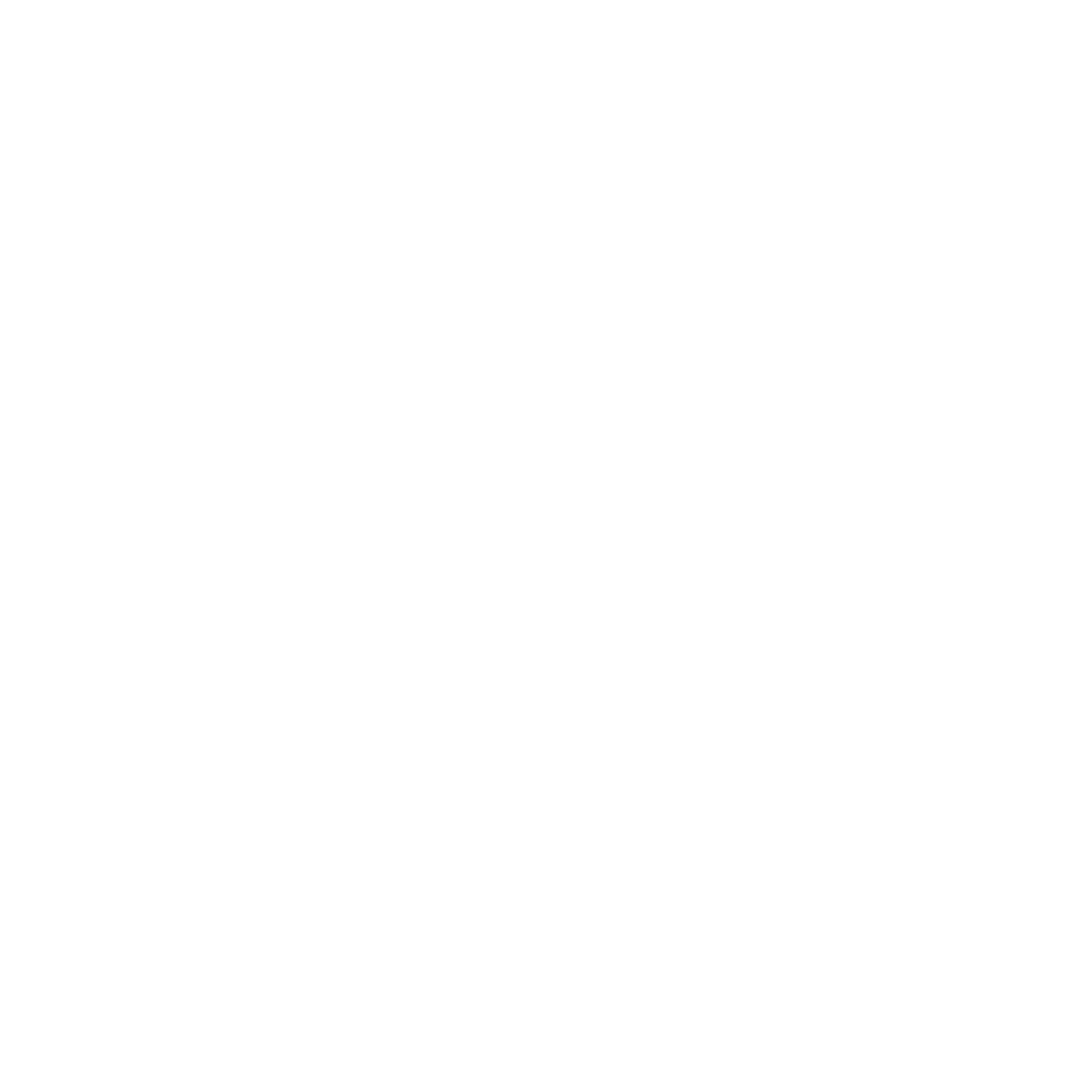
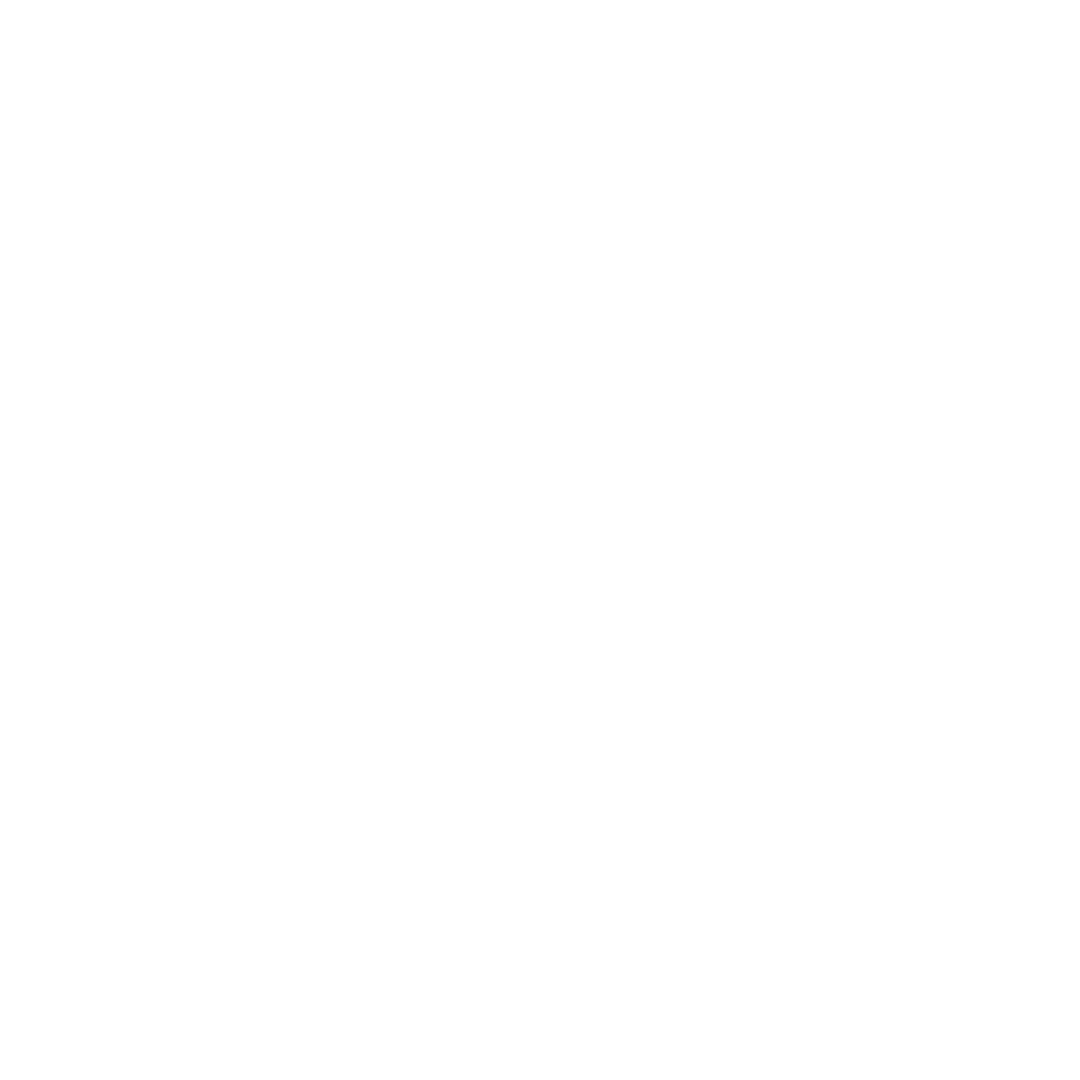
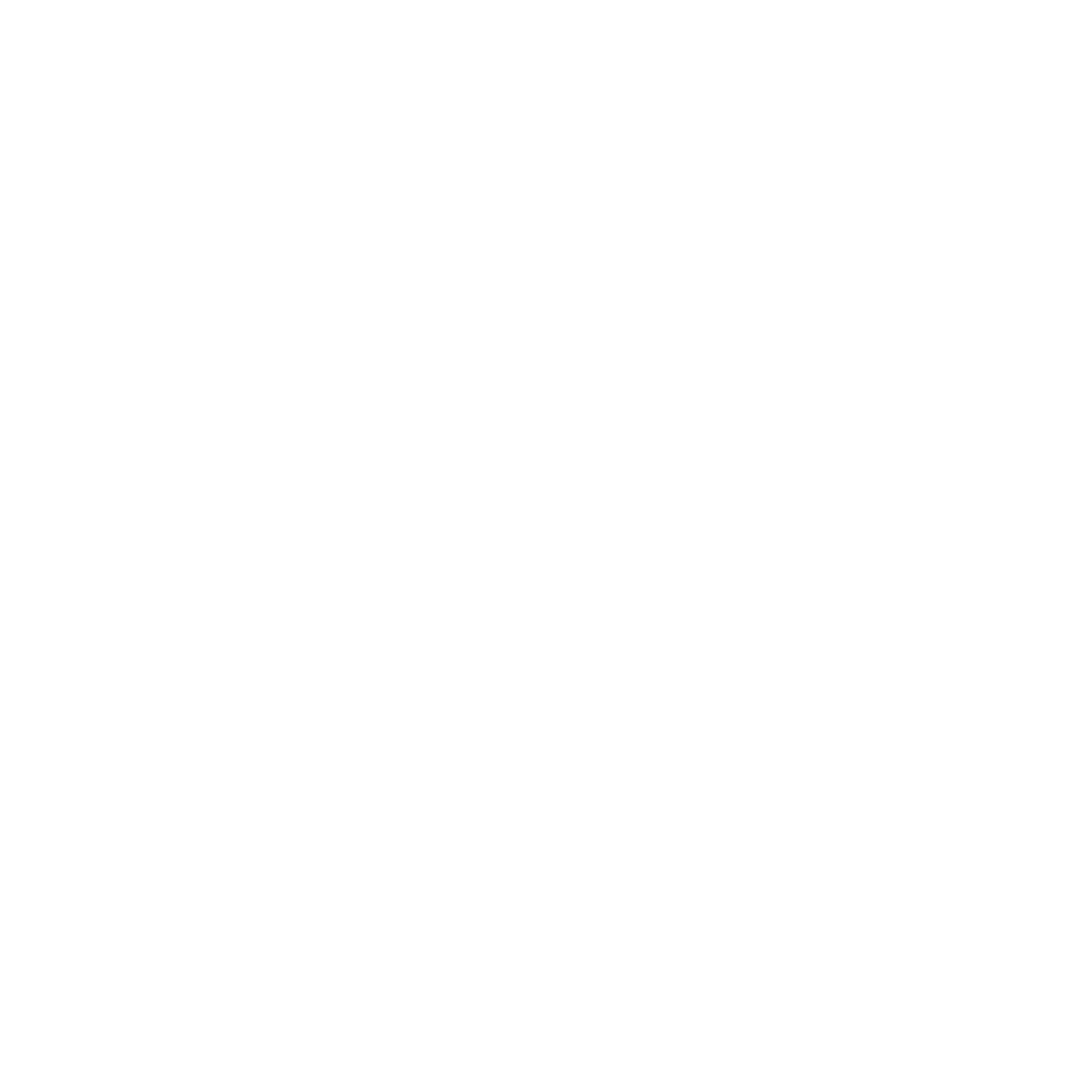
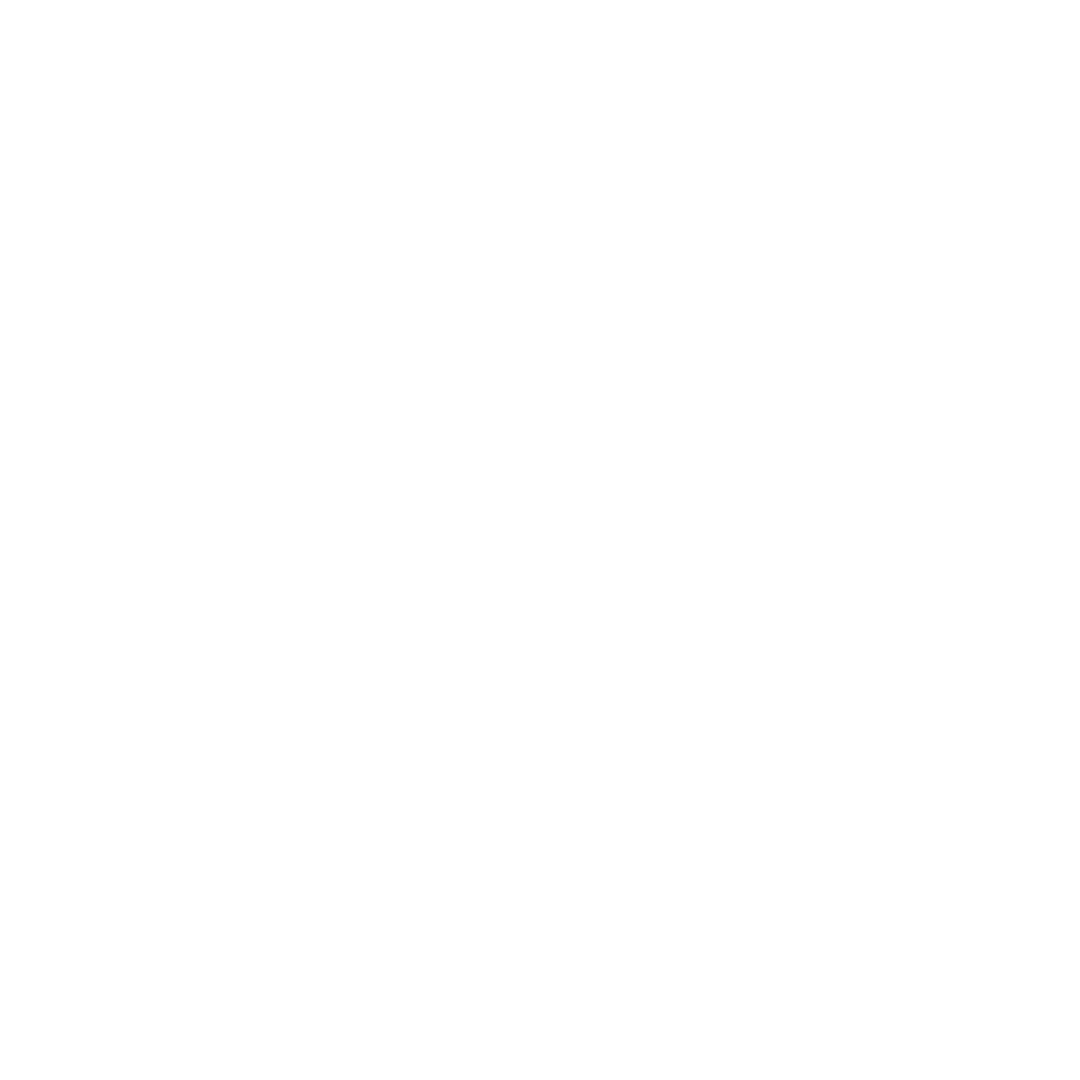
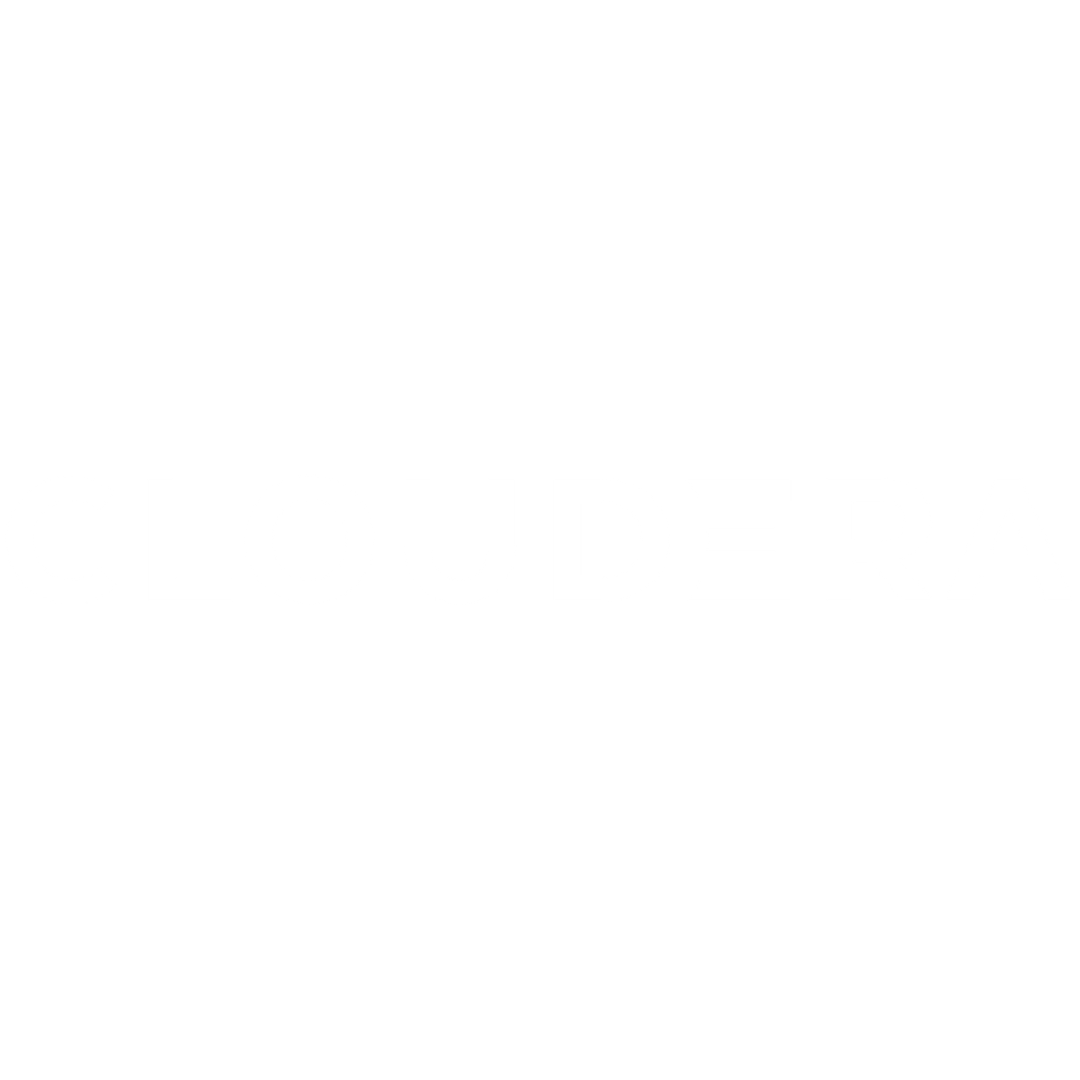
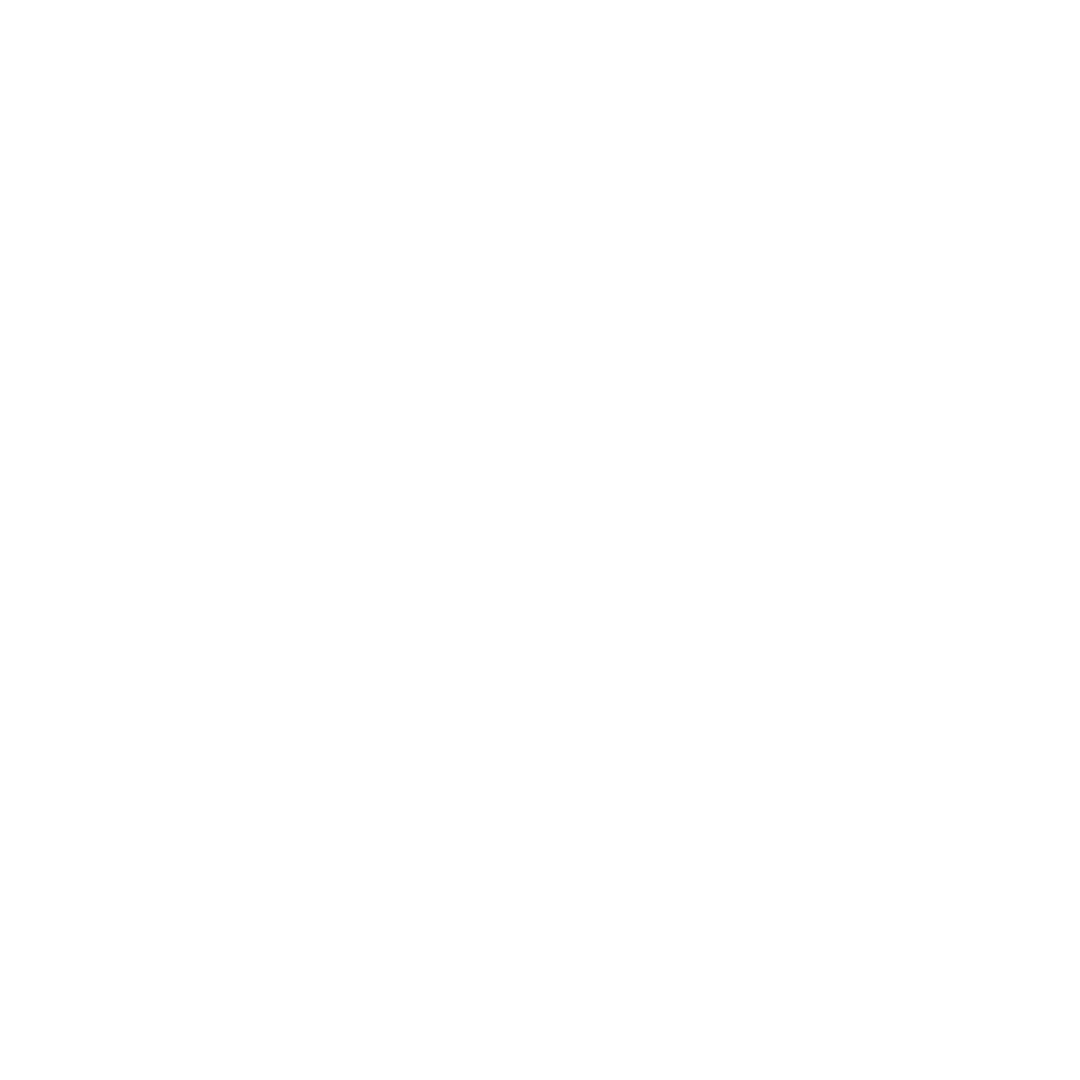
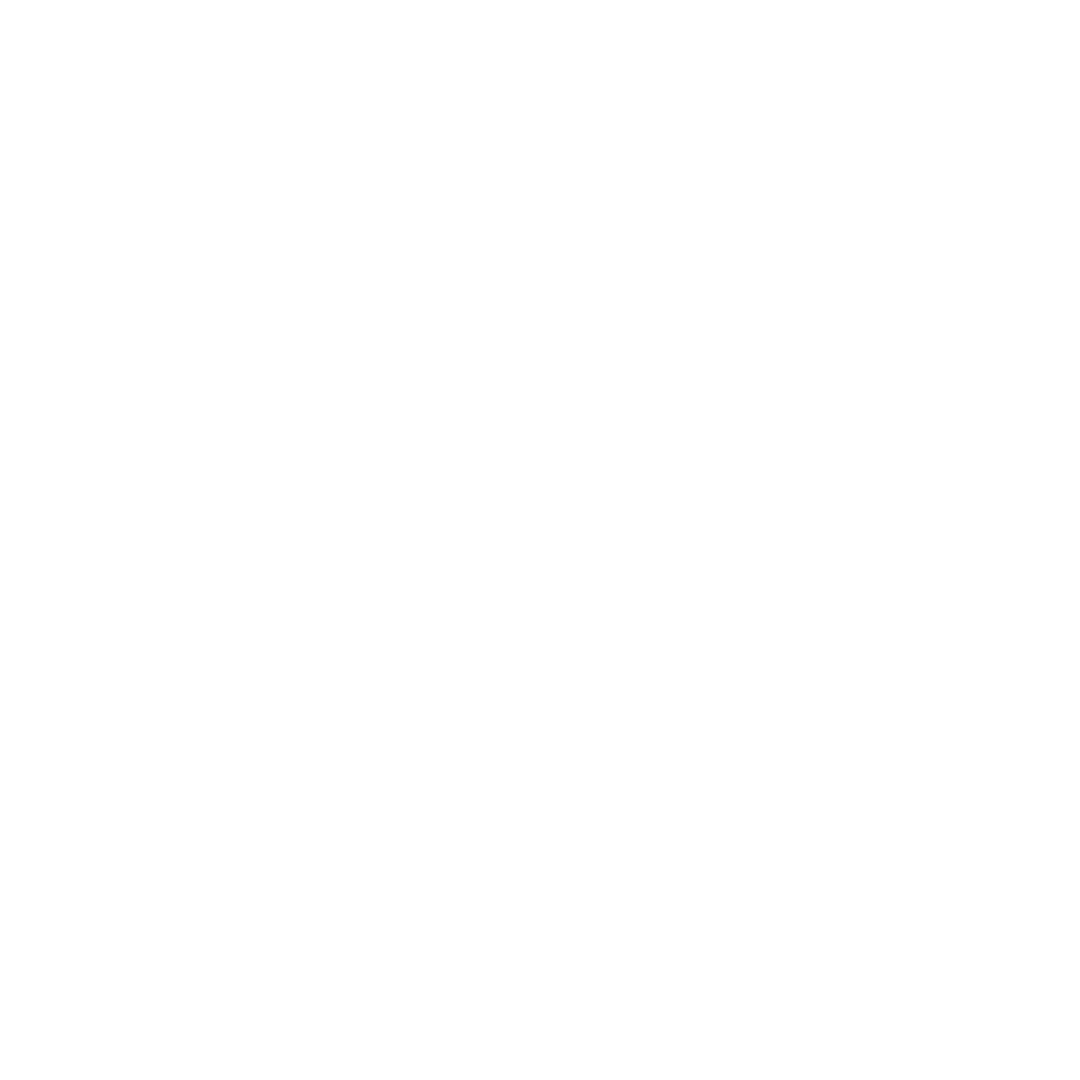
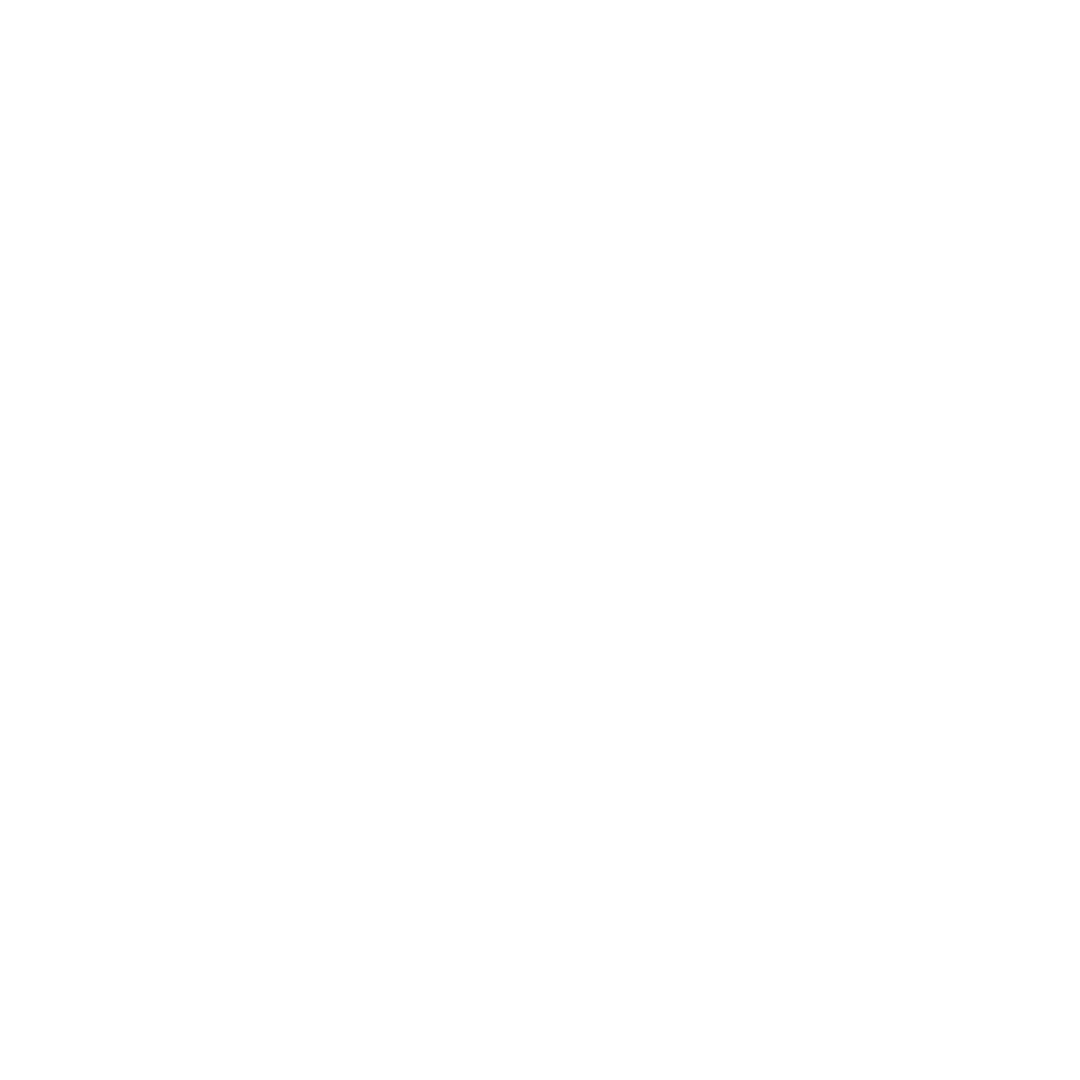
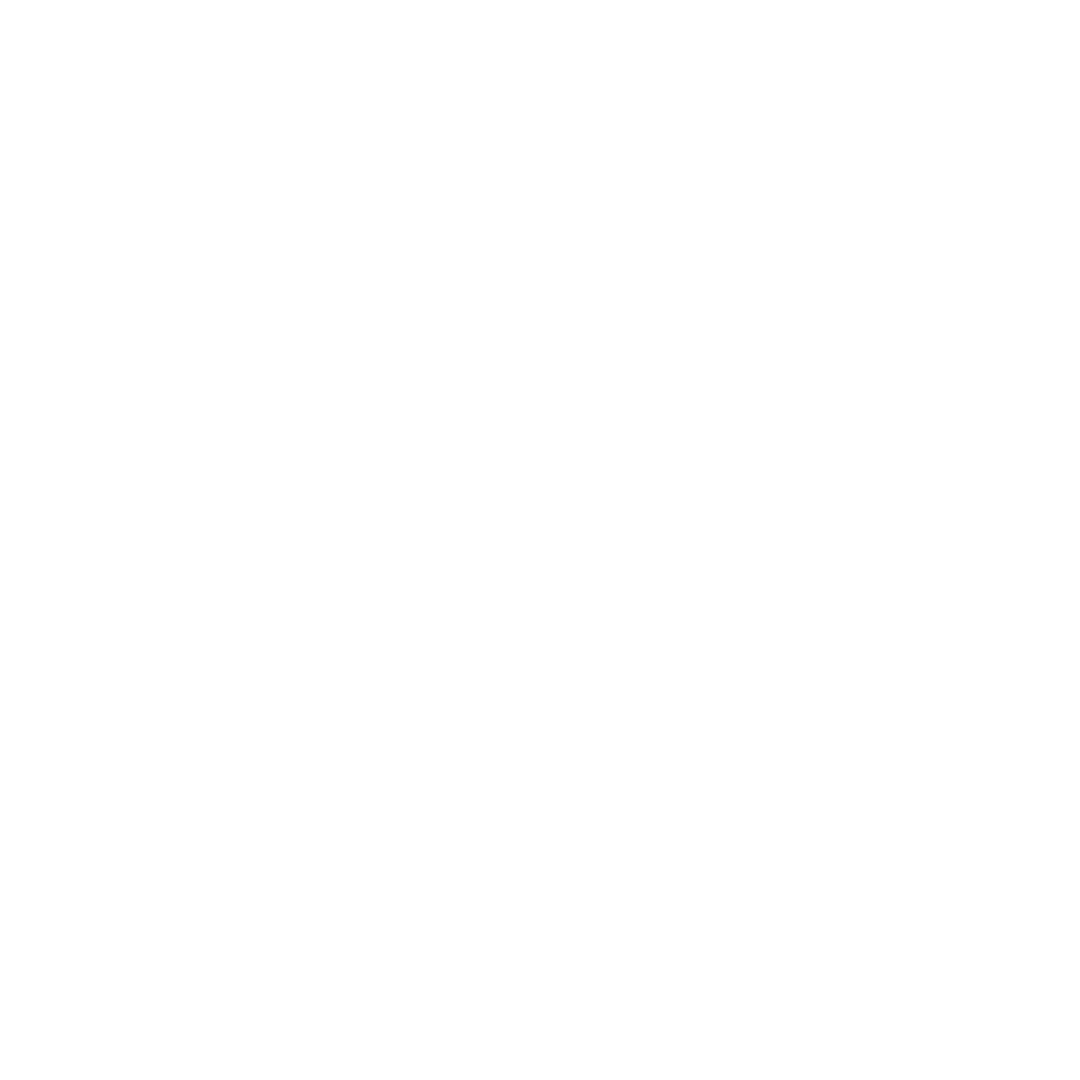
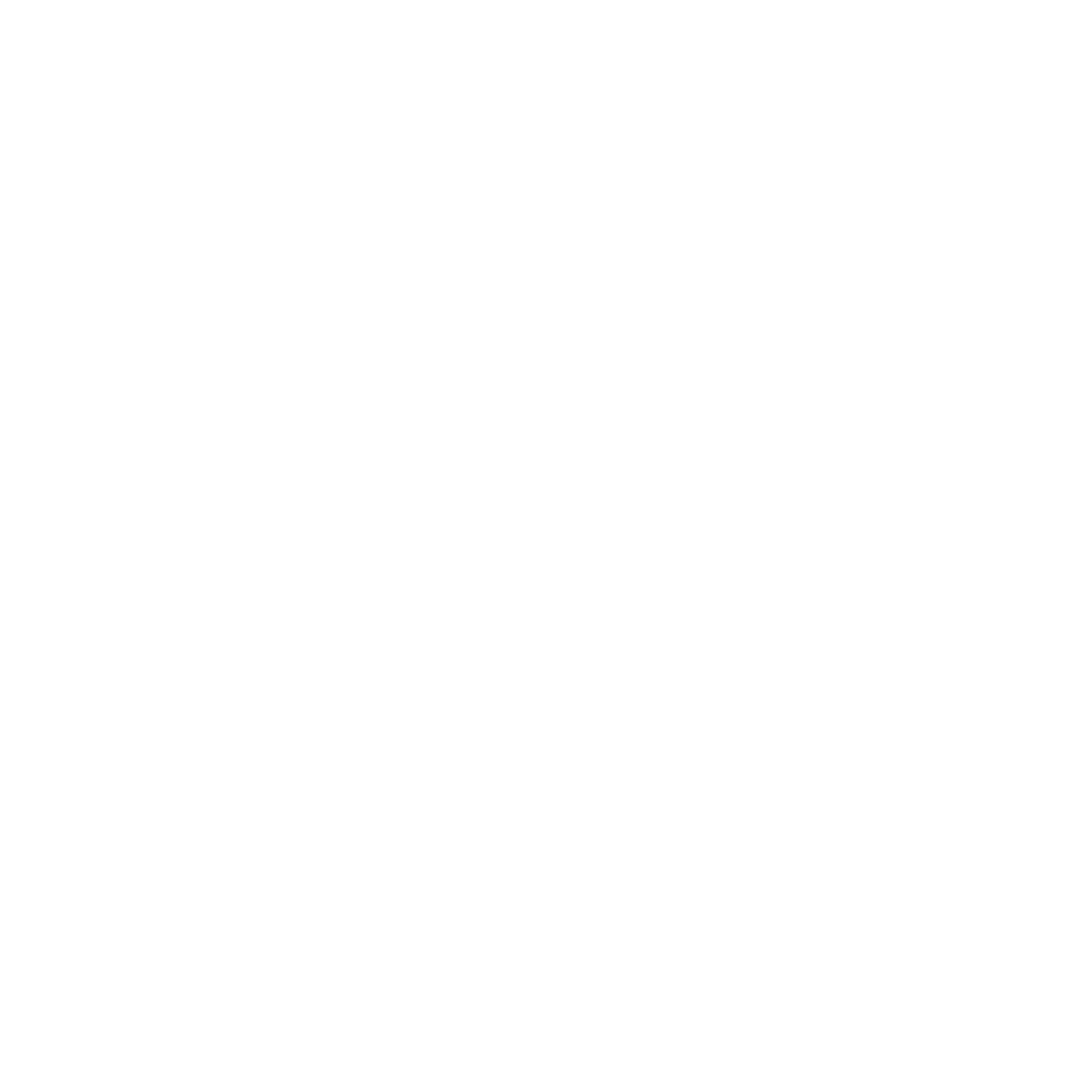
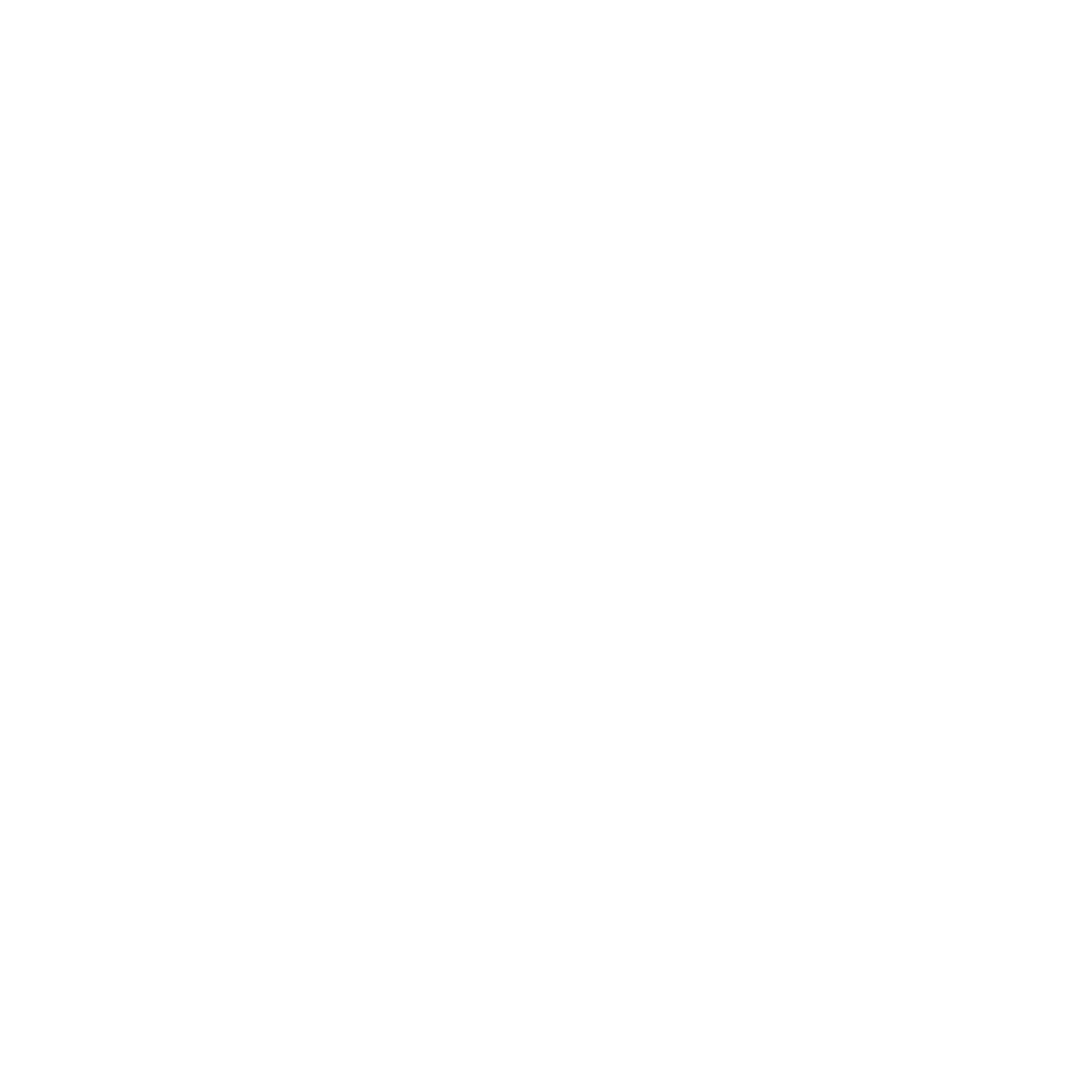
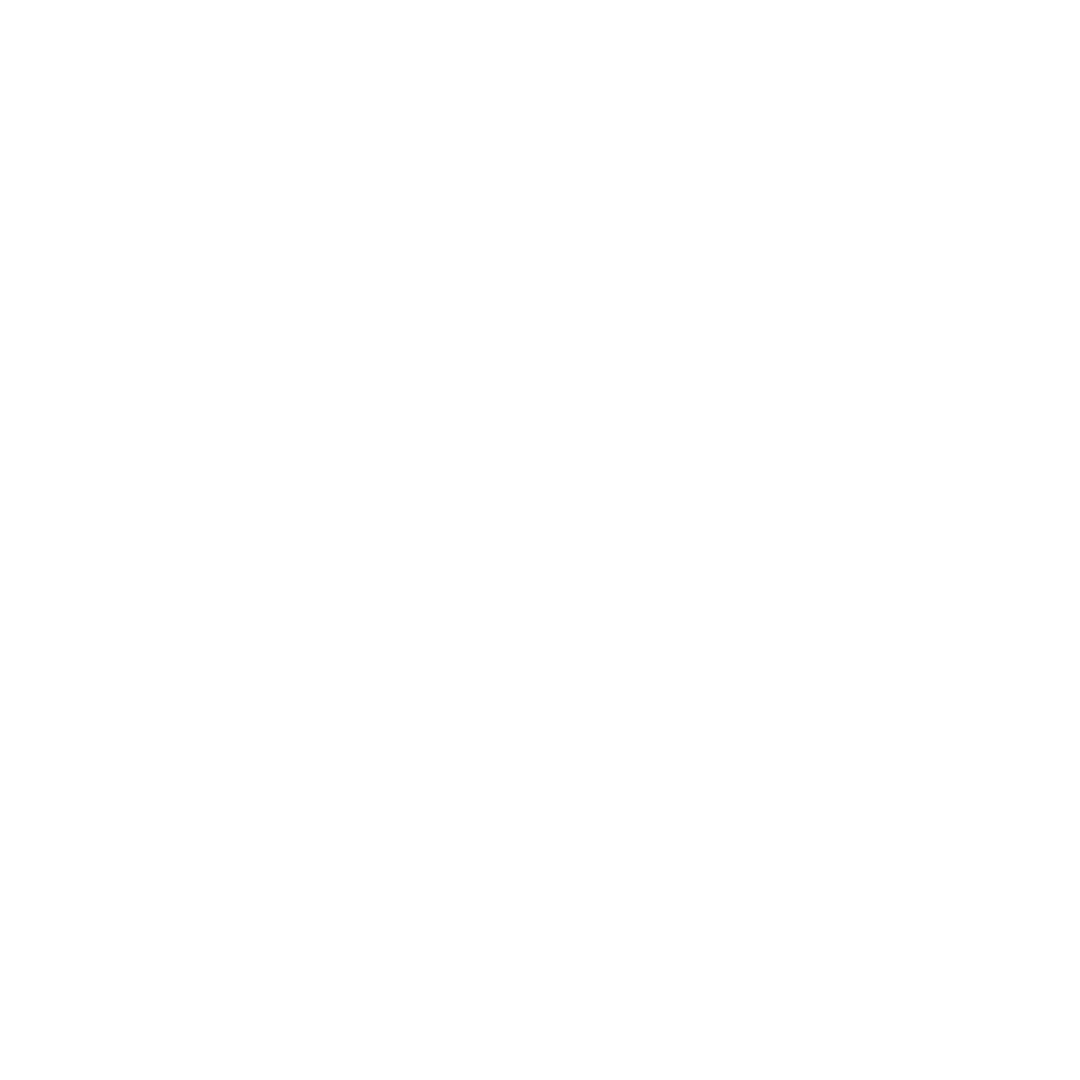
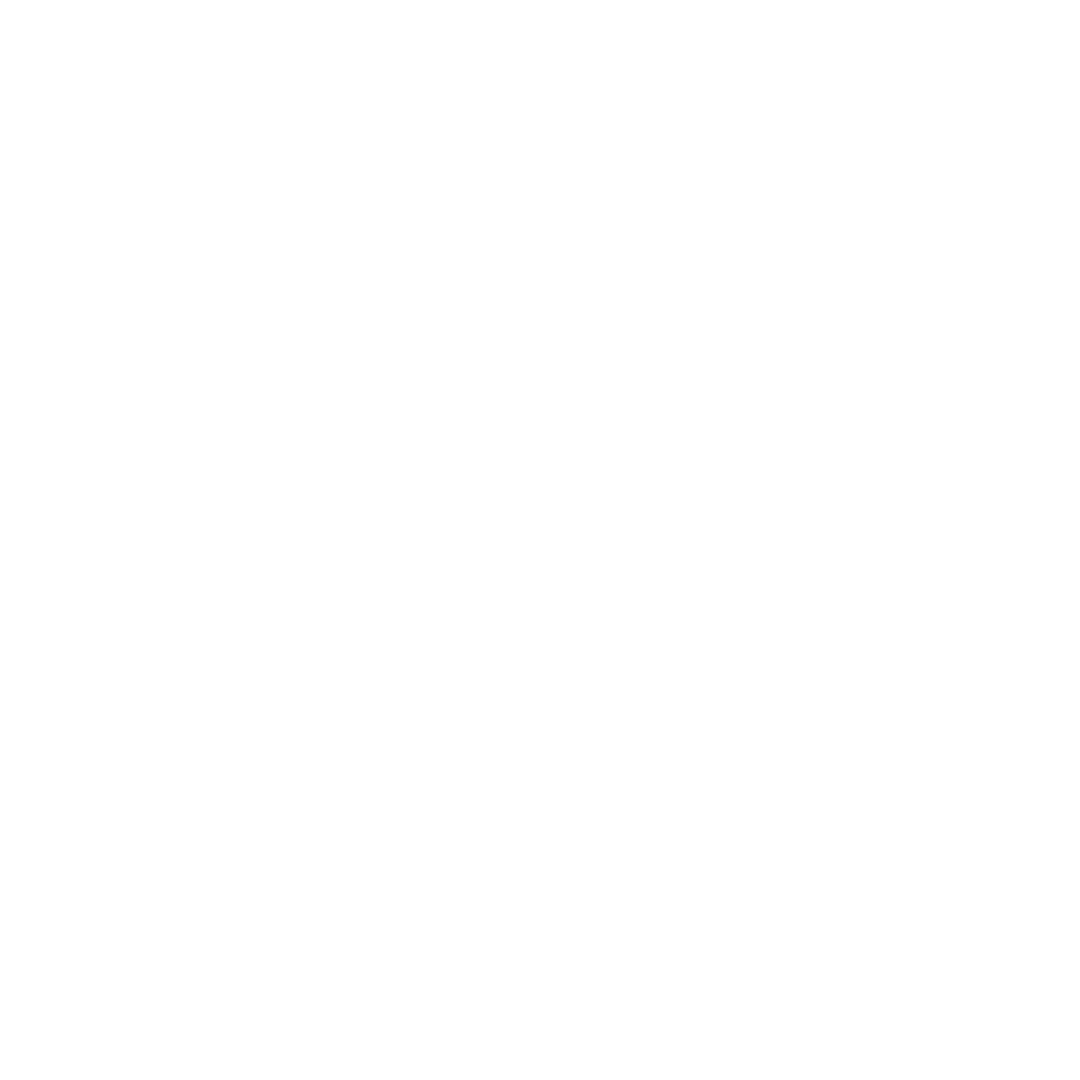
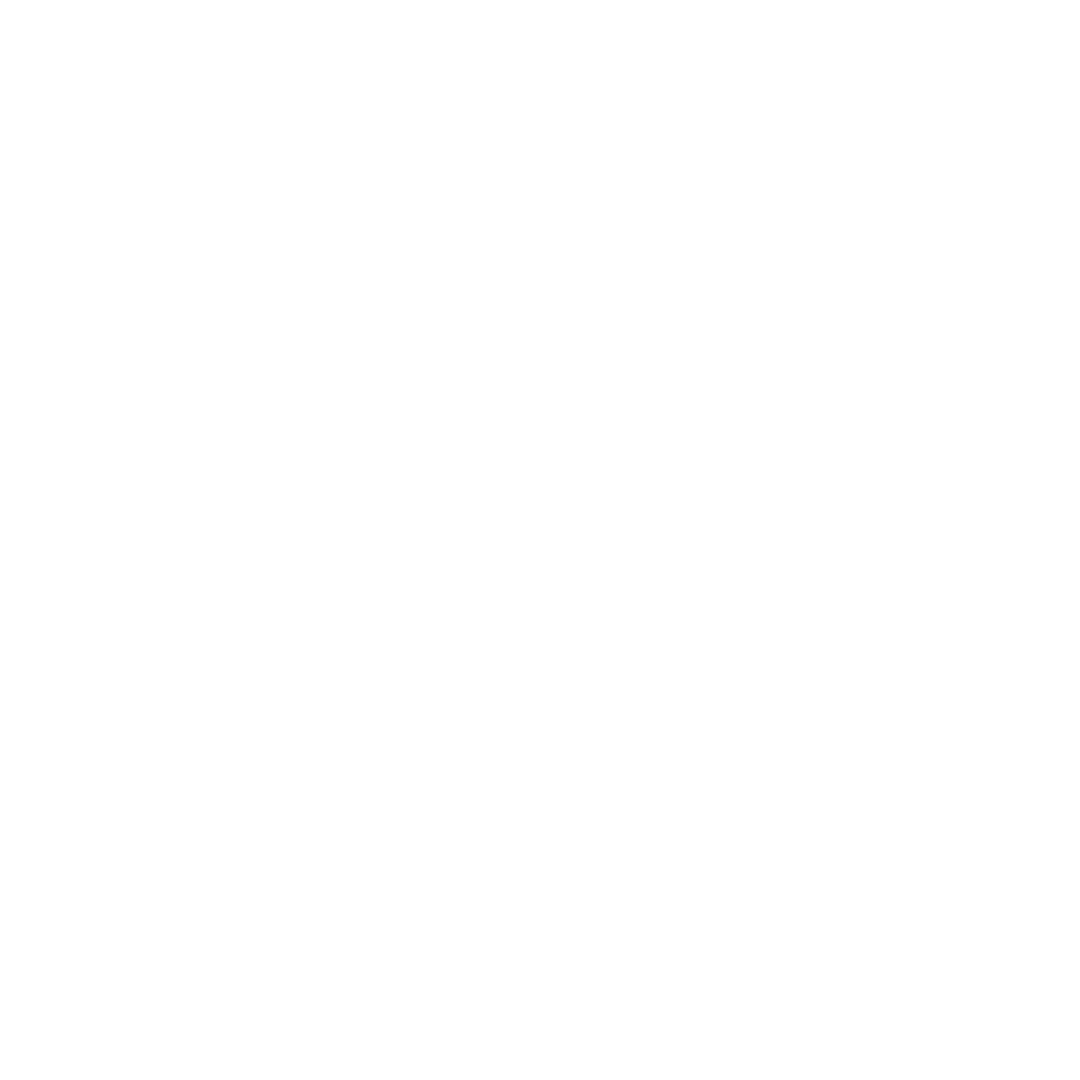
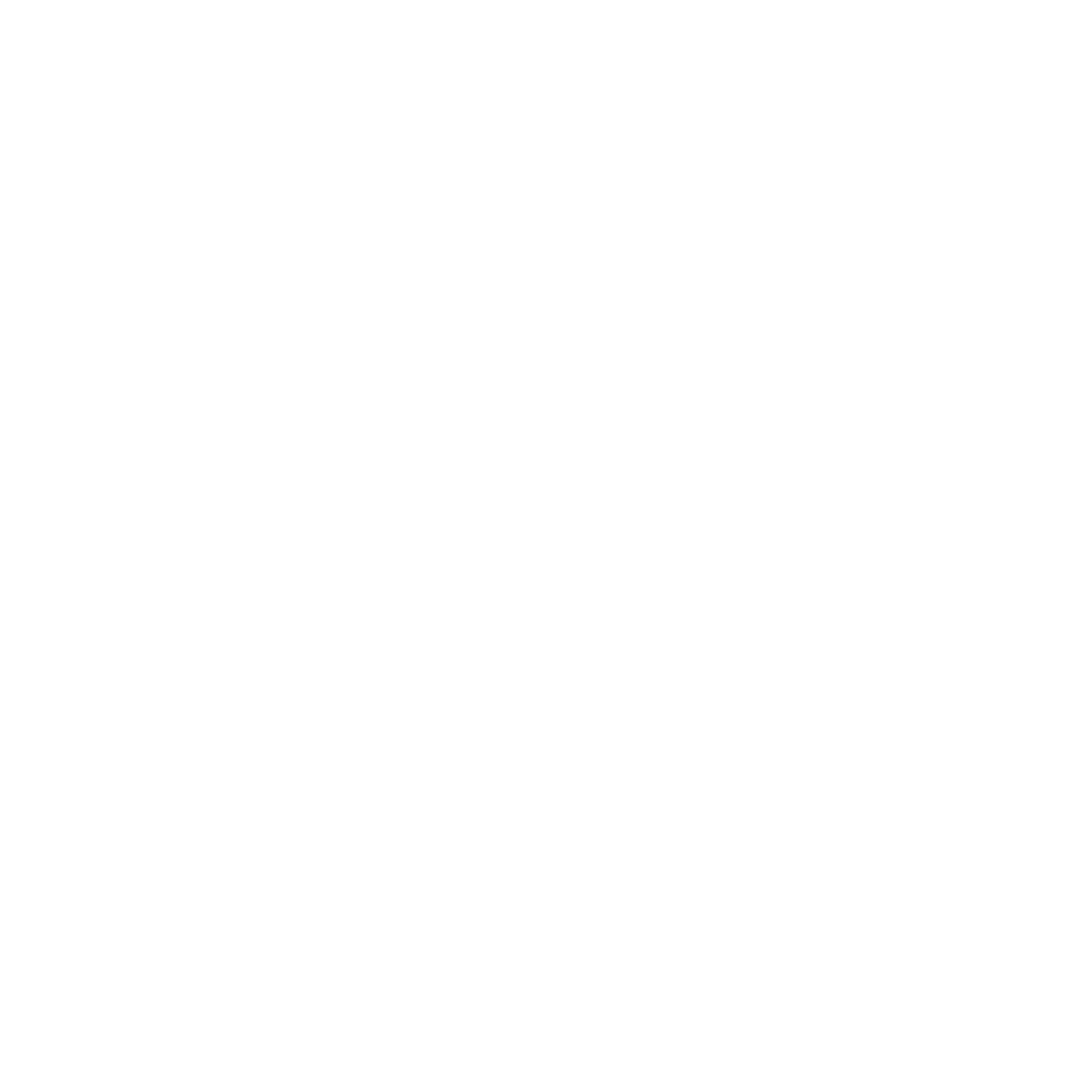
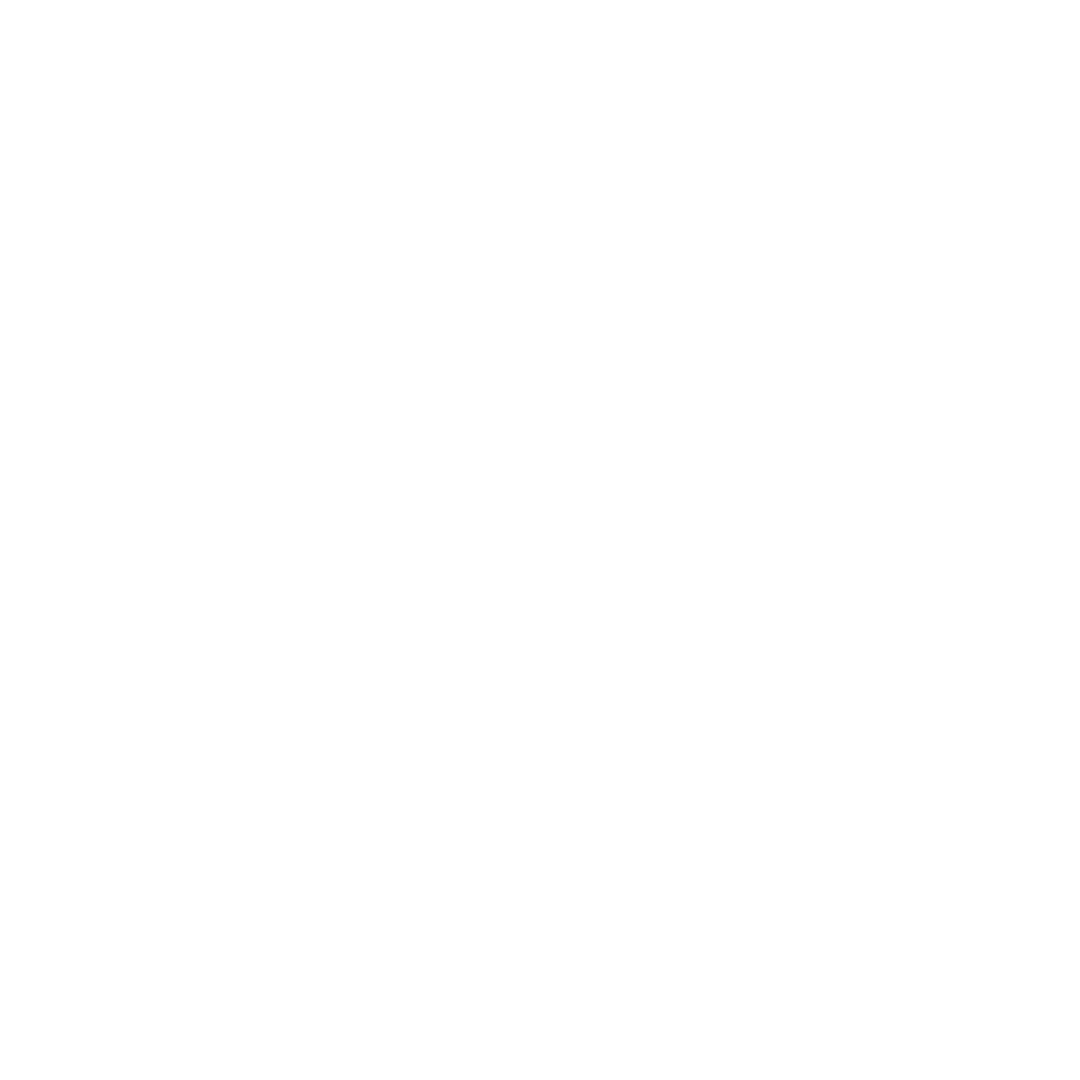
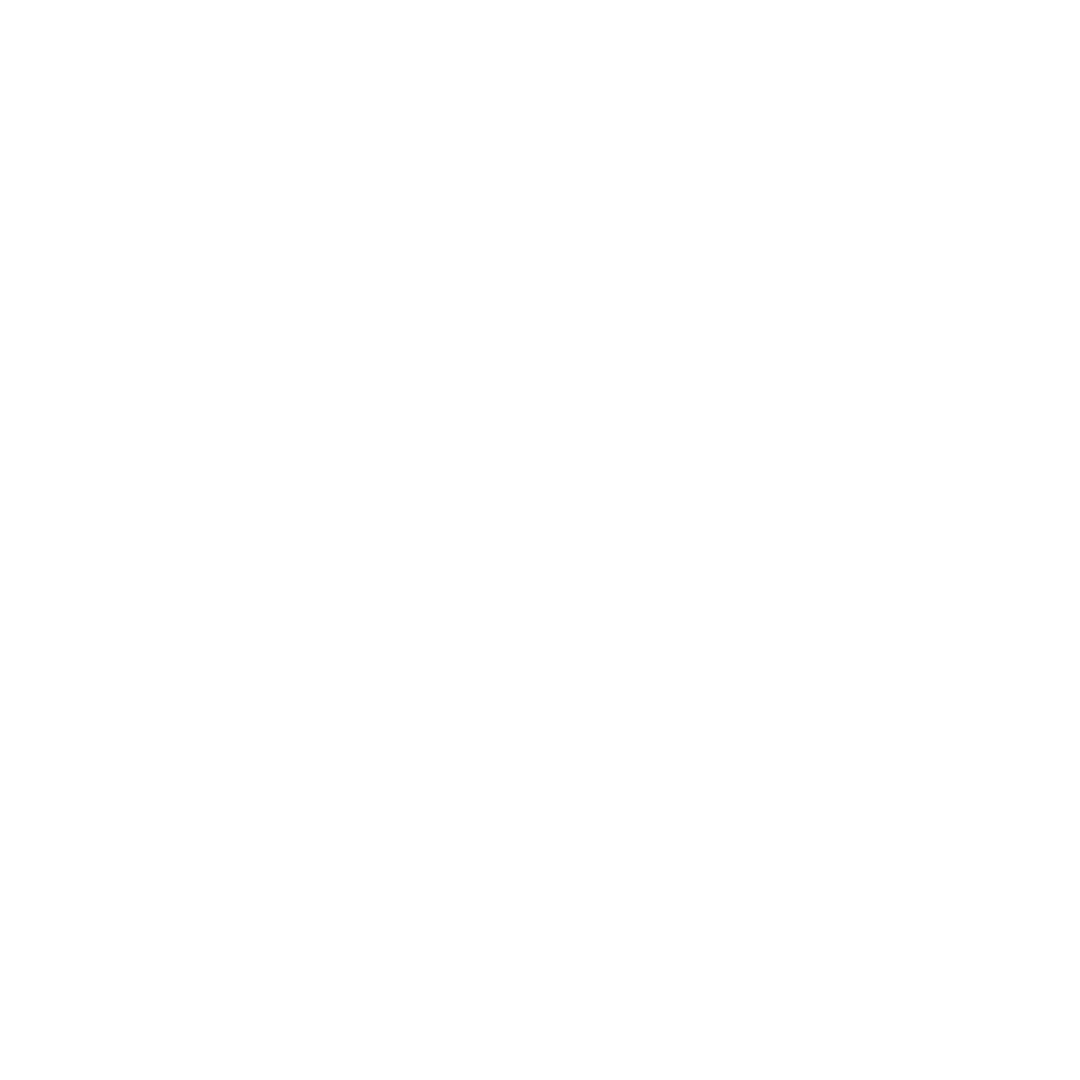
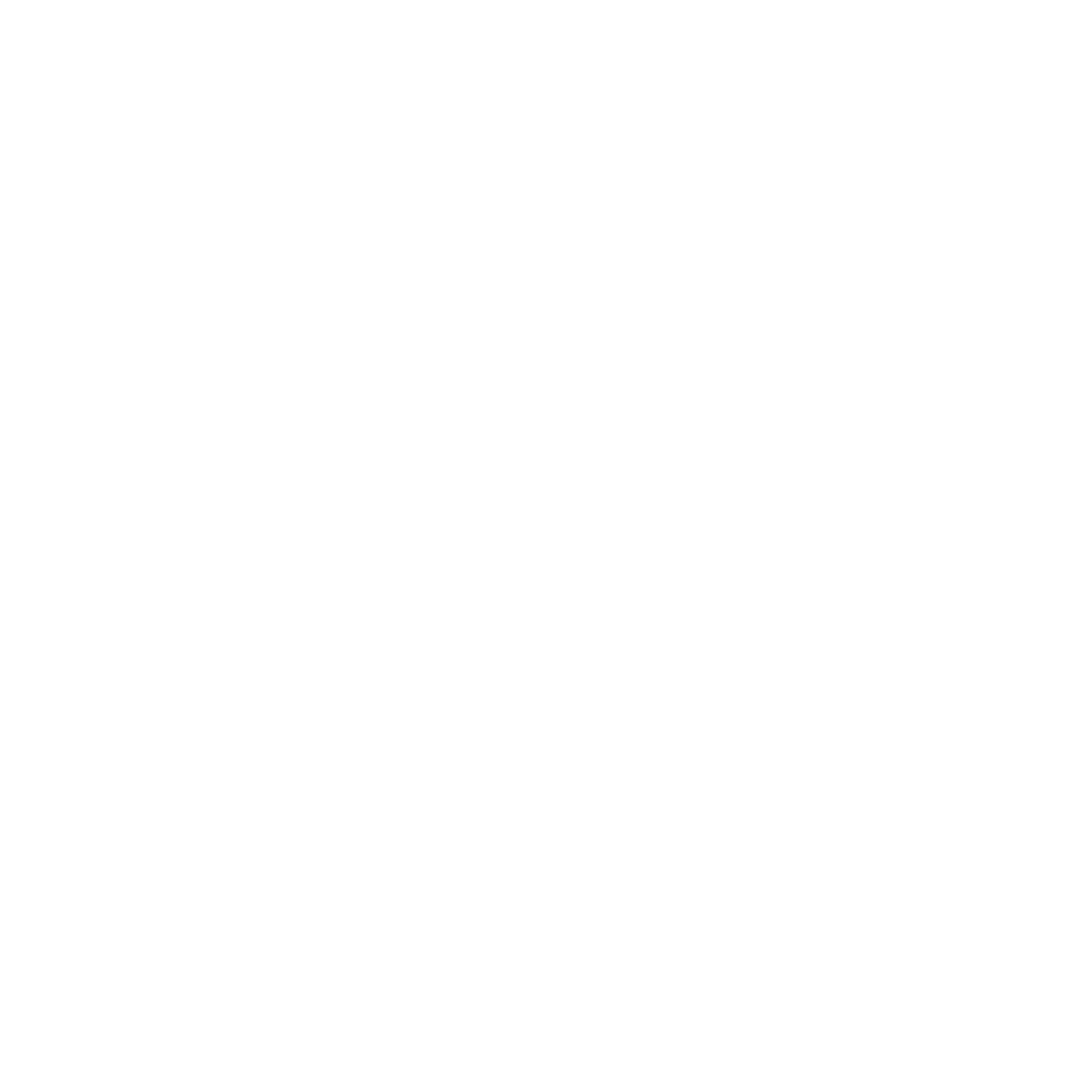
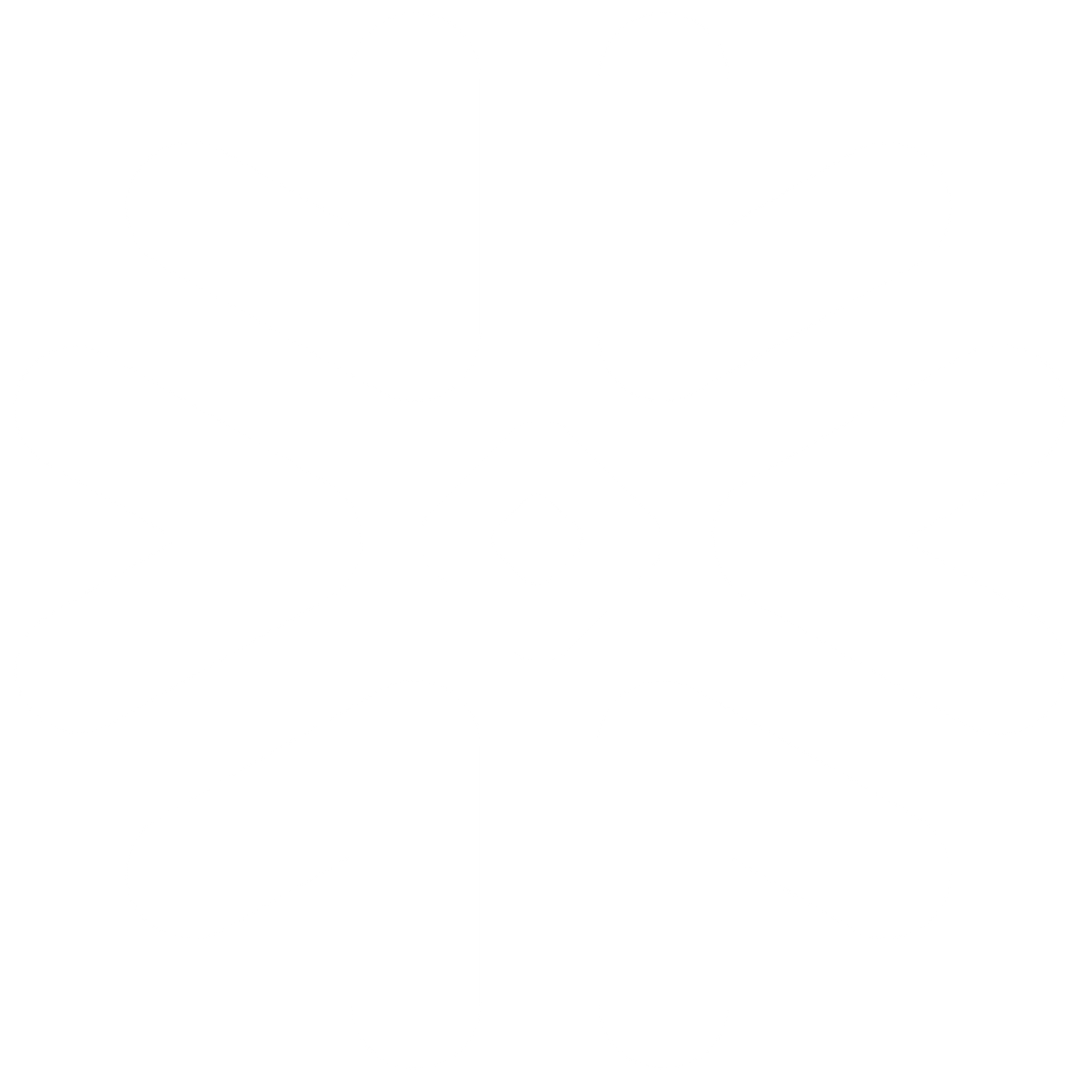
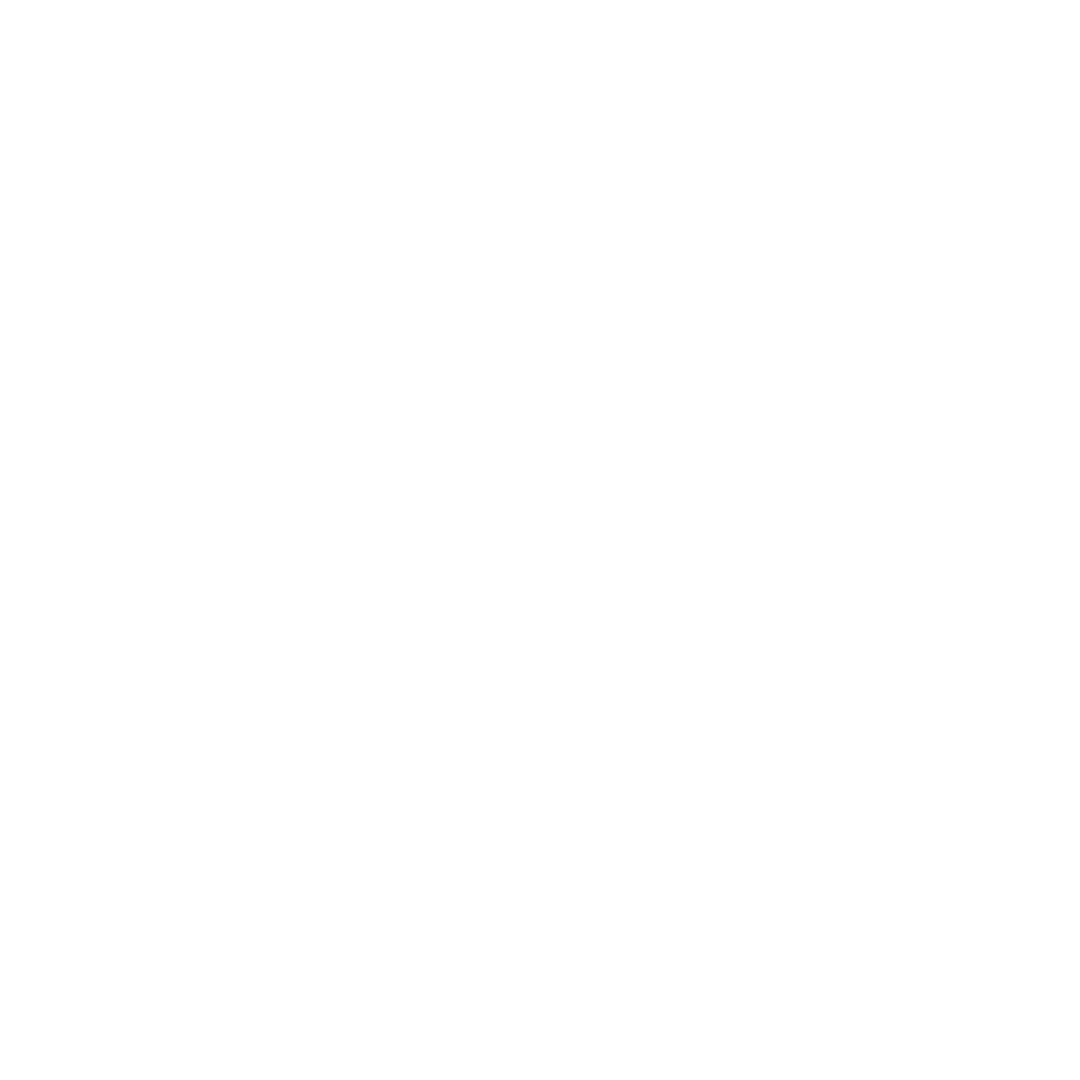
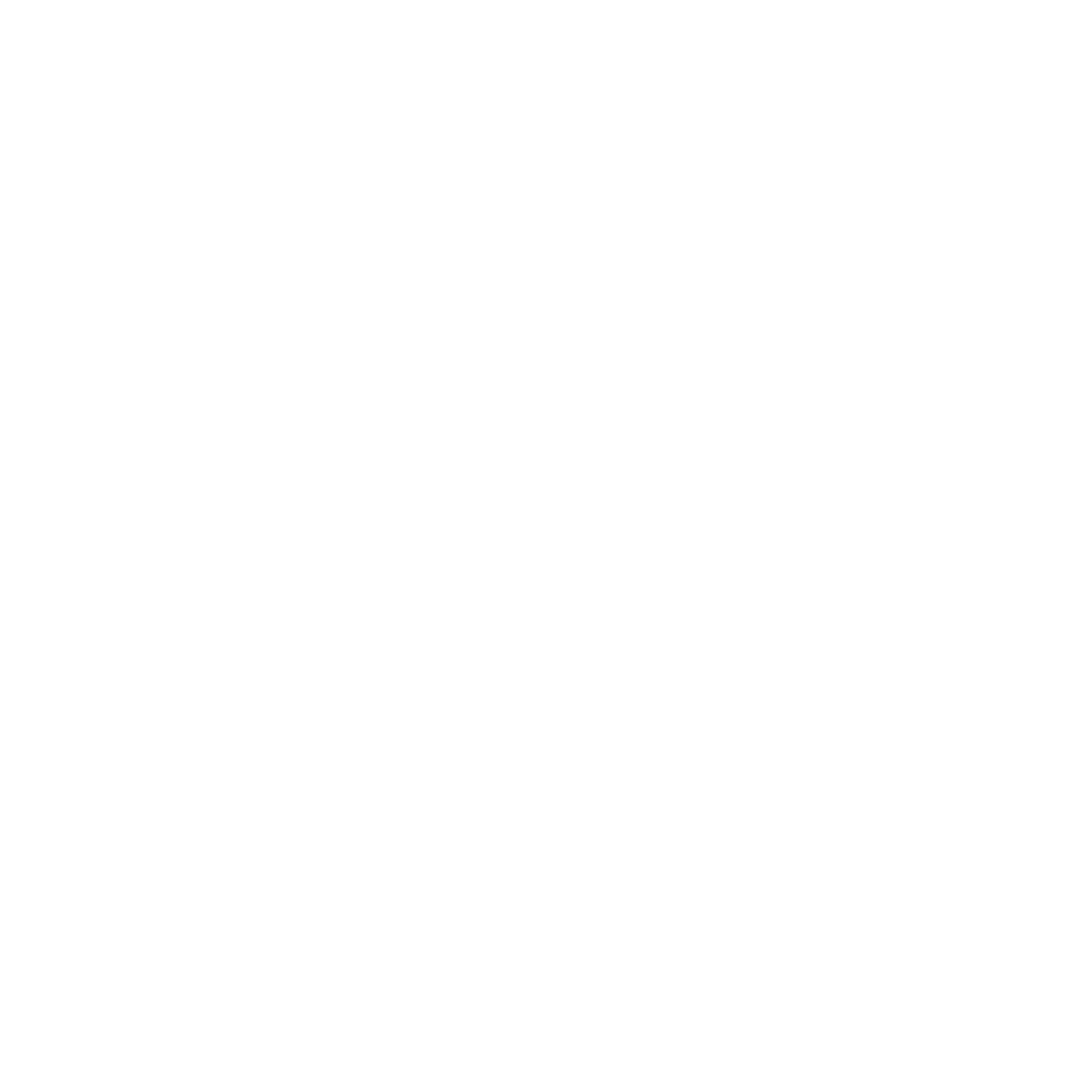
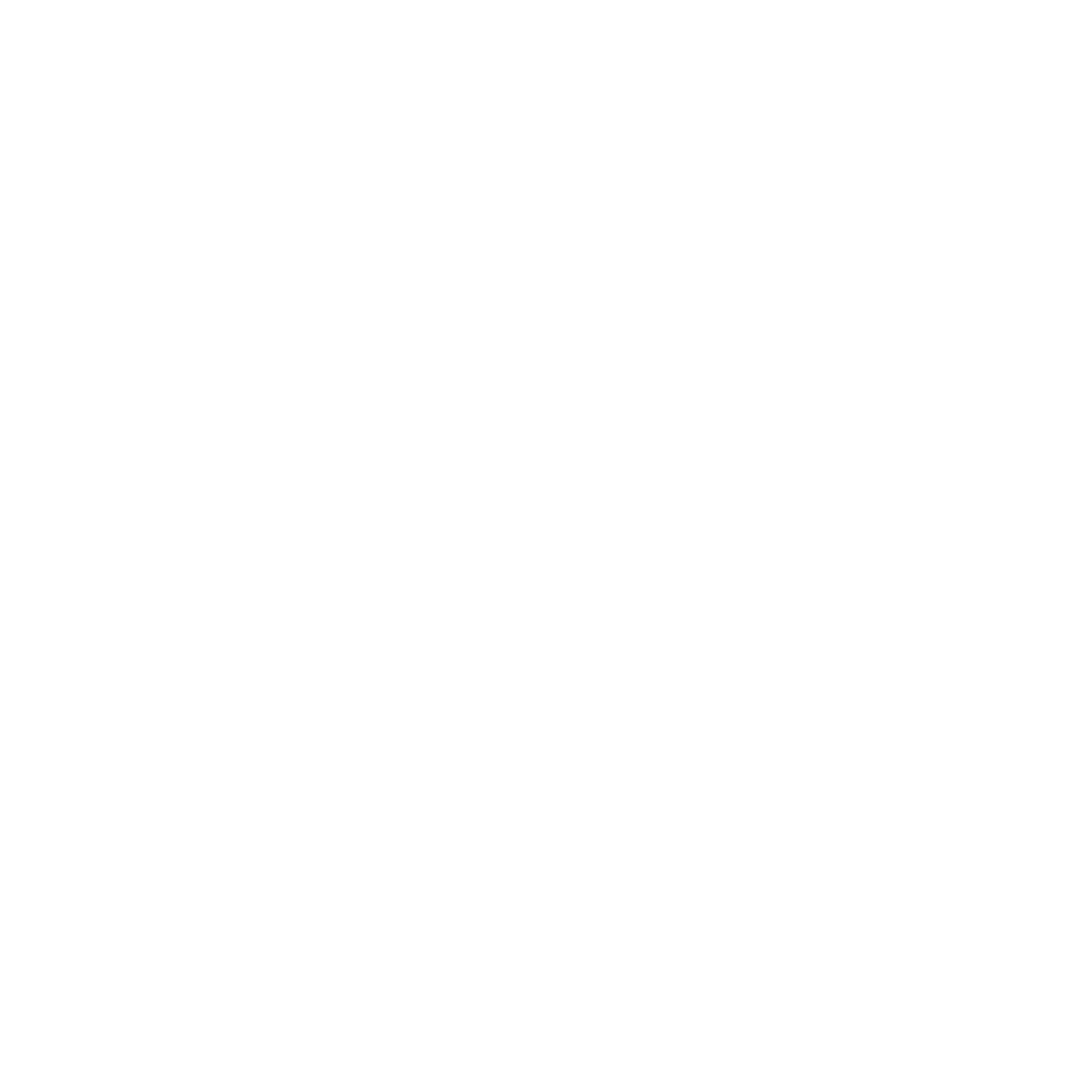
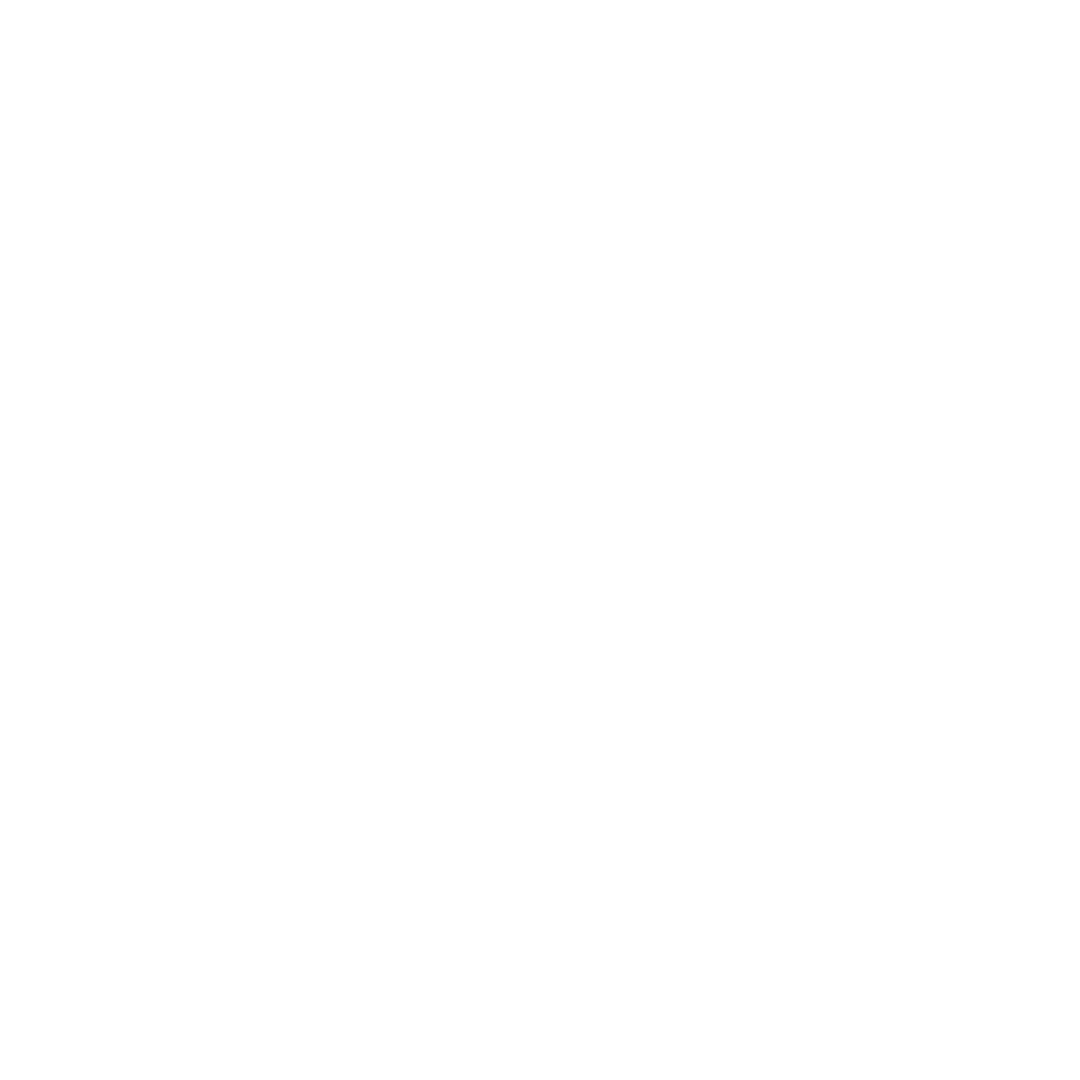
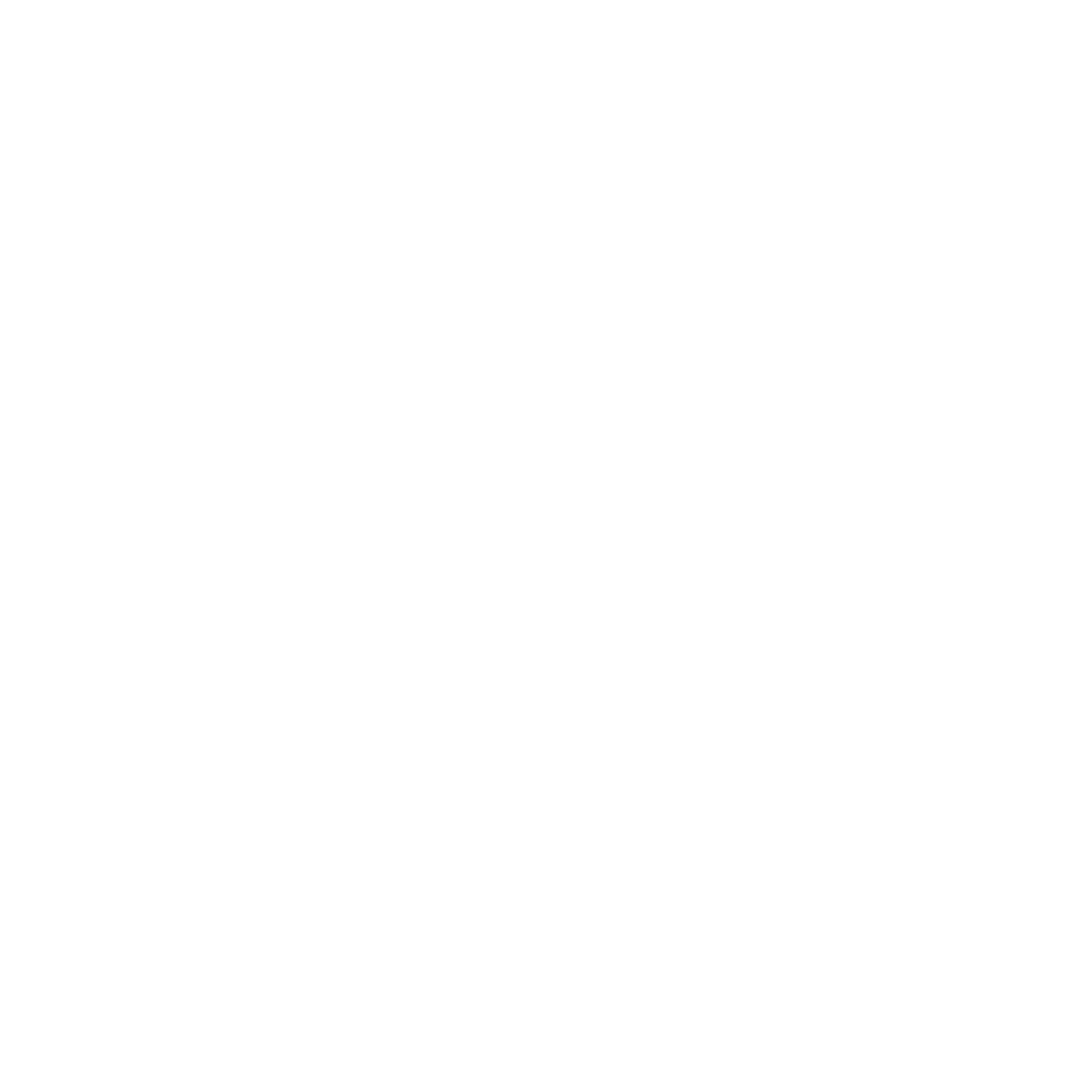
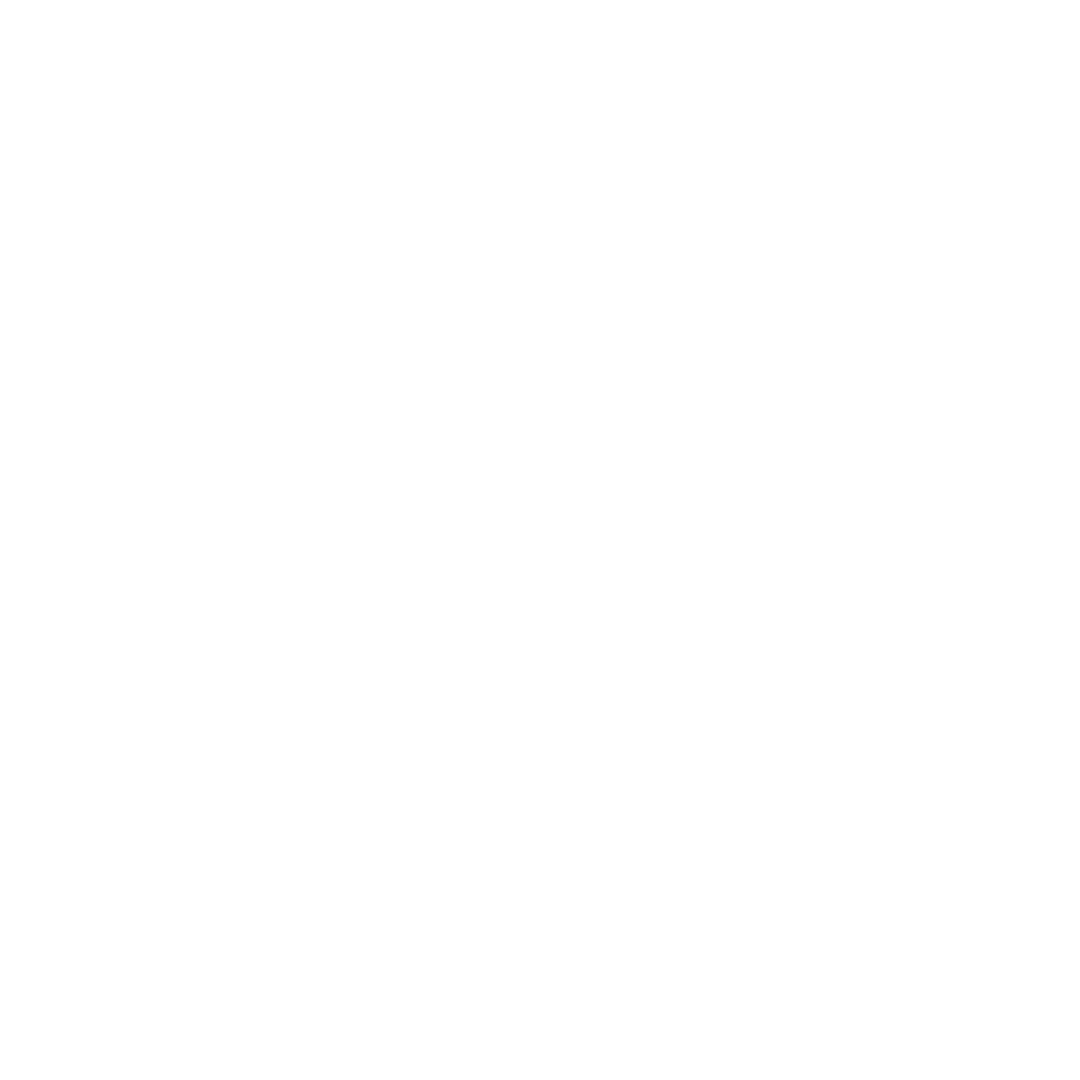
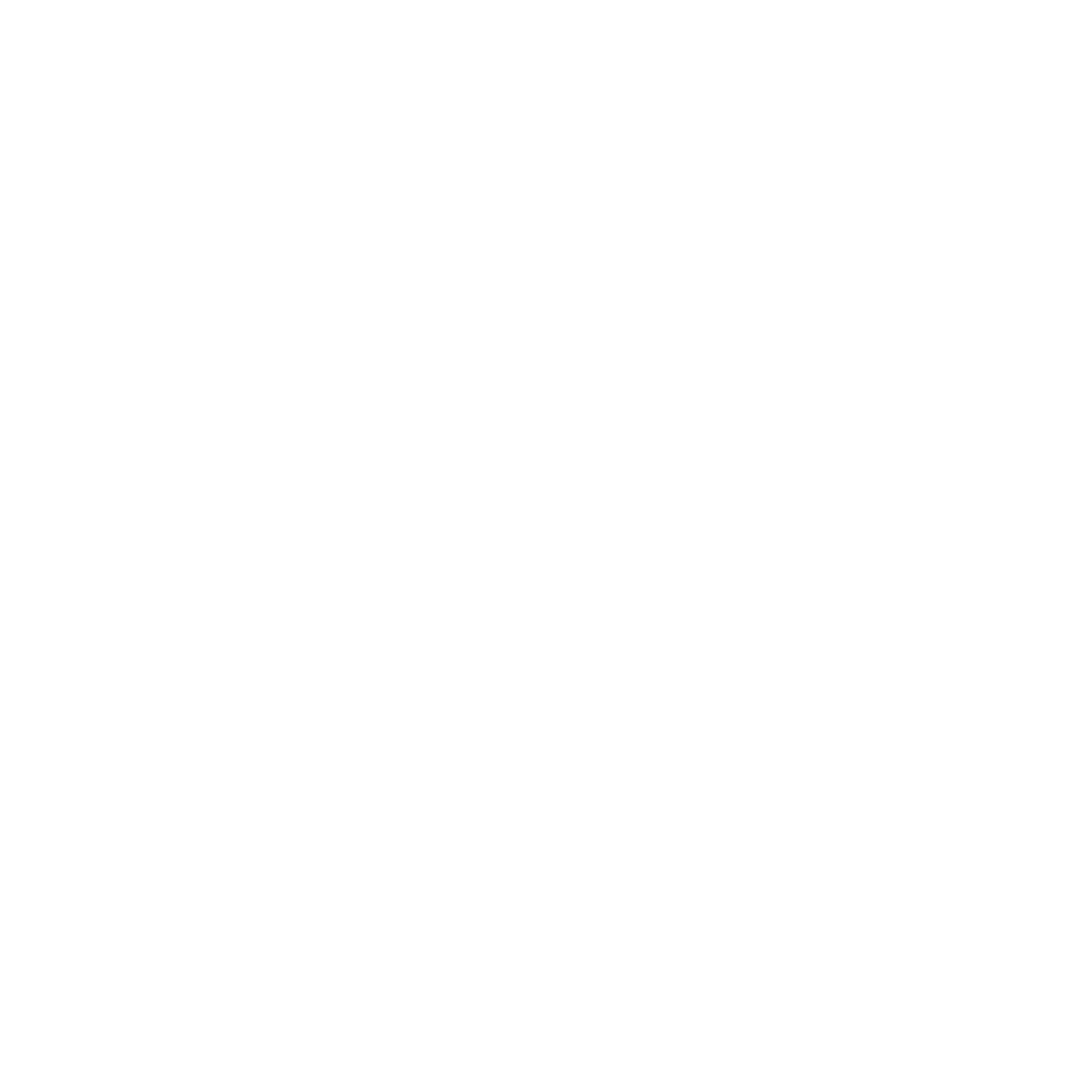
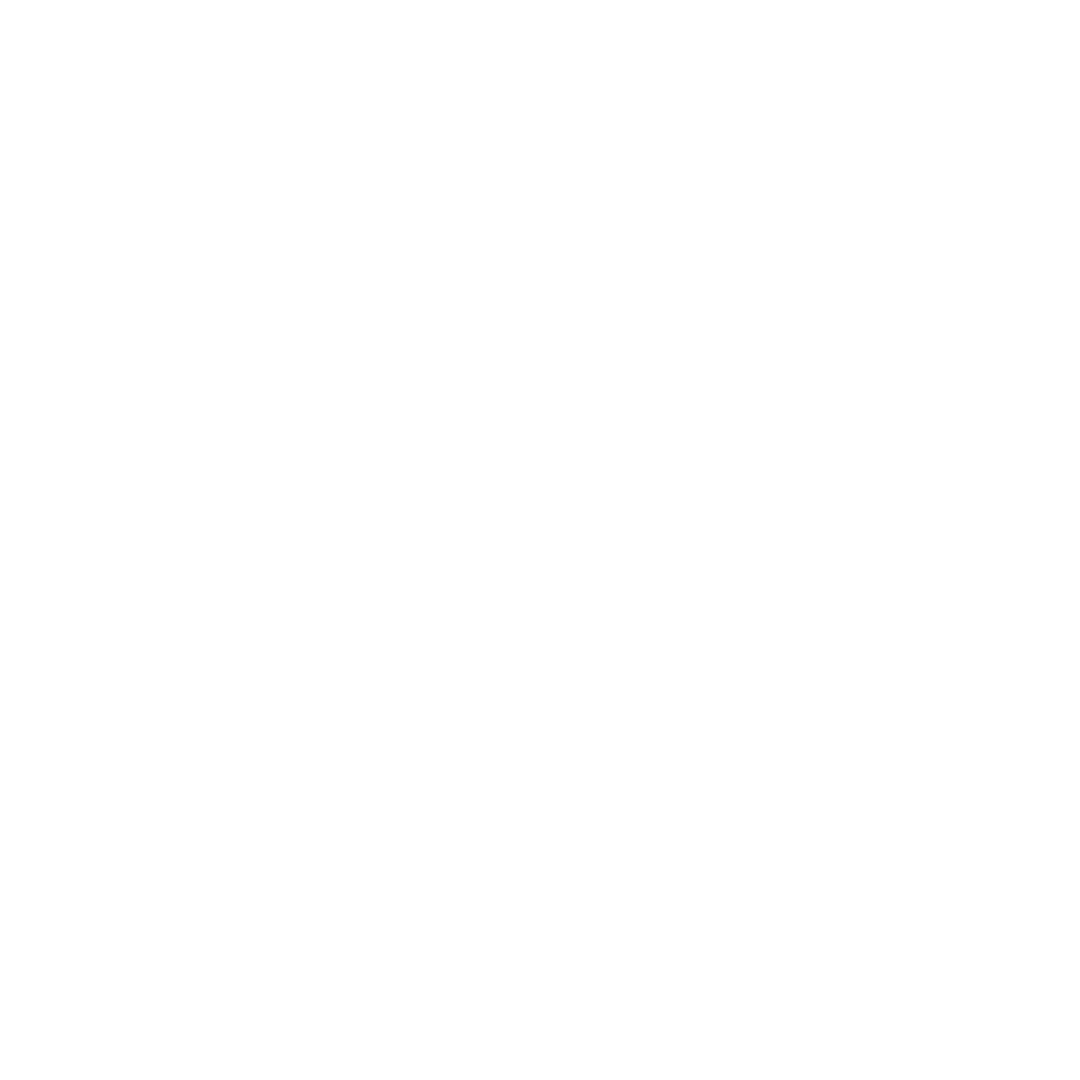
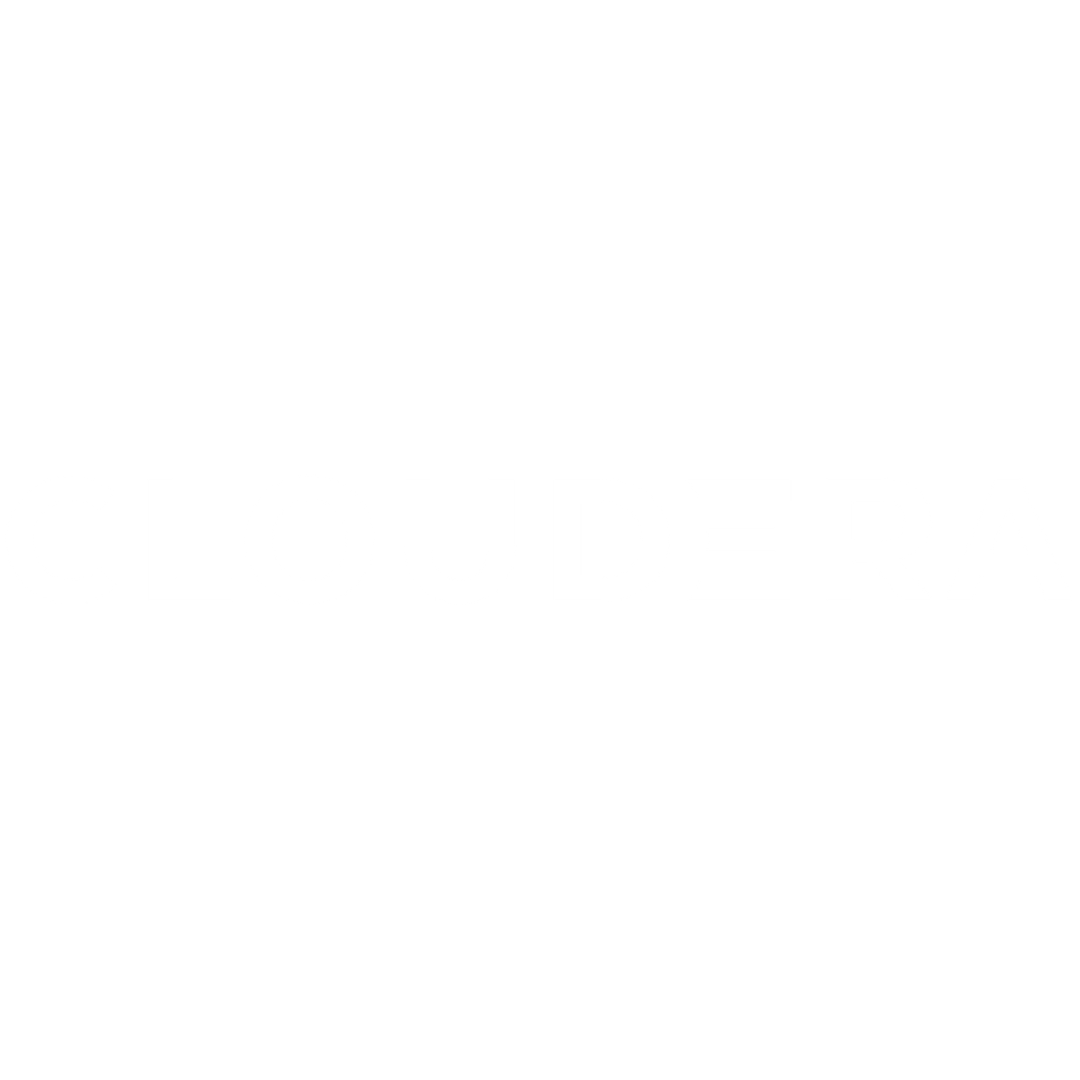
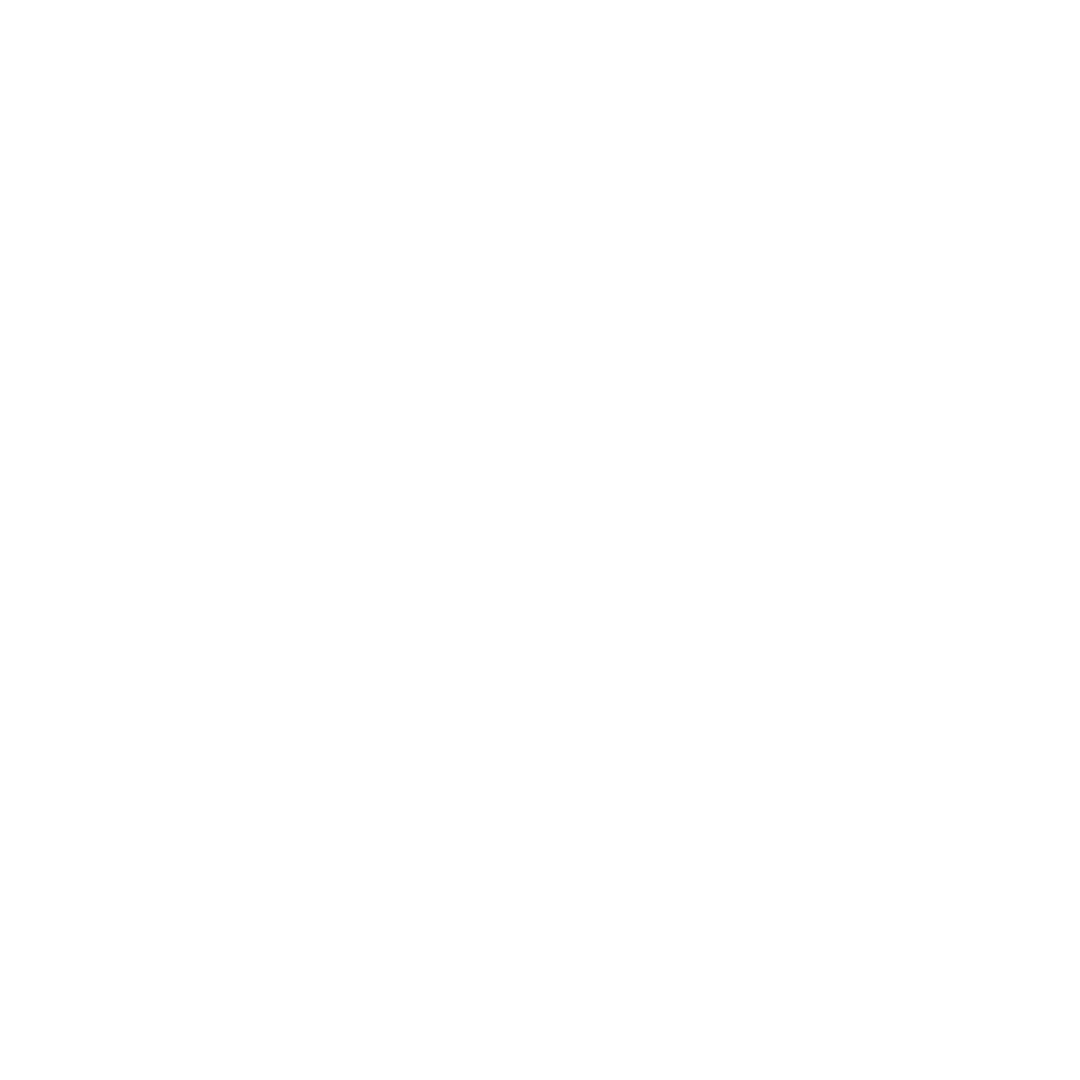
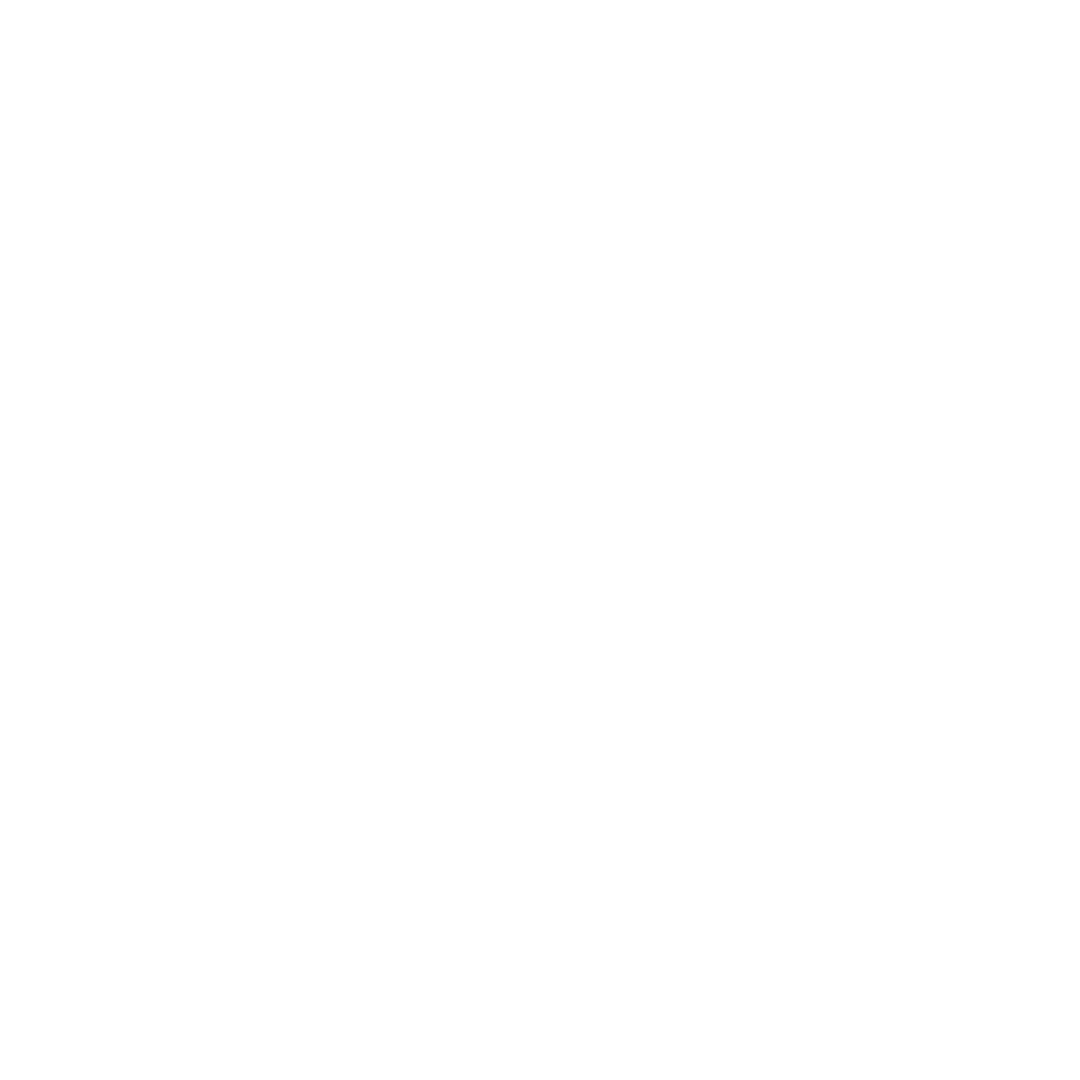
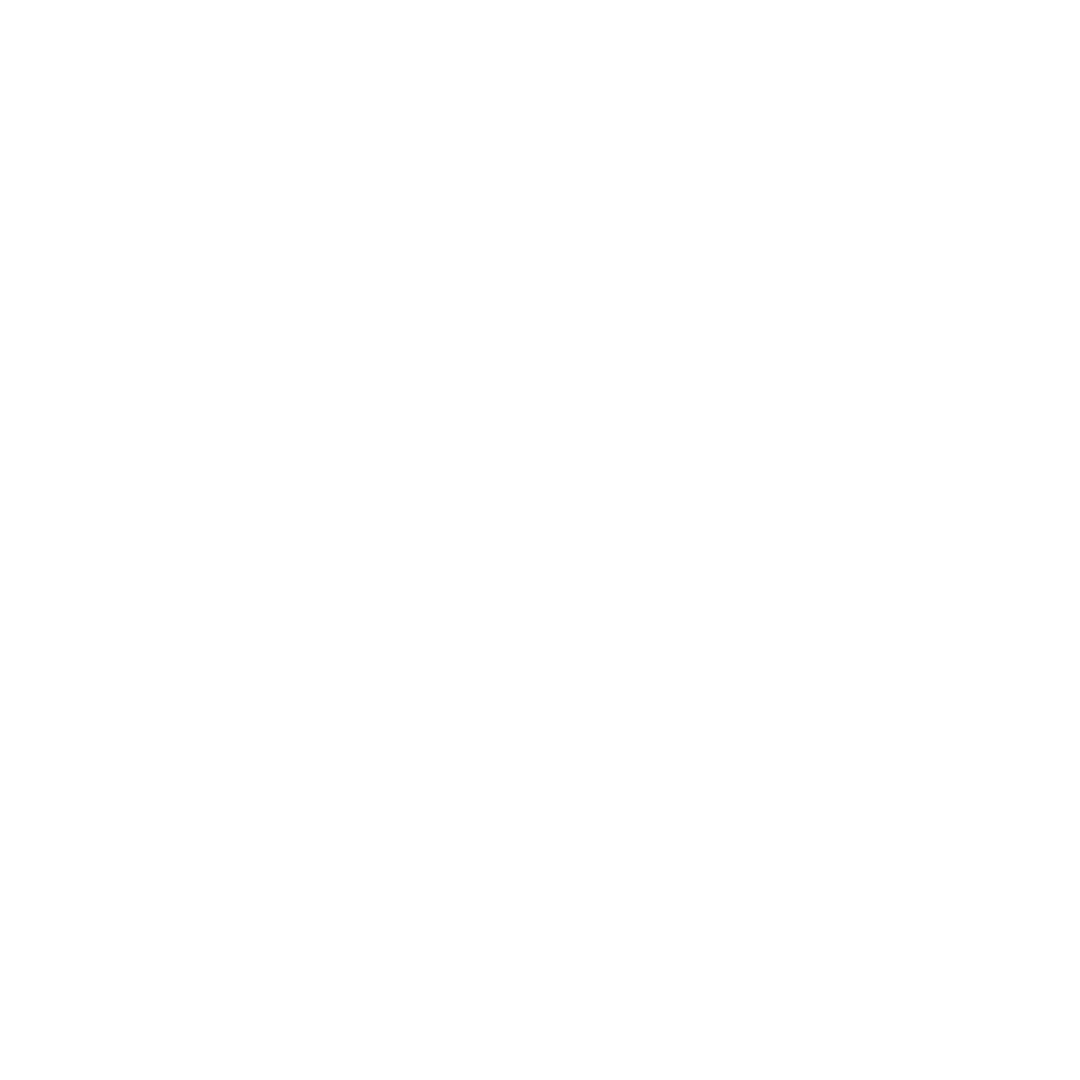
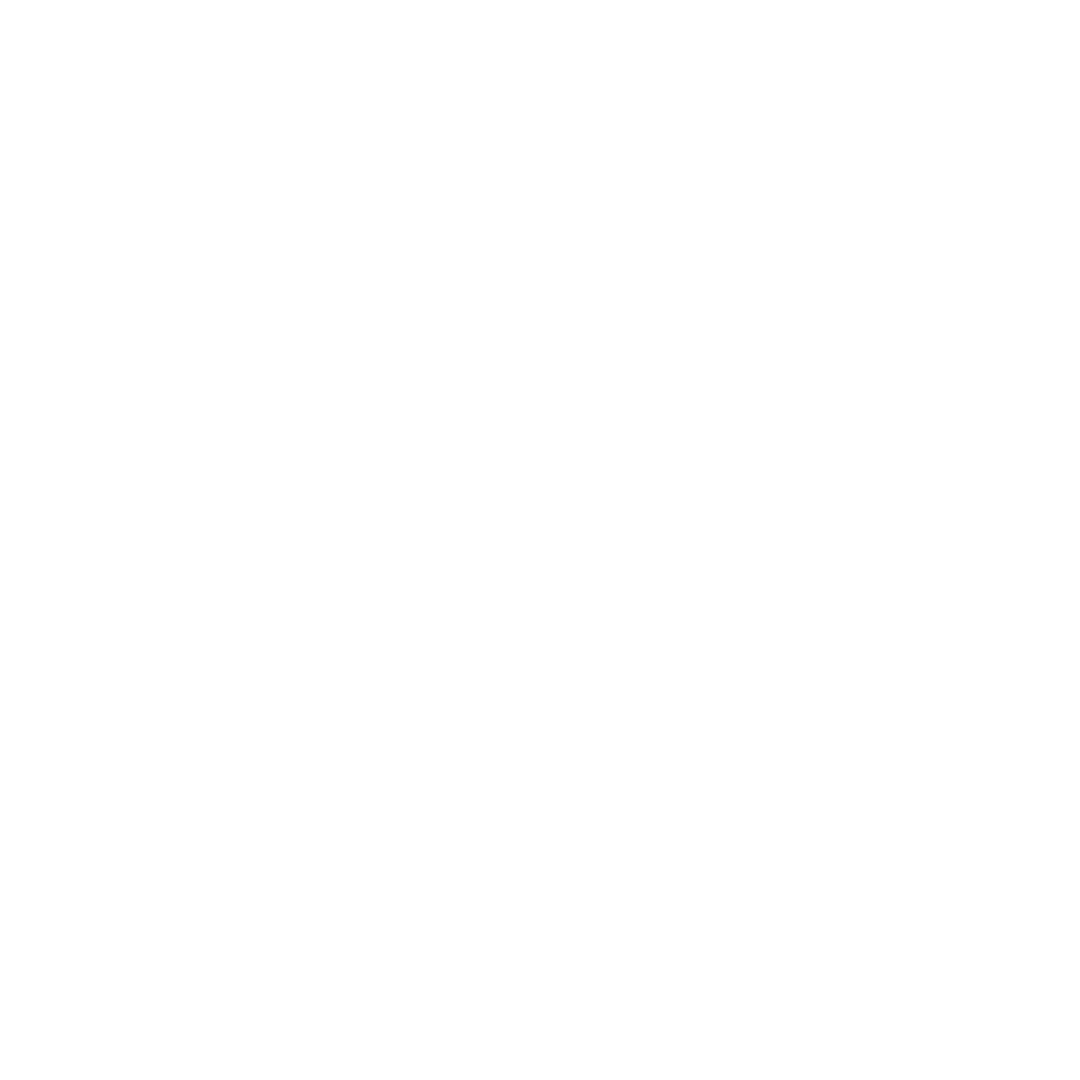
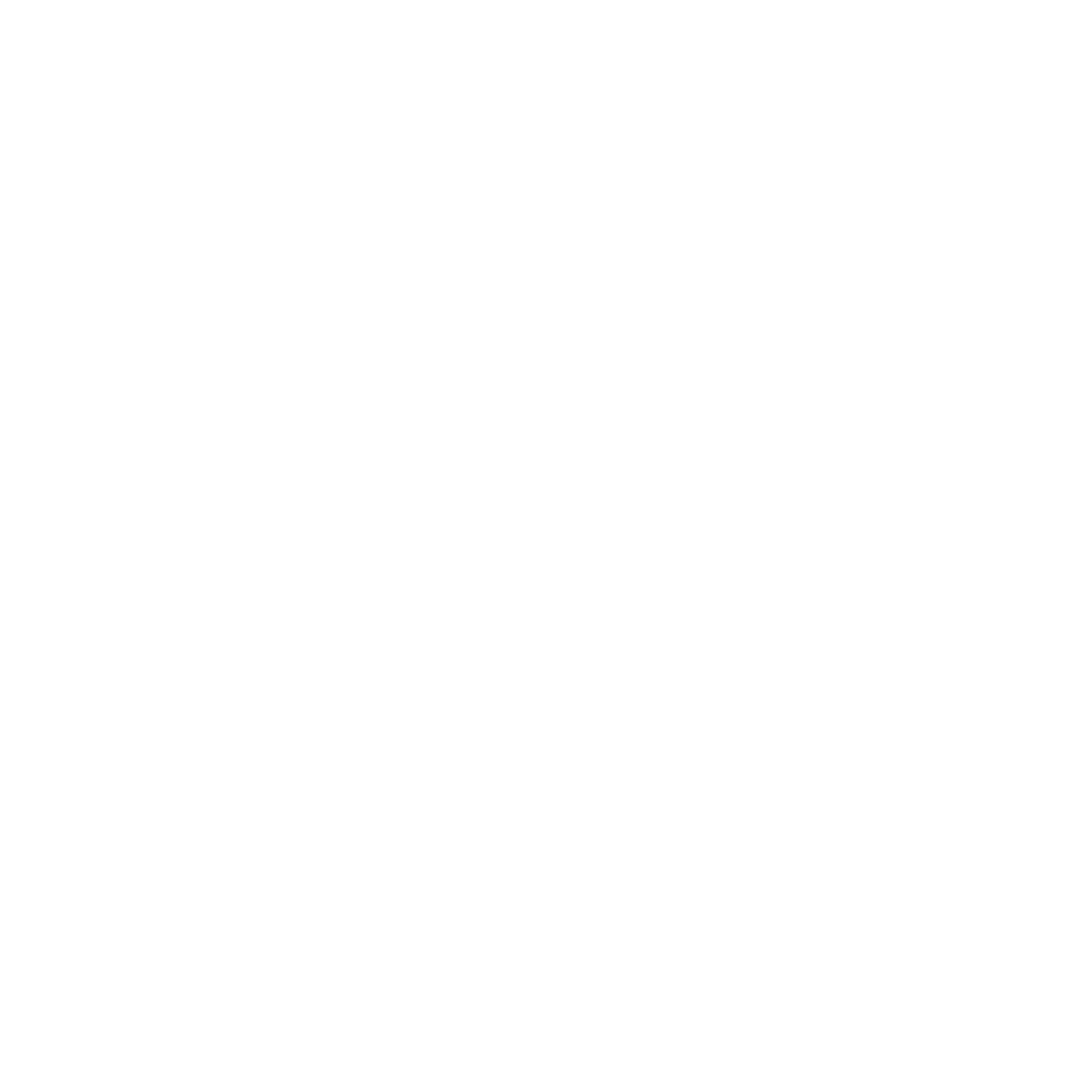
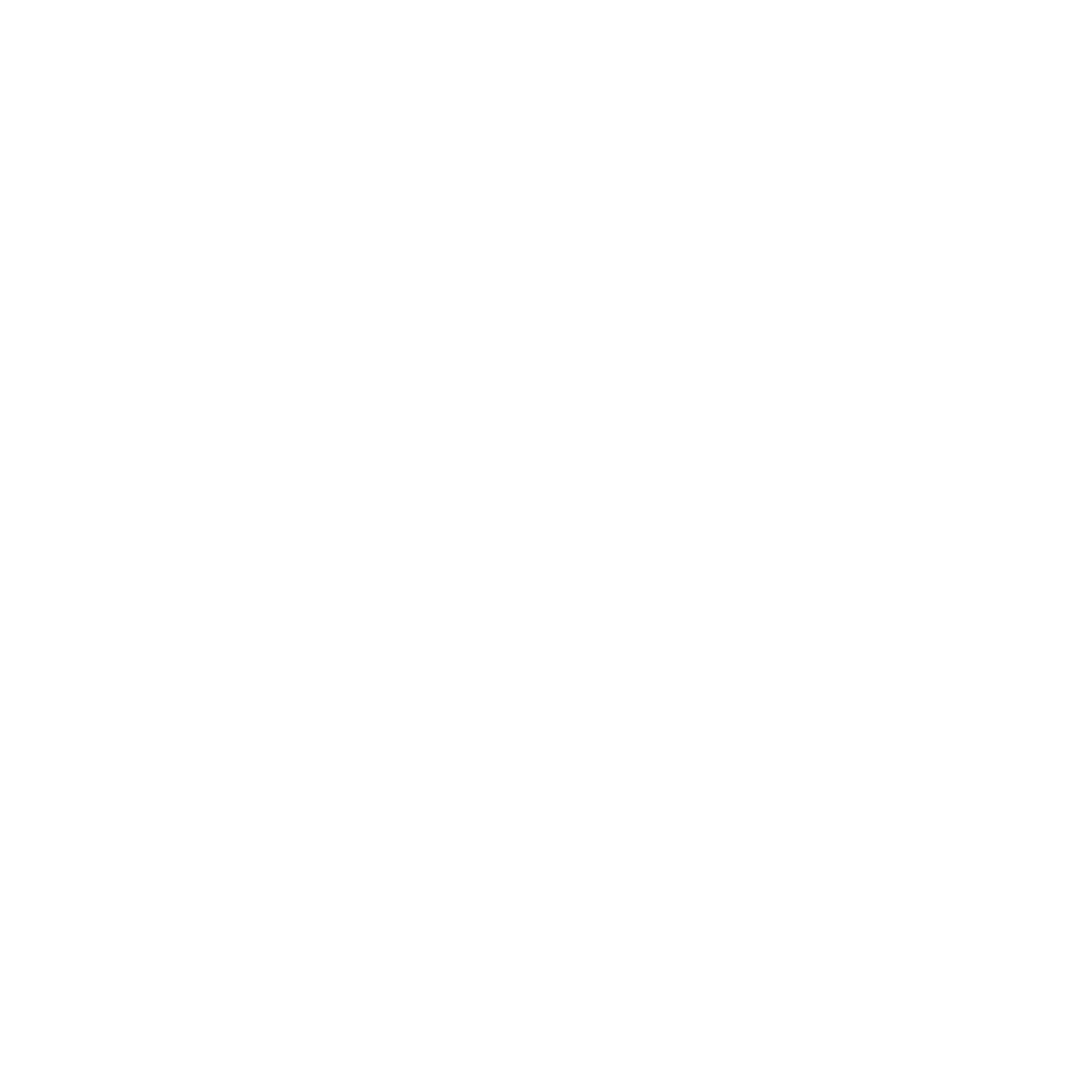
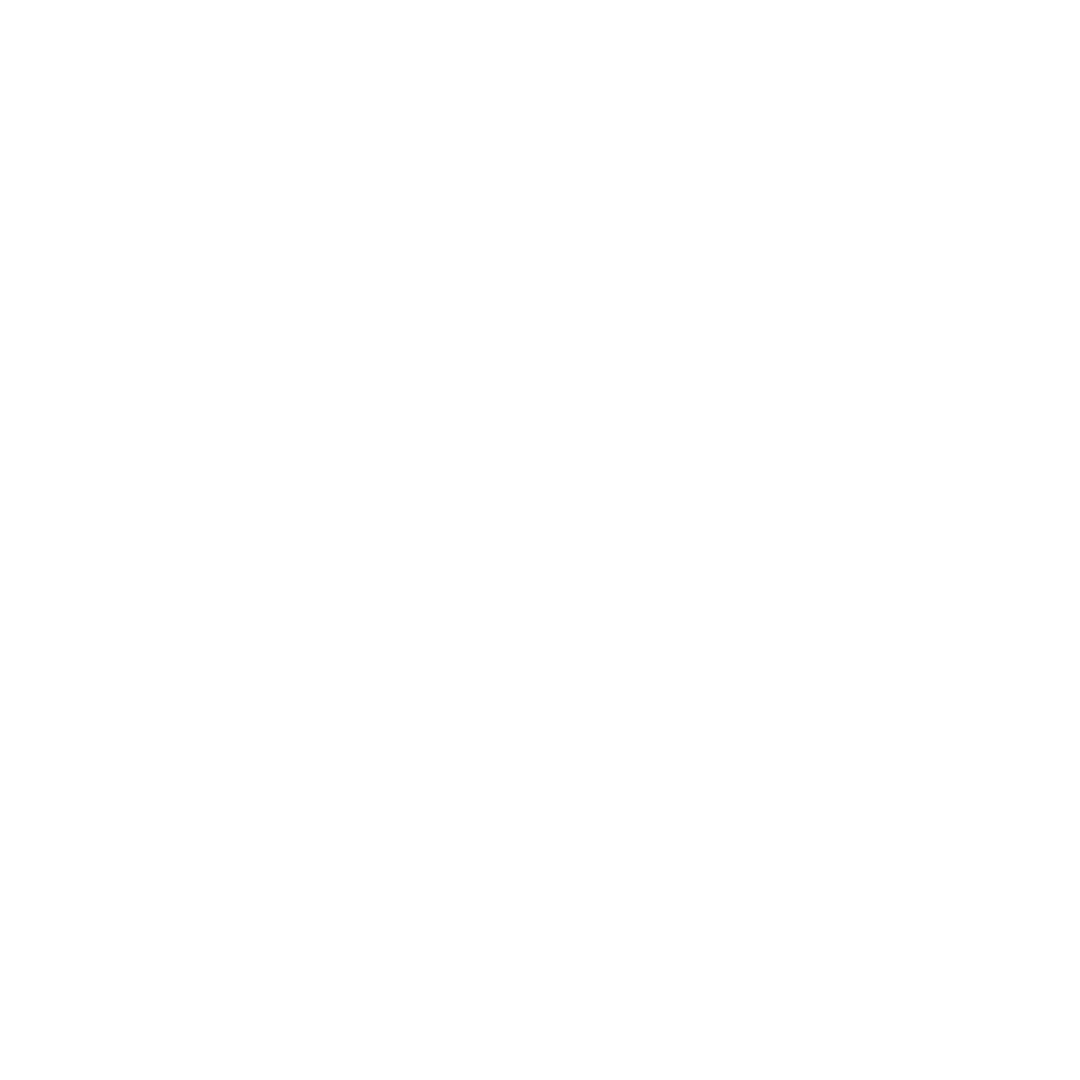
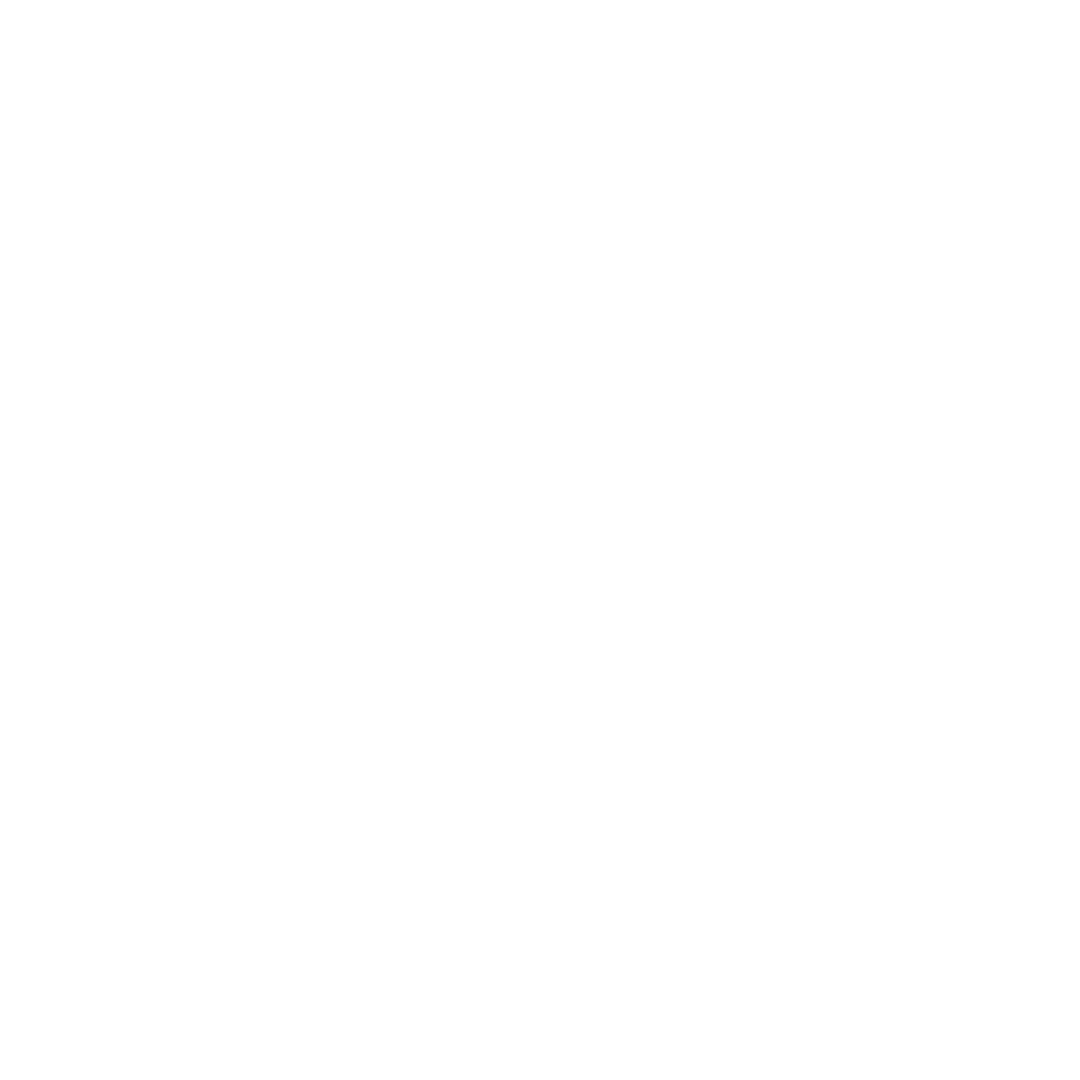
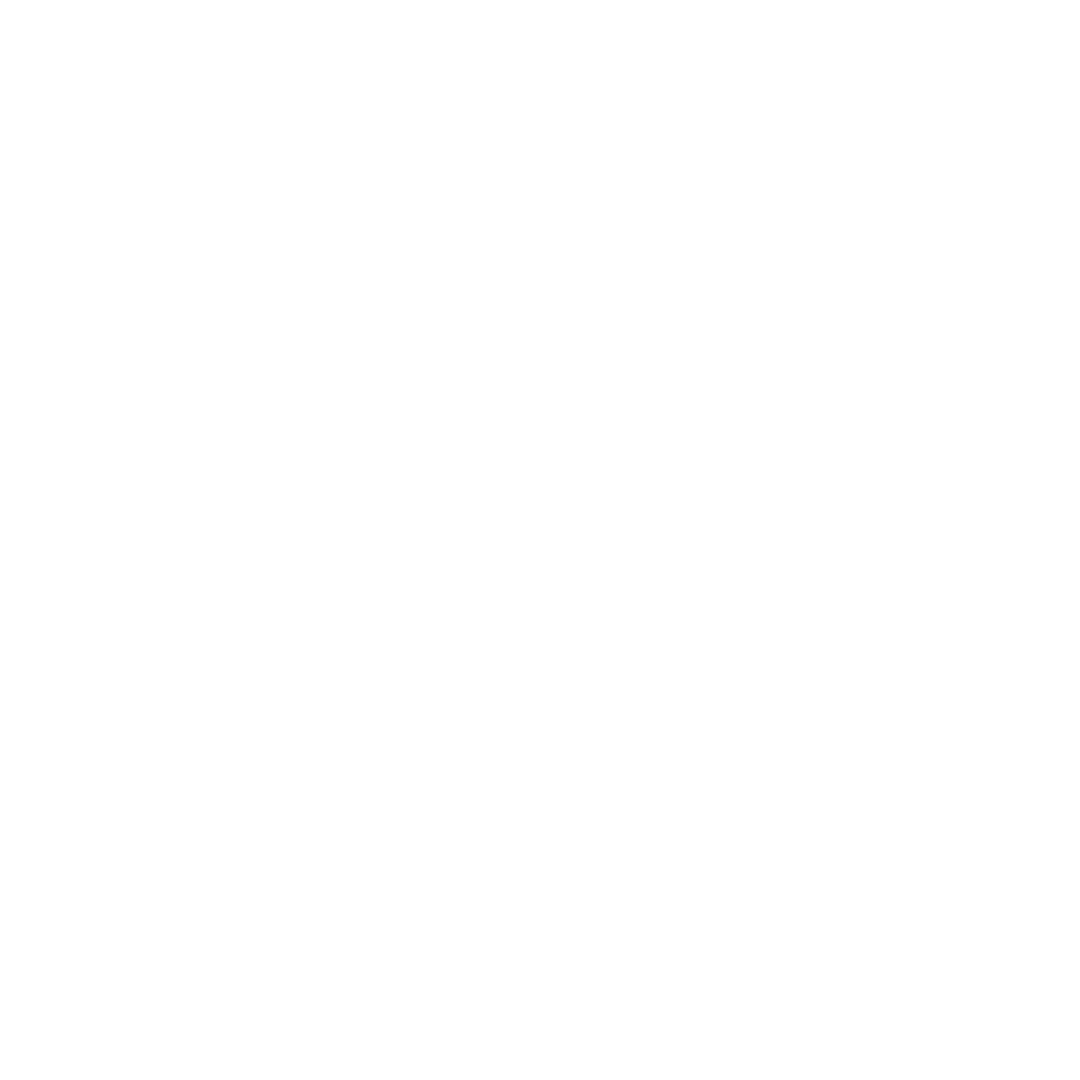
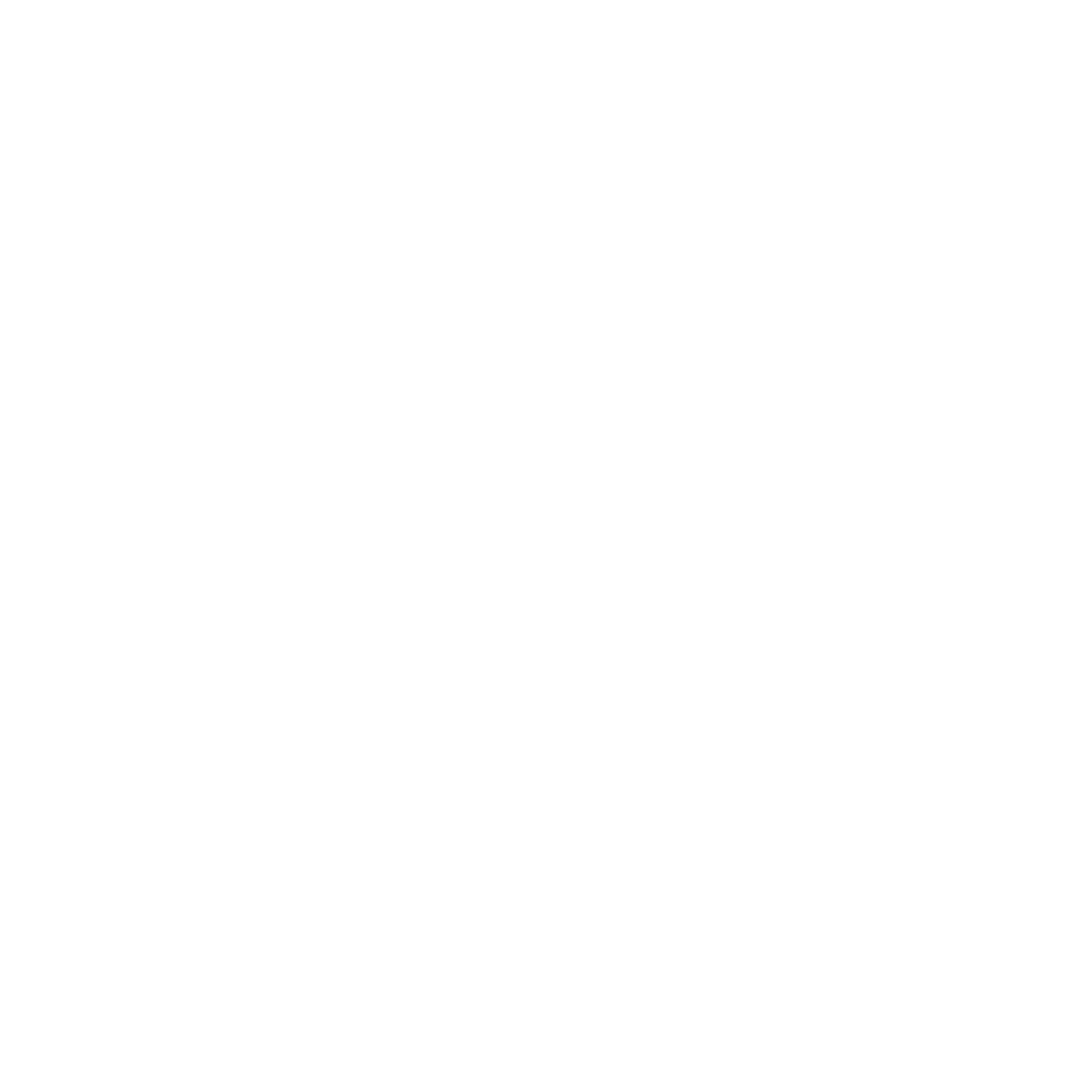
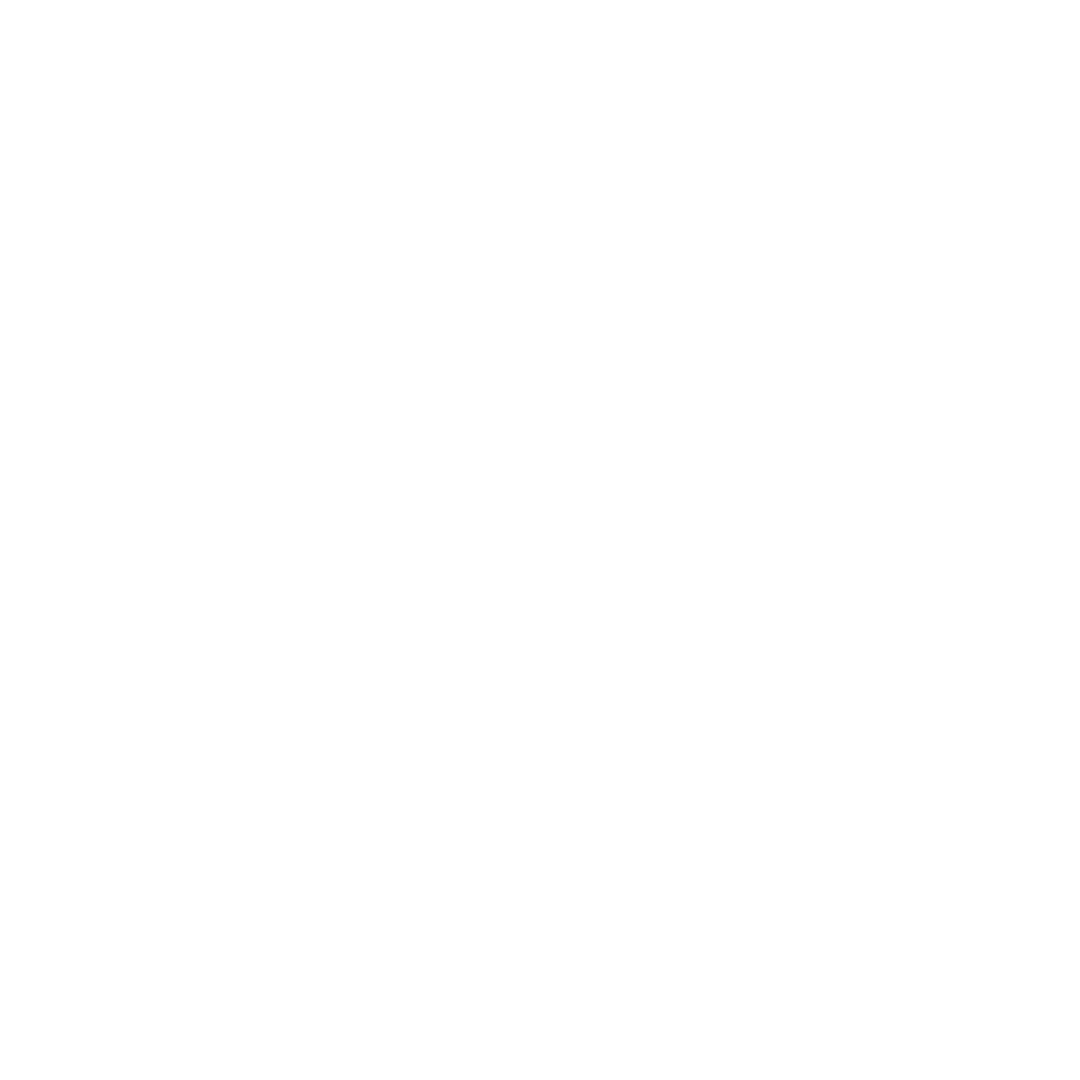
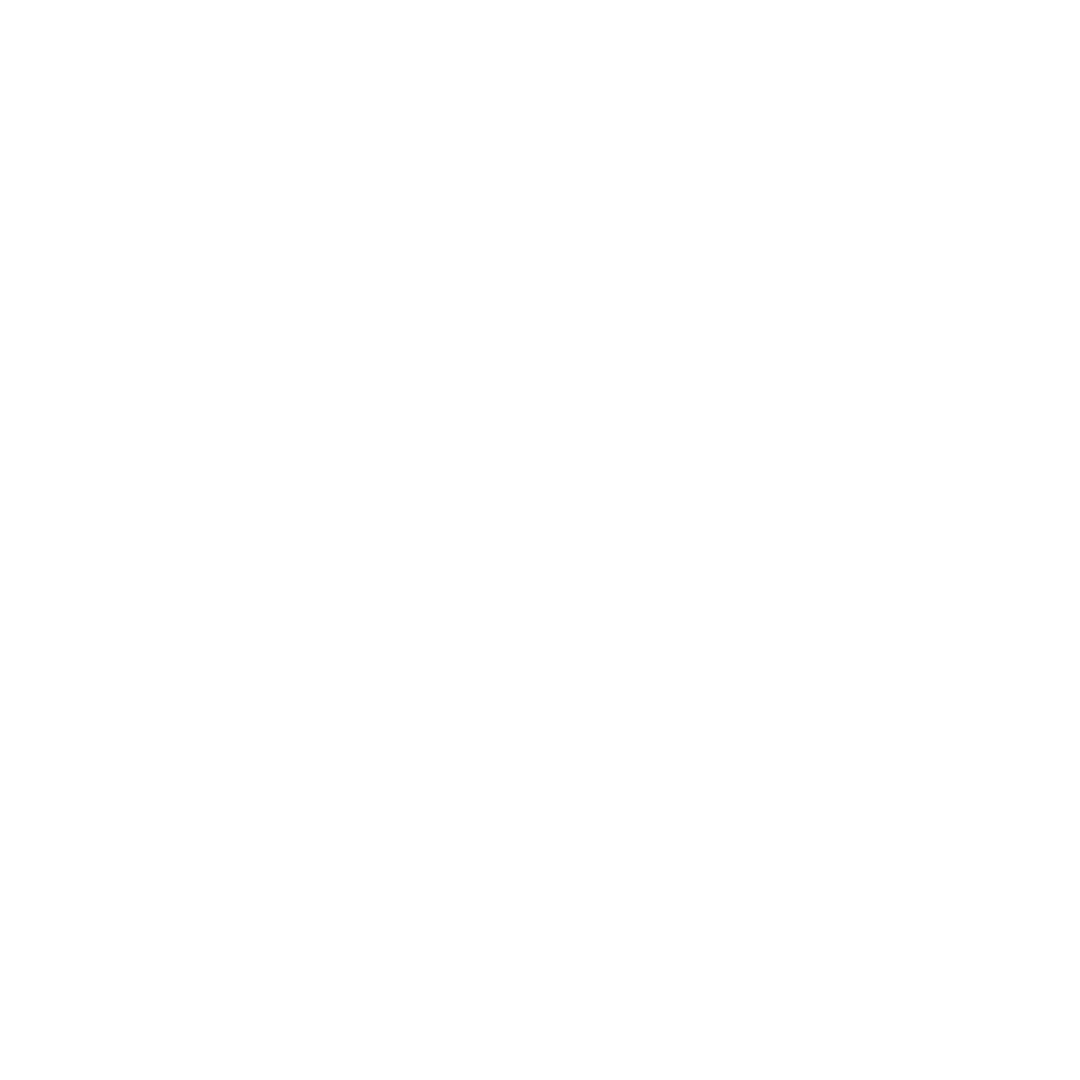
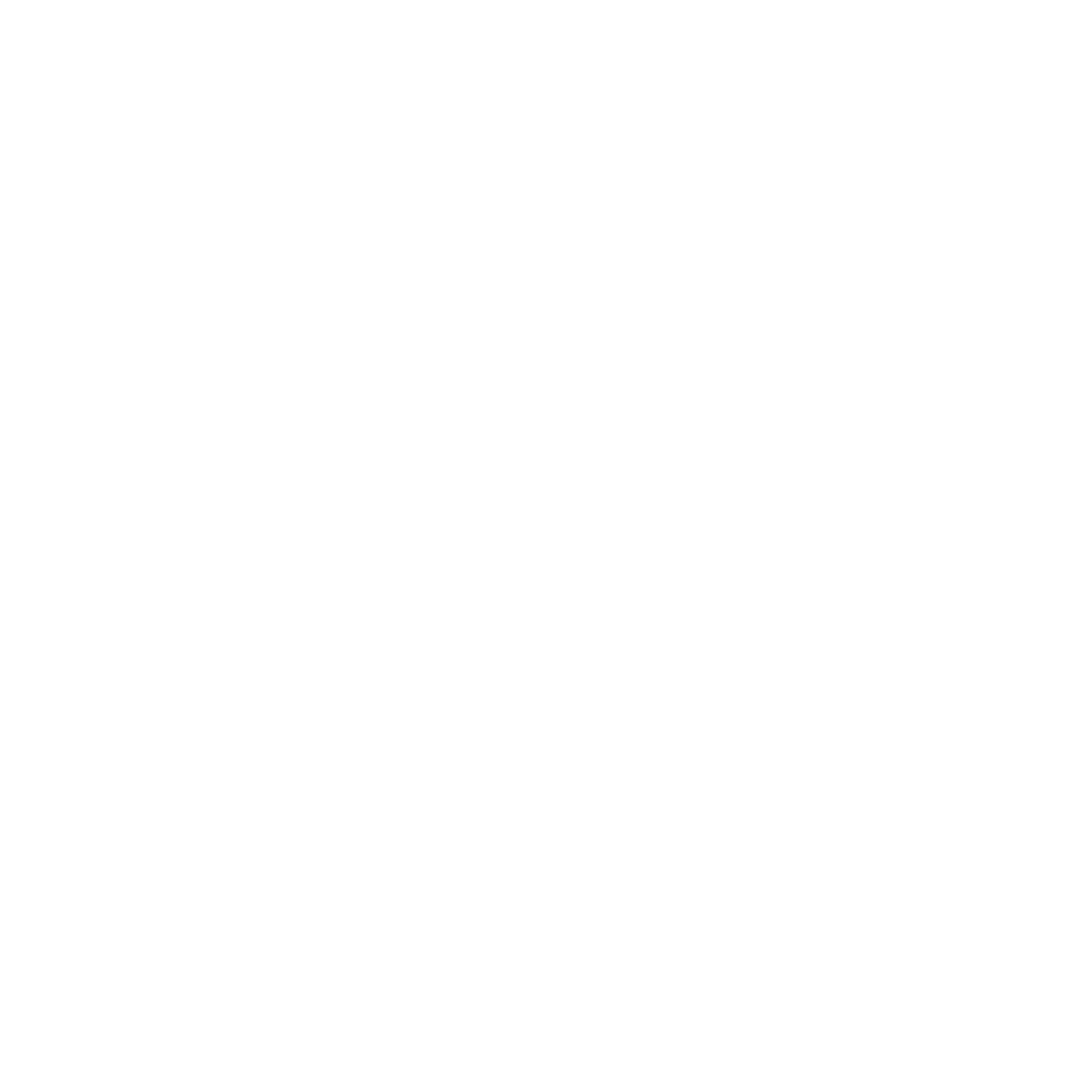
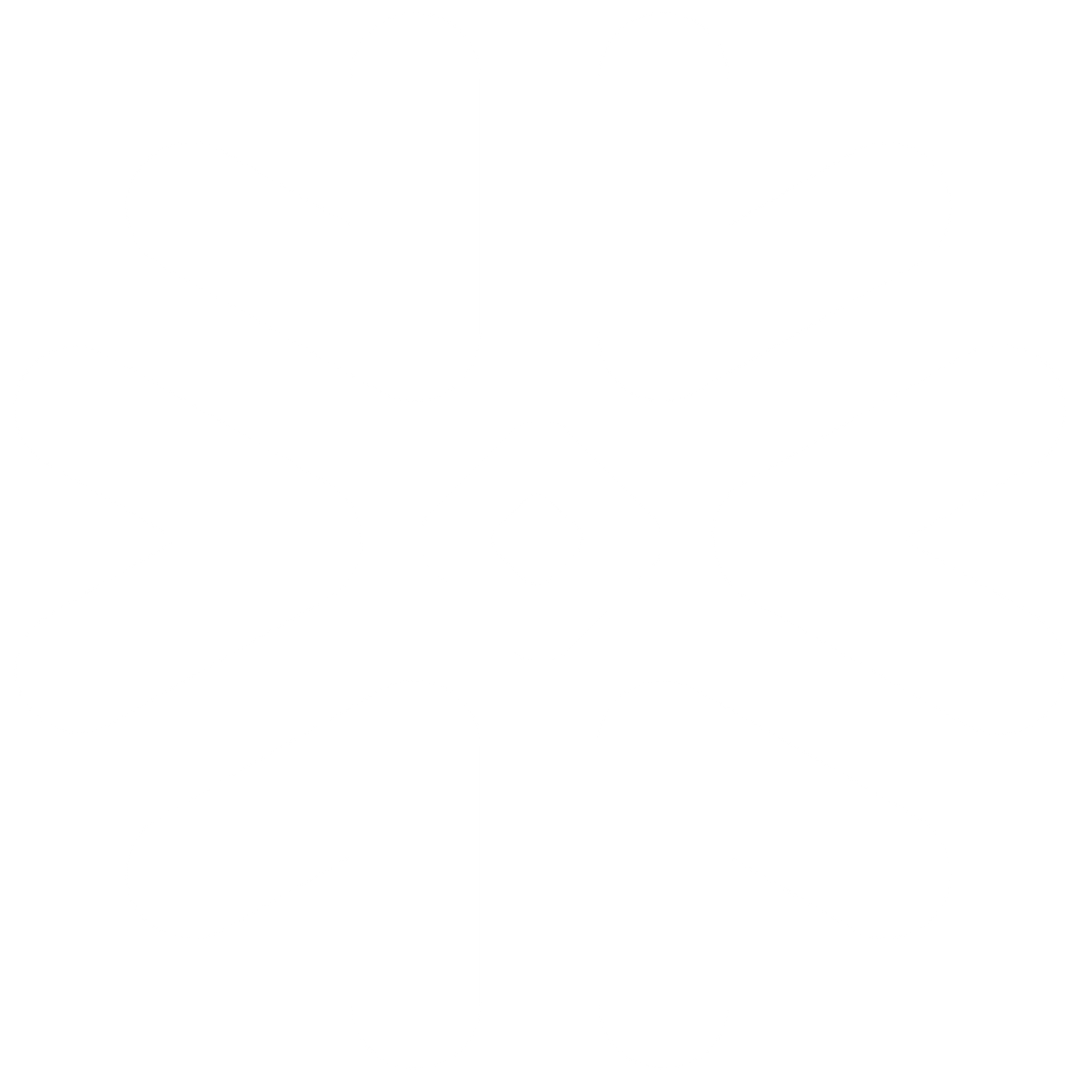
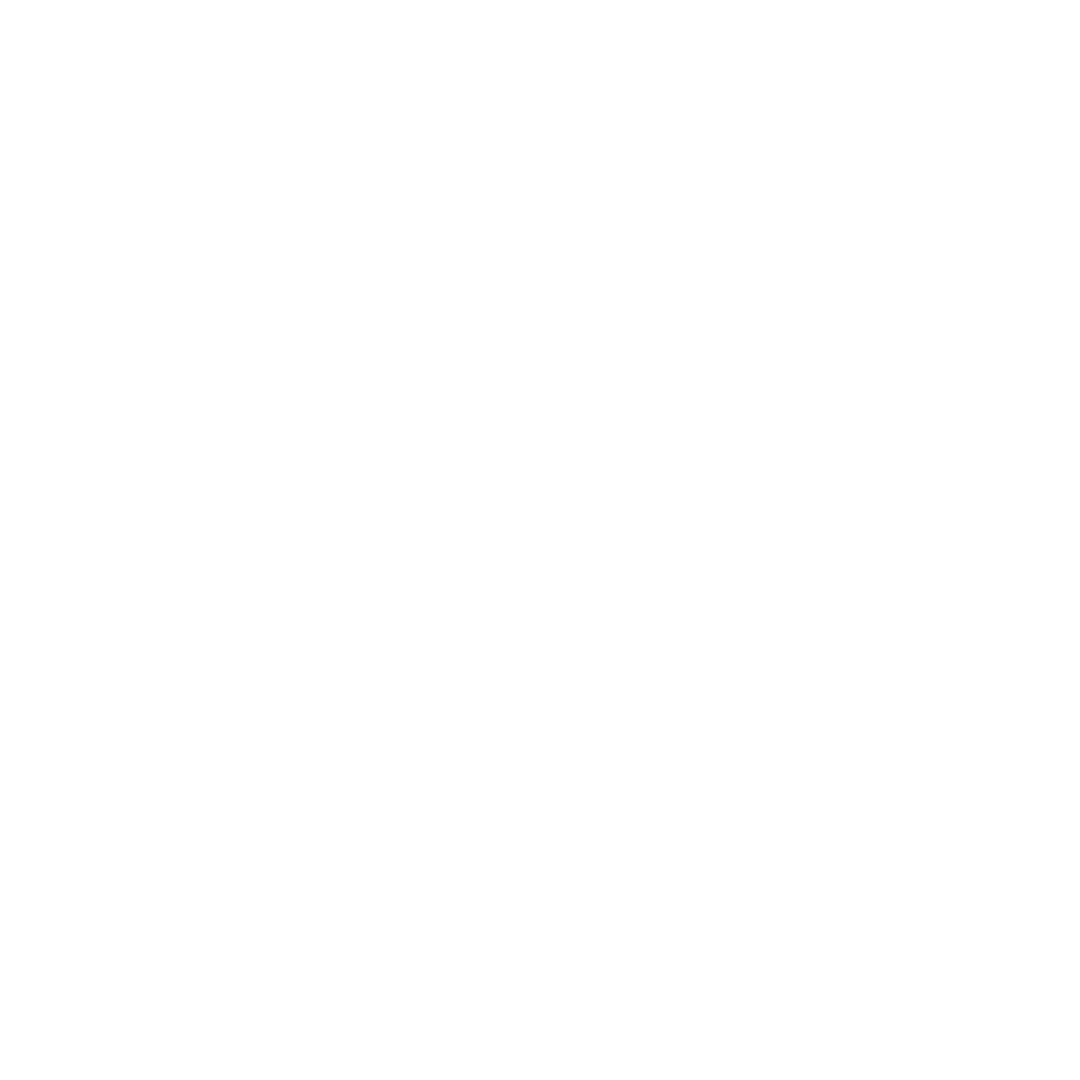
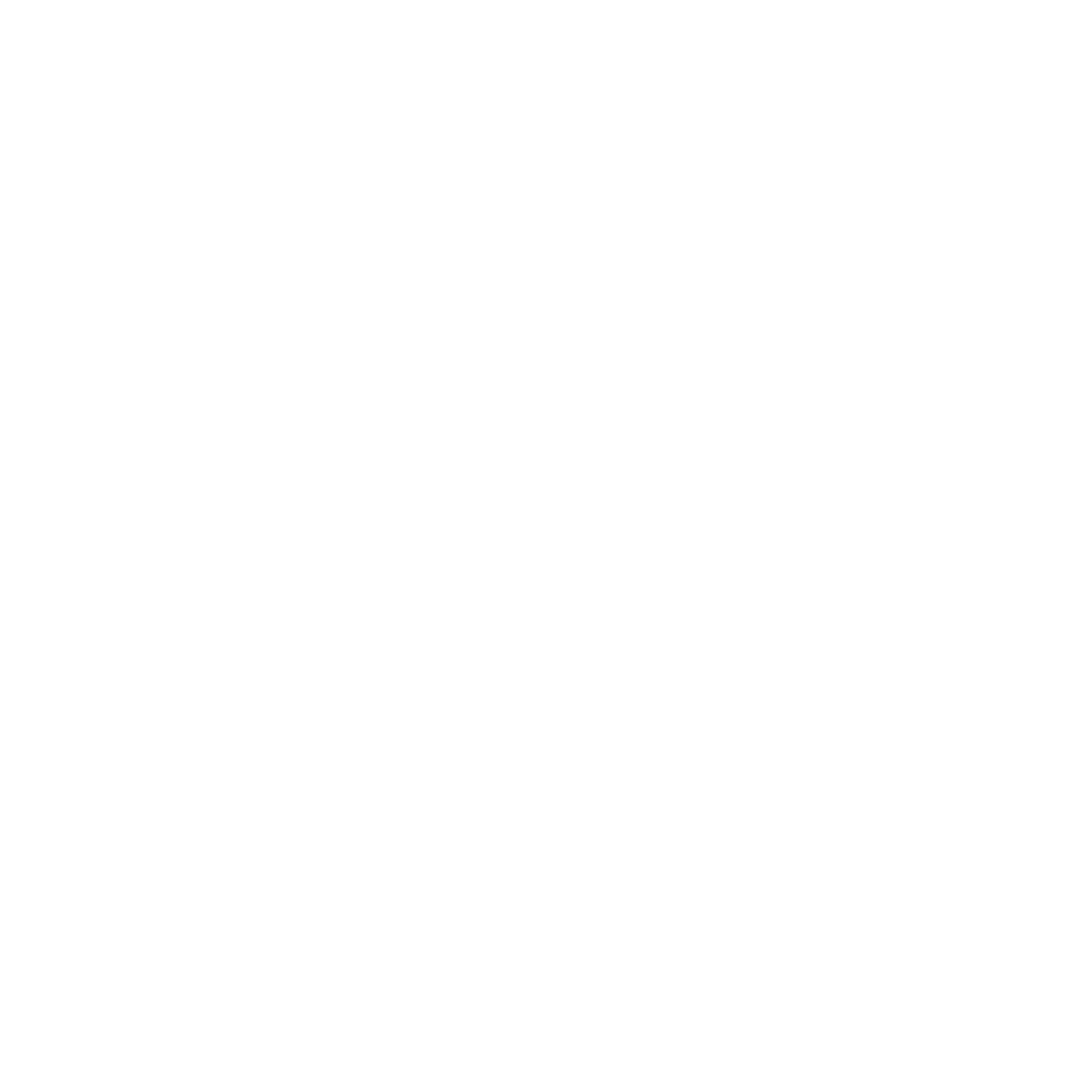
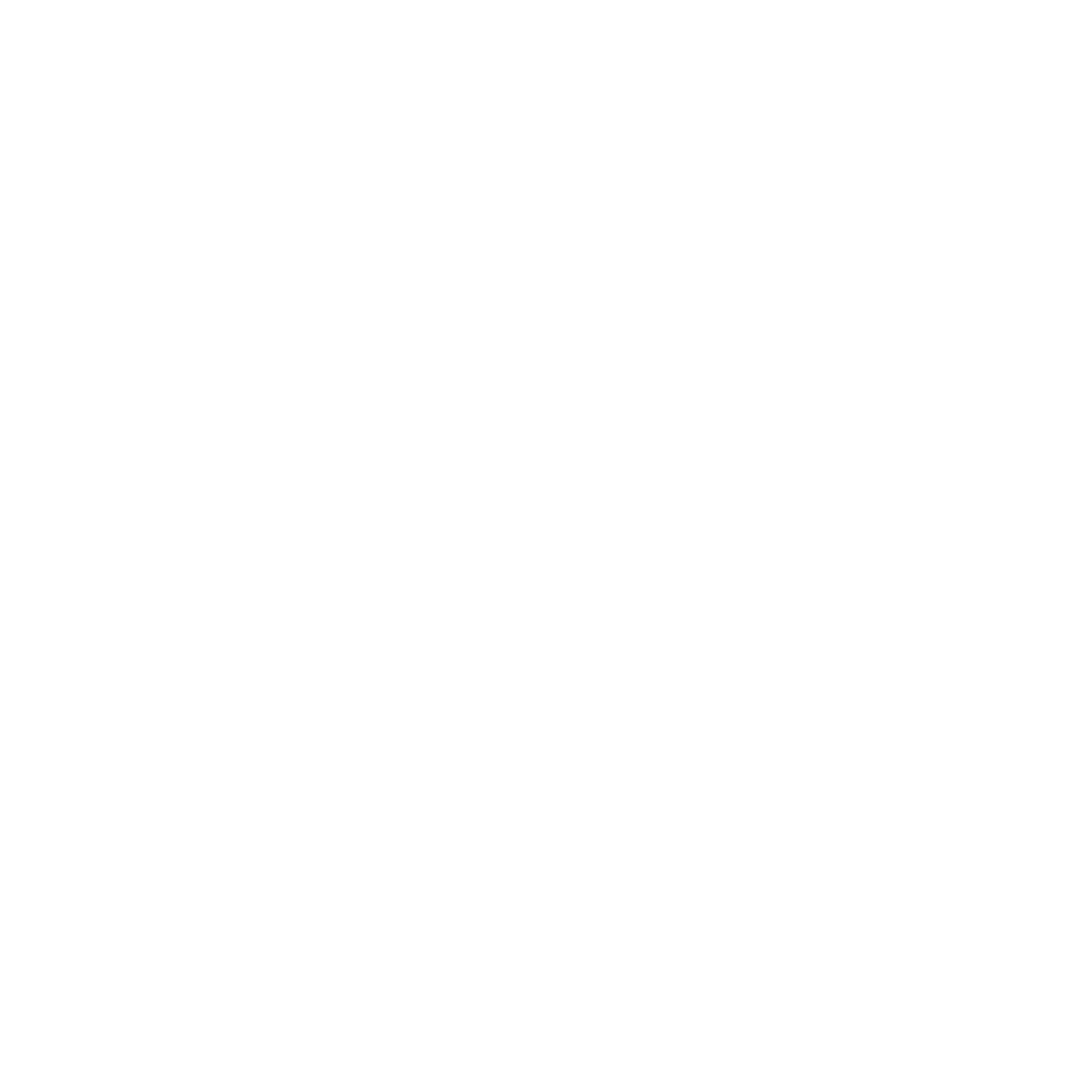
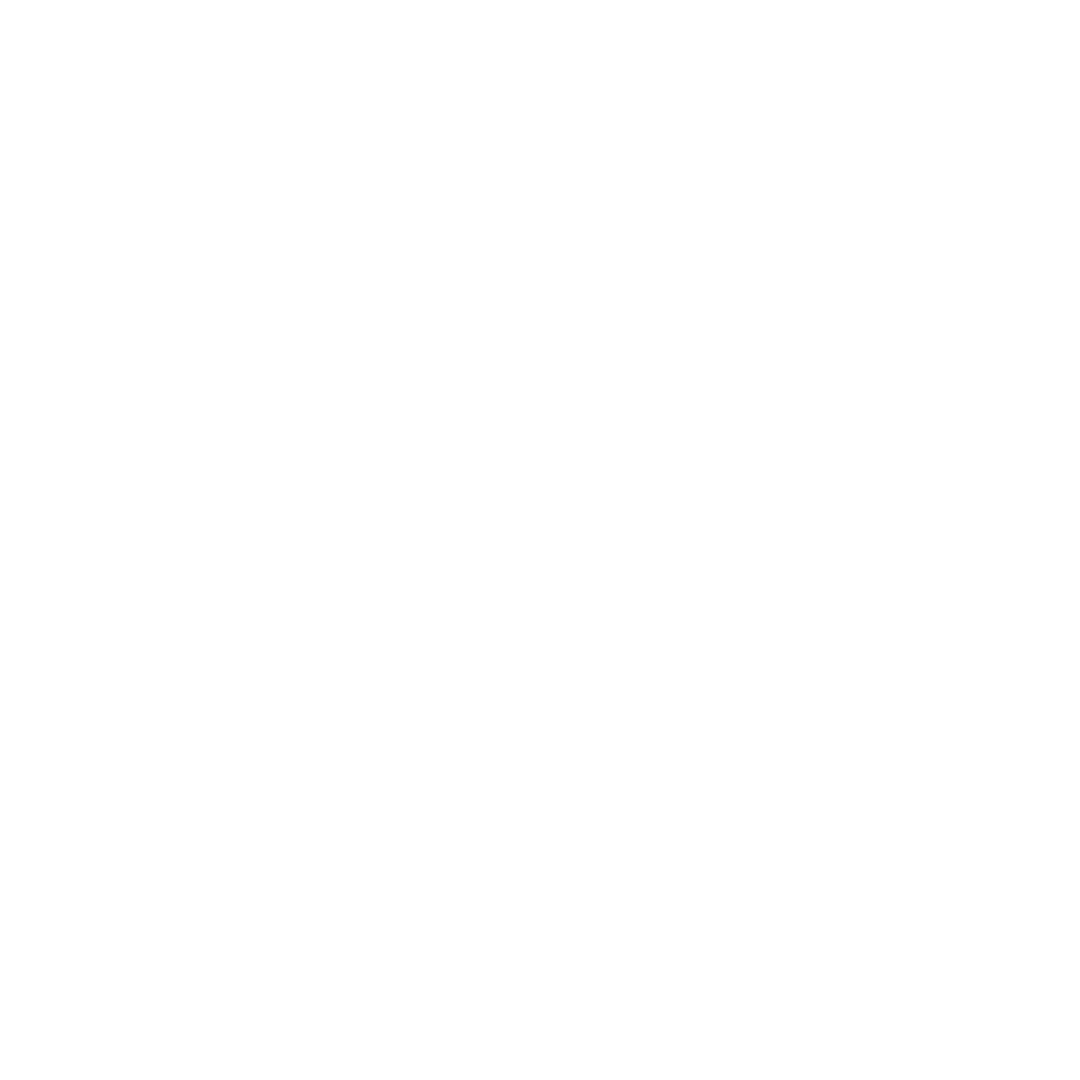
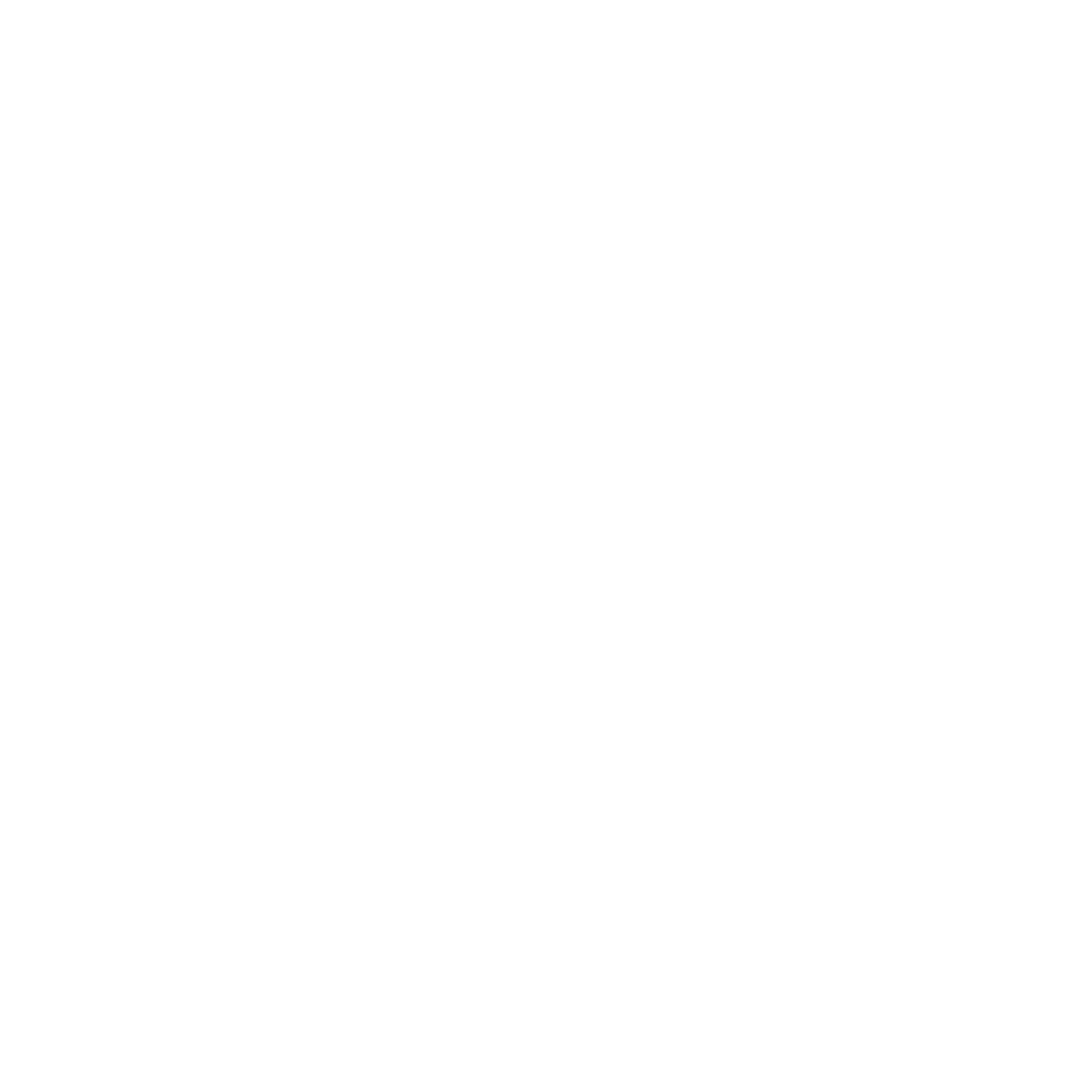
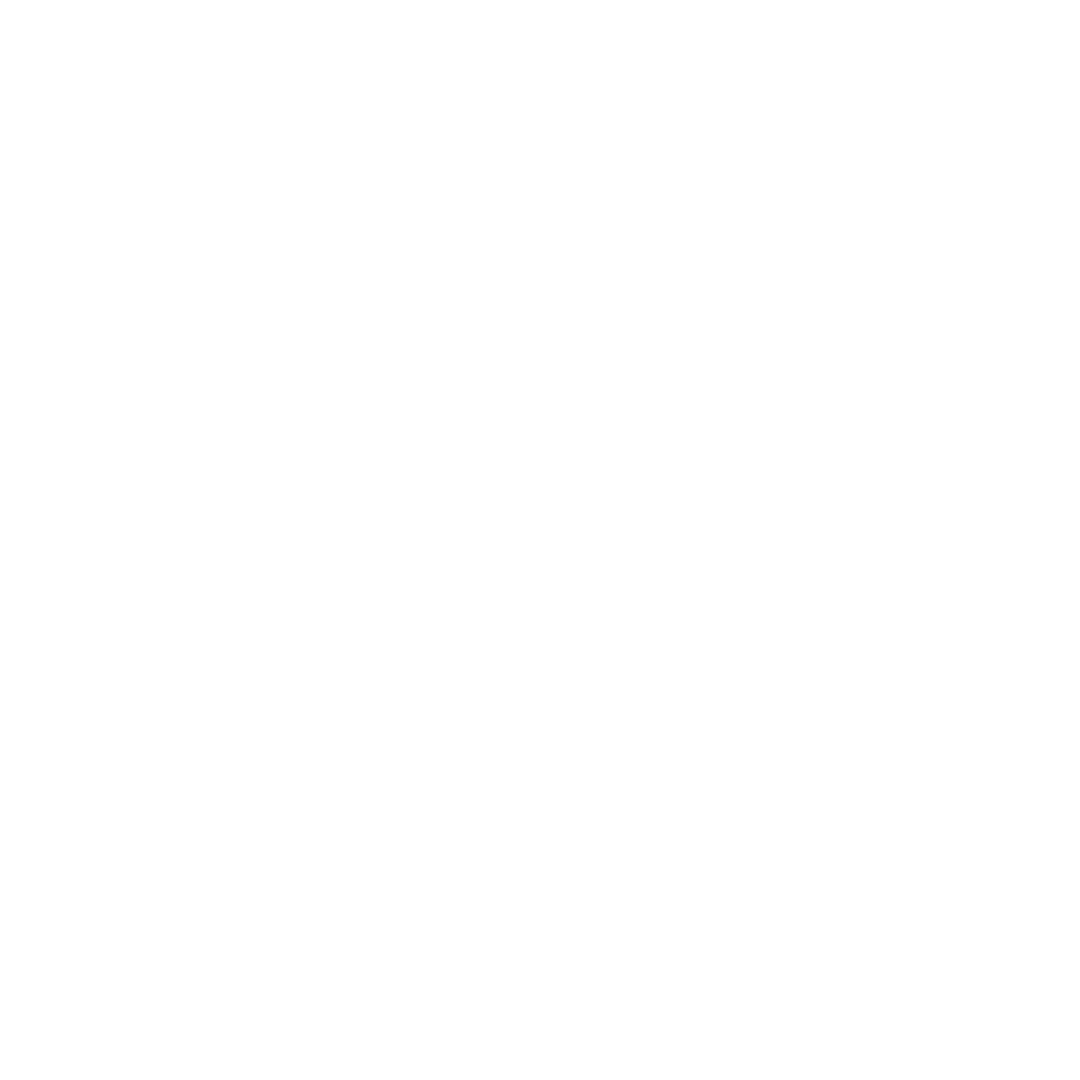
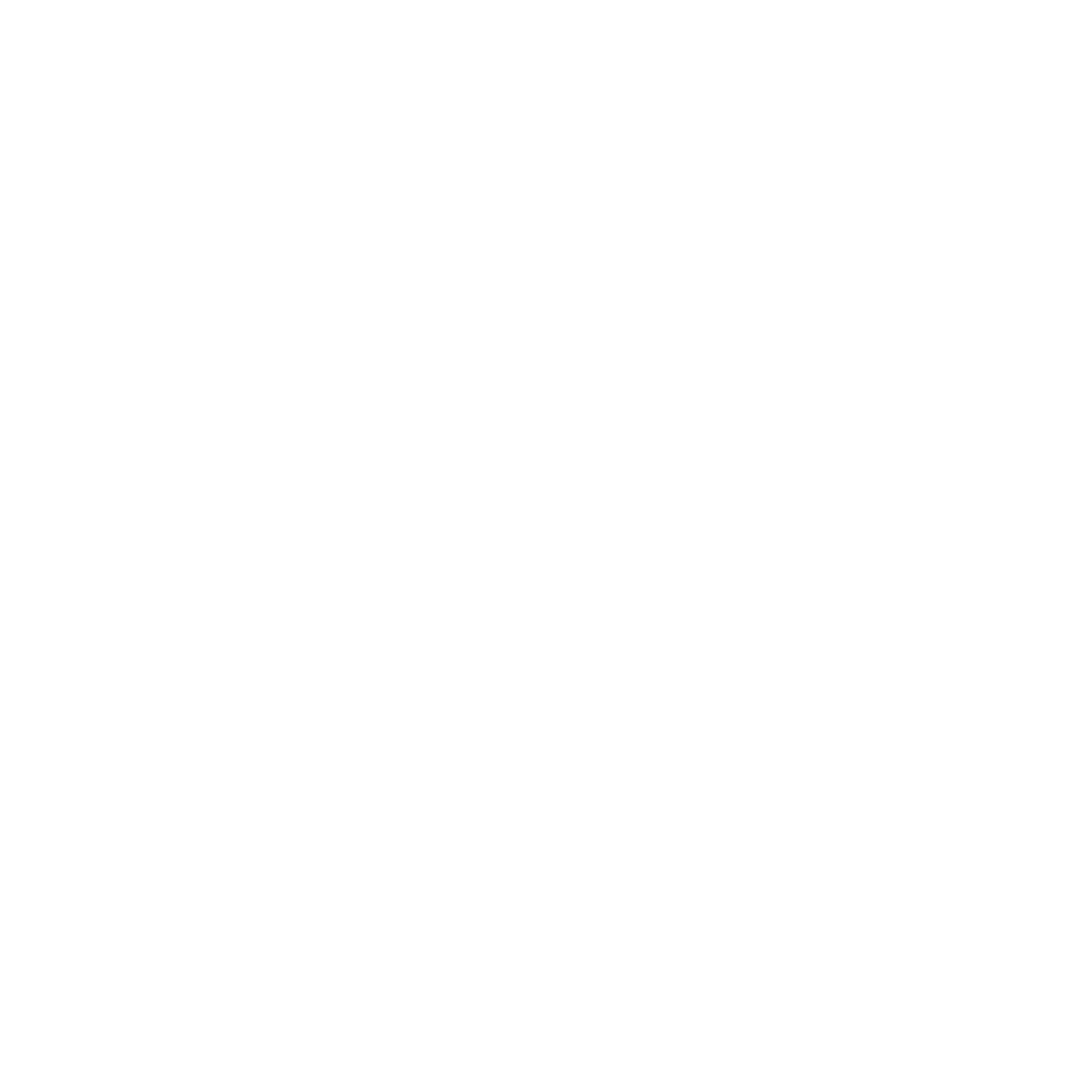
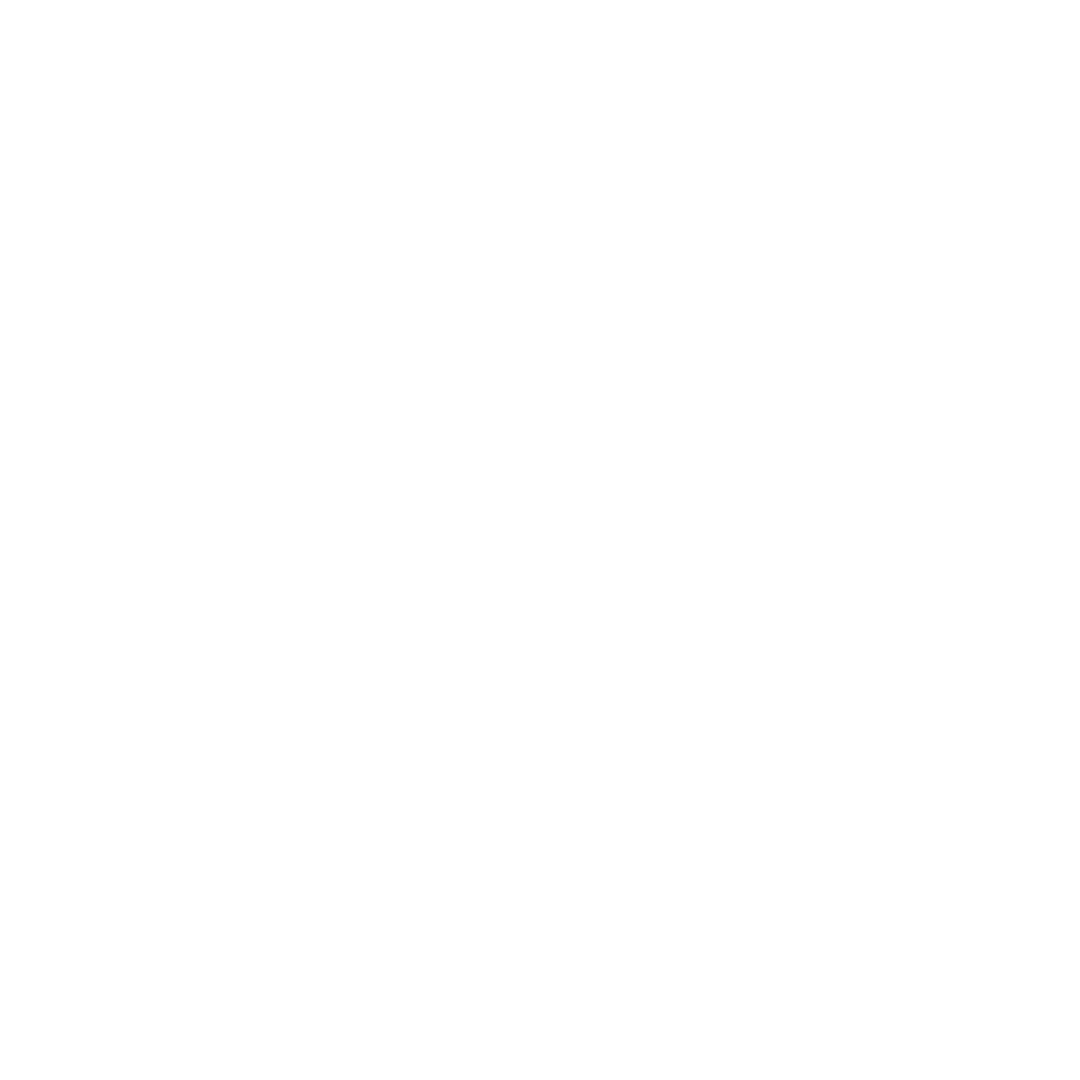
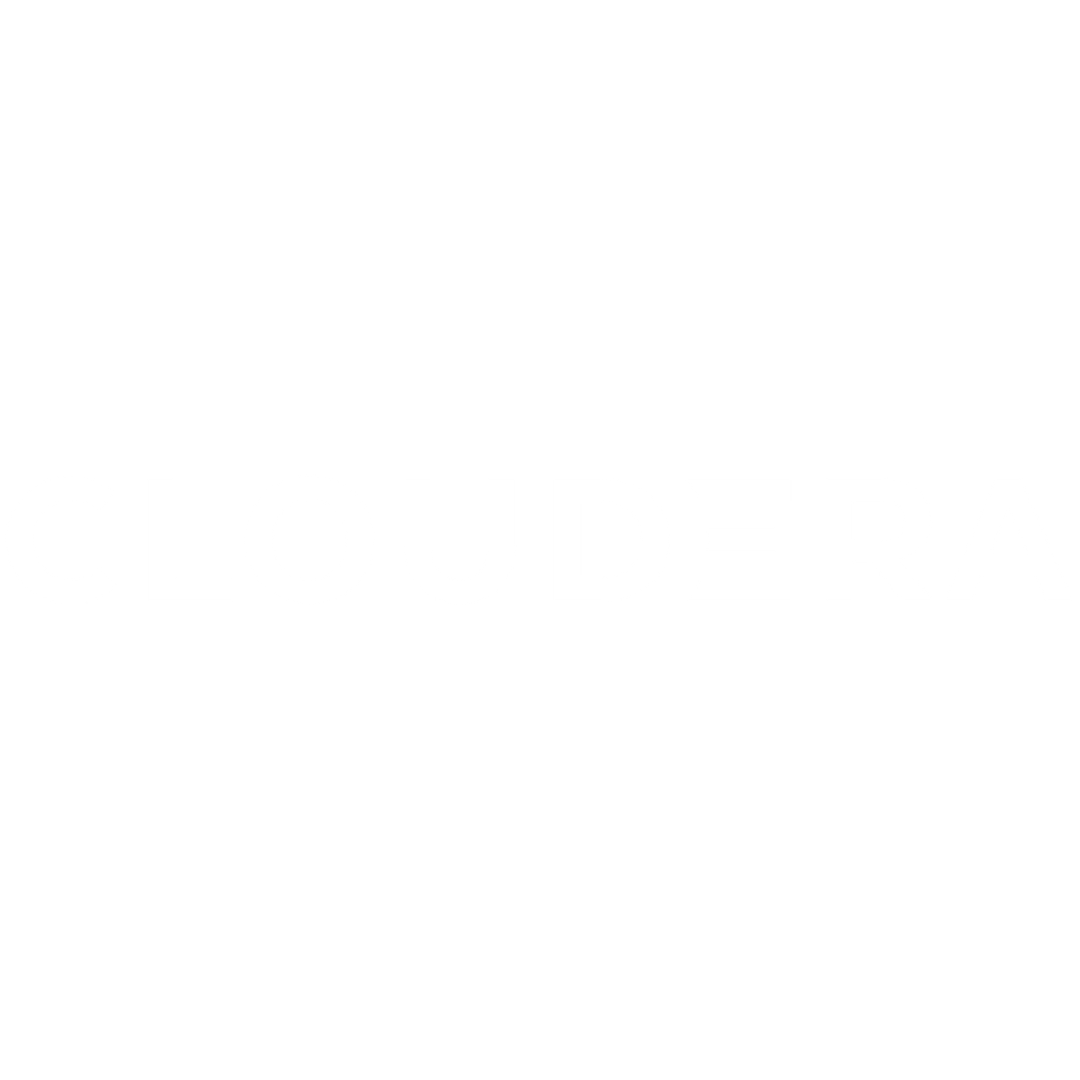
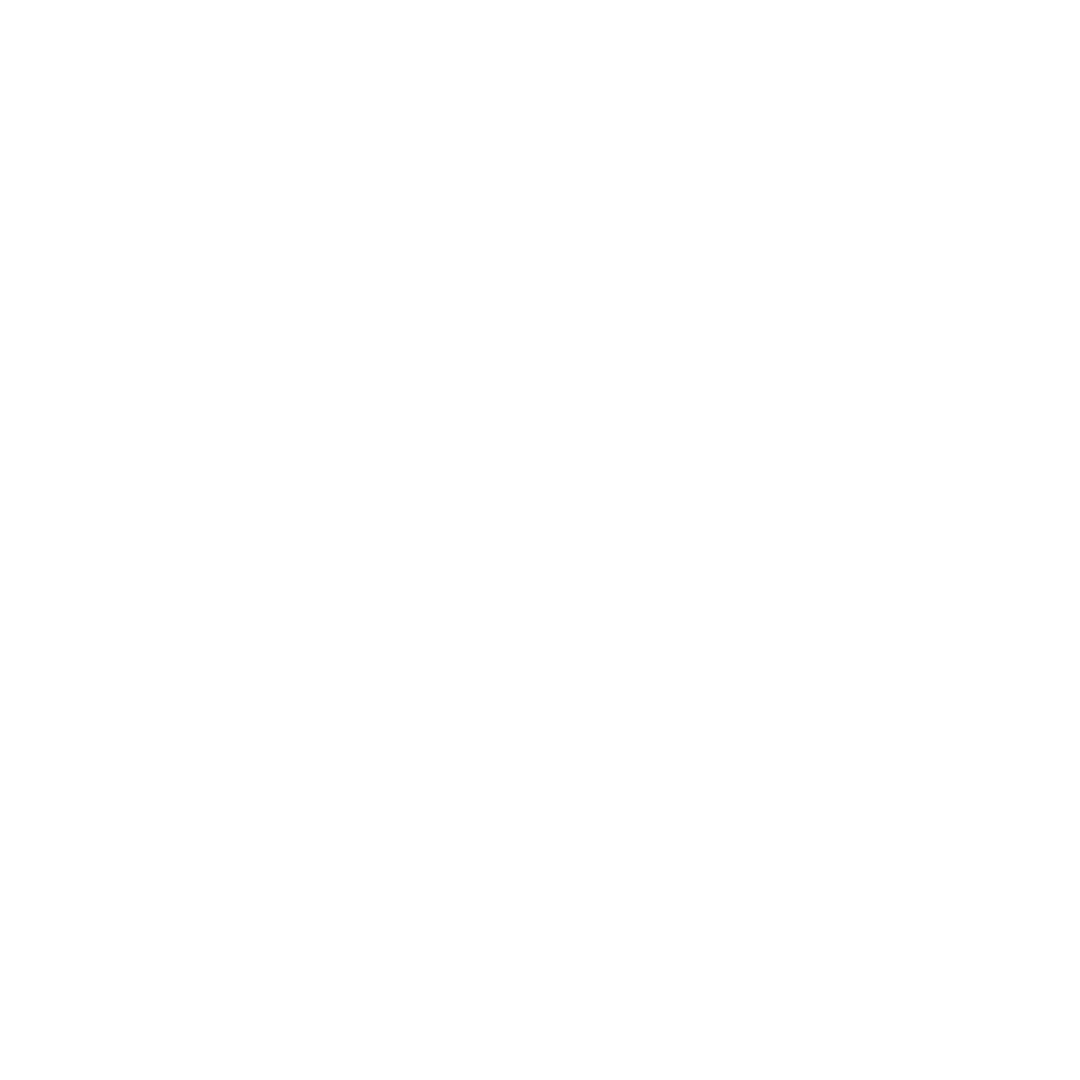
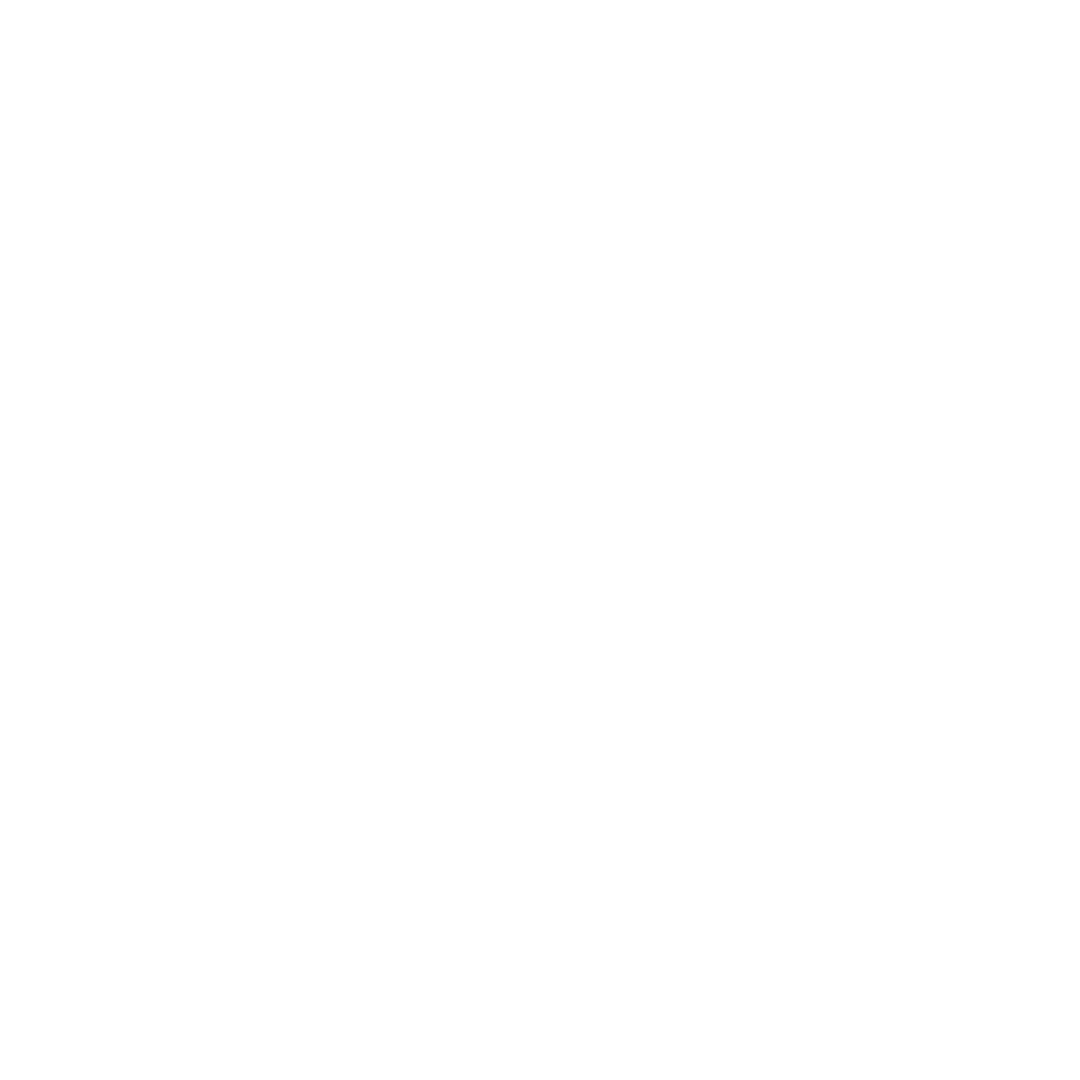
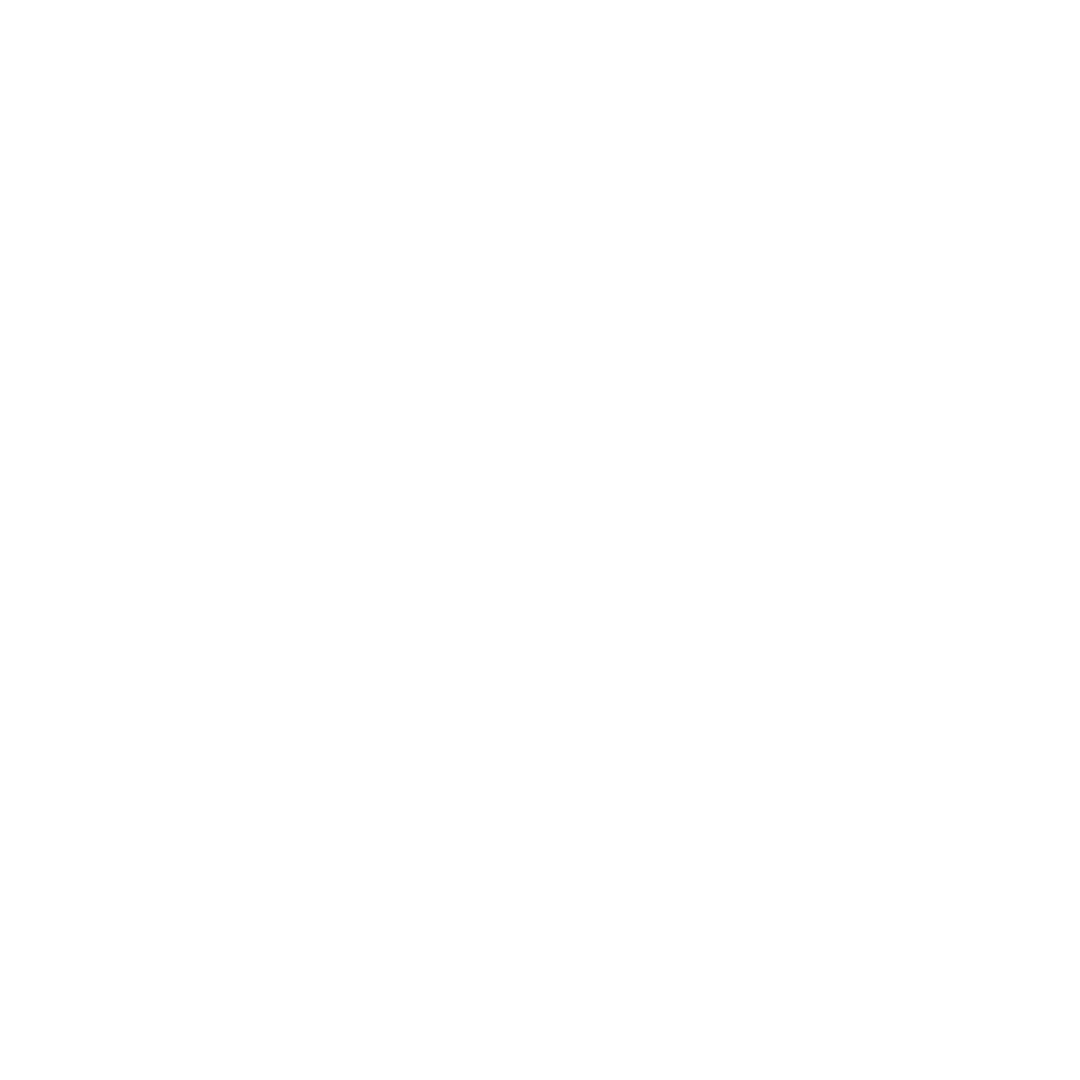
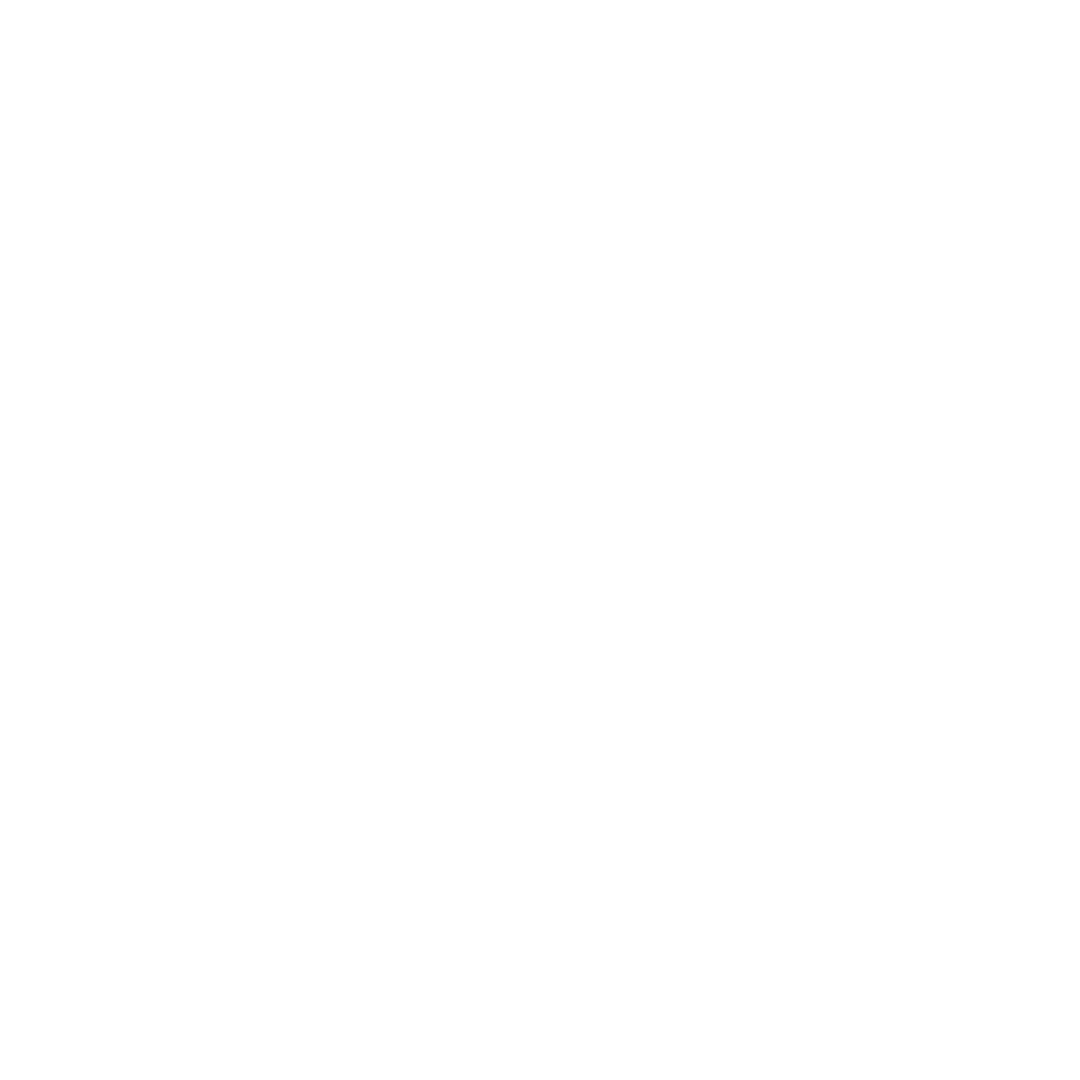
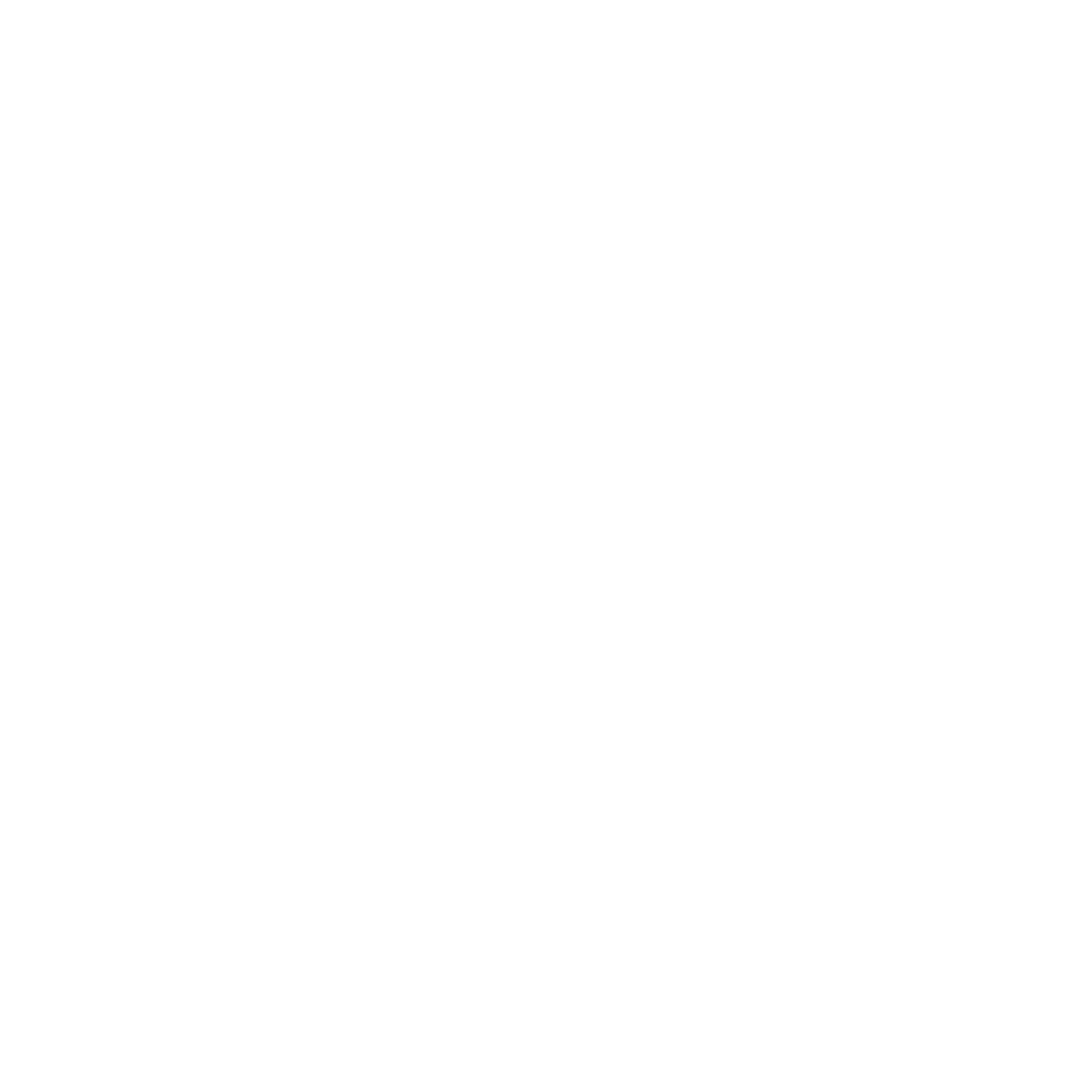
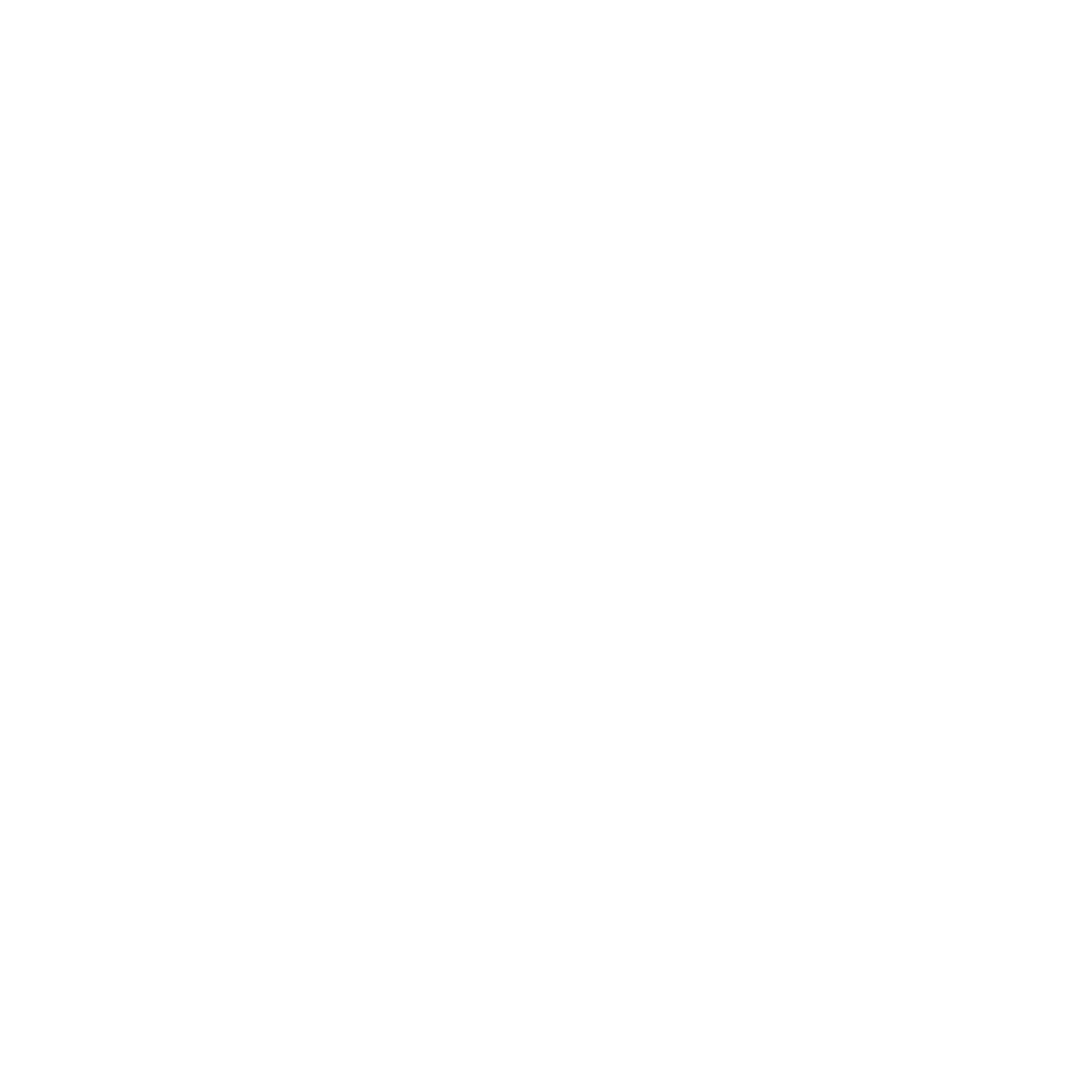
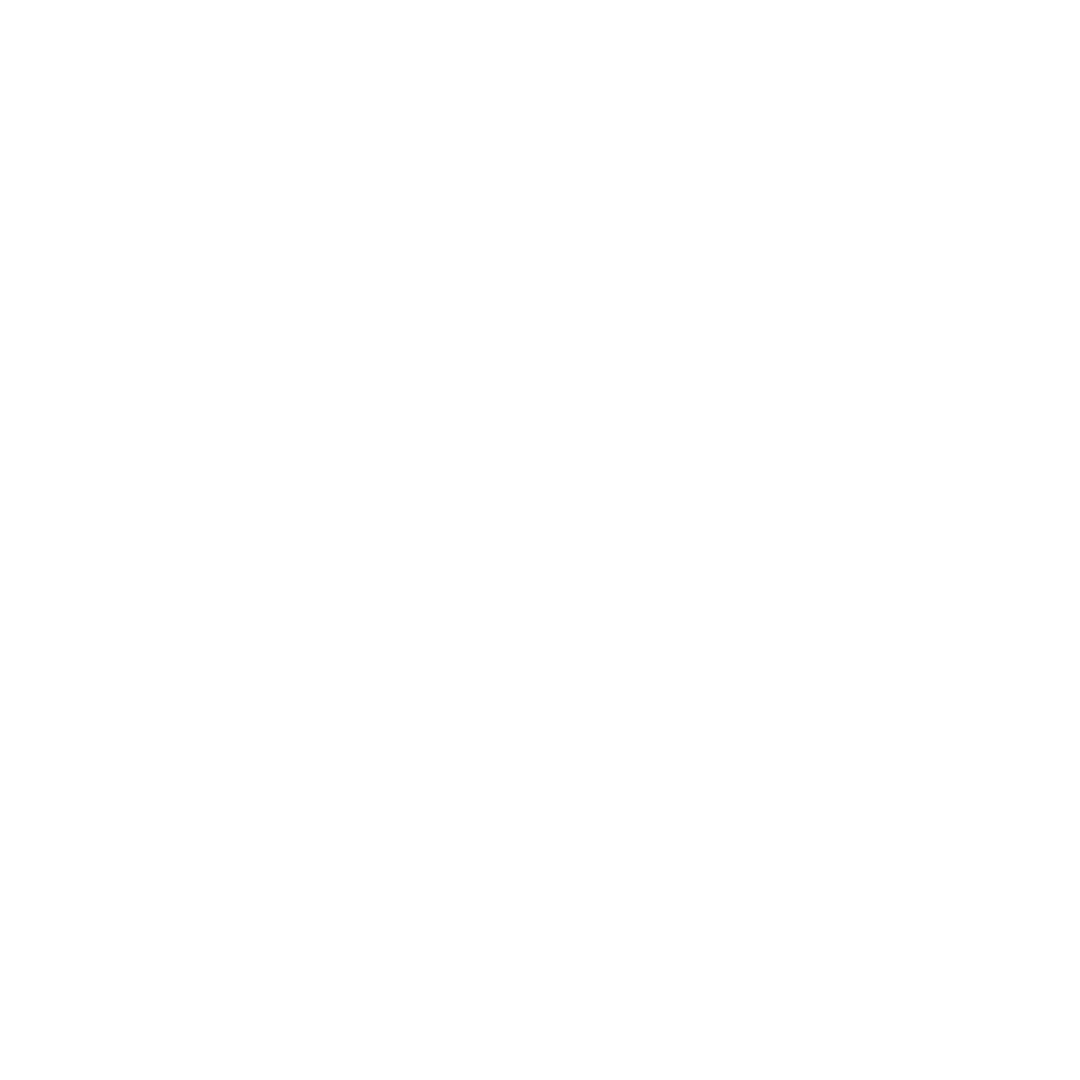
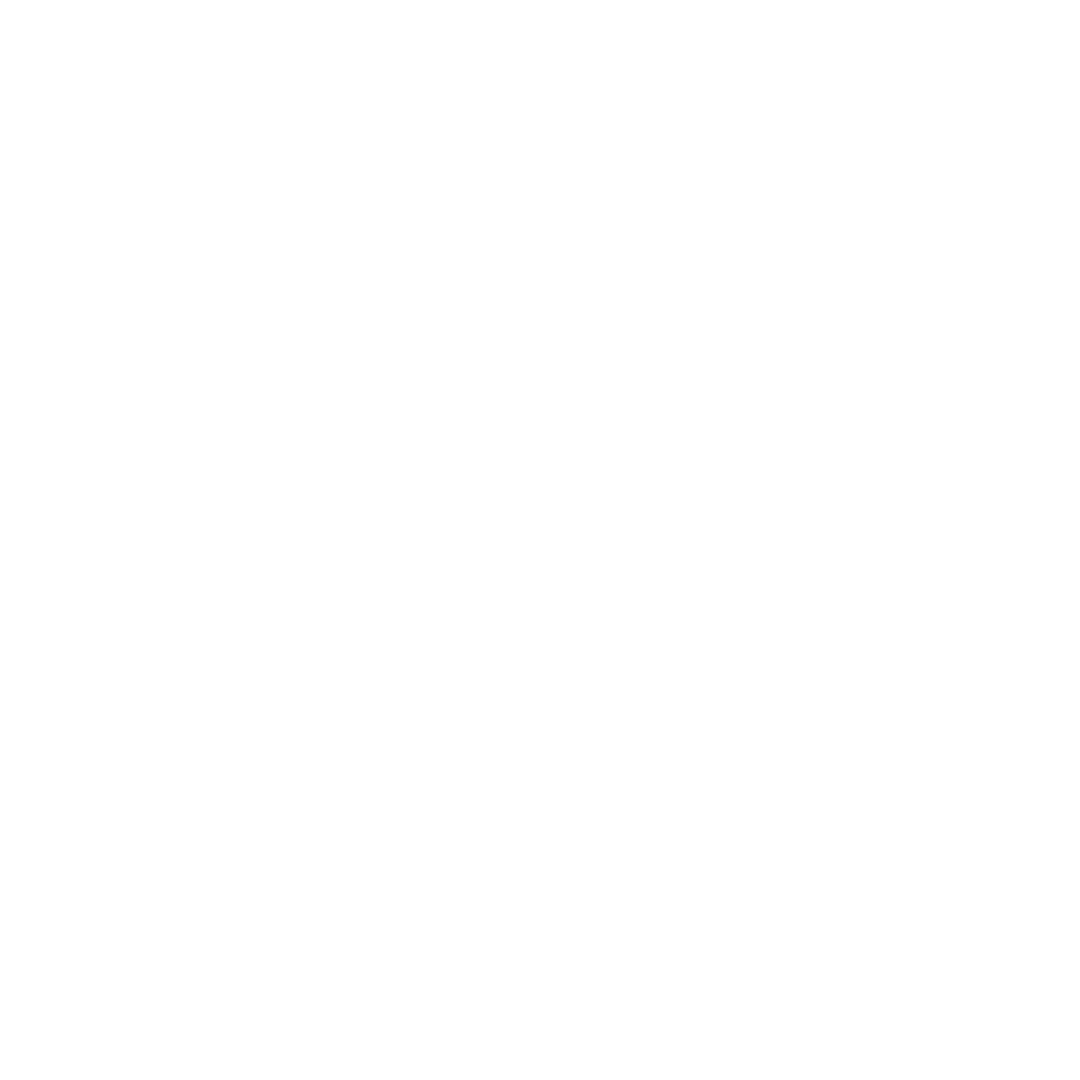
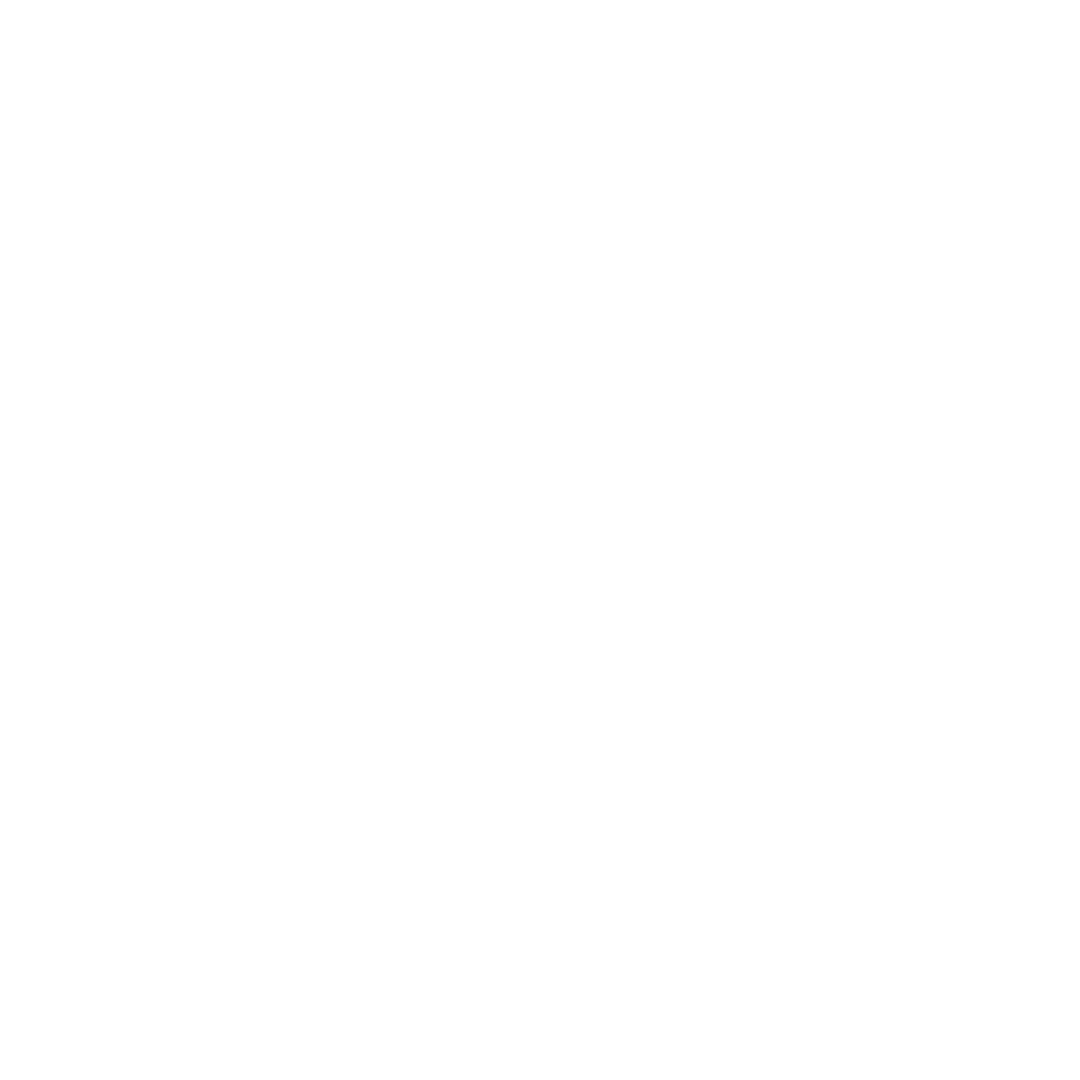
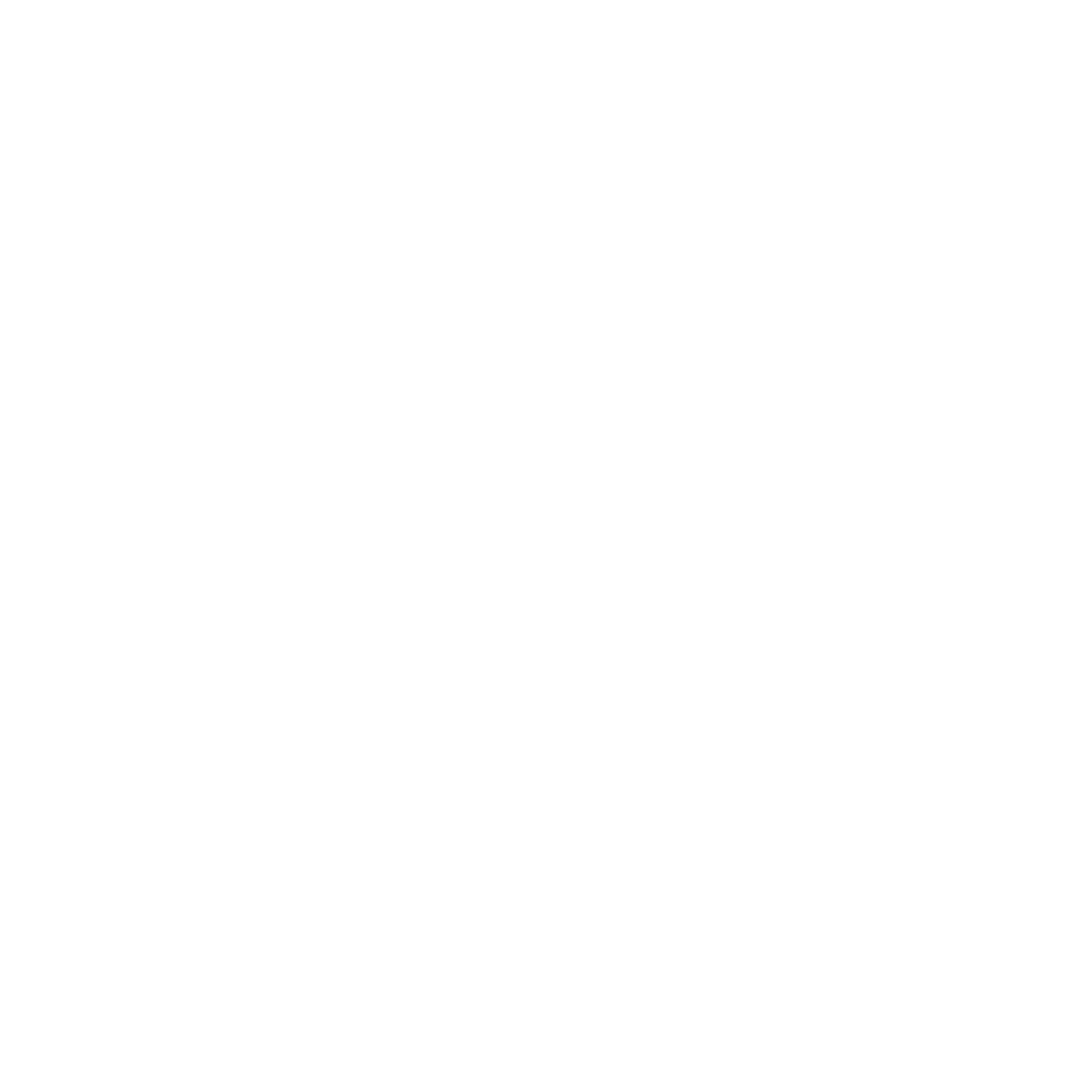
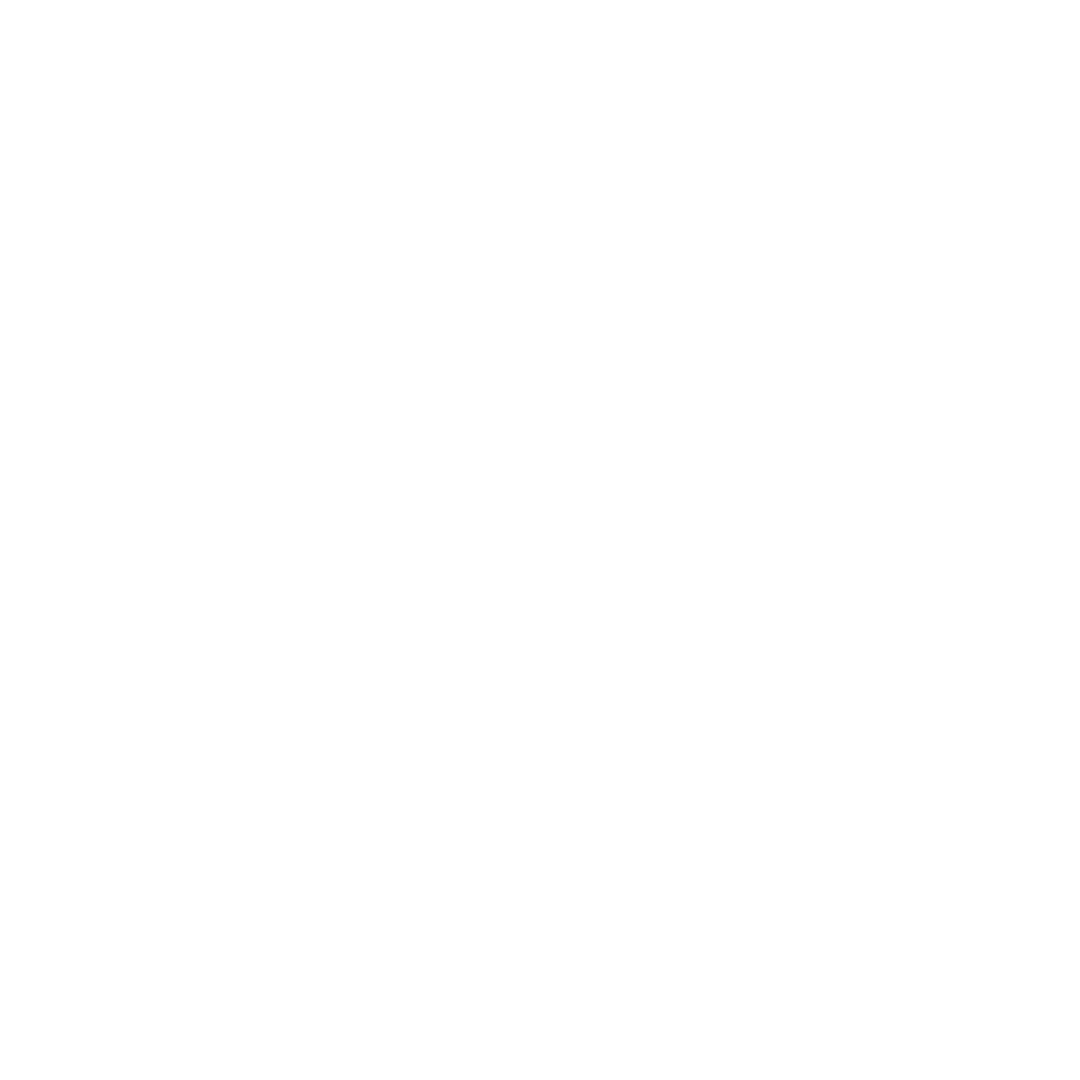
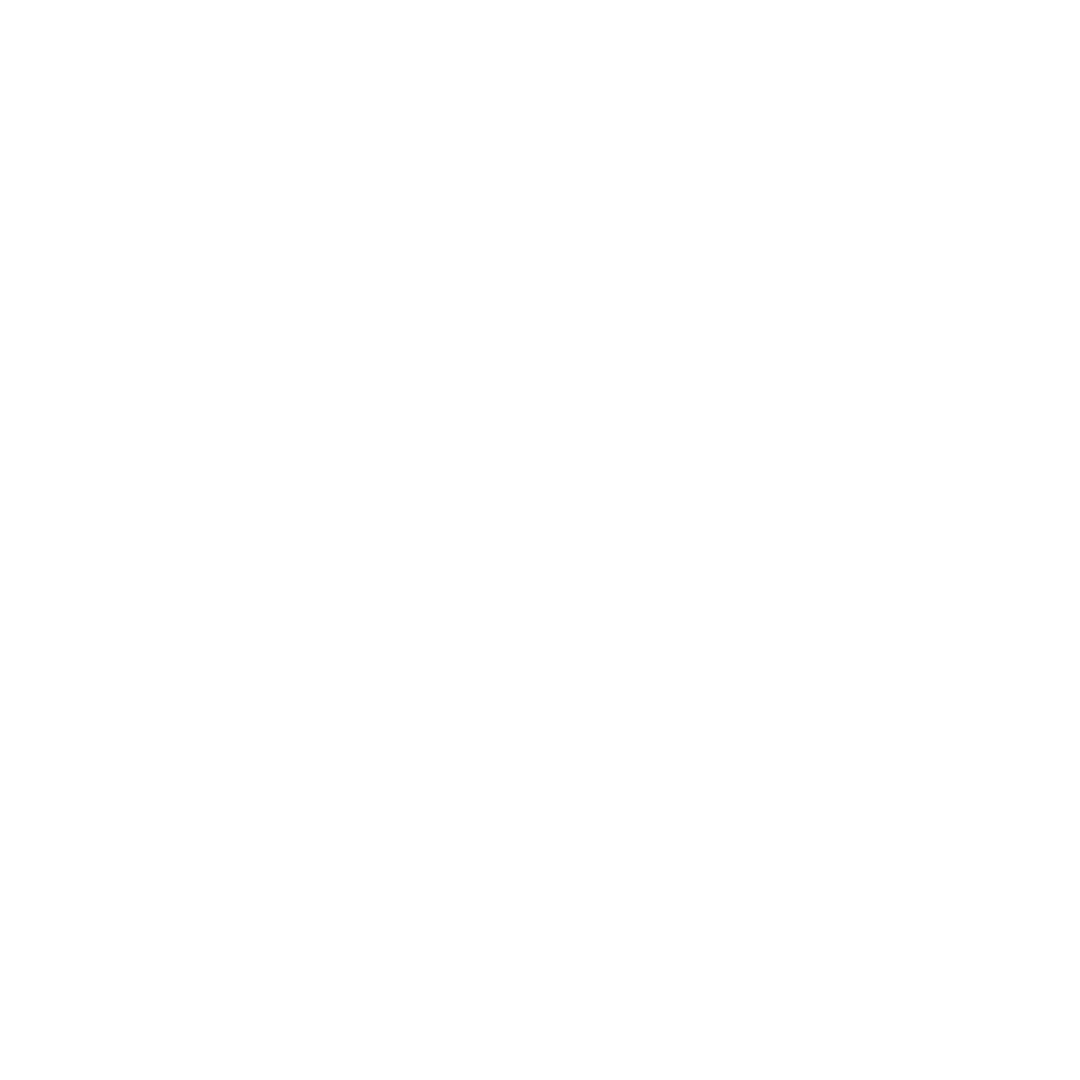
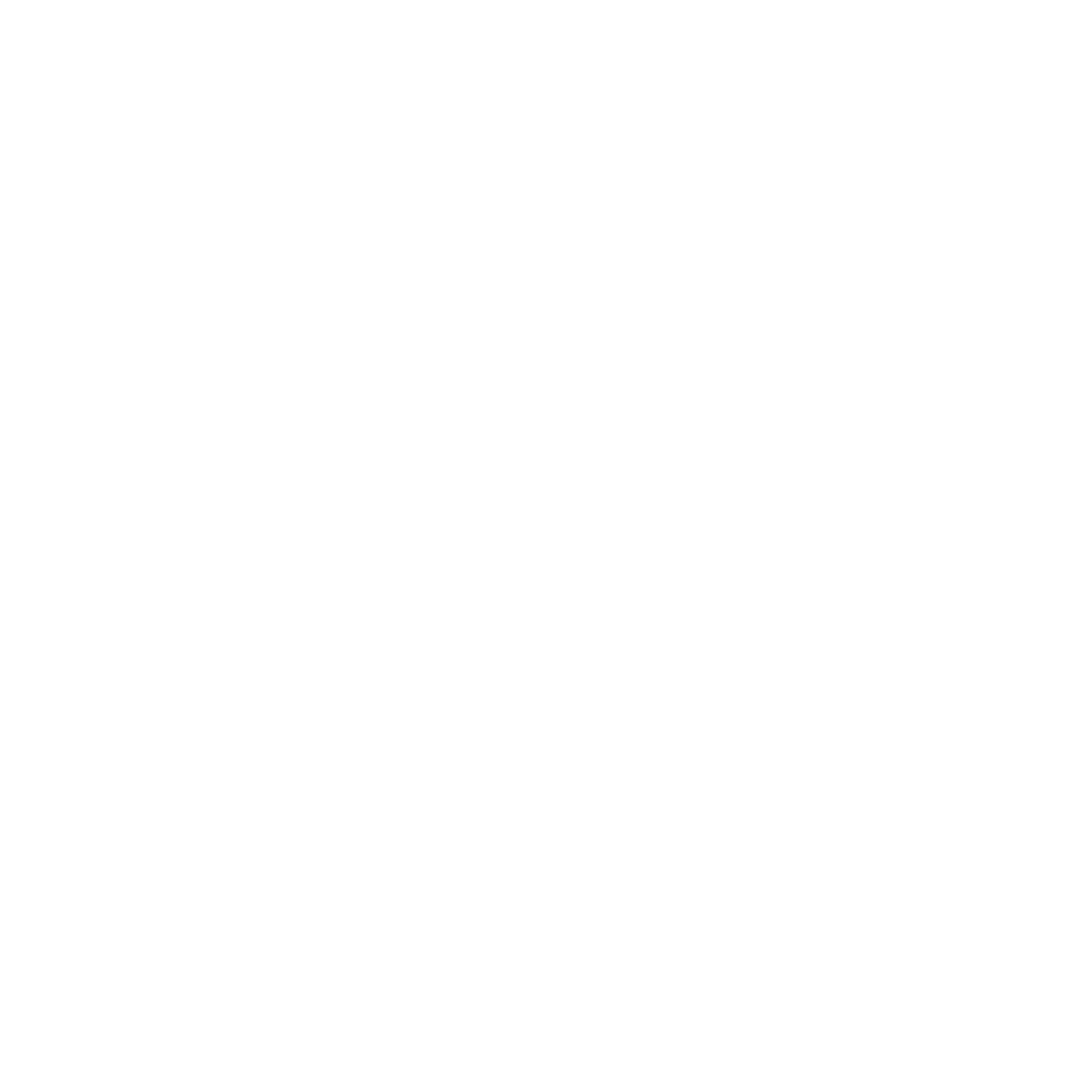
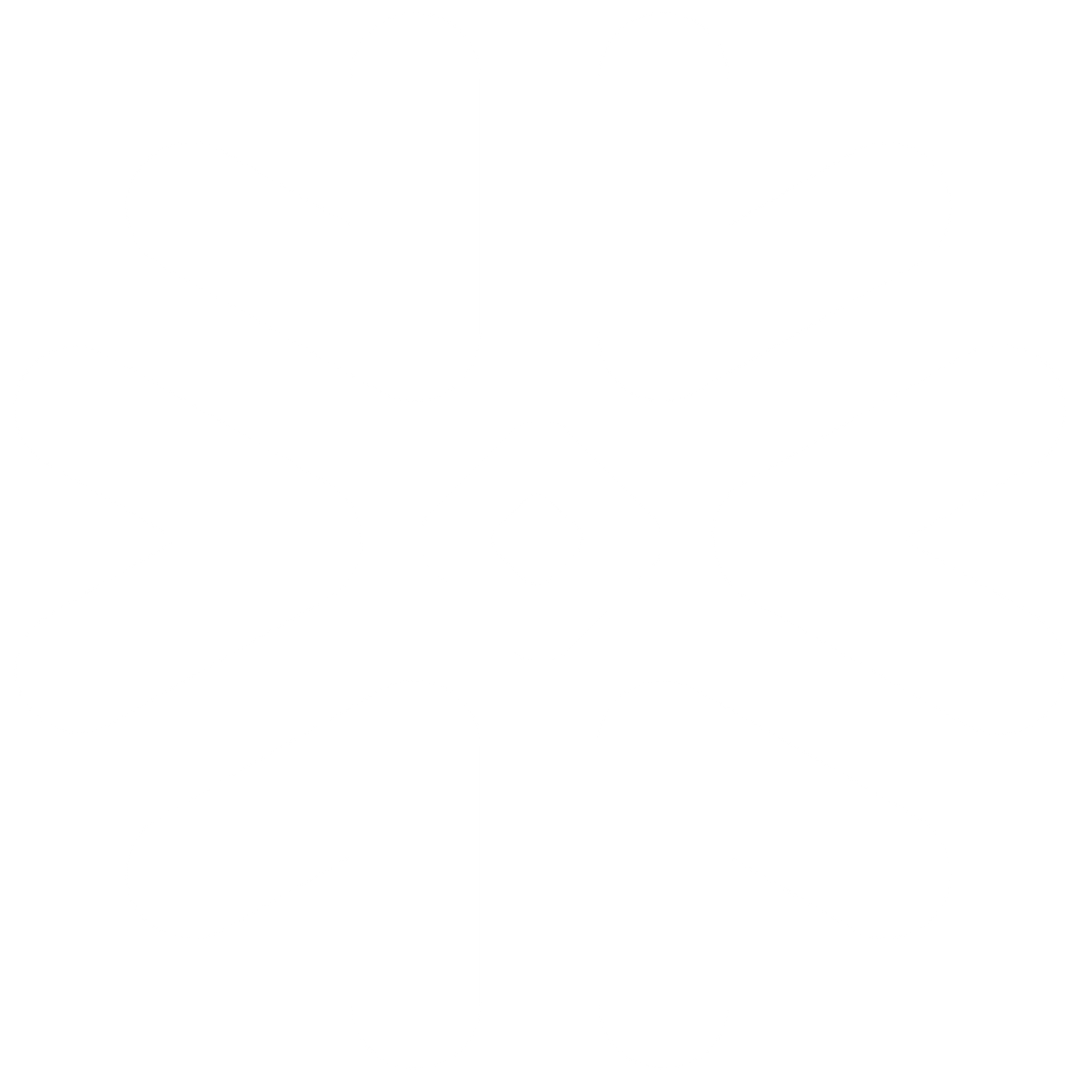
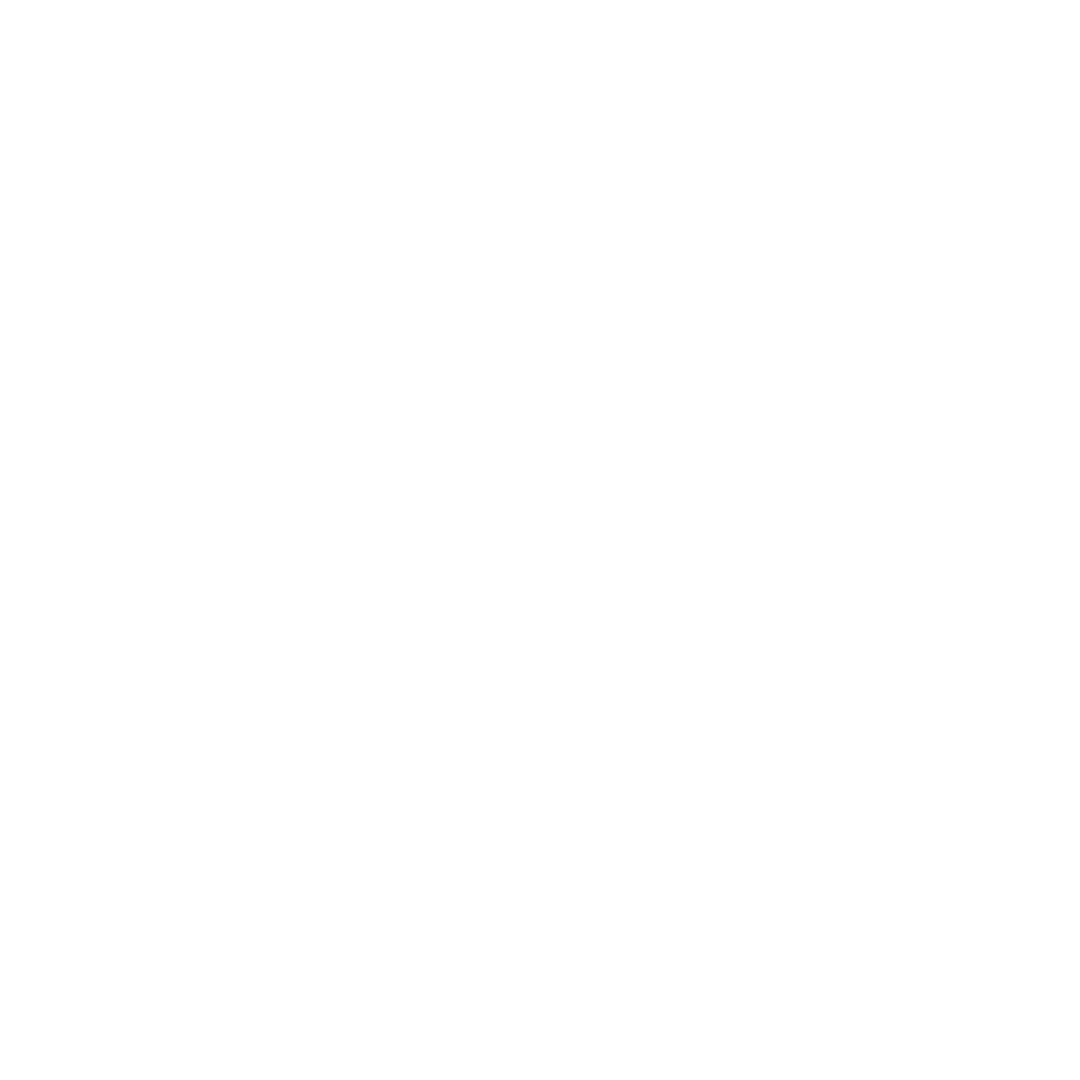
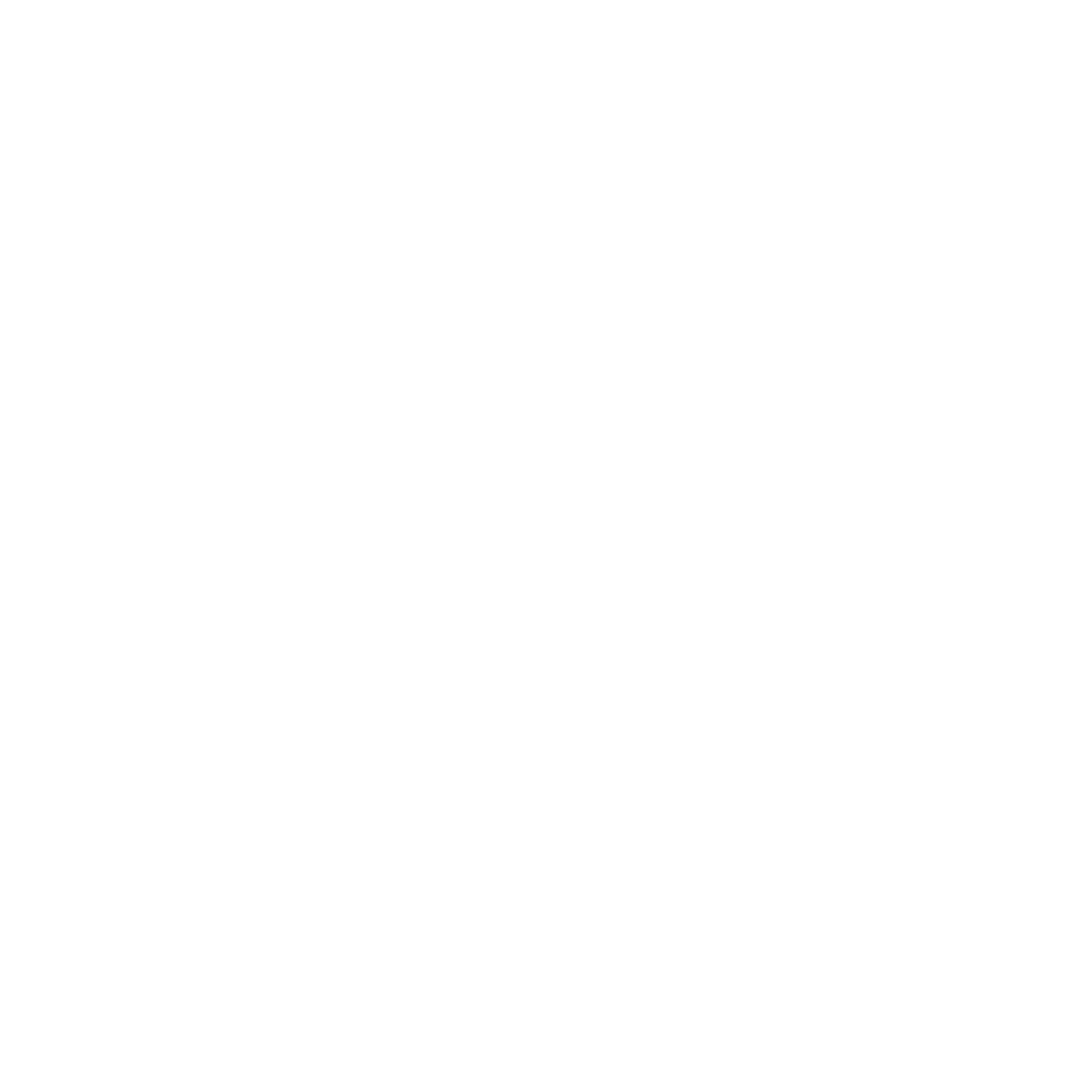
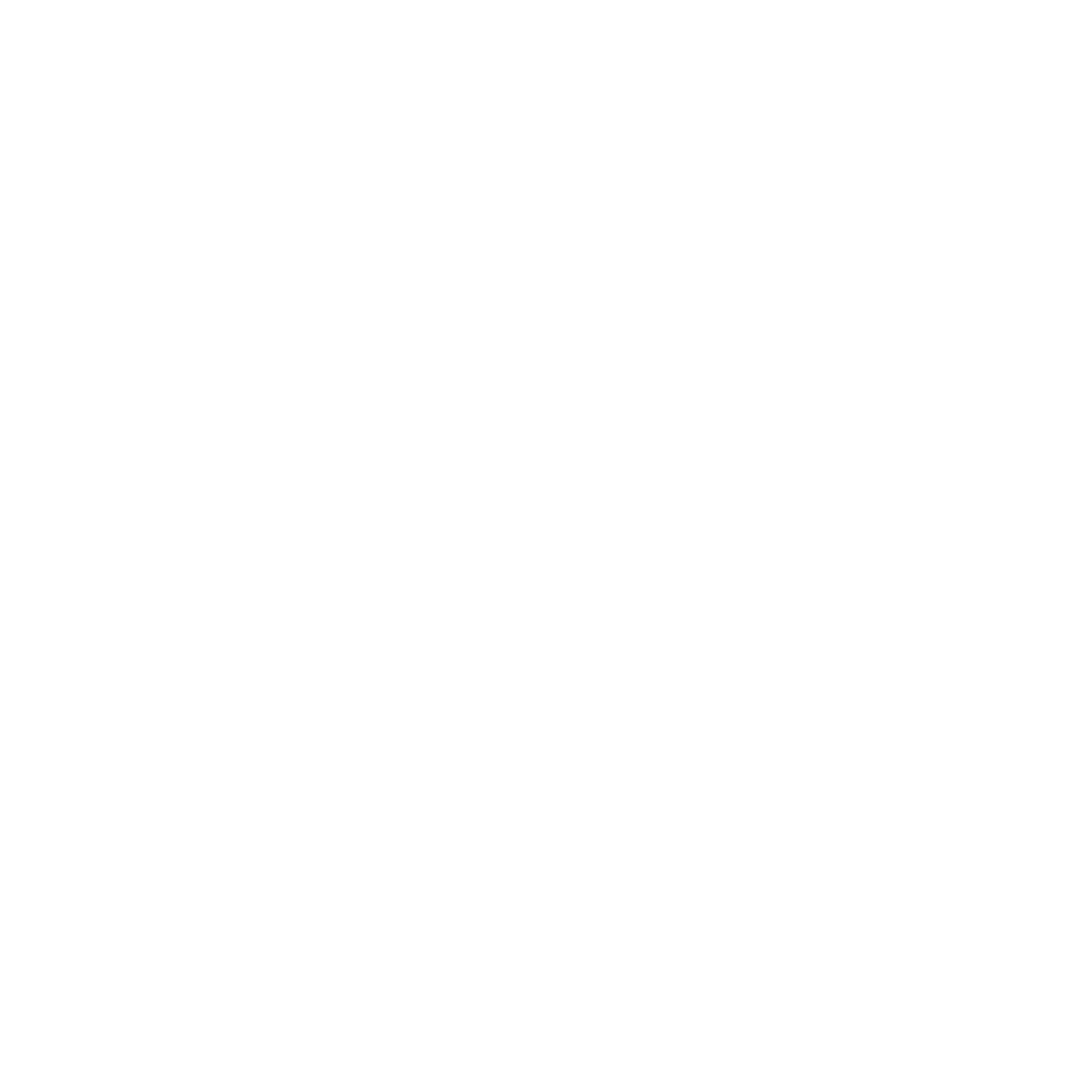
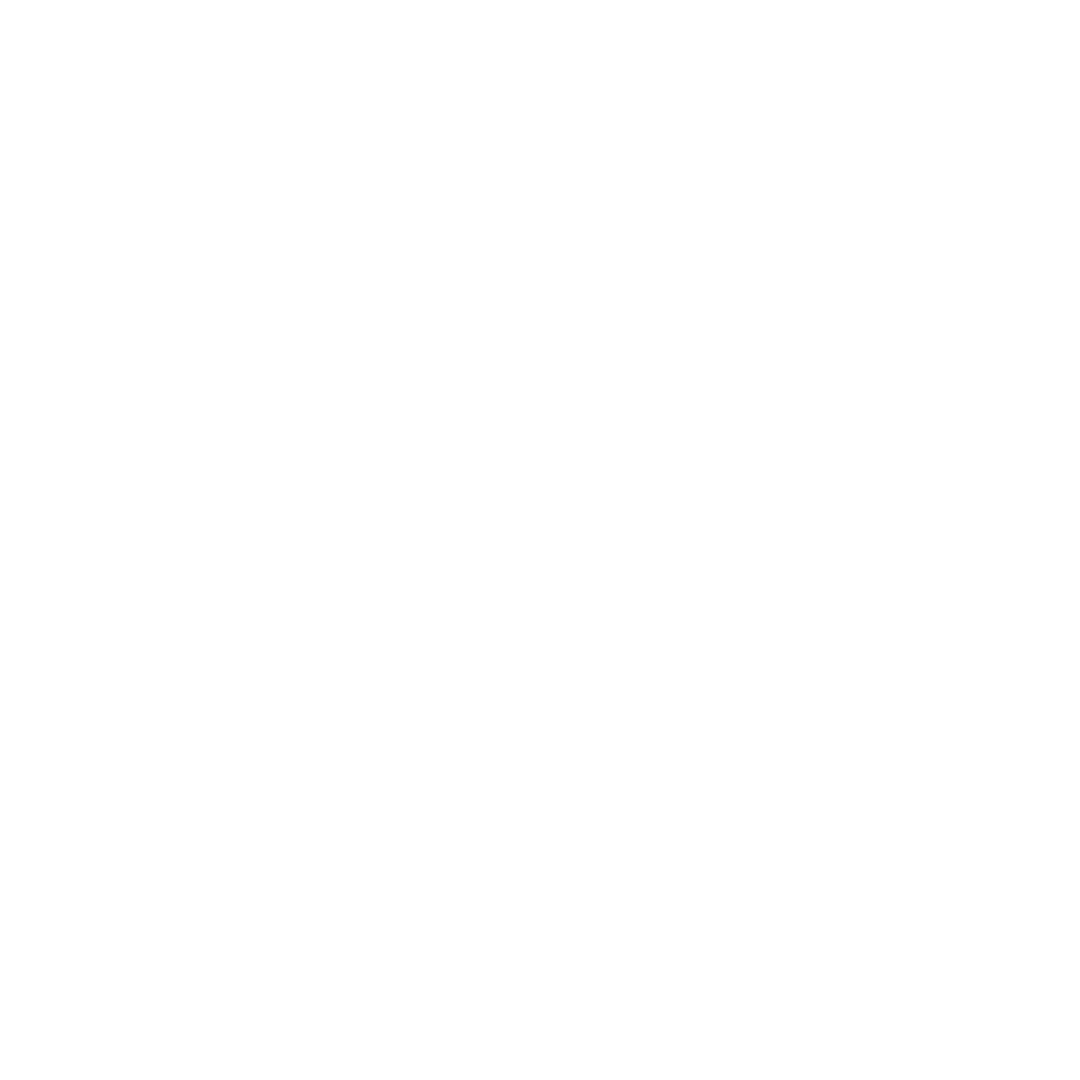
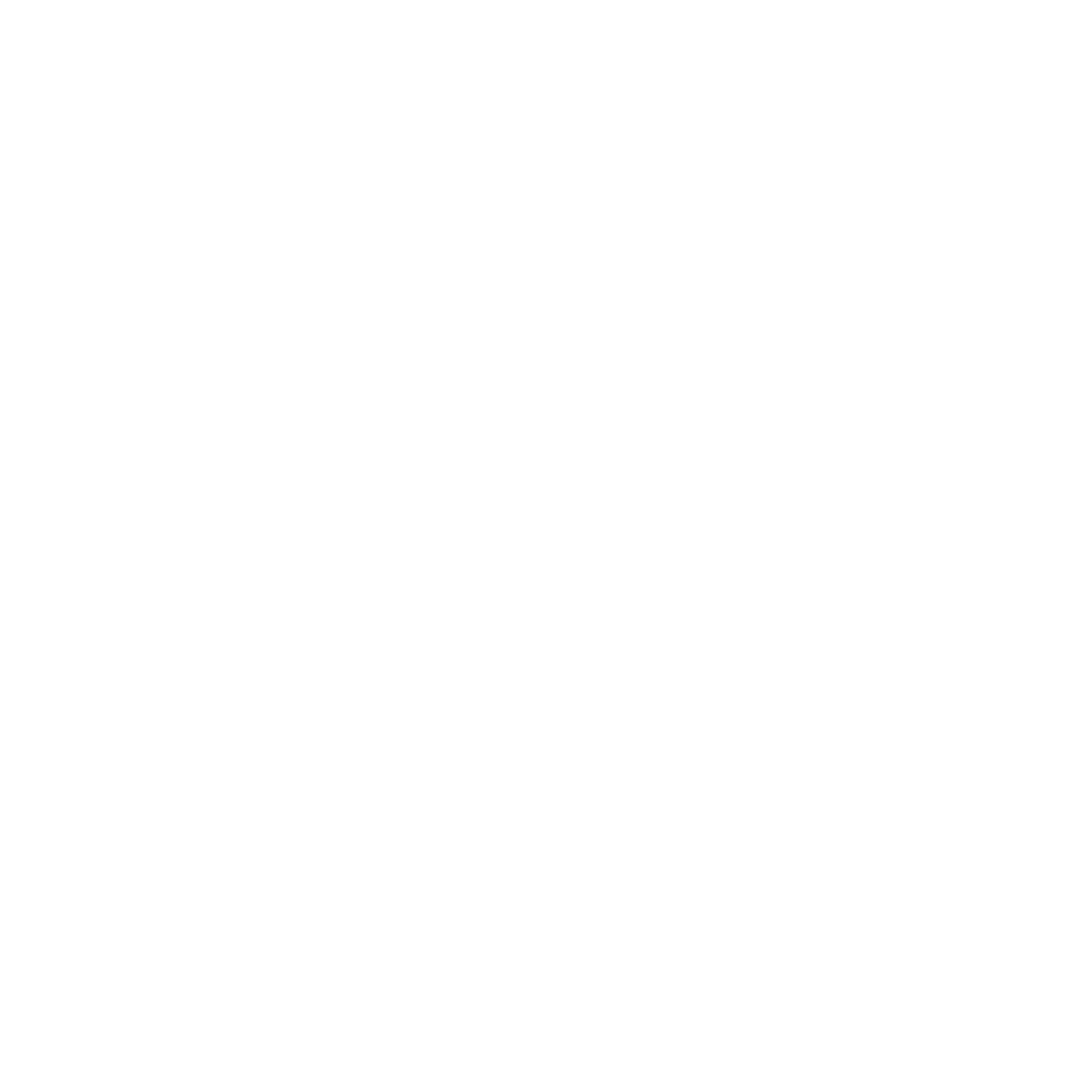
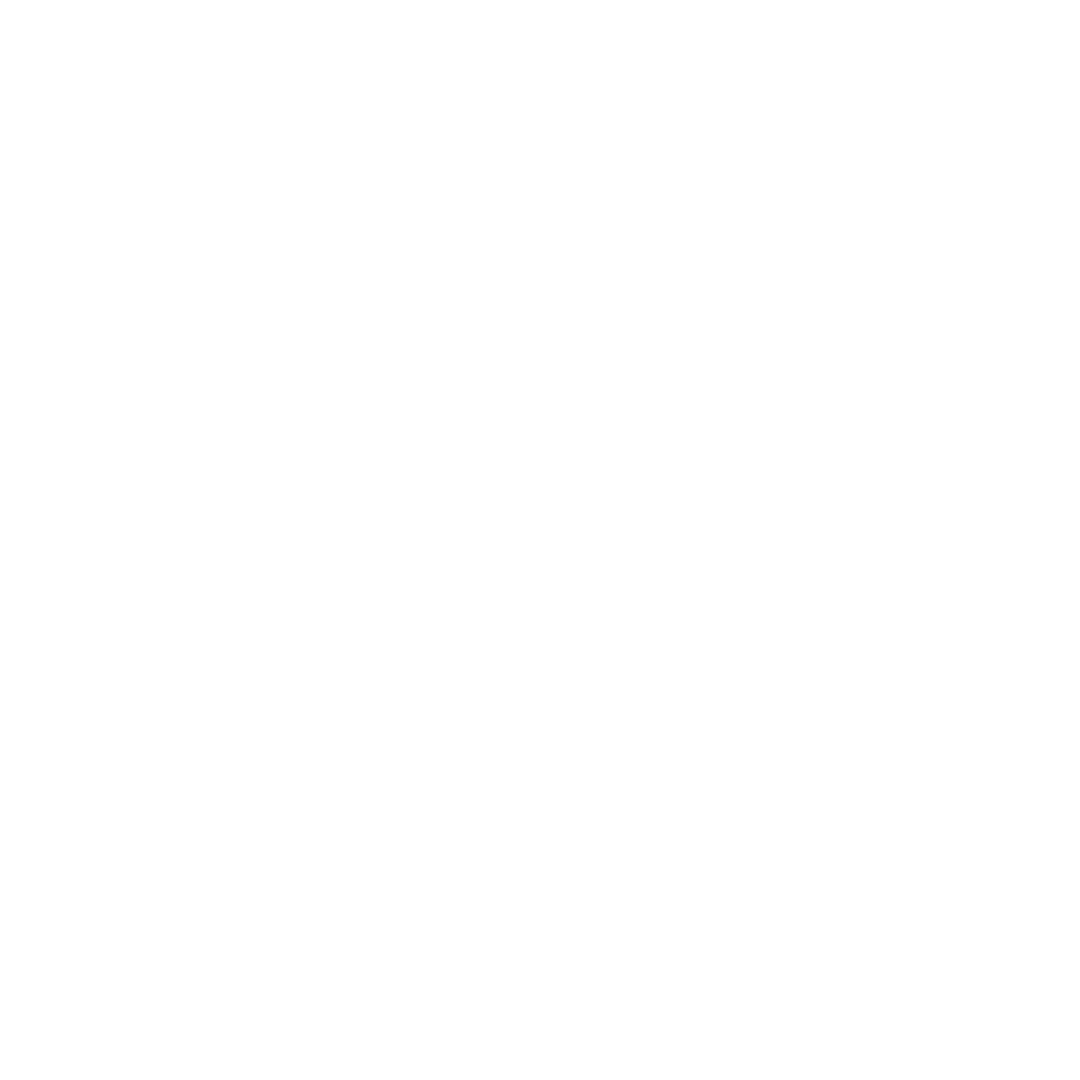
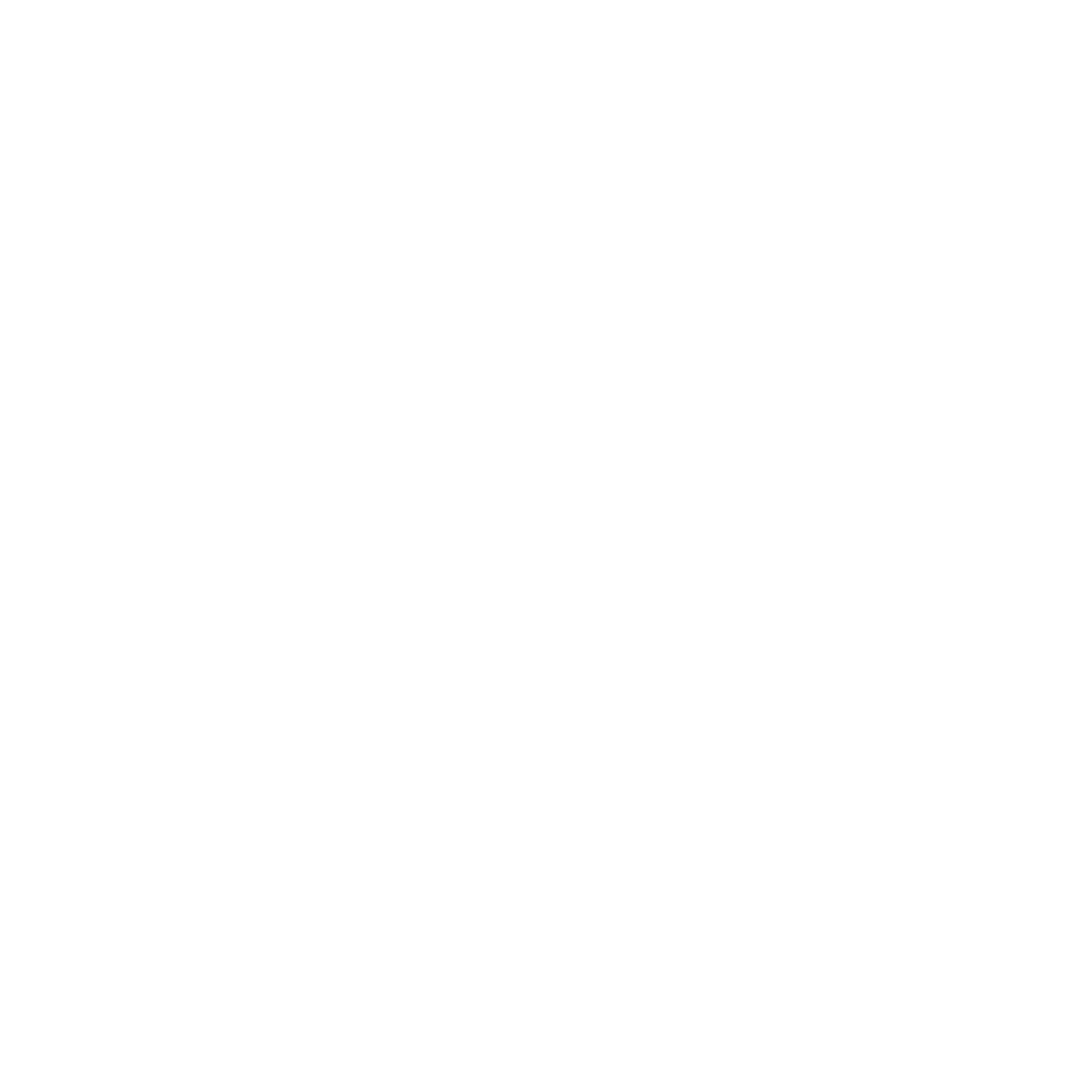
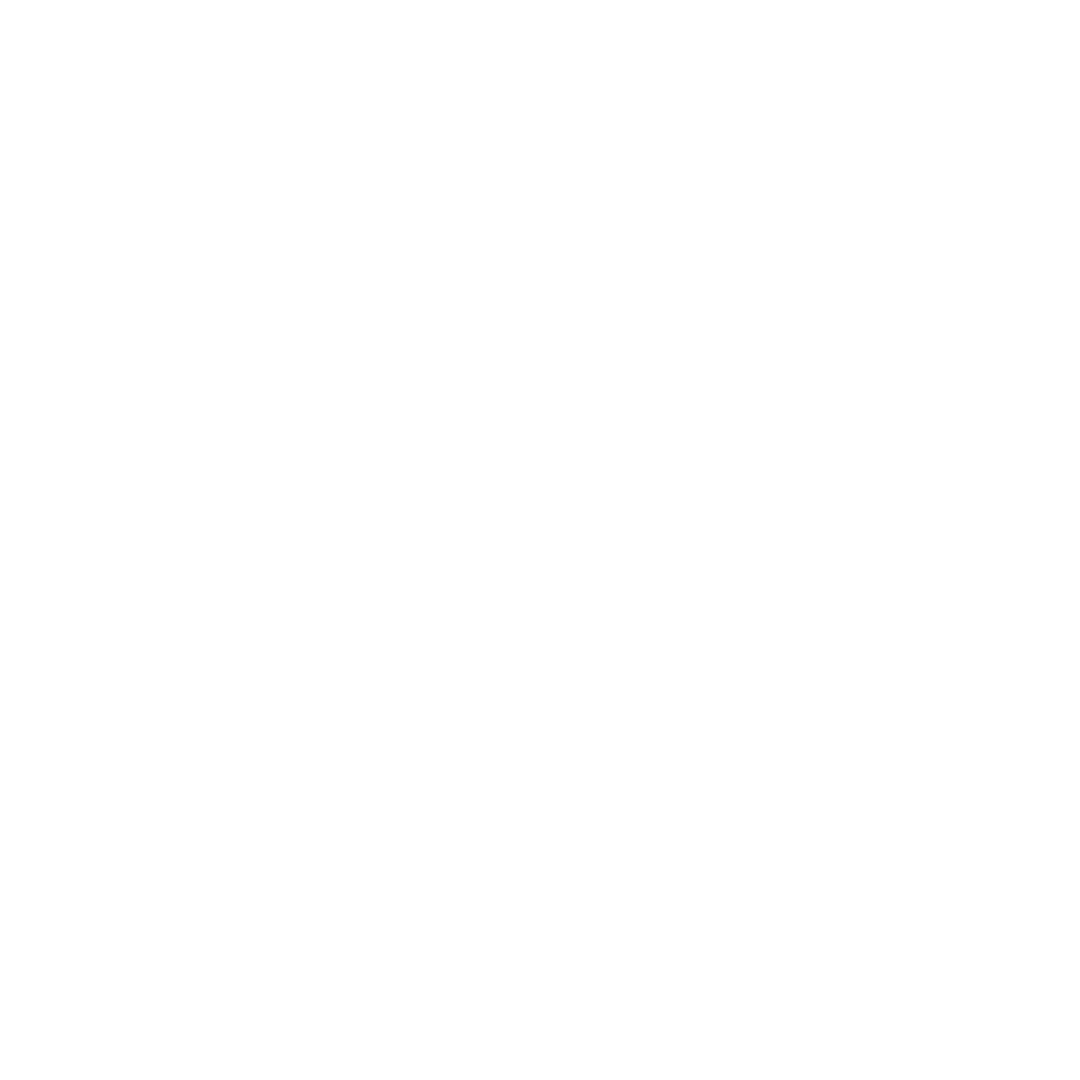
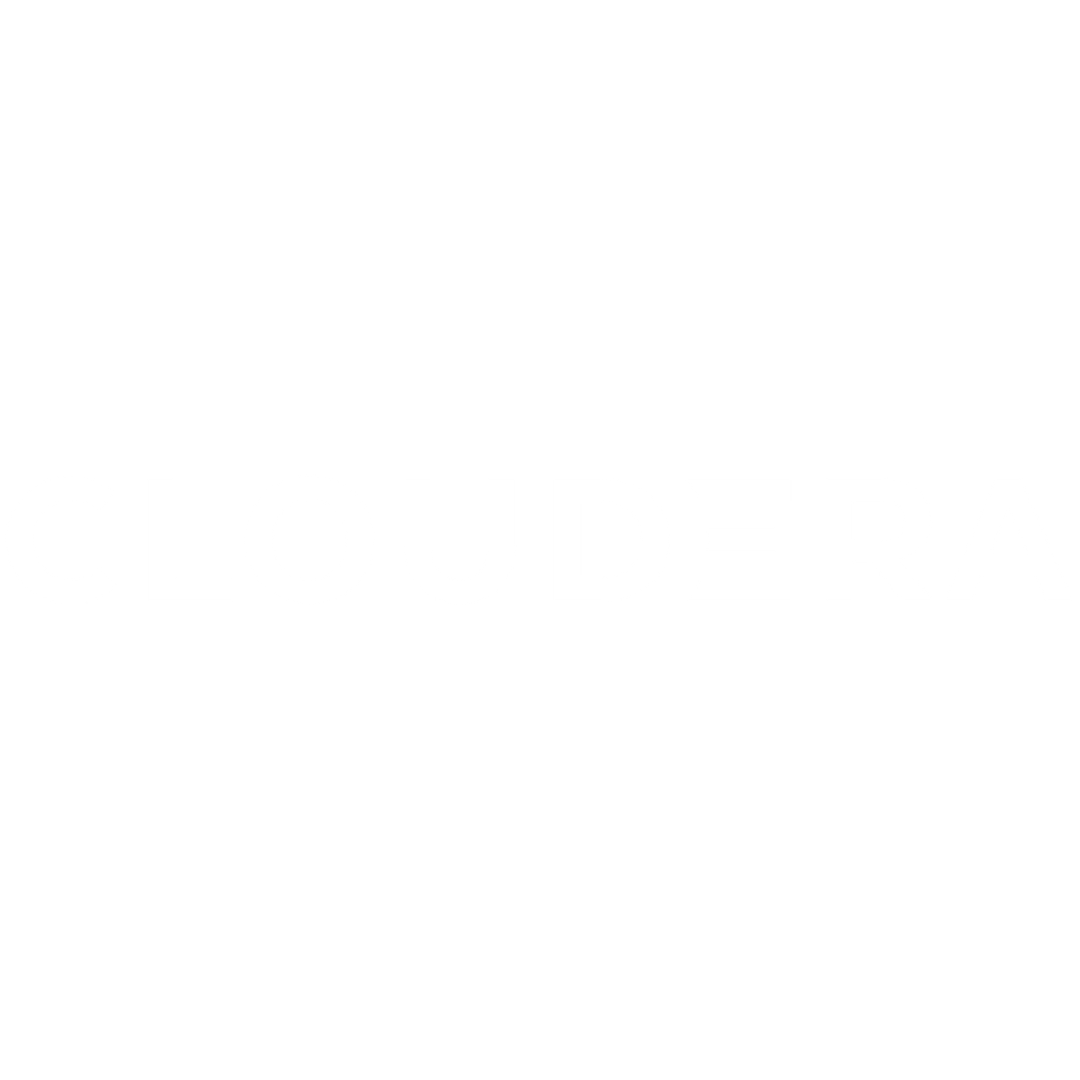
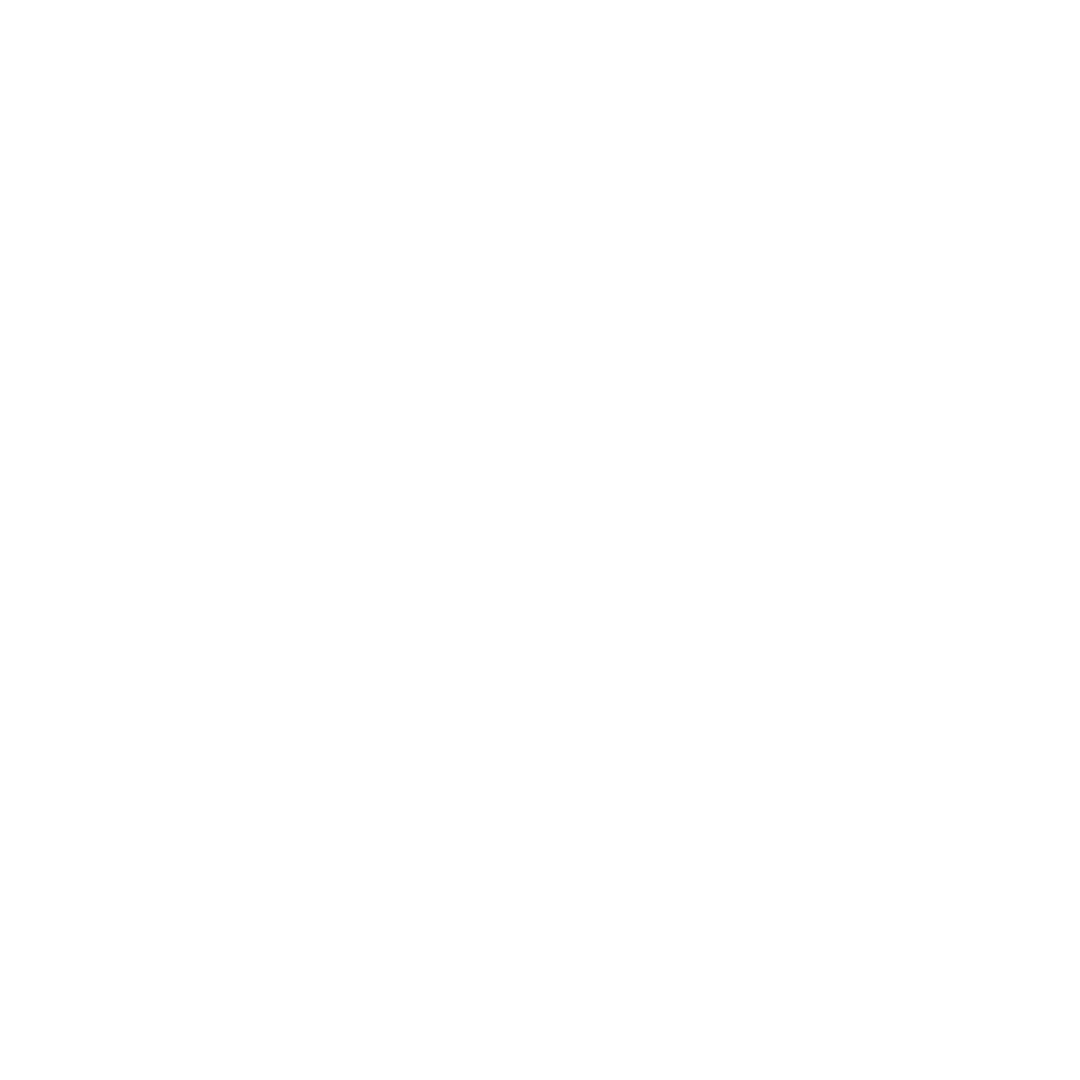
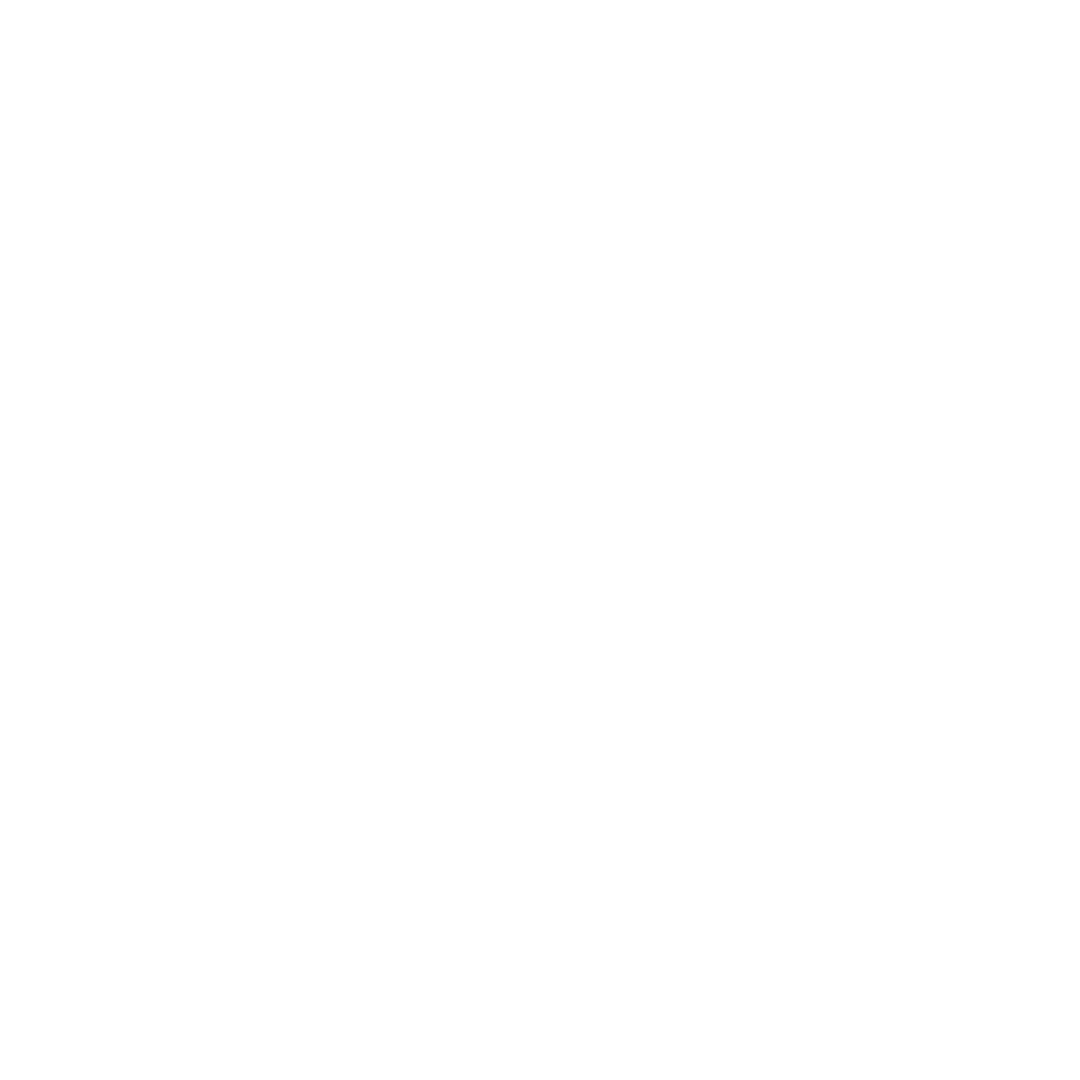
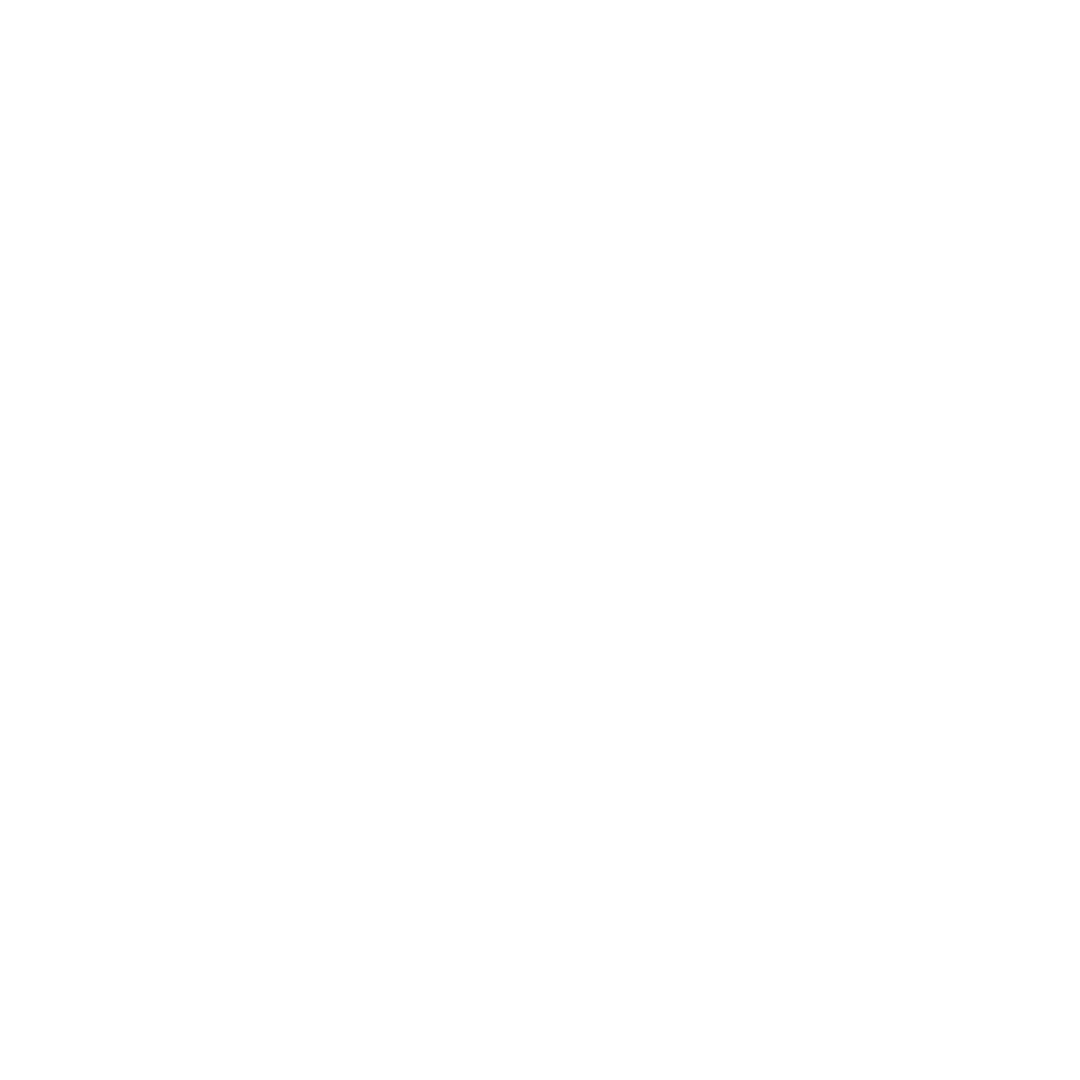
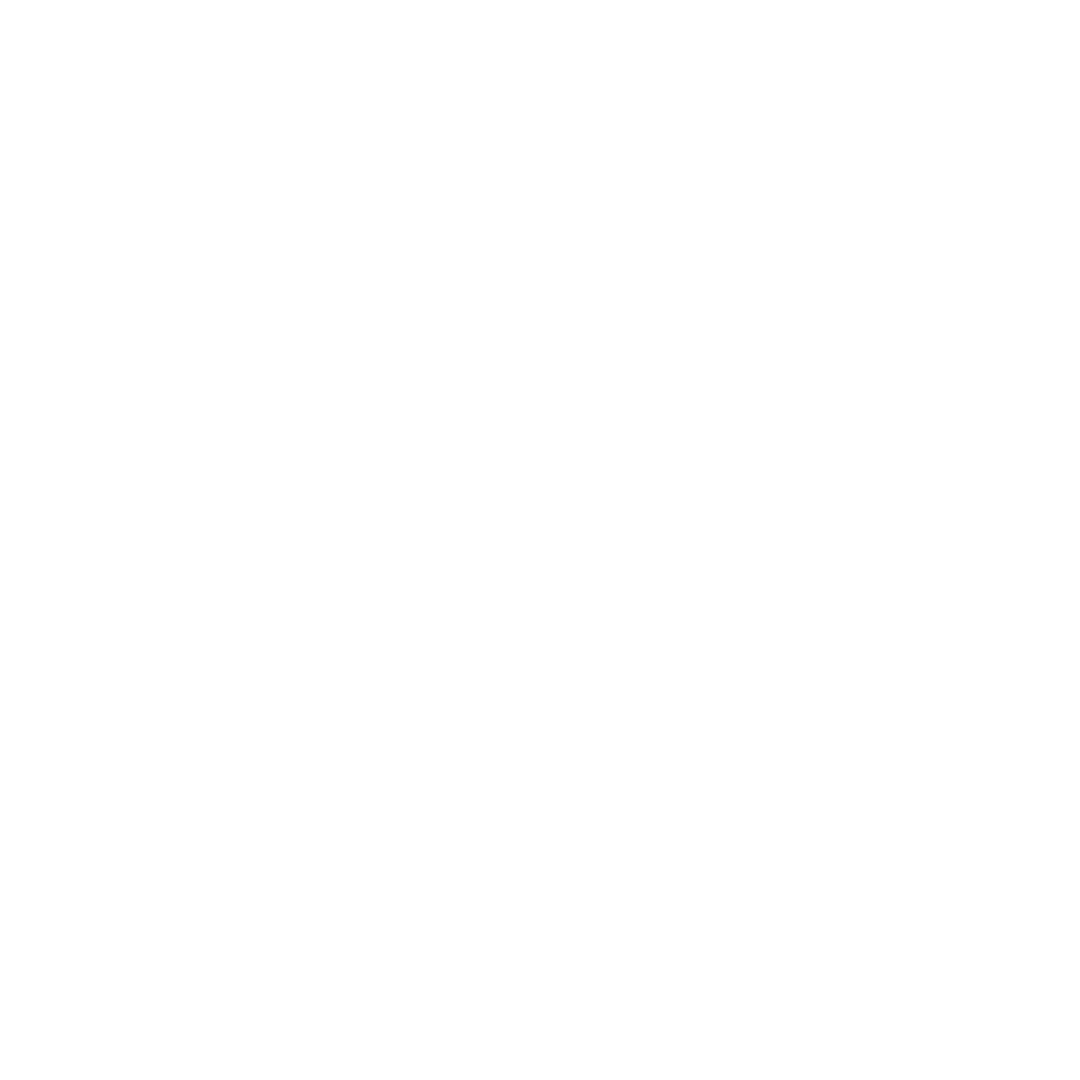
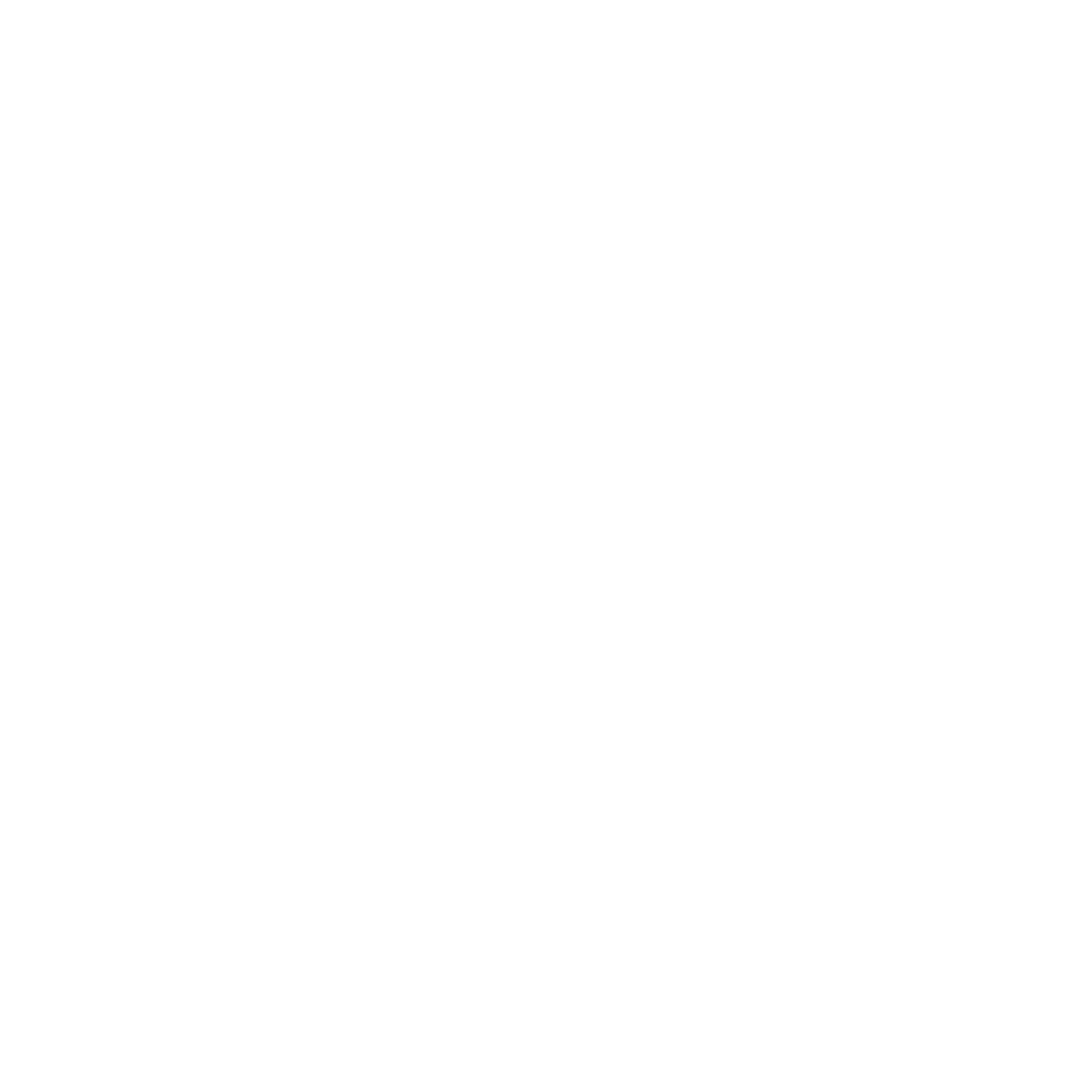
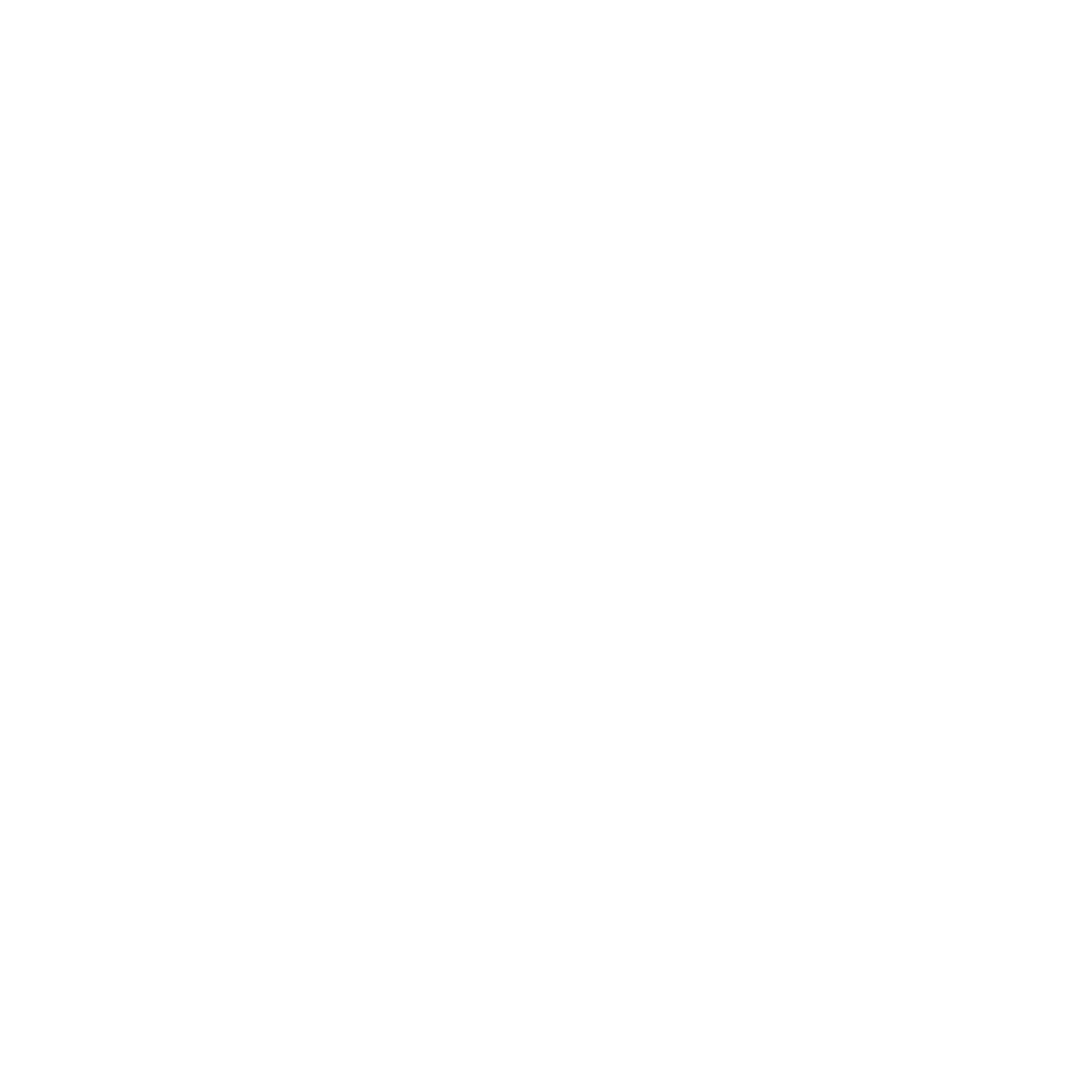
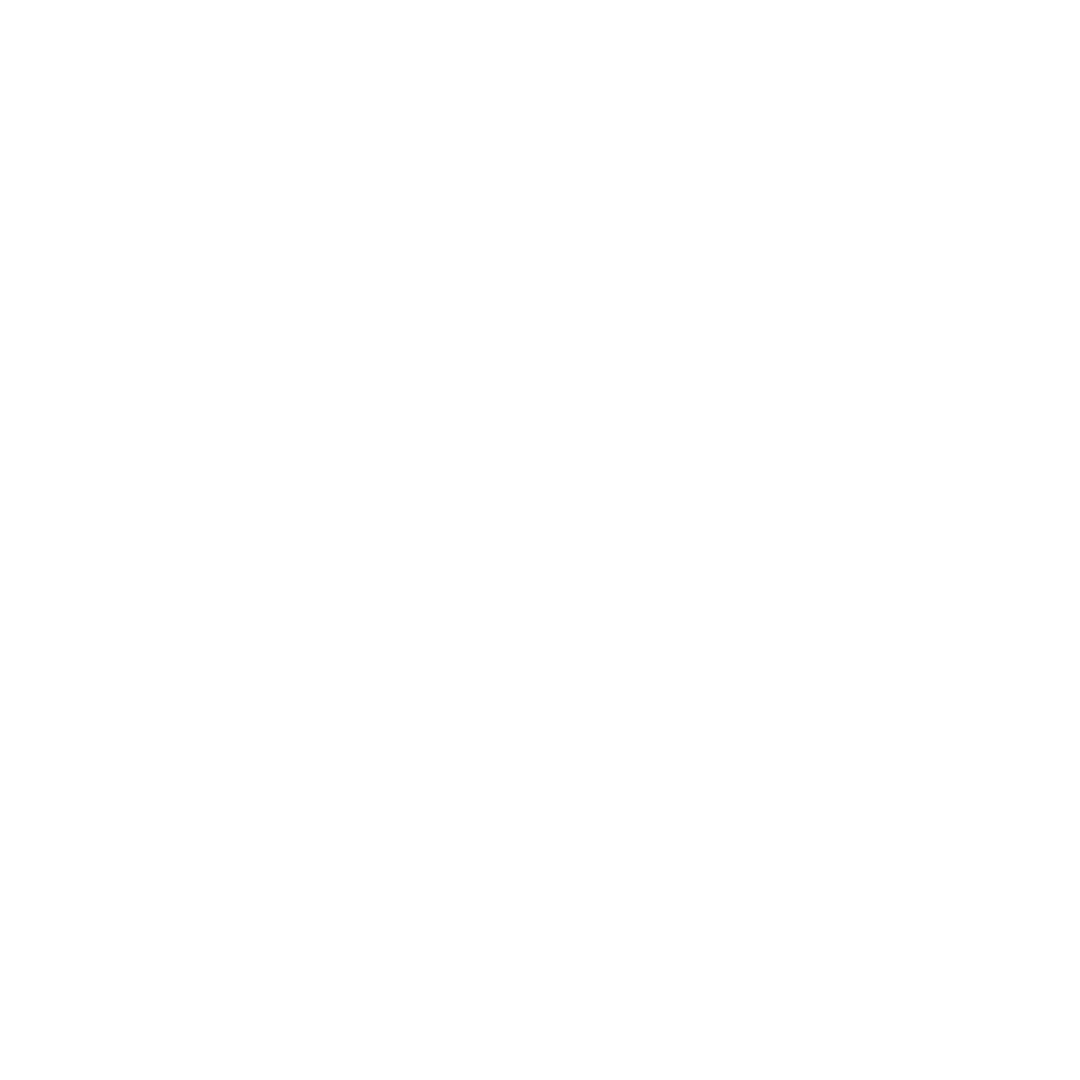
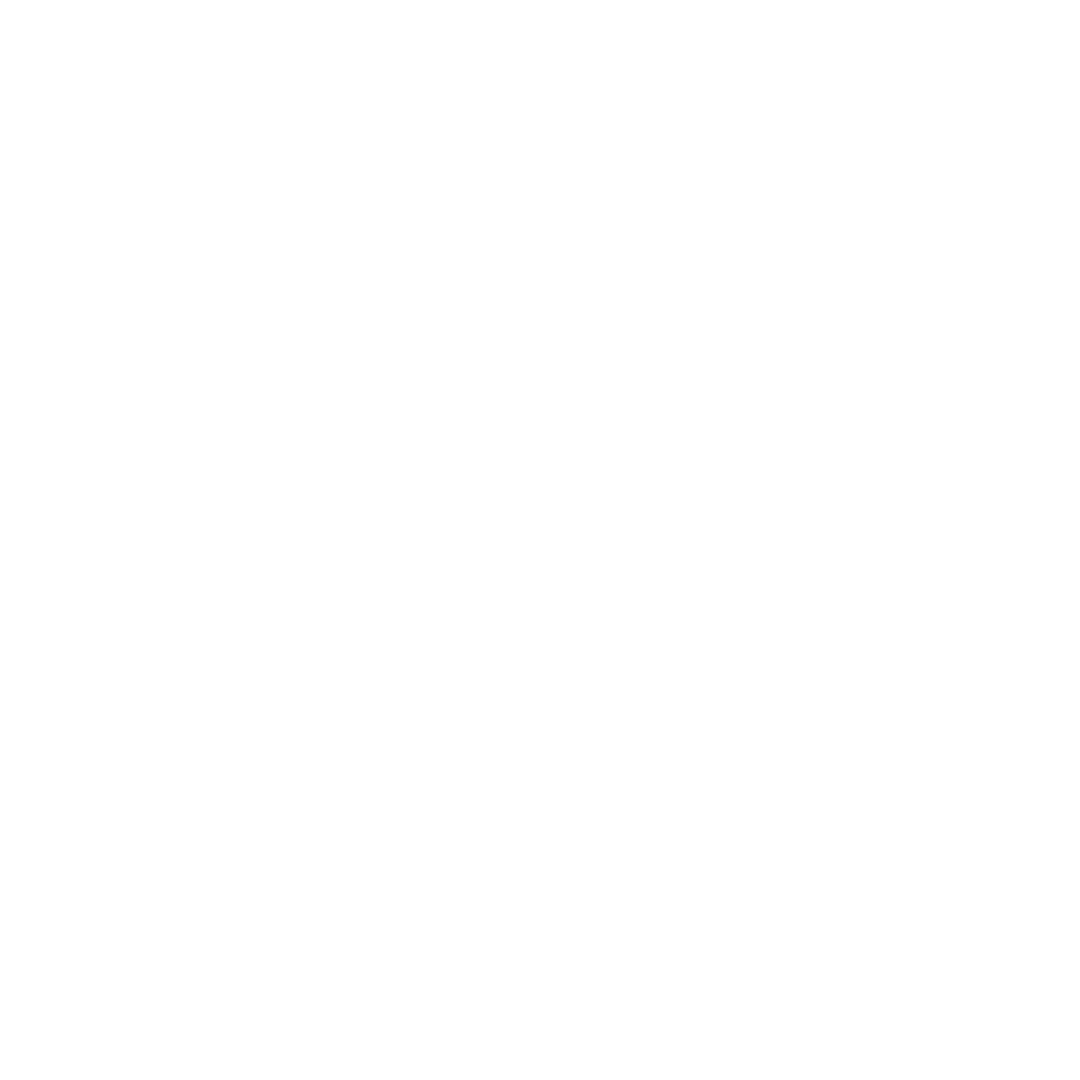
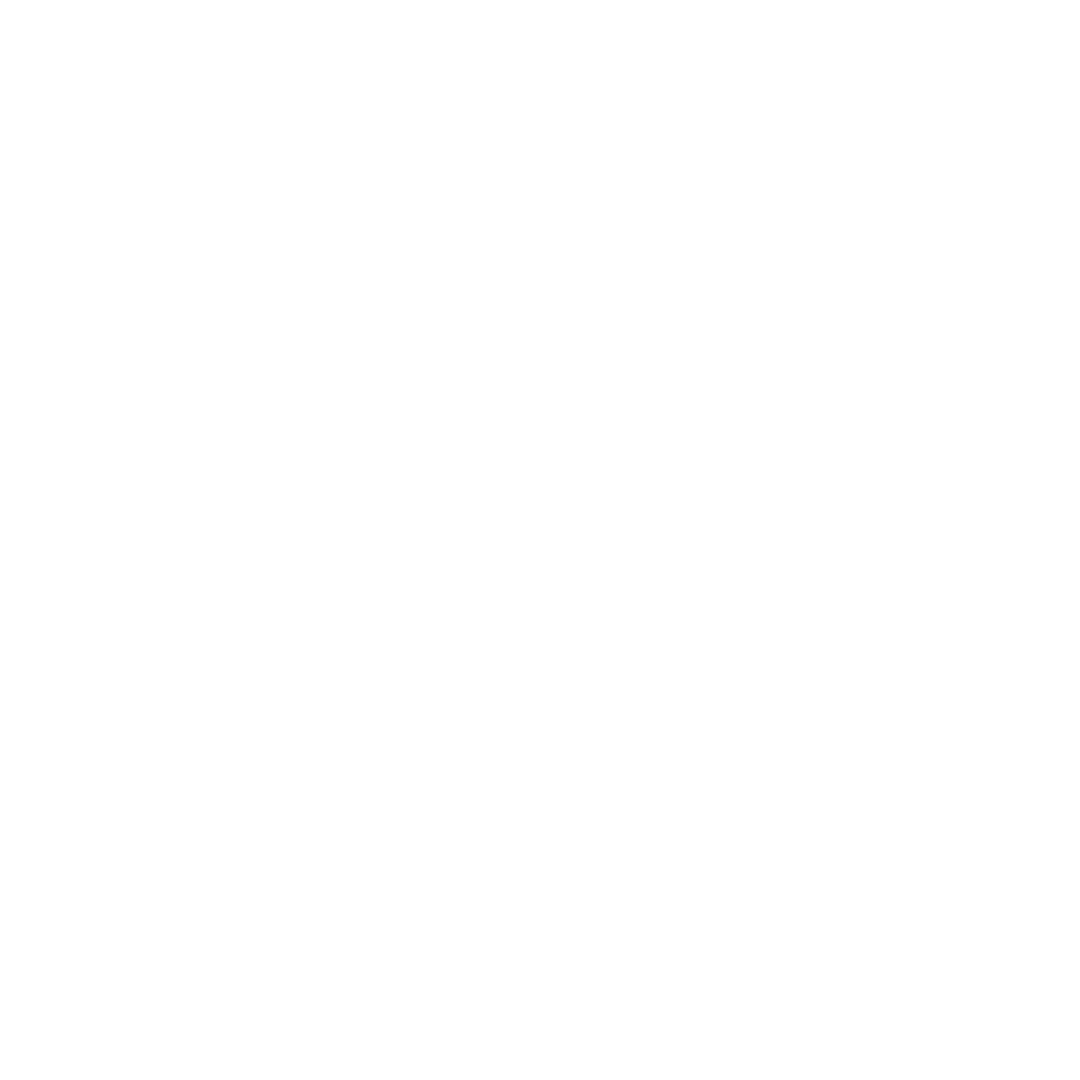
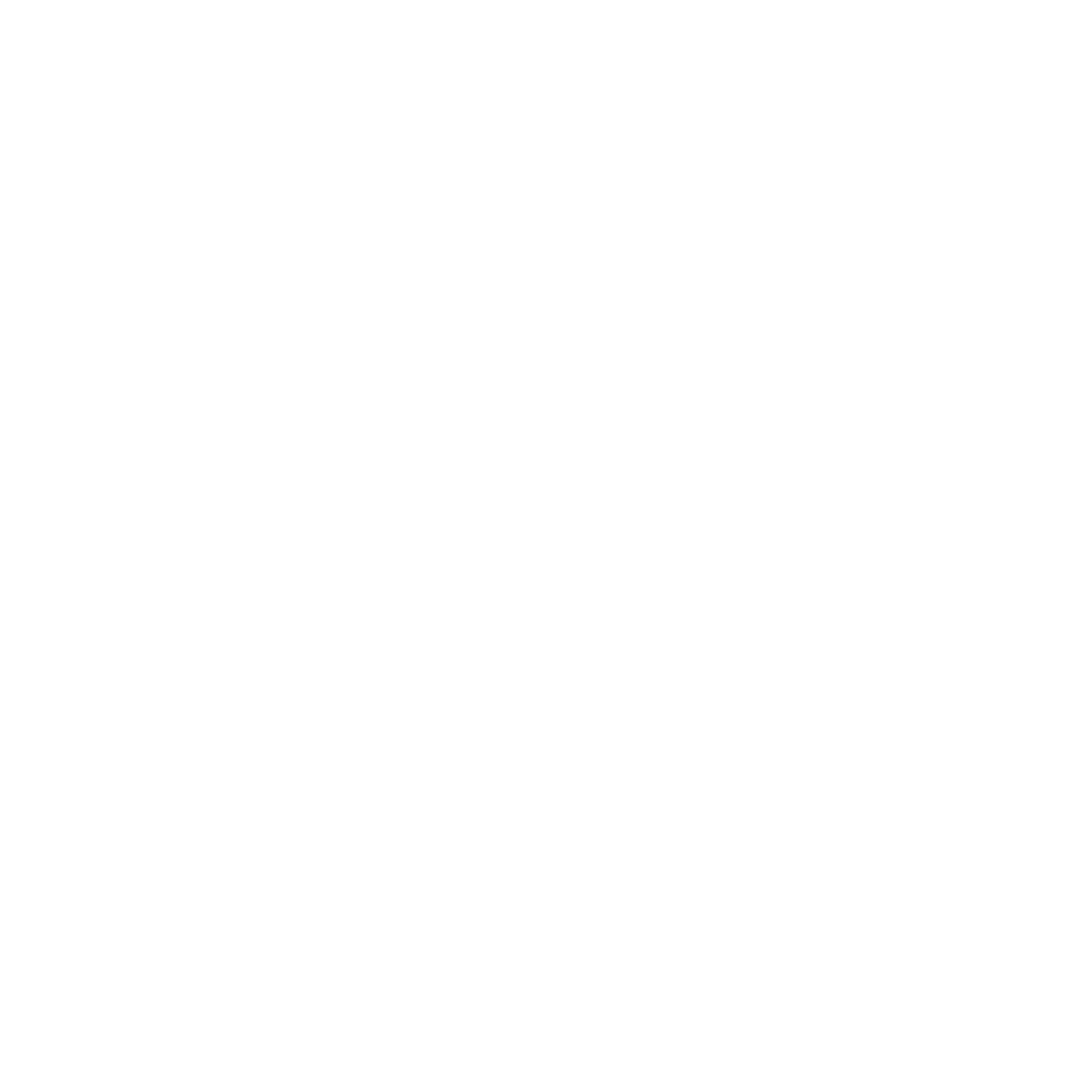
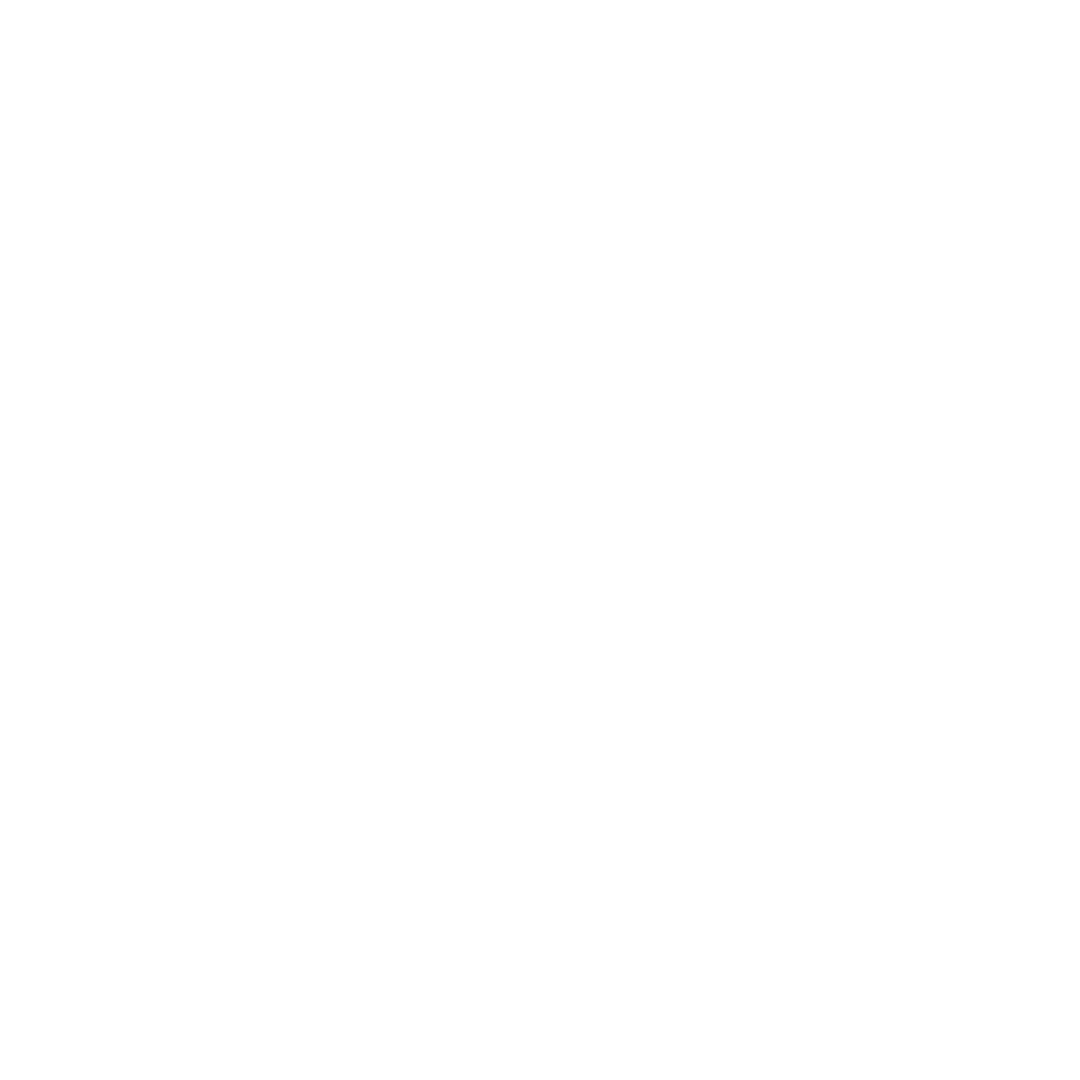
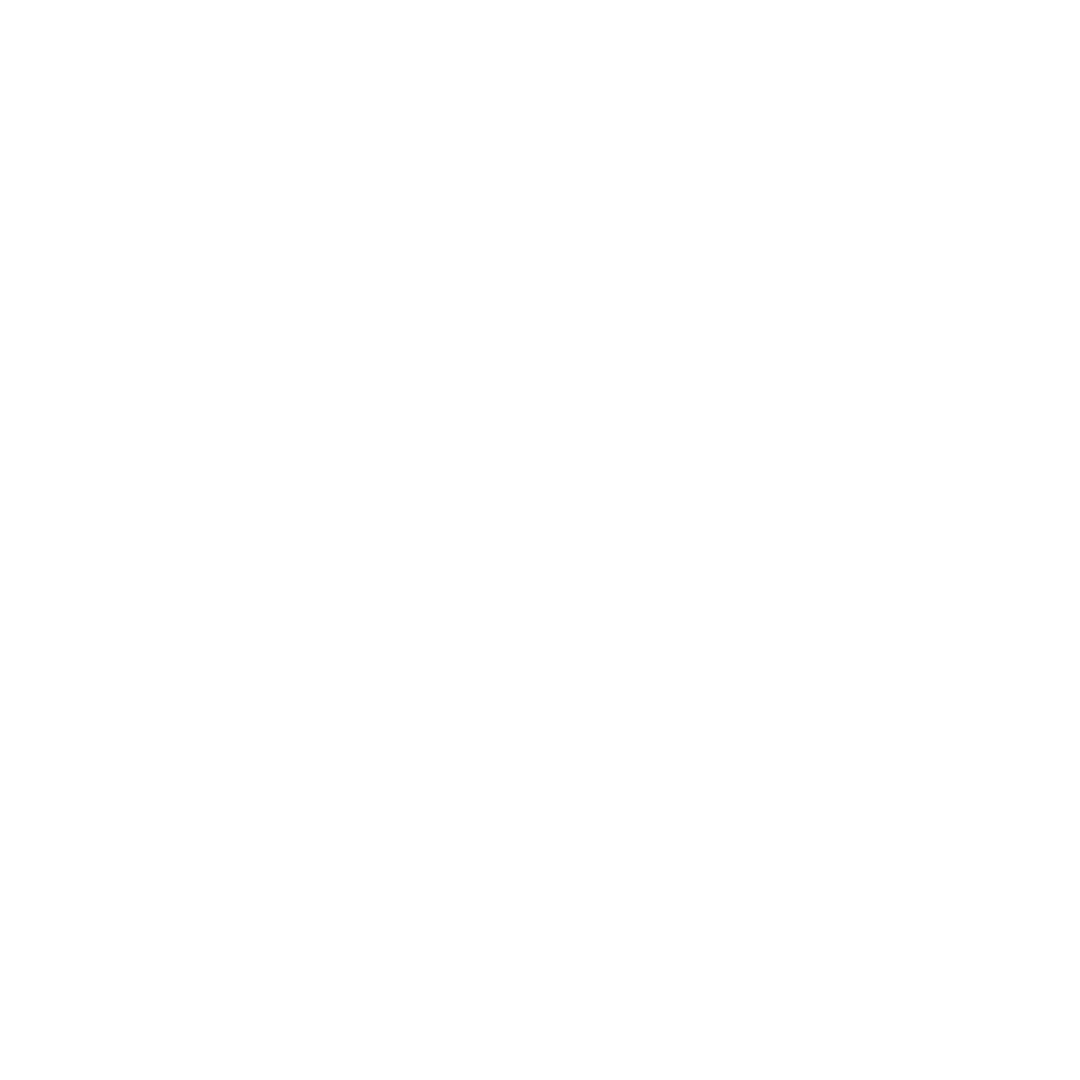
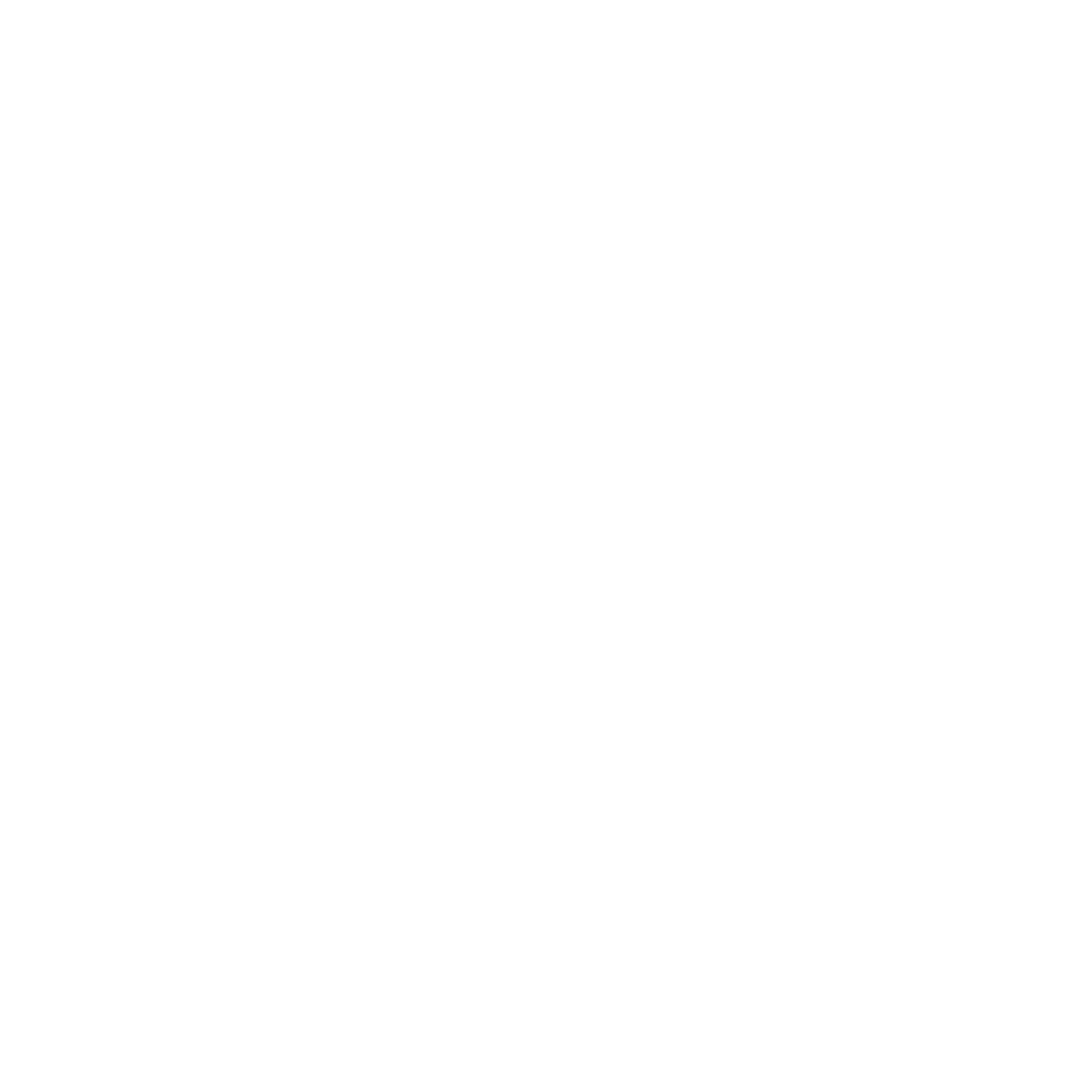
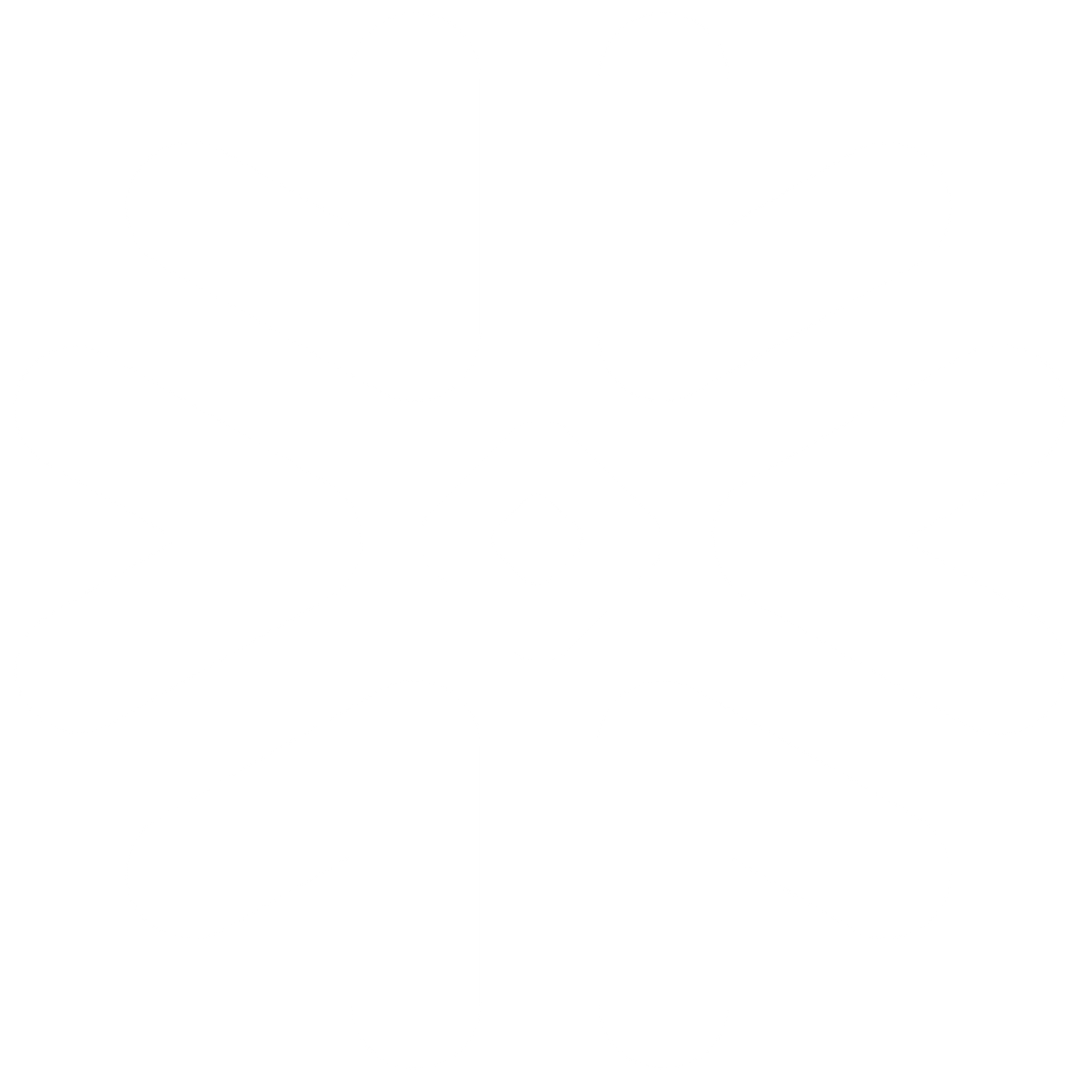
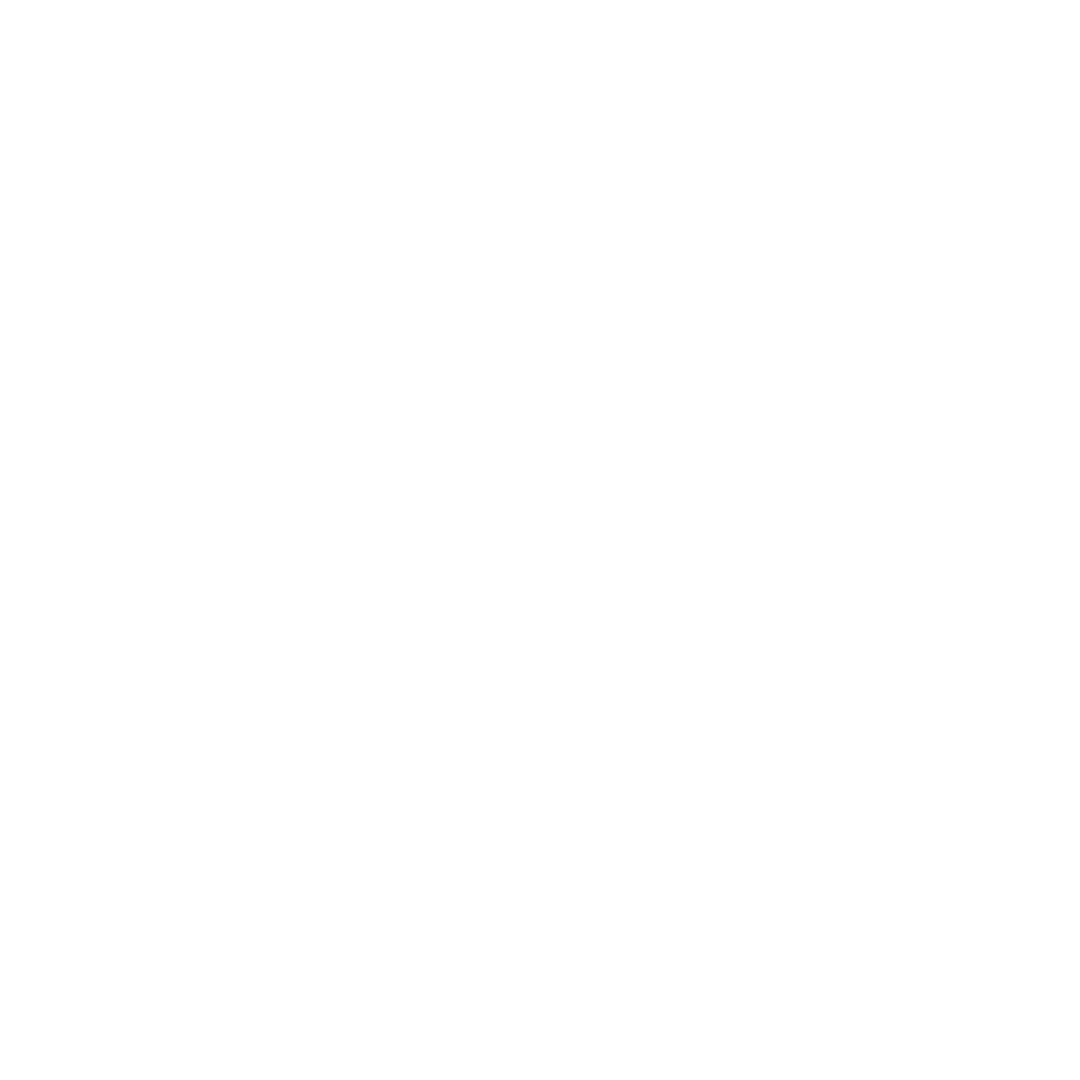
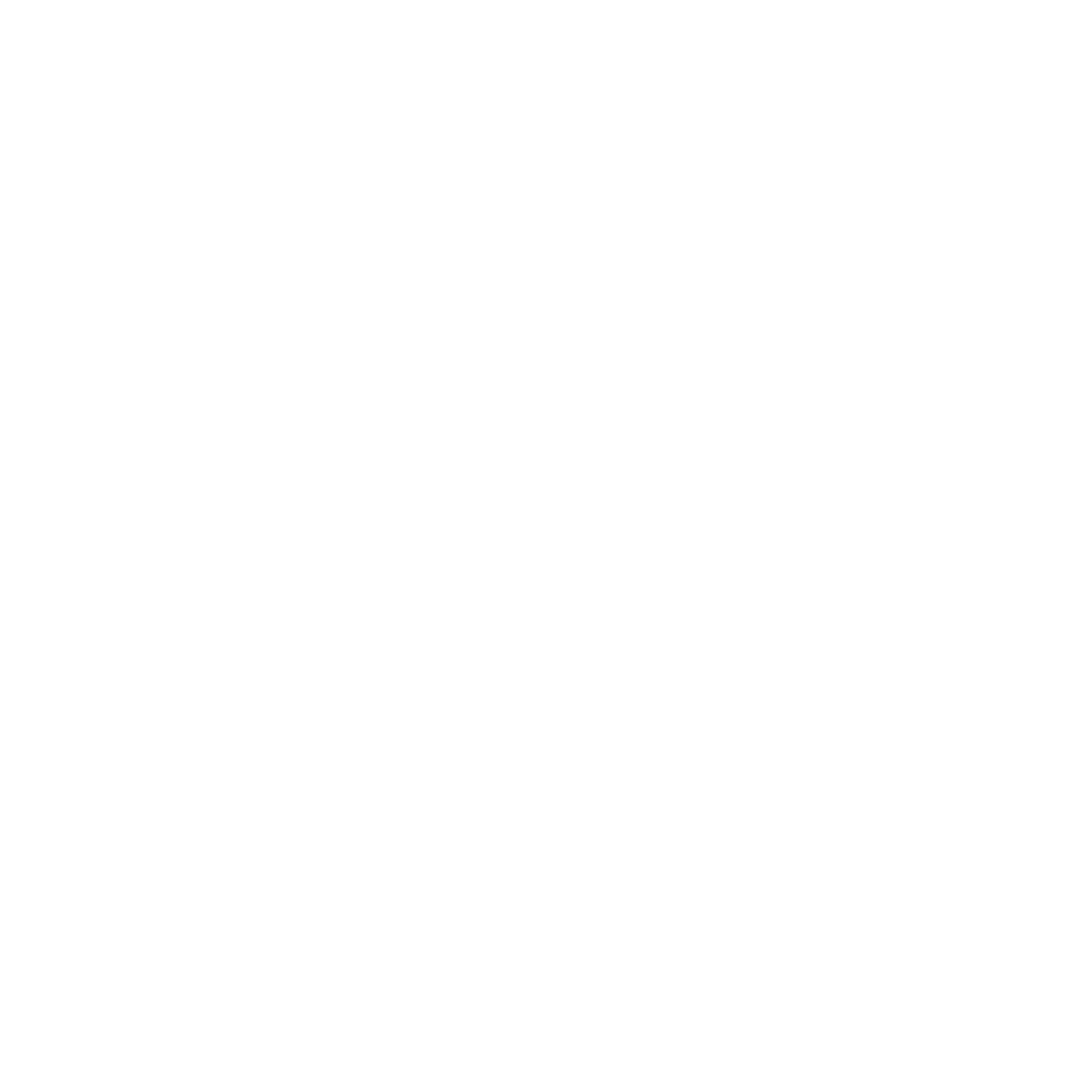
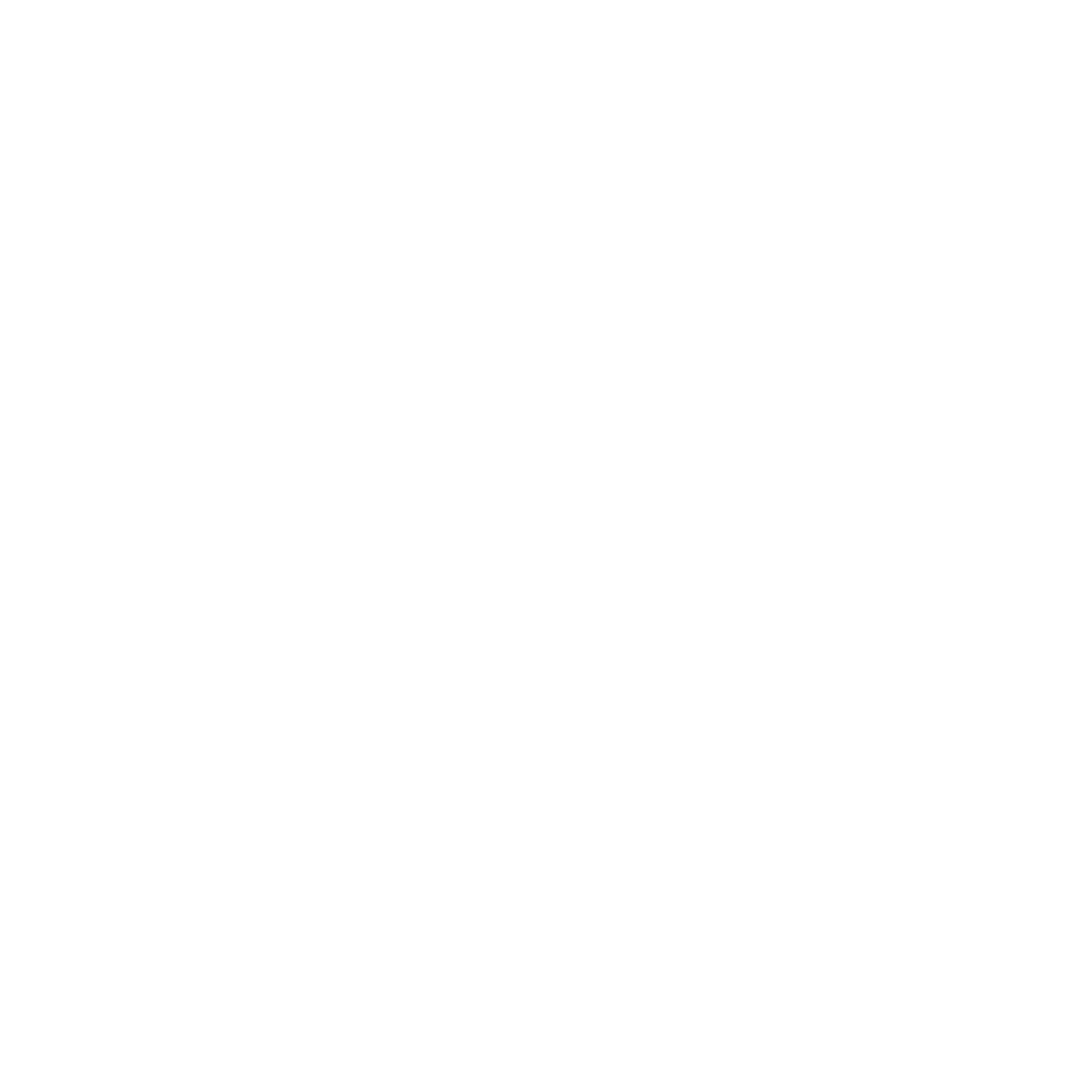
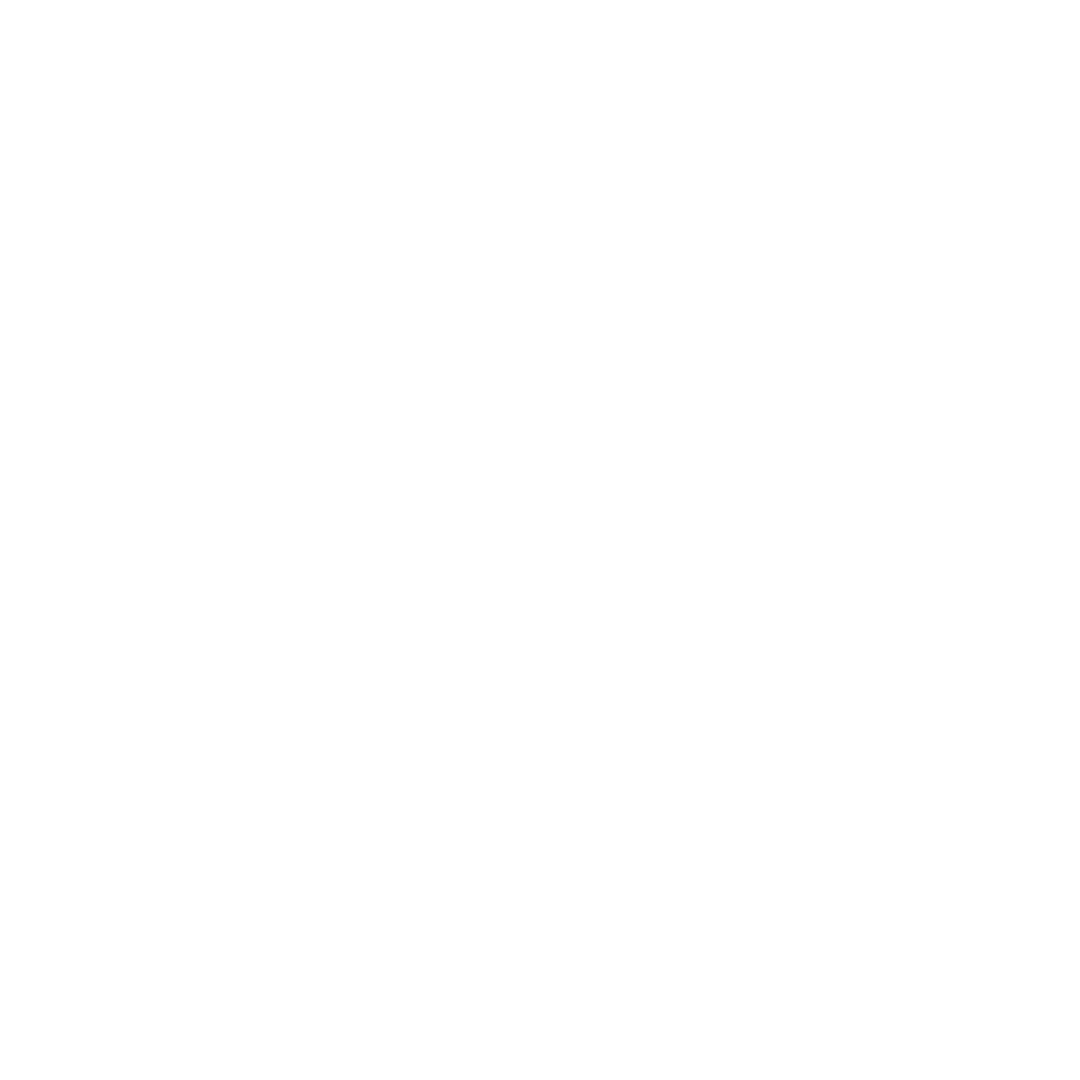
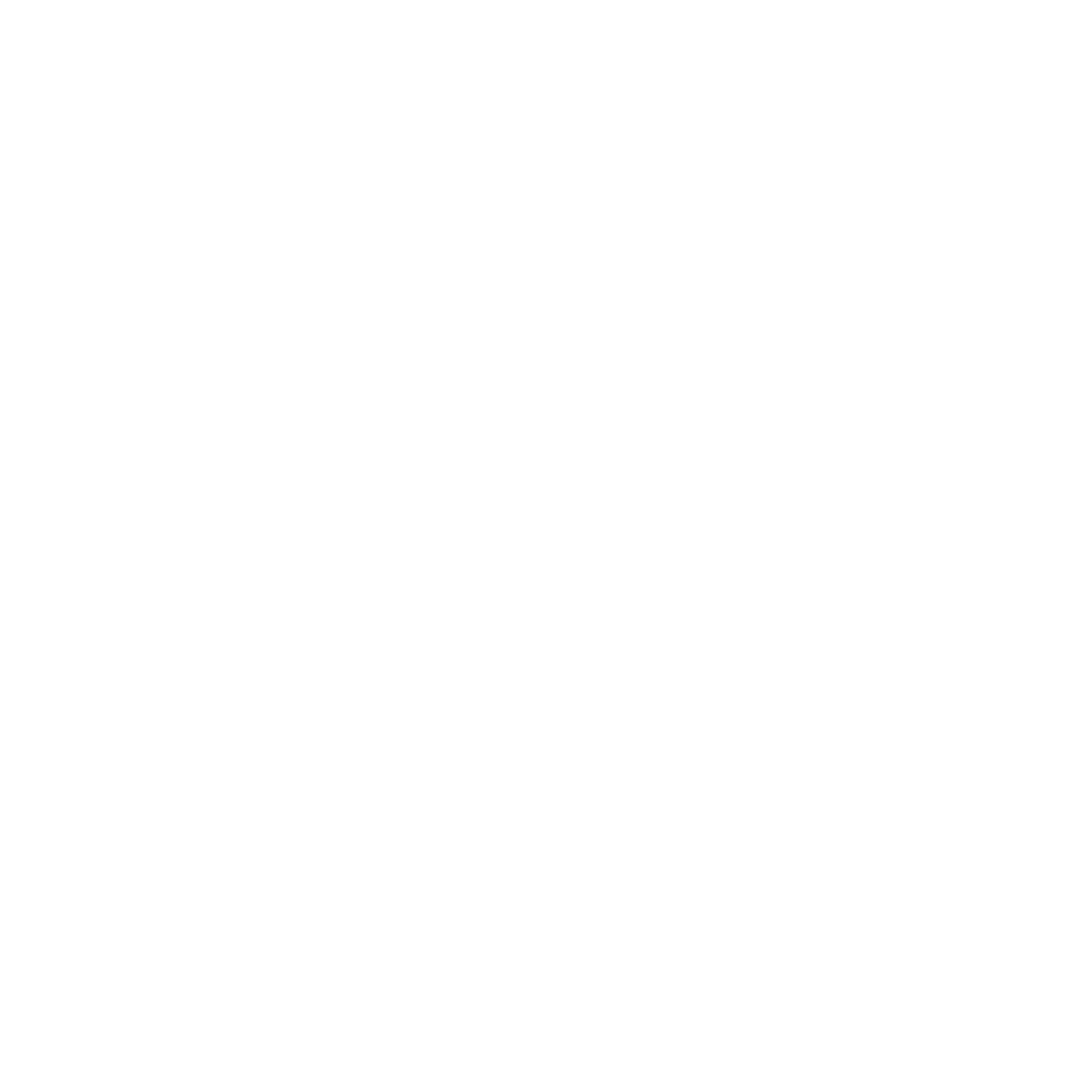
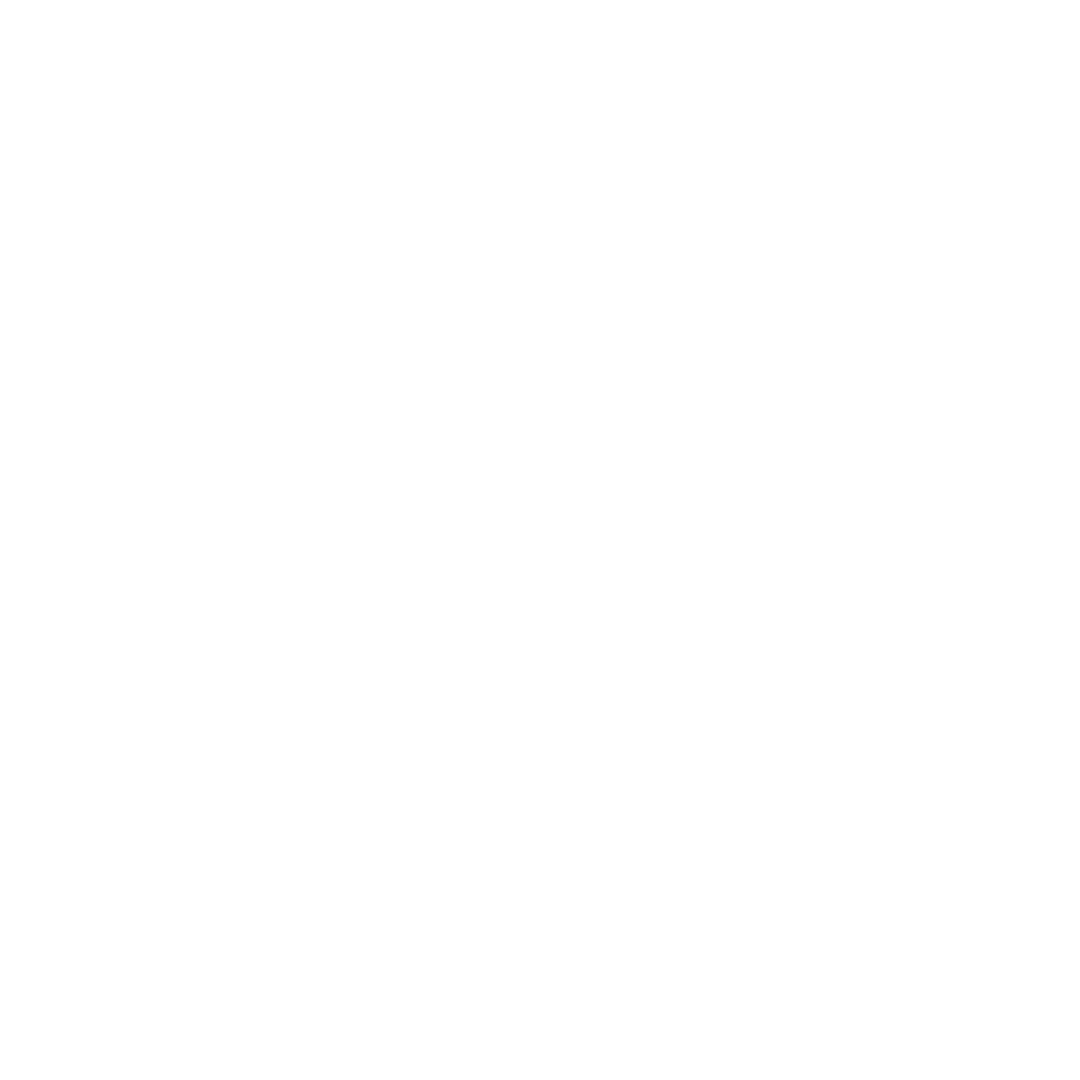
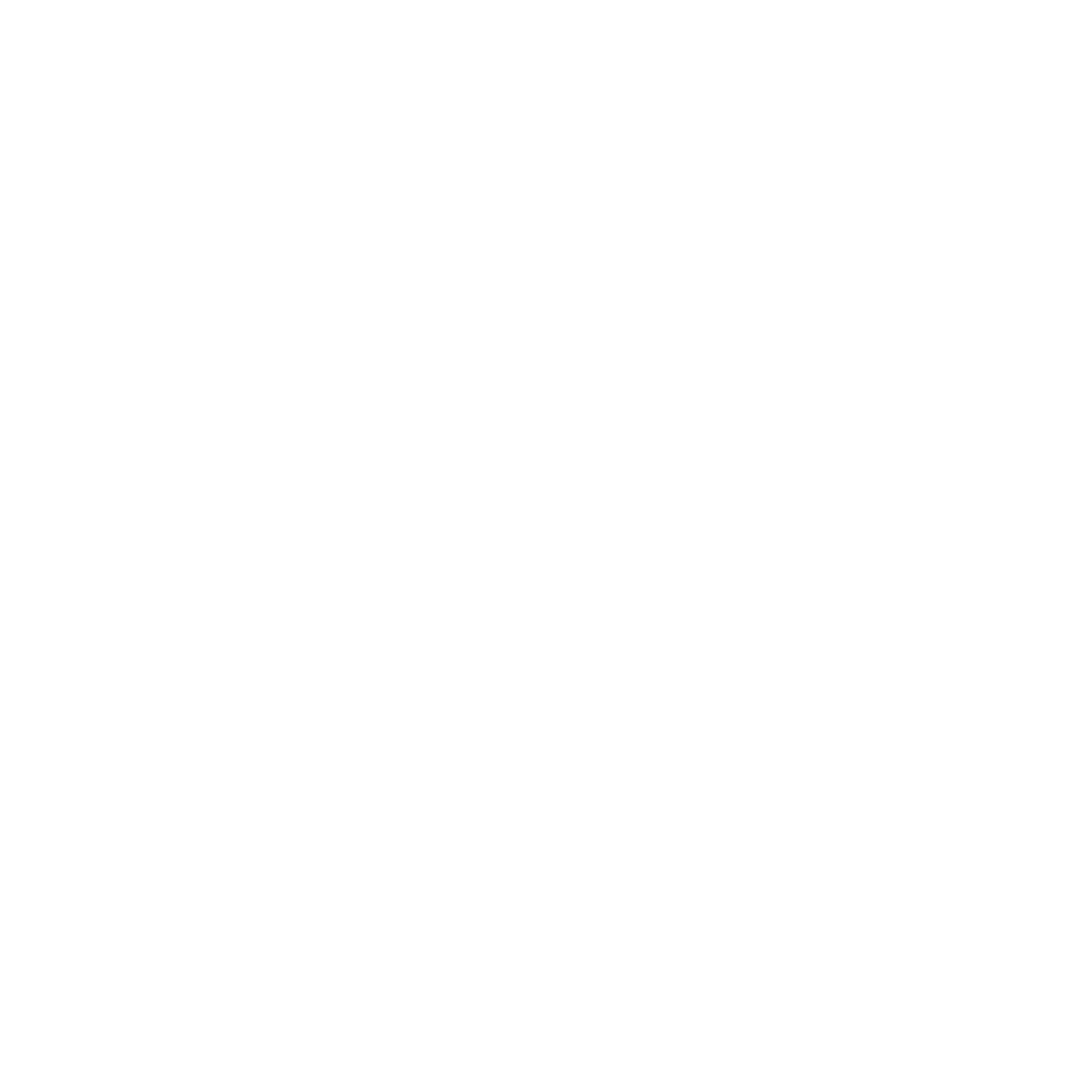
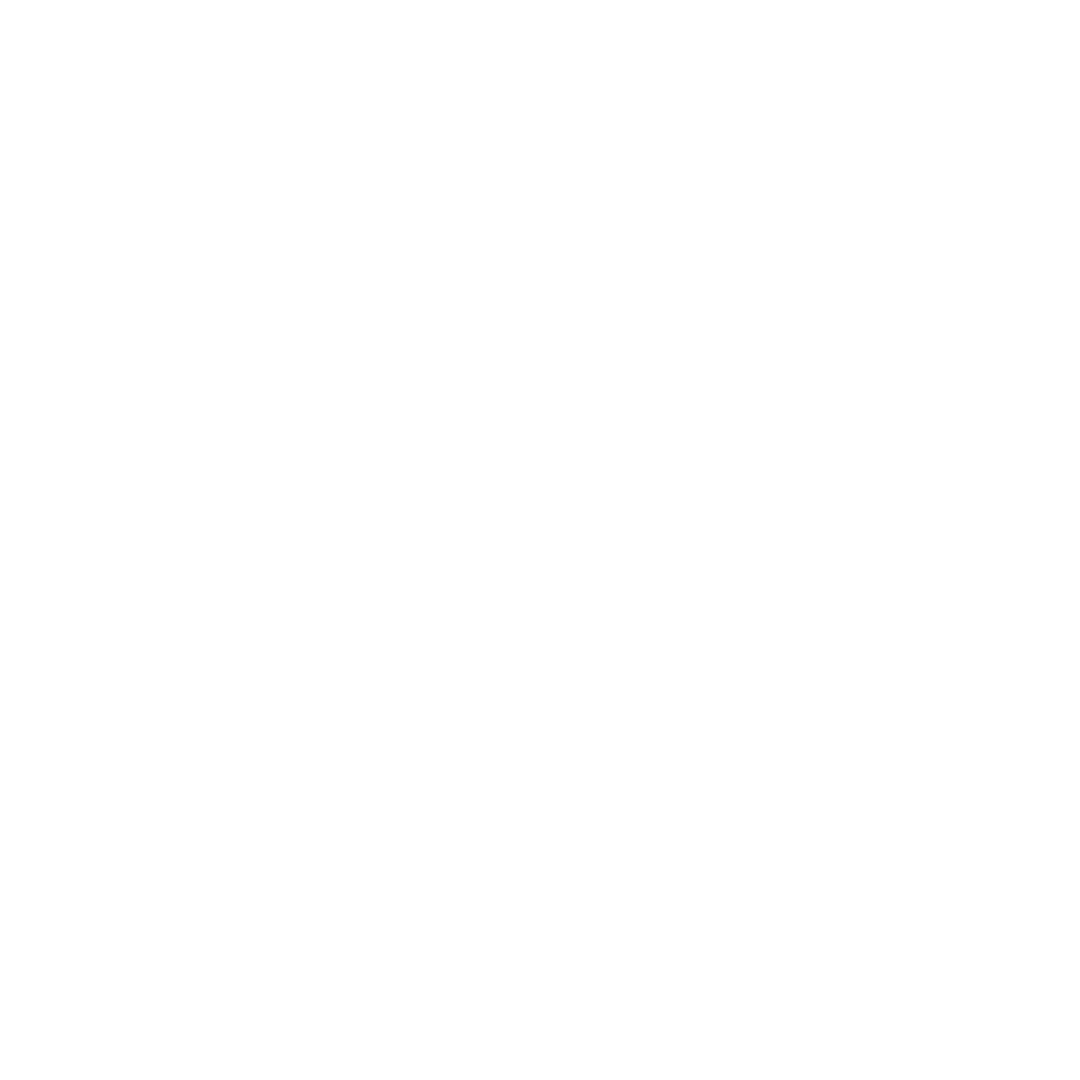
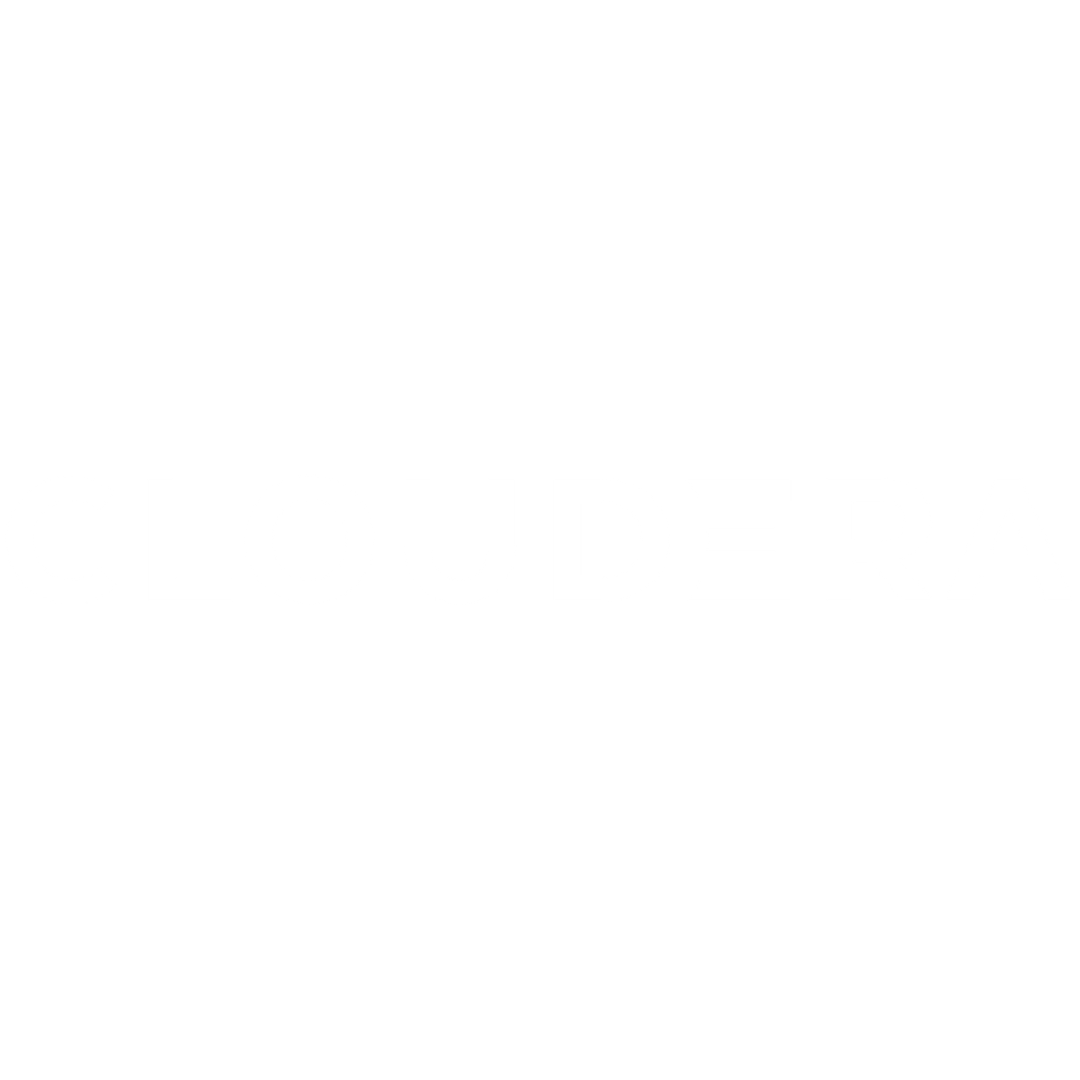
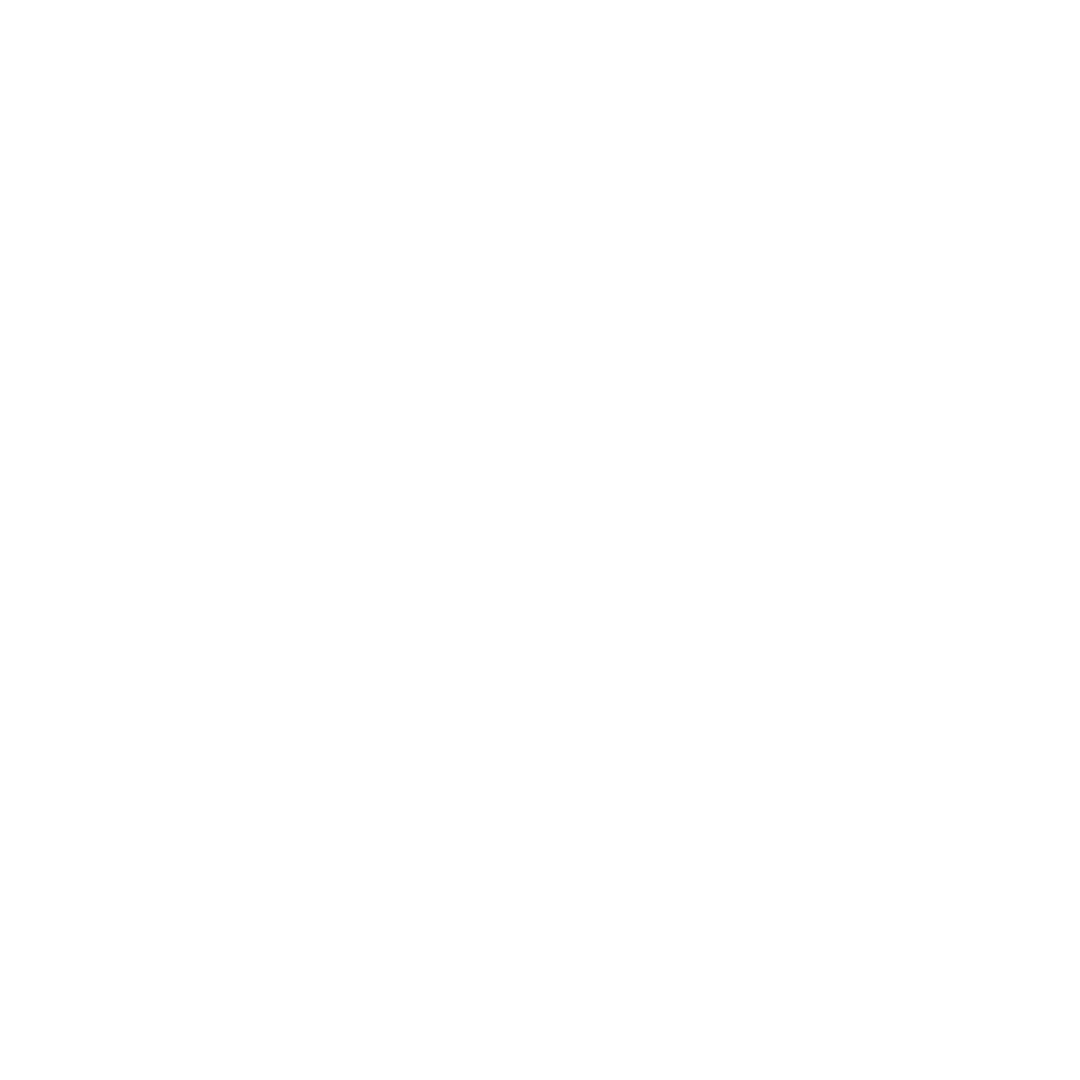
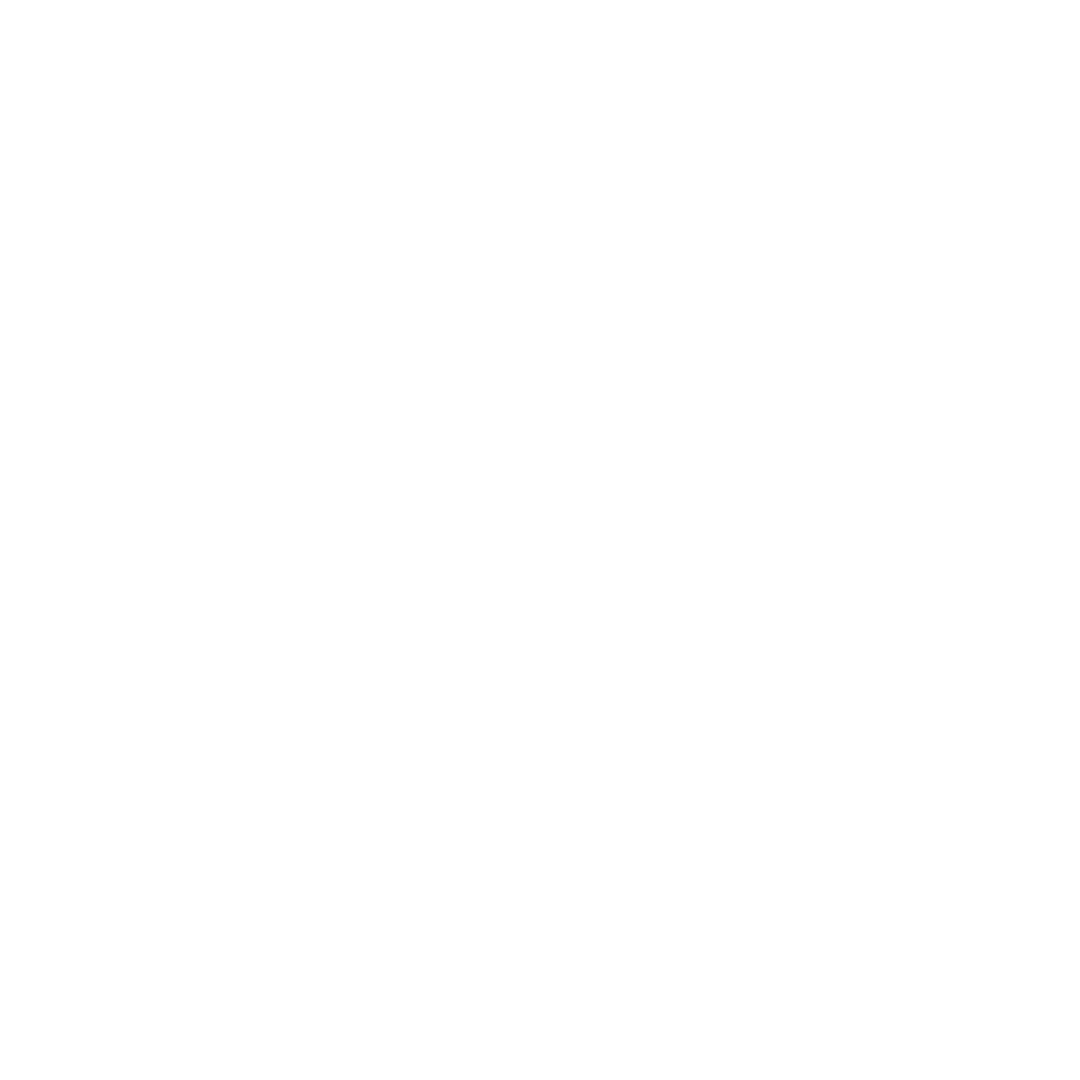
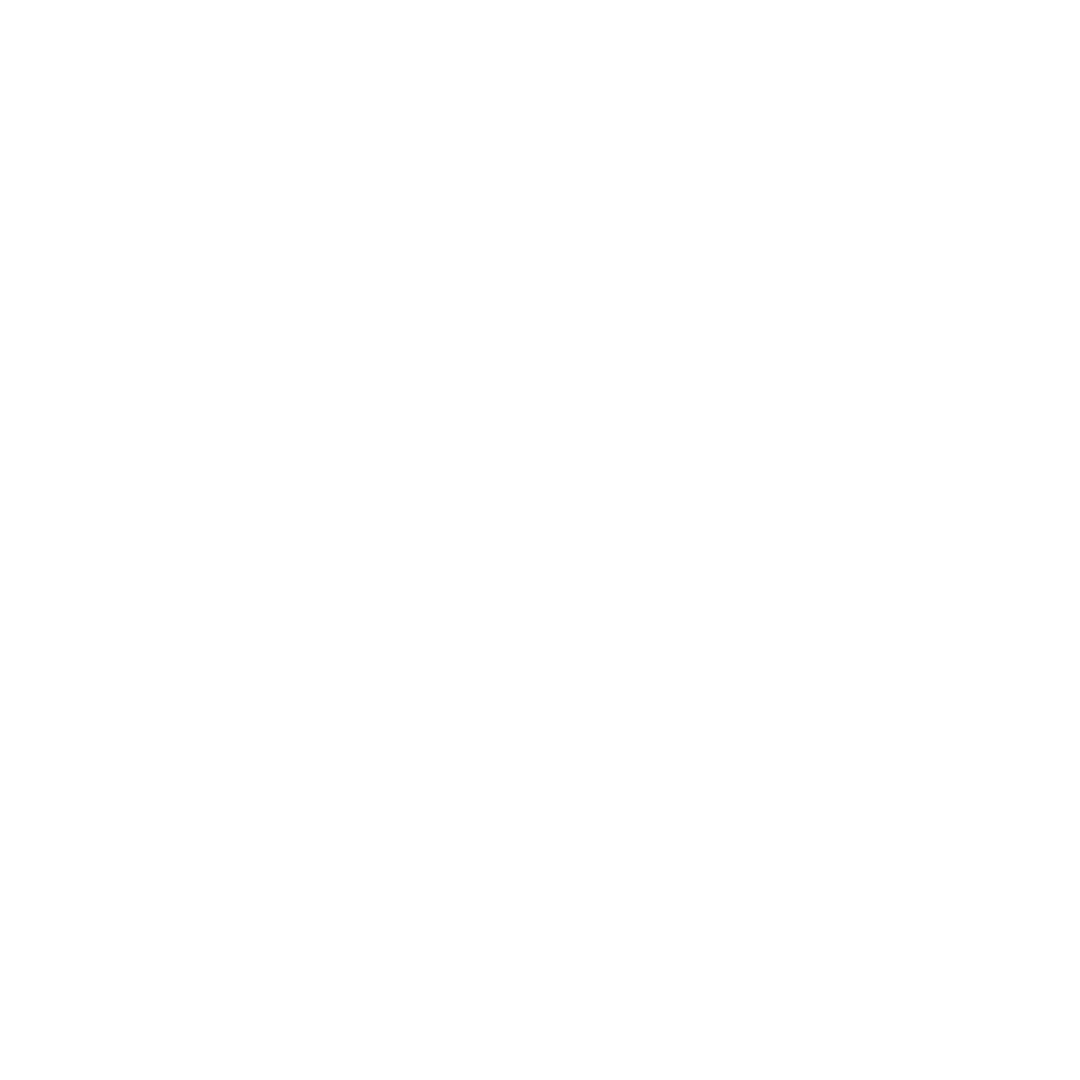
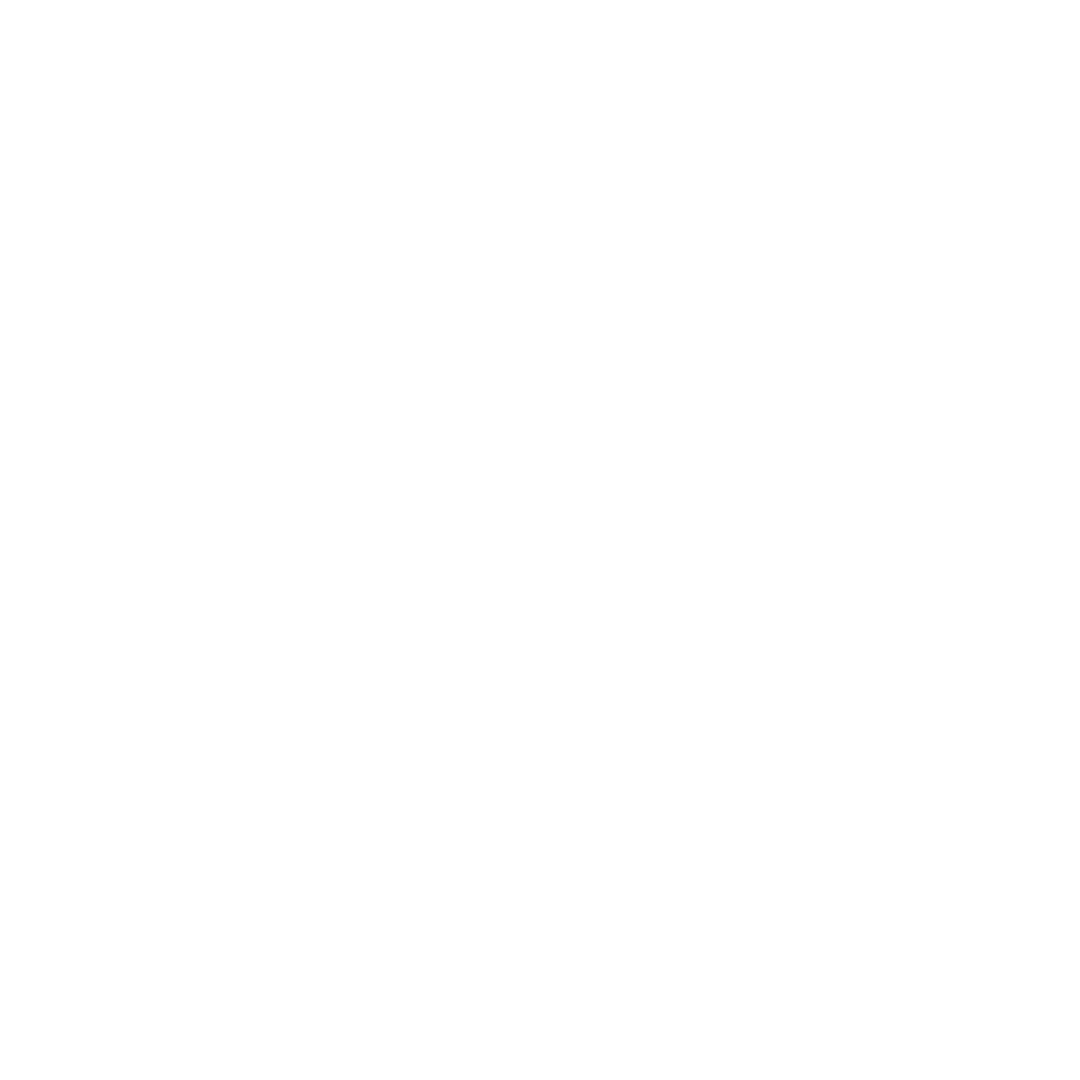
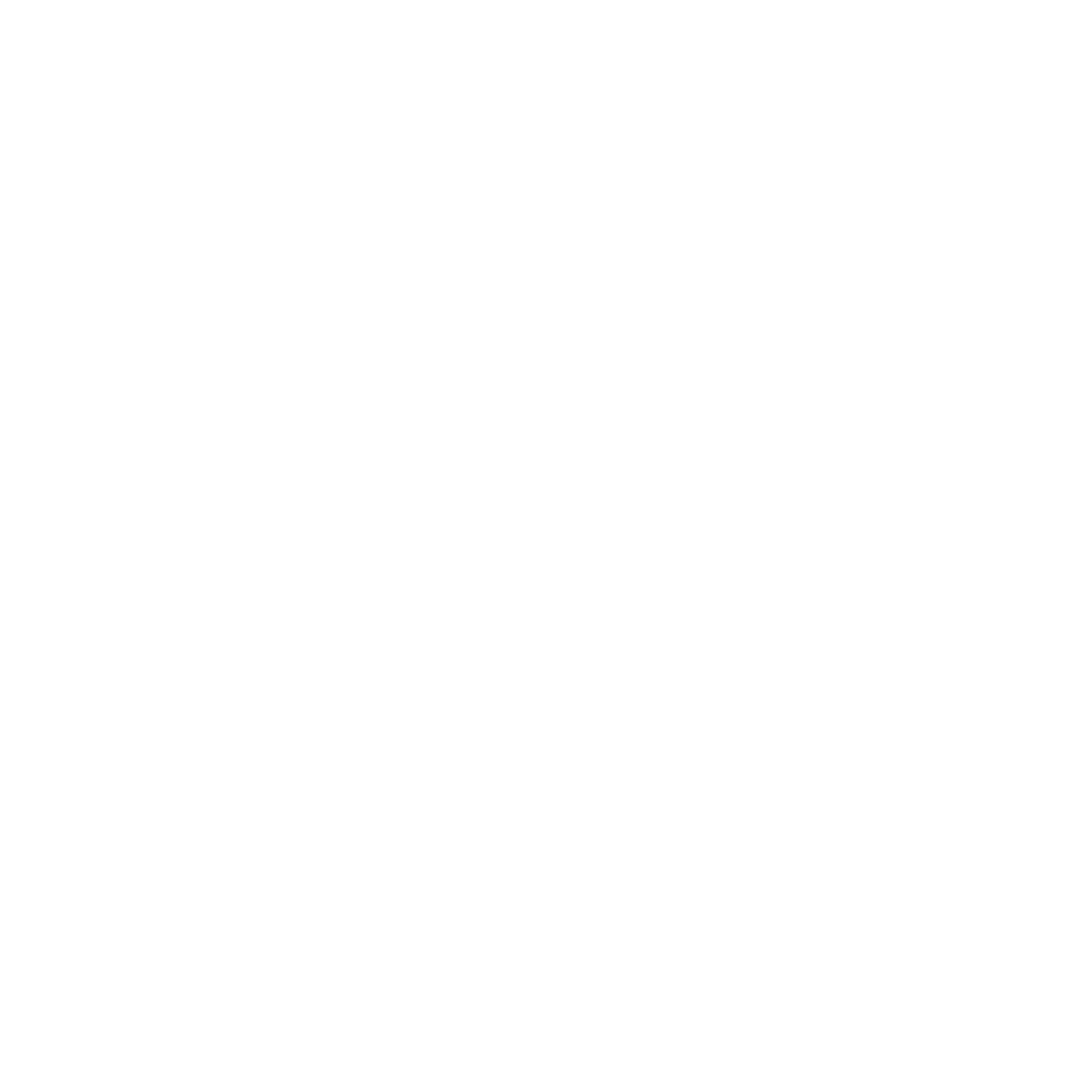
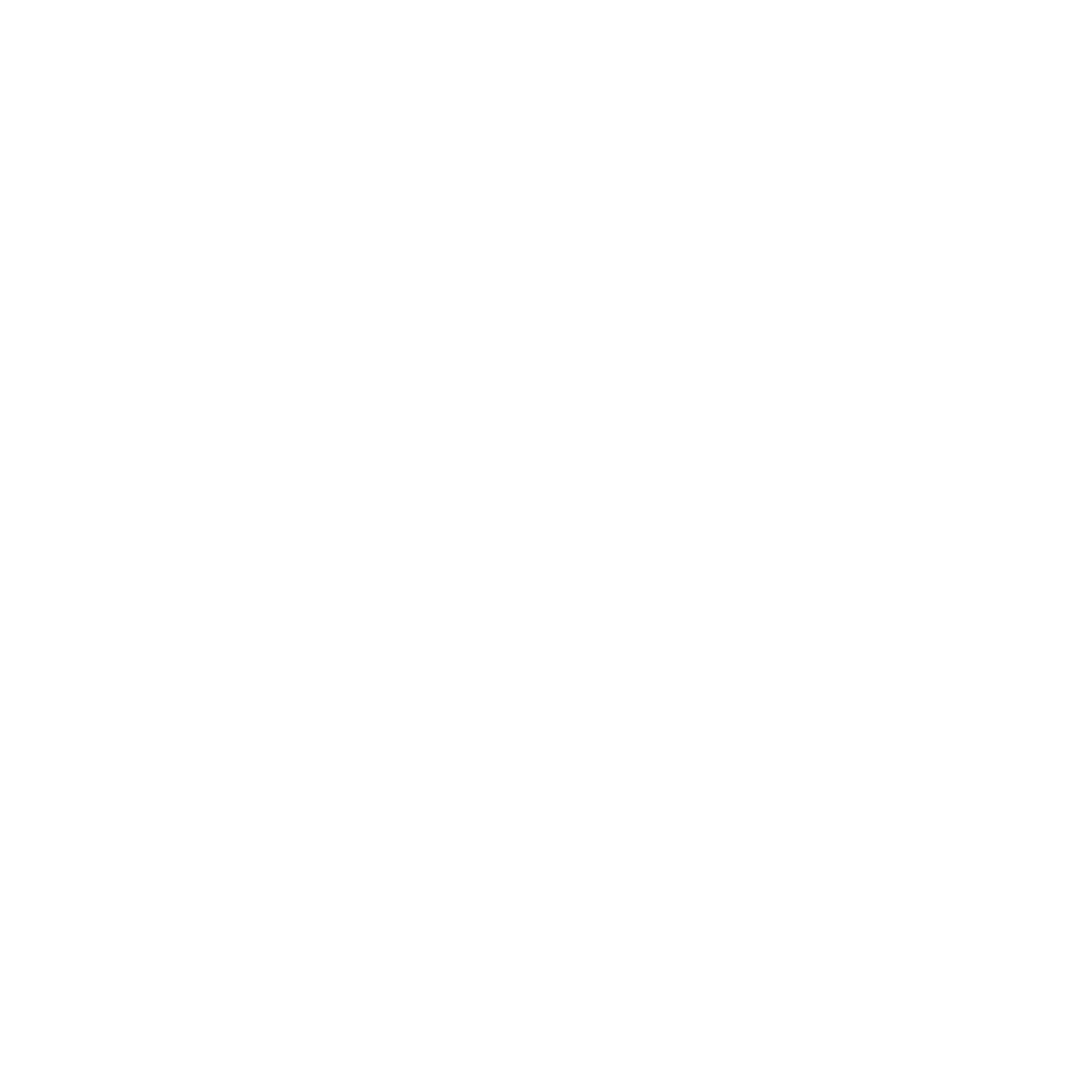
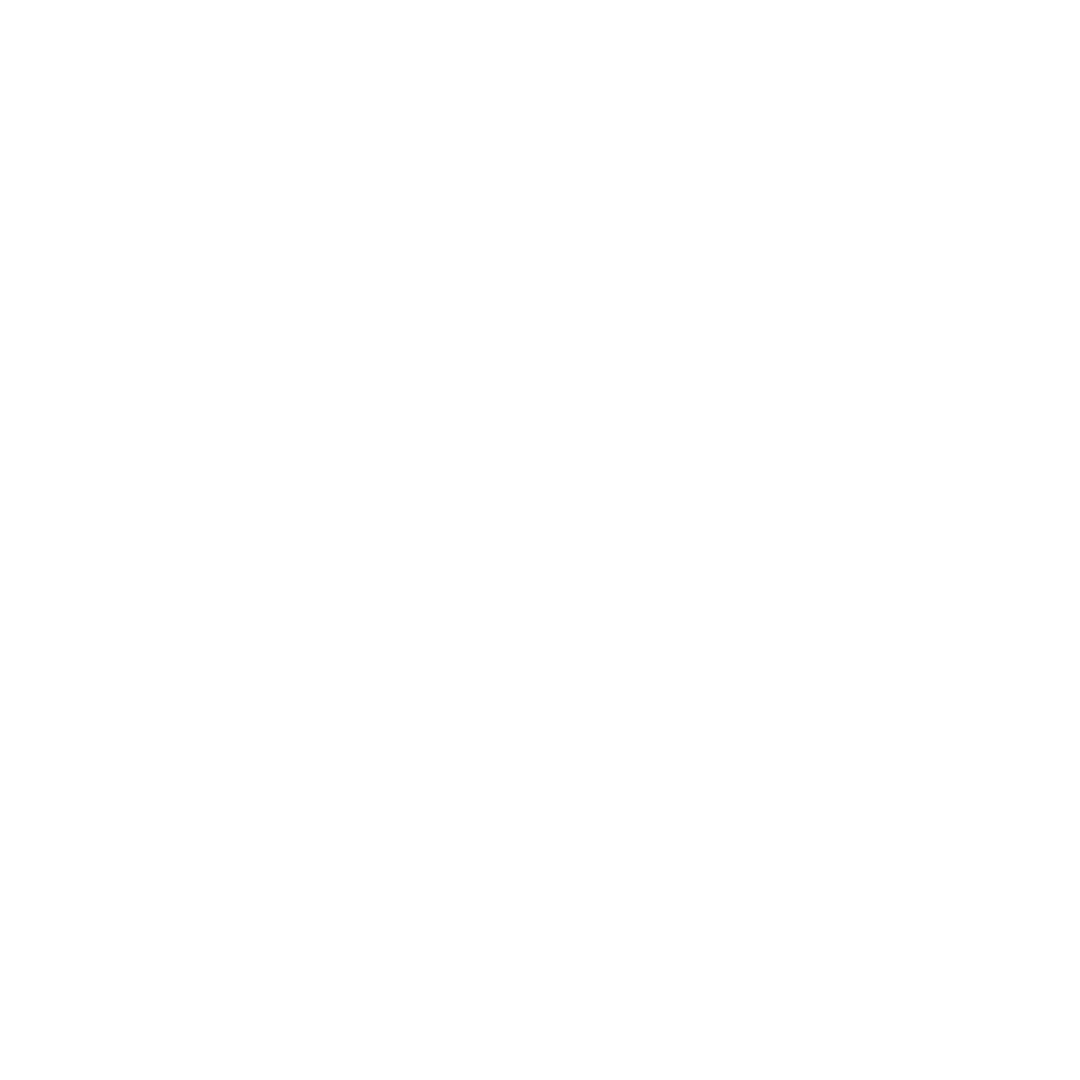
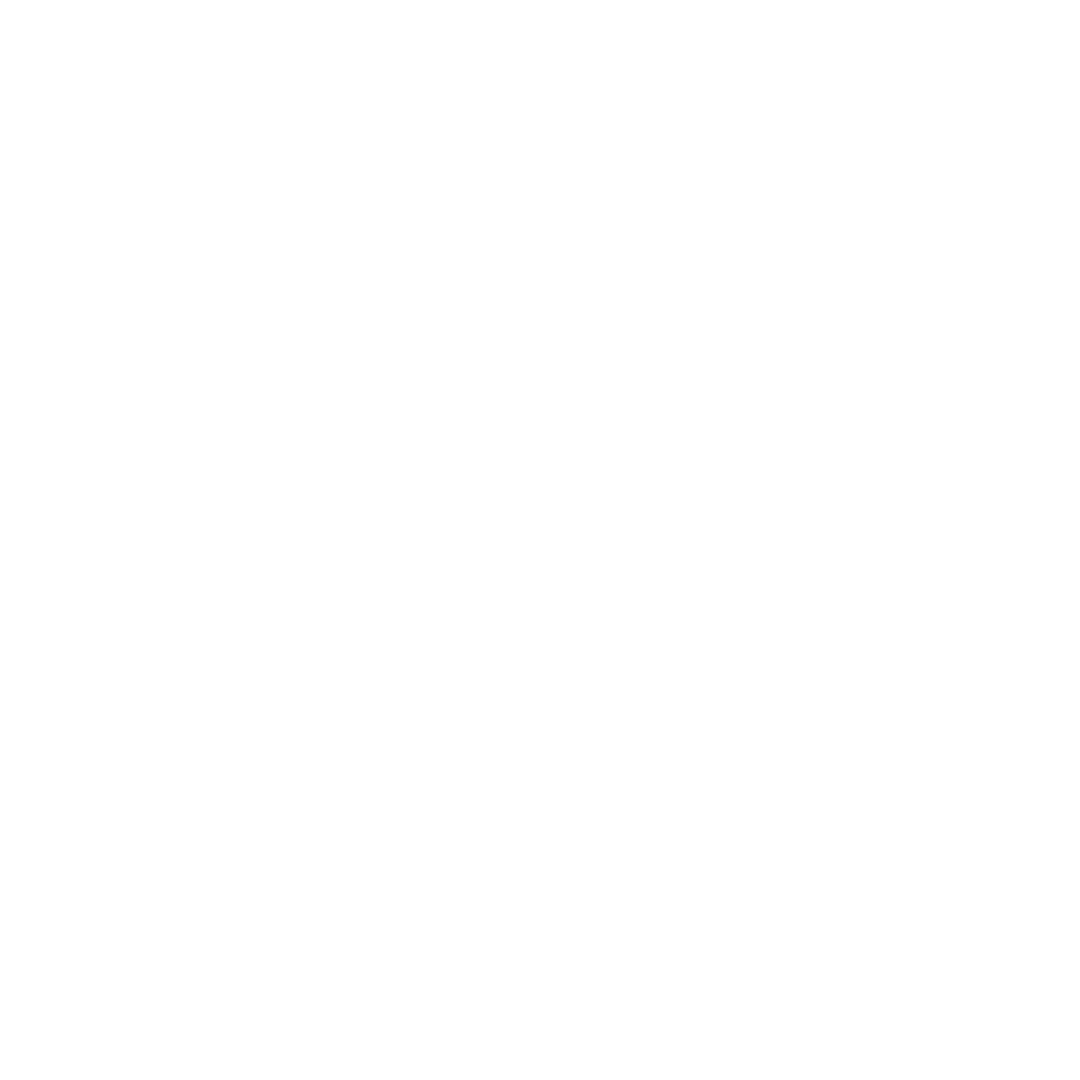
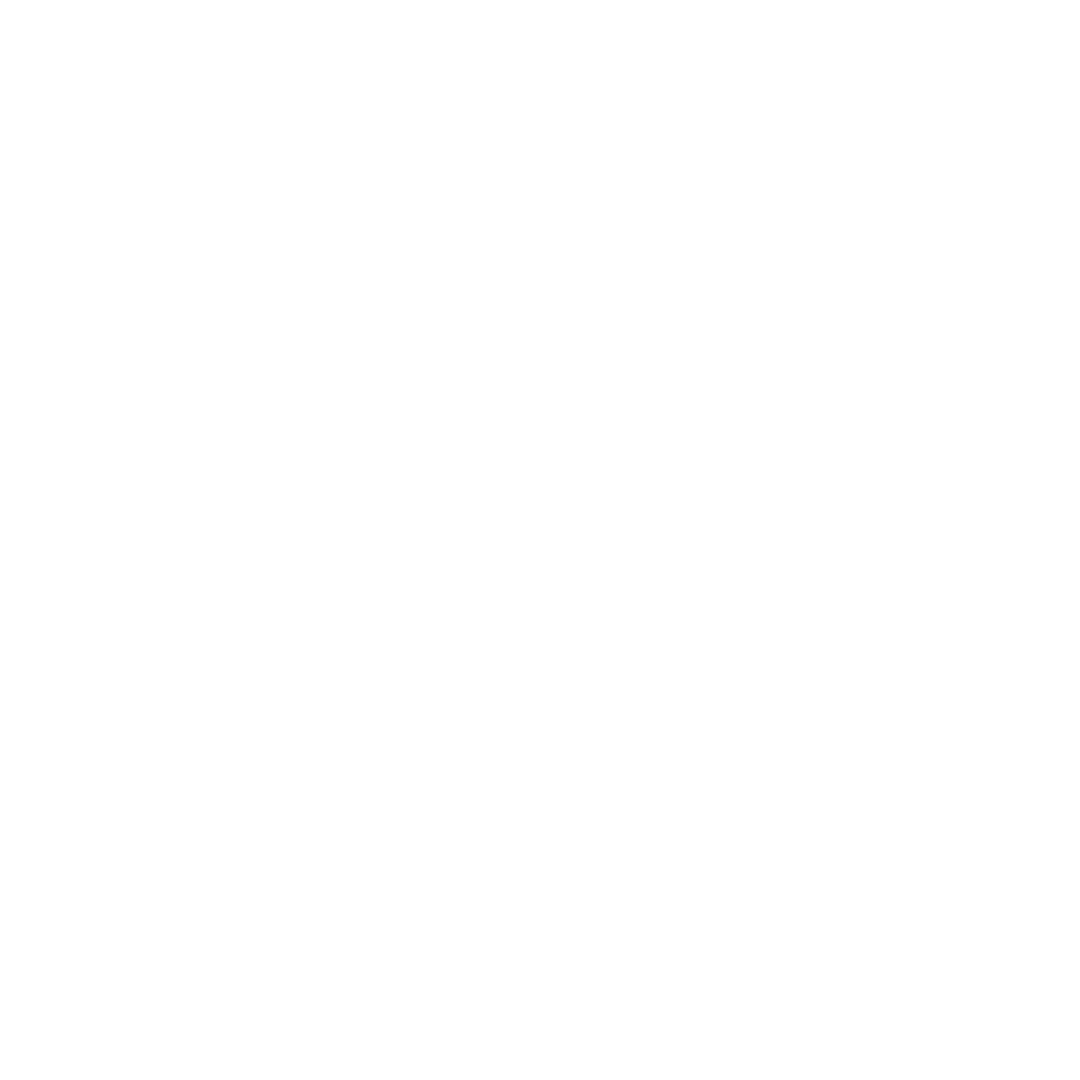
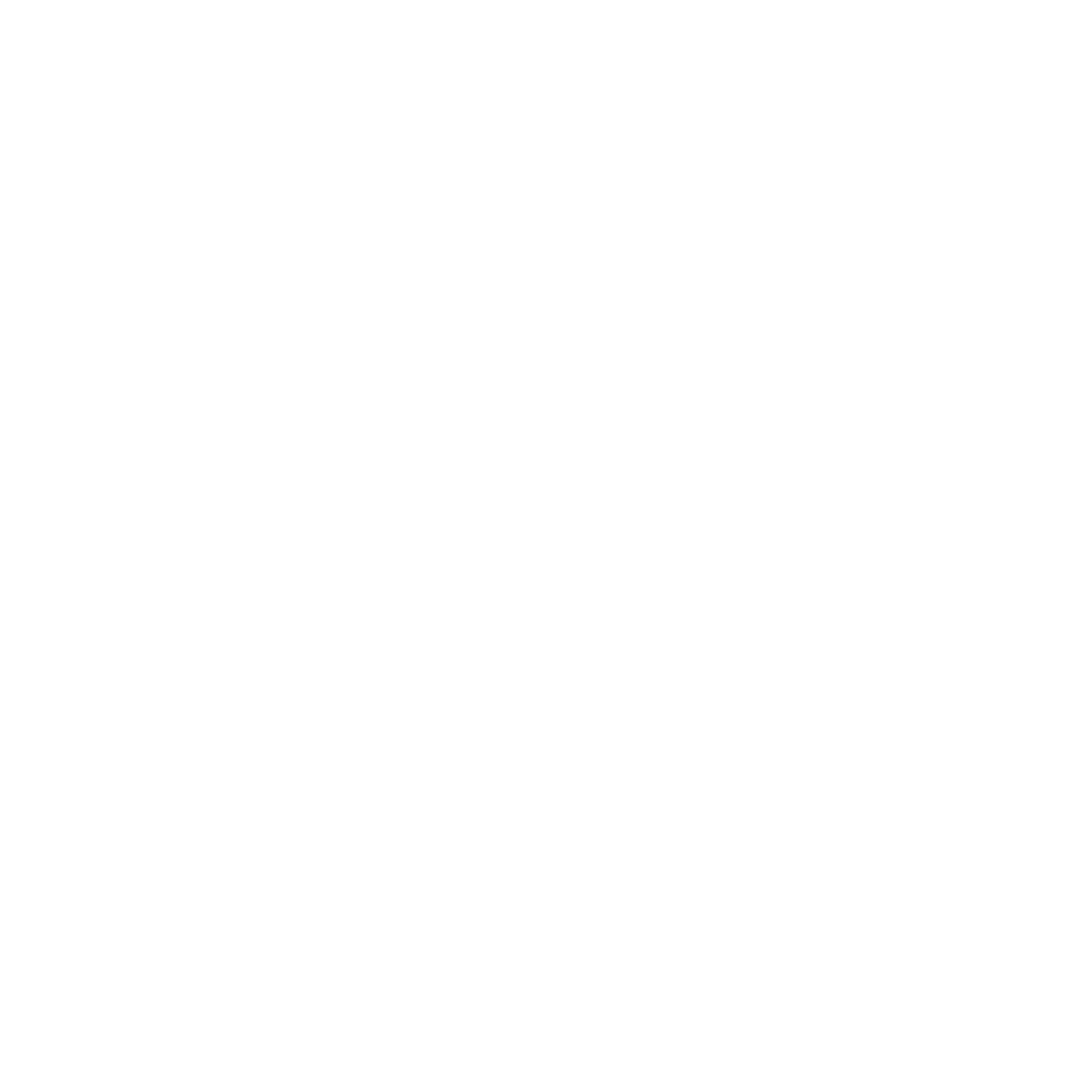
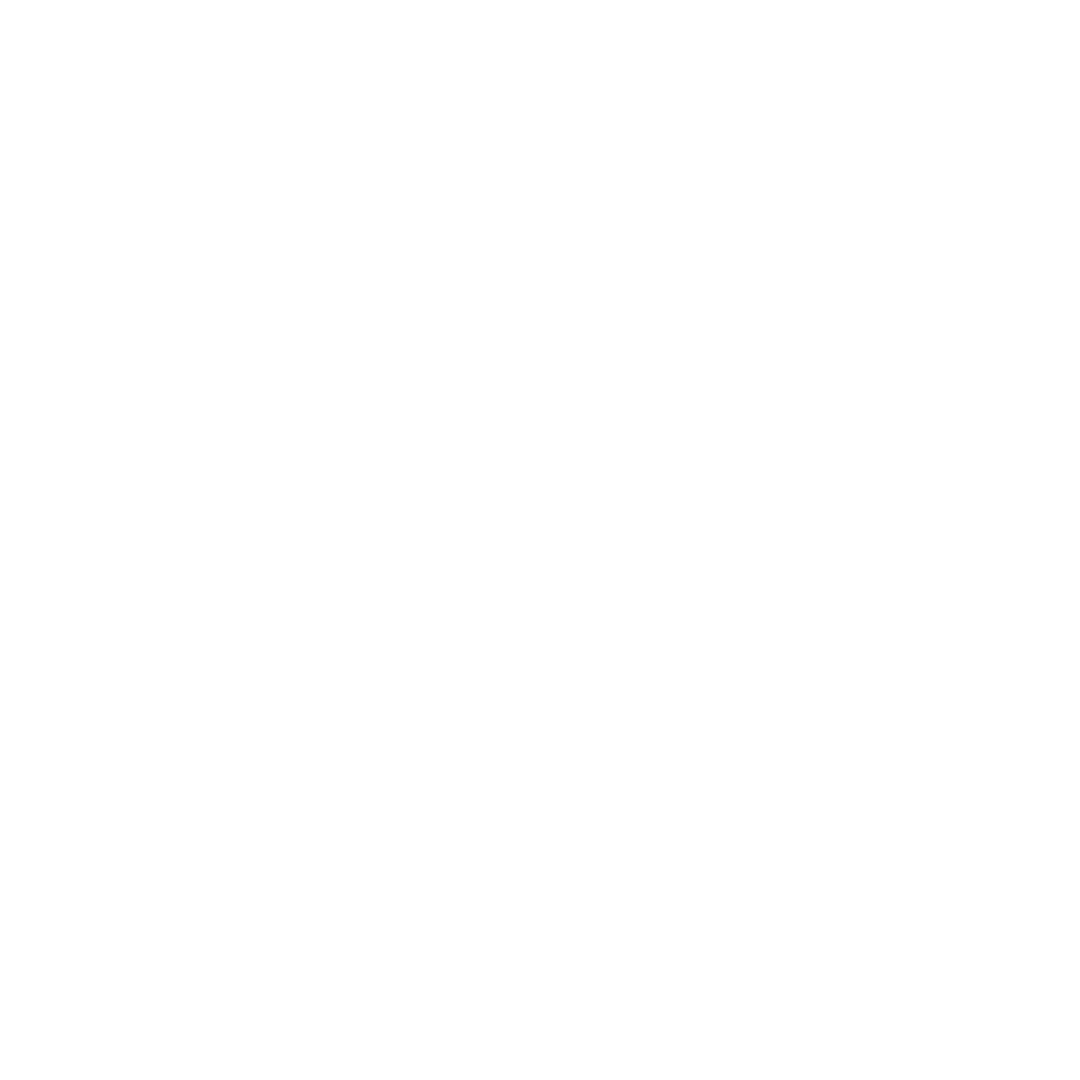
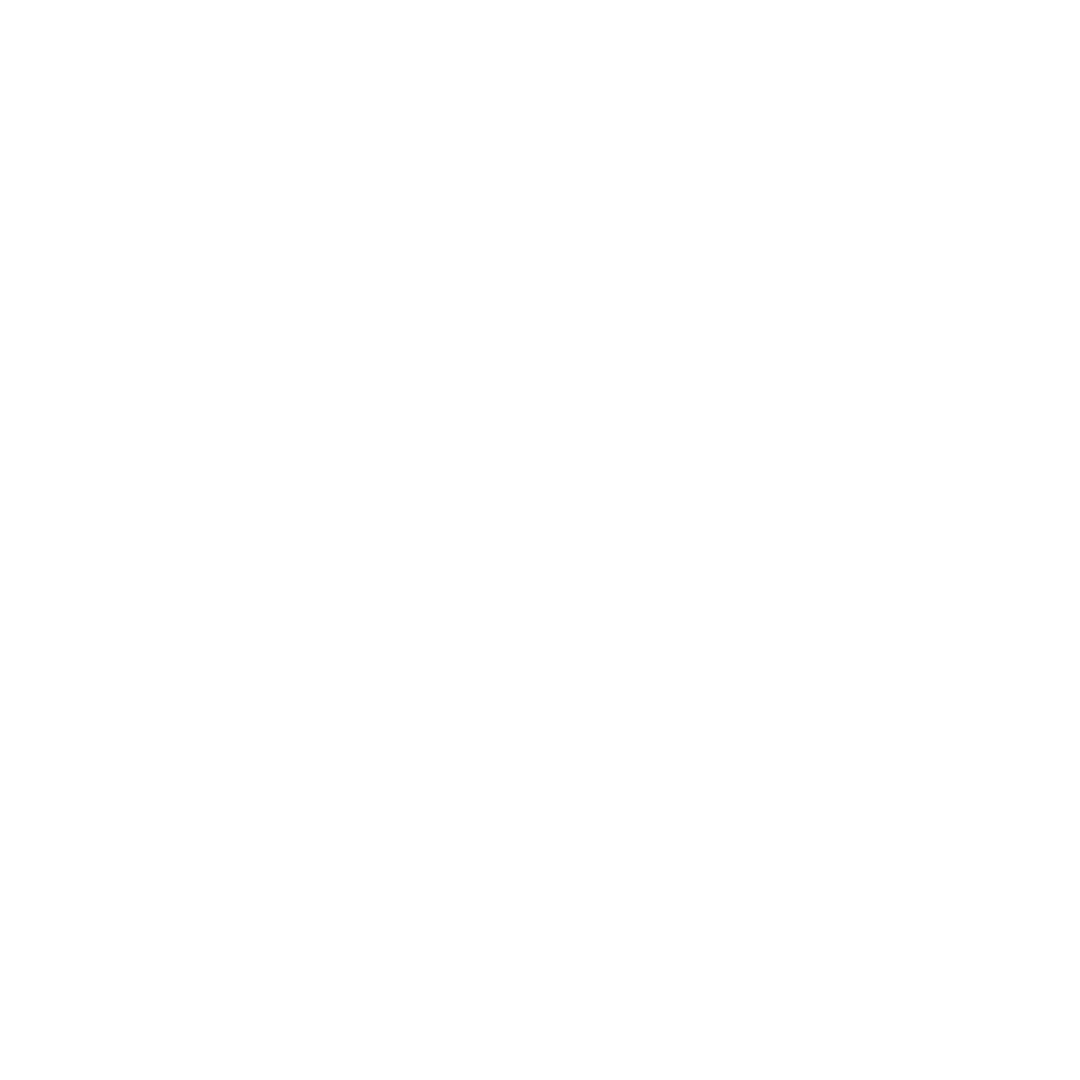
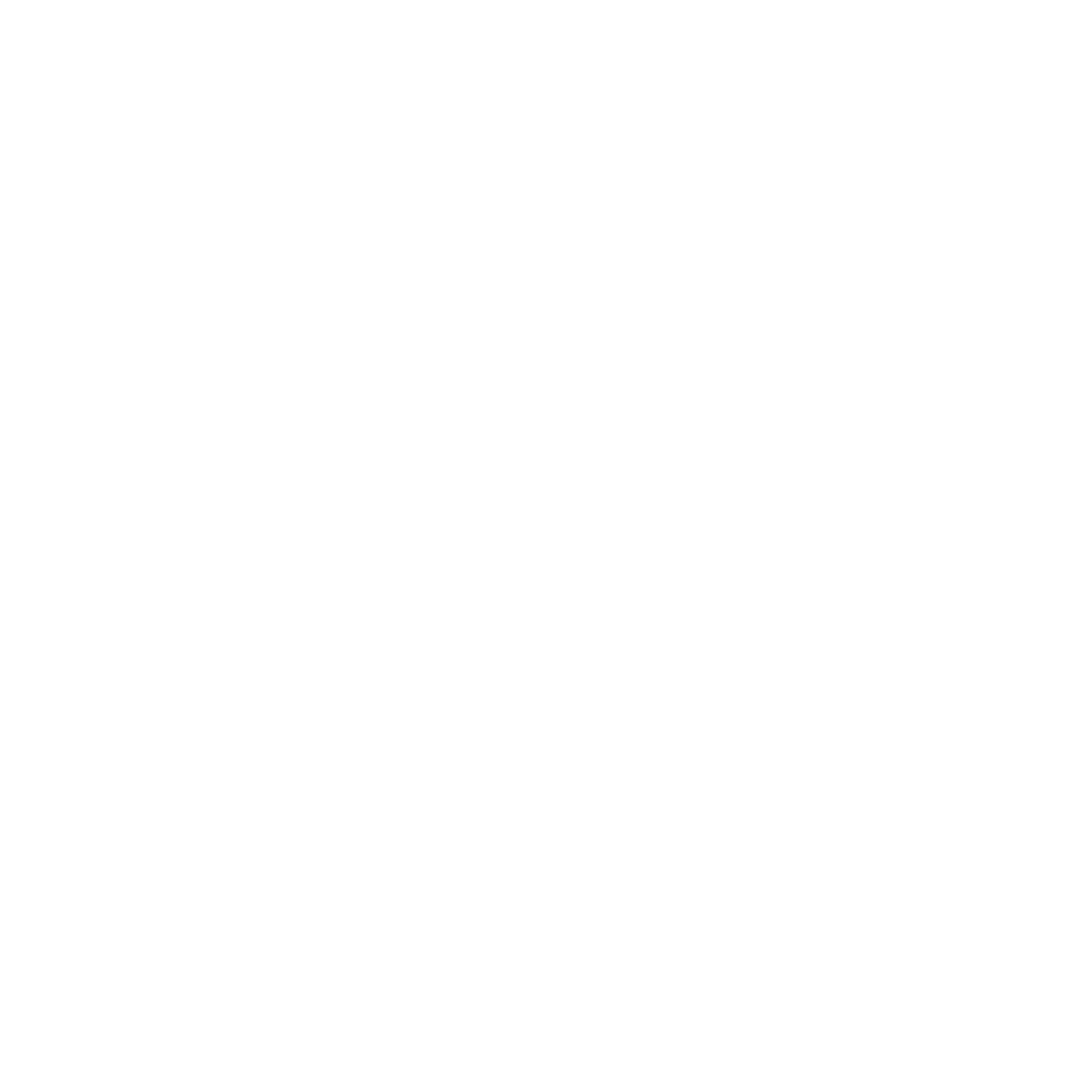
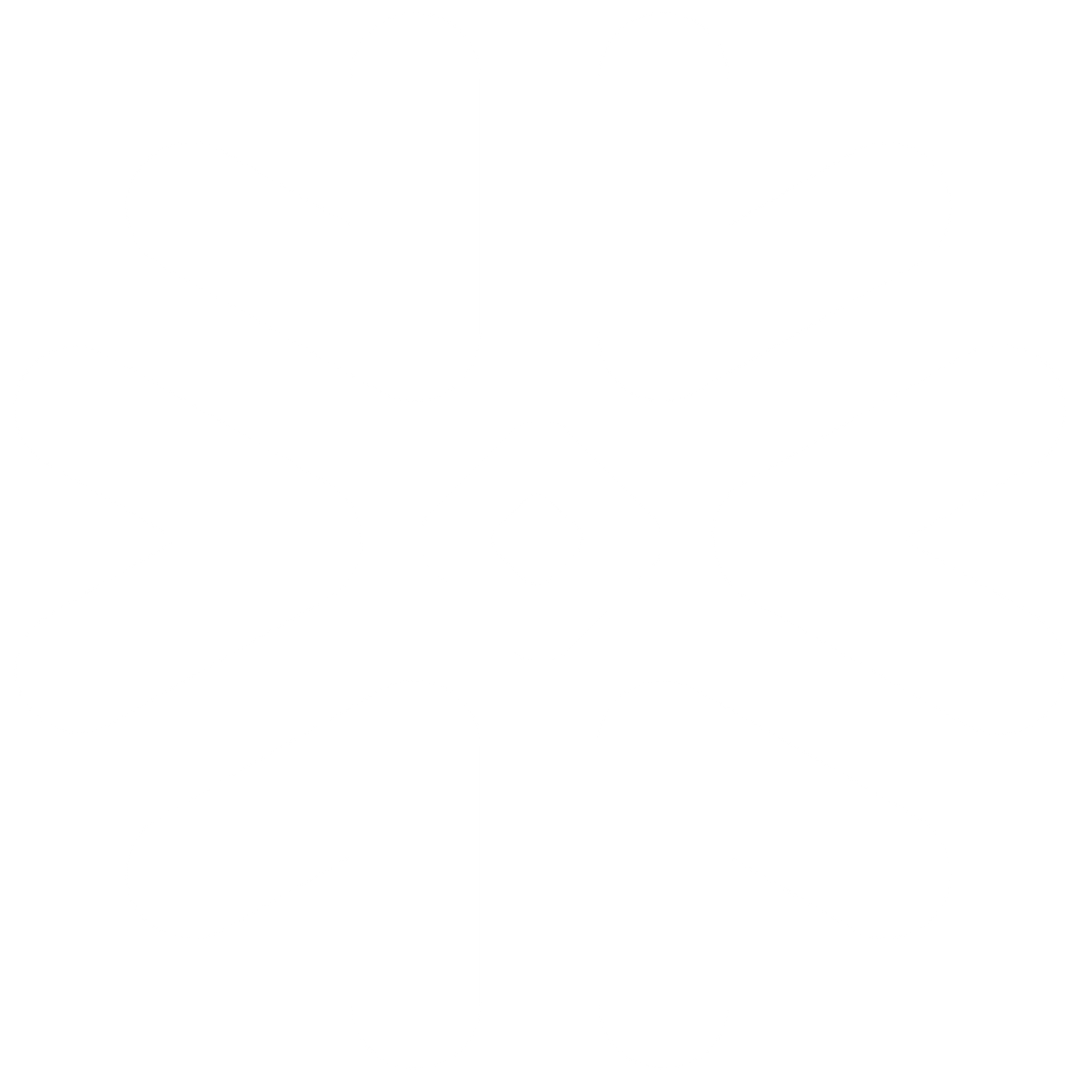
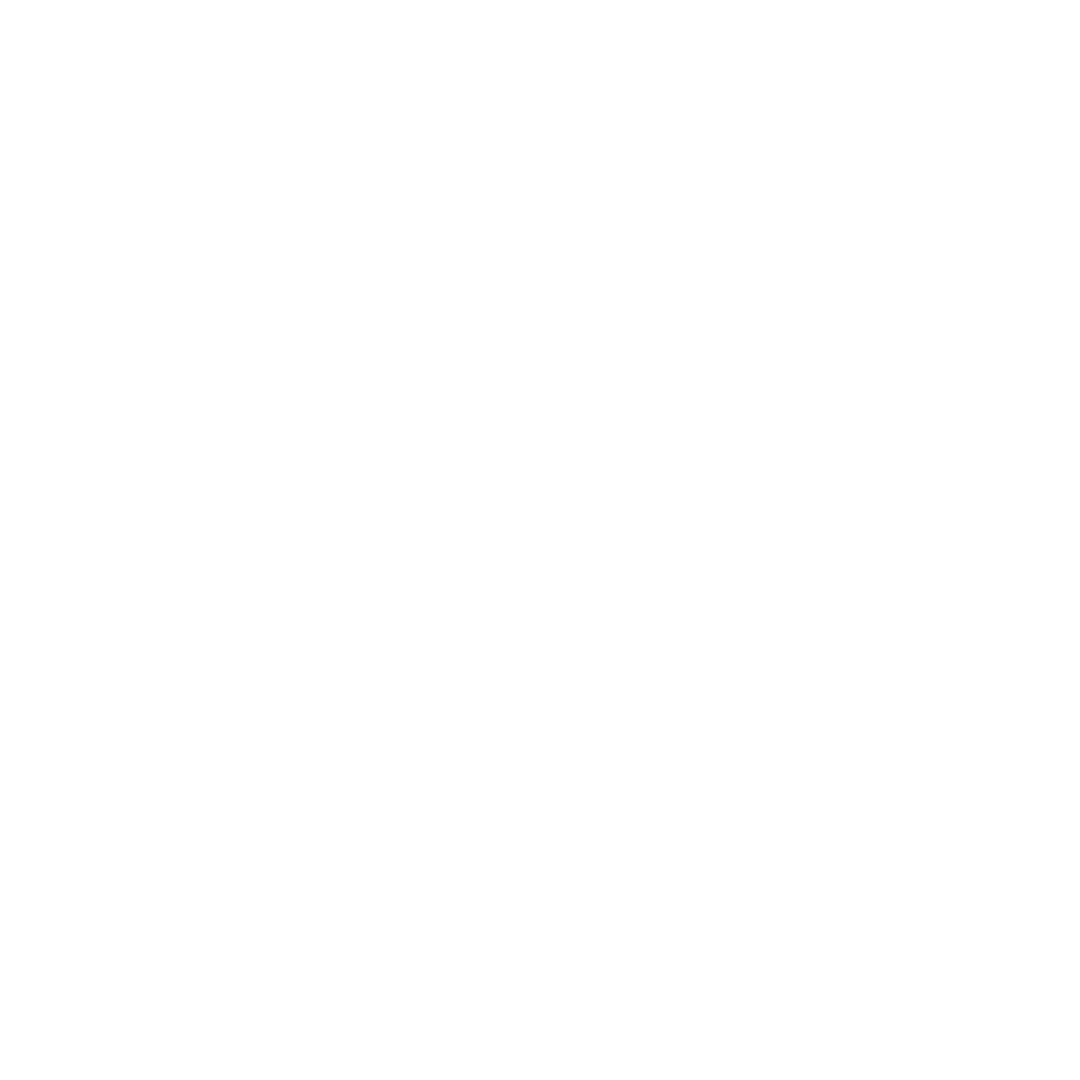
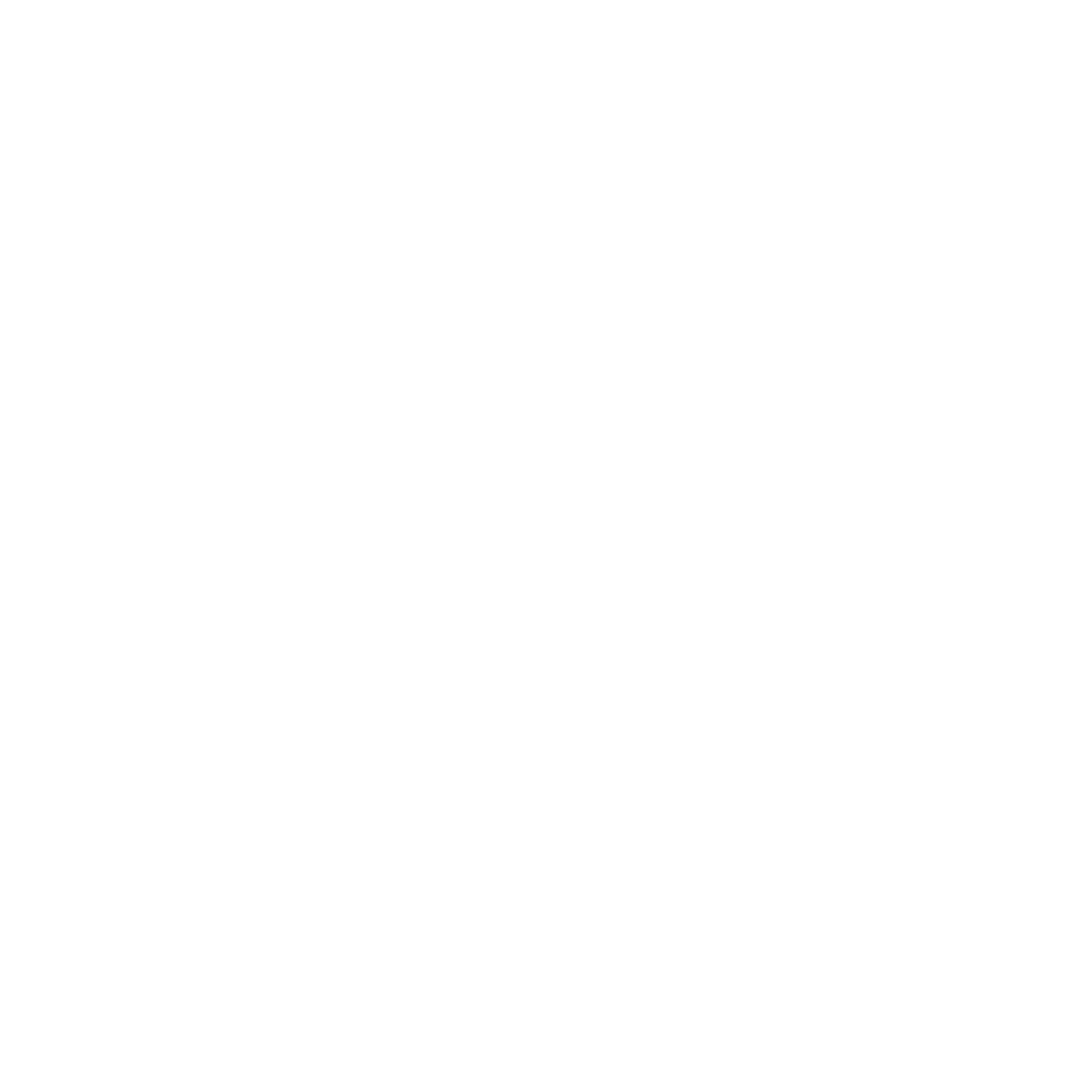
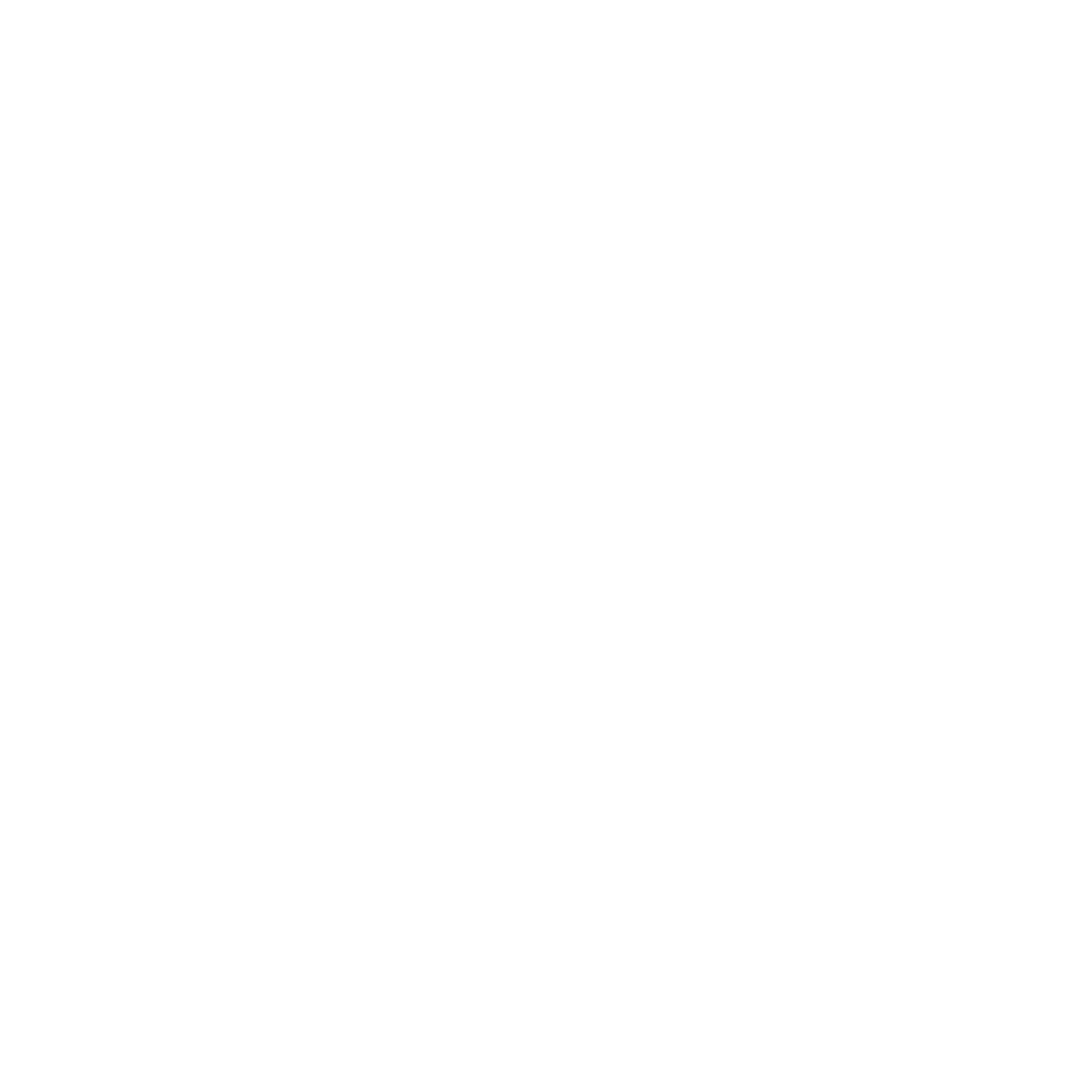
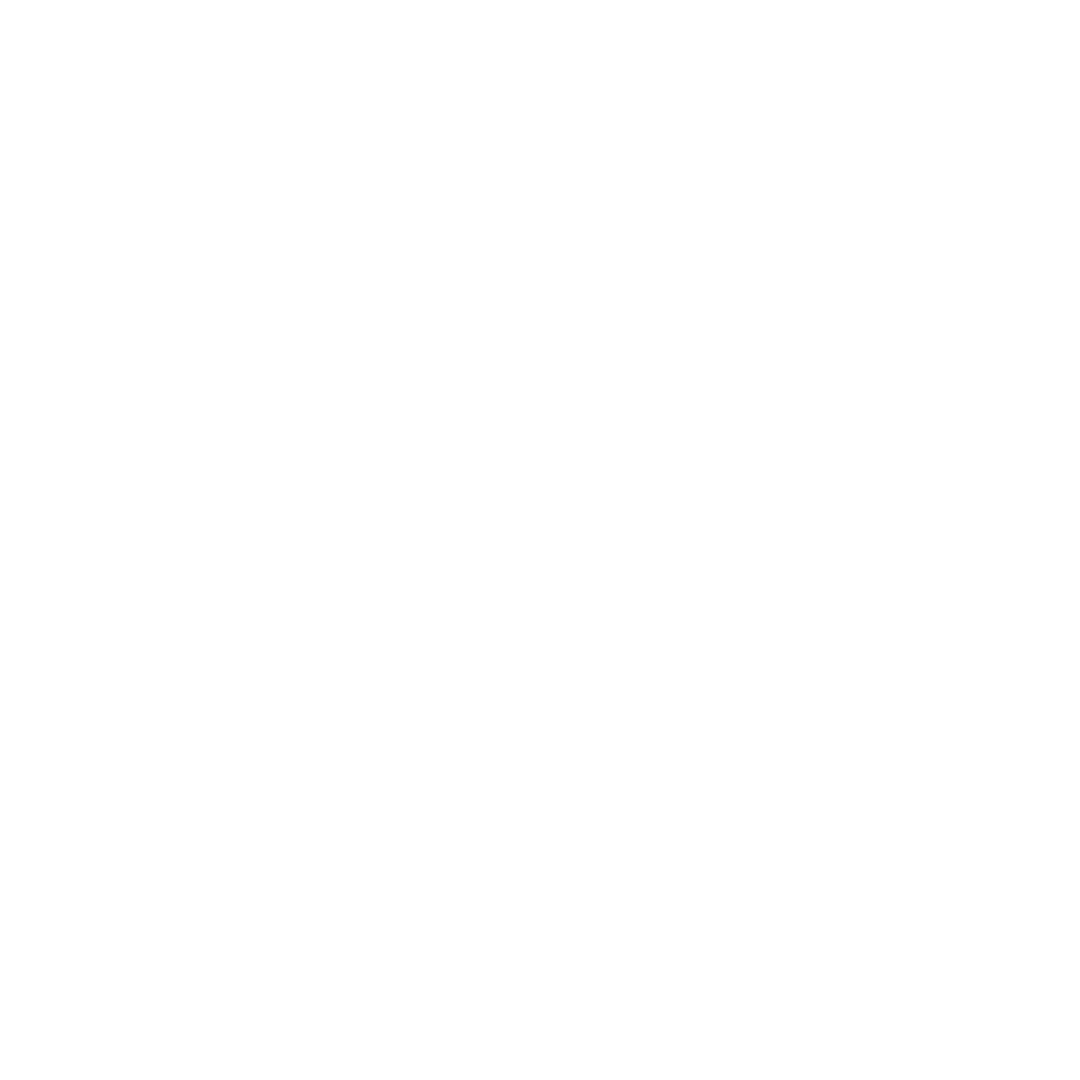