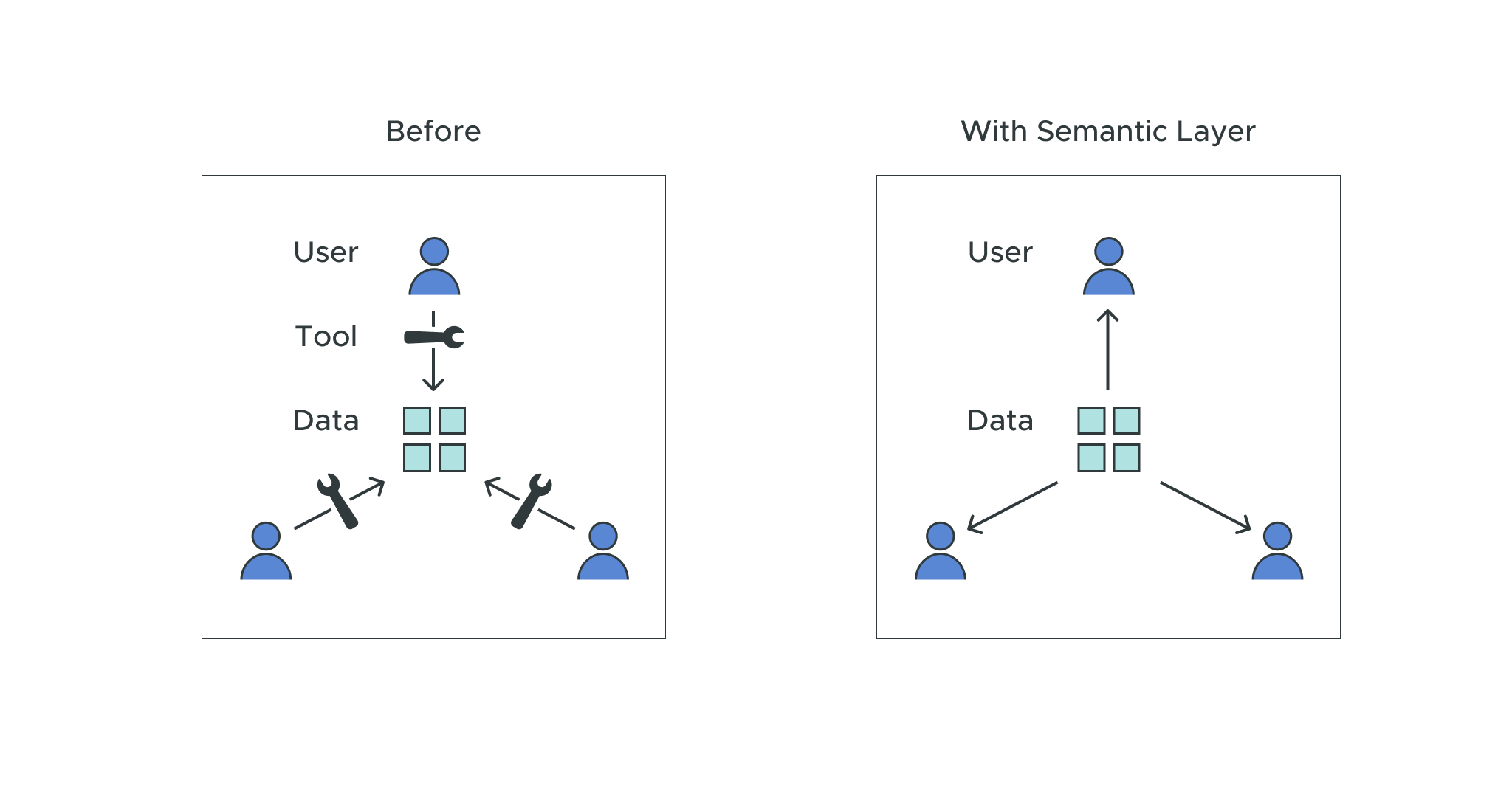
In my talk with AI Evangelist, Ben Taylor, we discussed how to use a Semantic Layer and augmented intelligence (AI) to bridge the gap between AI and business intelligence (BI) teams to drive business impact.
DataRobot is a powerful enterprise AI platform designed to accelerate the delivery of AI to production for organizations. With over $1 billion invested and over 750 data scientists and engineers, it is currently the most comprehensive platform on the market.
While AtScale is responsible for the semantic model for the data, DataRobot is responsible for the machine learning model that is designed to generate new features and new predictions.
We also touch on the increasing importance of augmented analytics, and discuss how artificial intelligence is expected to change in five years’ time.
What is the Purpose of a Semantic Layer?
A semantic layer enables us to define data once, and then leave it in place without being required to scale an entire data platform. It also allows users to make it work in any format that they want to be able to explore the data in.
For instance, data scientists may want to use Python to explore the data, whereas a BI user might want to use Tableau or Excel. A semantic layer provides teams with actionable insights by making the data discoverable and usable. It essentially brings the data directly to the people who need to use it, instead of sending people to the data and training them on different tools.
As a result, the semantic layer is typically used to create business representations of data, and to create a unified, consolidated view of the data that can be accessed by anyone across an organization in a ‘business-friendly’ way. This provides teams with advanced analytics that they can use to make informed decisions.
Providing Subject Matter Experts with Actionable Insights
One of the benefits of AtScale is that subject matter experts can now participate in the featurization and accountability of the data. Previously, this has been left to individuals – and when they leave a company, they take all of their knowledge and expertise with them.
Traditionally, documentation has typically been used like “the semantic layer” as a way to transfer knowledge between team members. However, documentation is not a good substitute, because it is often not prioritized by organizations. This means that it quickly becomes stale and outdated.
Constructing a Semantic Model From Scratch
One of the key benefits of the AtScale platform is that it gives subject matter experts the ability to create models and gain access to data-driven analytics themselves instead of handing the task over to someone else. It essentially makes something that has not previously been a team sport begin to feel like a team sport.
A subject matter expert can create a semantic model and make it very easy for anyone to be able to understand the data and use it to make decisions. This model can then be used to generate new predictions, which can be compared to actual data to see whether targets have been met.
In this section, we’ll cover the steps involved in constructing a semantic model from scratch:
1. Feature Creation
Feature creation begins with raw data. In this demonstration, we used the Snowflake warehouse (there are multiple other options such as Databricks, and Google BigQuery) to build a business-friendly interface that is both tool and platform-independent.
The goal of this stage is to provide us with live access to more first-party, third-party, and SaaS data, and to allow us to blend disparate data sources and model business processes with conformed dimensions, such as length of time. It also enables us to create complex features quickly with a no-code modeling canvas.
2. Feature Serving
This stage allows us to query the semantic layer and discover what the new features are, and do some descriptive and diagnostic analysis. It also allows us to publish features to different audiences using the tools of our choice, and to create and govern data products with self-service.
This way, we can create data products and machine learning models that allow users to take advantage of the data and predict the future without having to know anything about where the data came from or how it was structured.
3. Feature Publishing
The next step is to create new machine learning features in the DataRobot platform and publish these features back to the AtScale semantic layer. This becomes automatically integrated into that semantic model, and the data is stored in Snowflake.
This essentially combines the work of subject matter experts creating the semantic models with AI experts creating the machine learning models.
This lets us create features and share these features across the spectrum to create new augmented data products that make it easier to add new features quickly and easily, and ultimately makes everyone smarter!
4. Ready-Built Data Products
Then, we ran through an example using a Jupyter Notebook designed to augment a sample internet sales model and provide us with predictions. The result is displayed in an Excel spreadsheet so it can be used by business professionals.
What Will Artificial Intelligence Look Like in Five Years’ Time?
At the end of the demonstration, we questioned Ben on what the state of AI would look like in five years’ time. said that AI has come a long way over the past few years, and that it is important to celebrate how far we’ve come.
He predicts that in the next five years we will see faster time to value, and more access to more data types and data sources. He also emphasizes the importance of defensibility, and explains that as we move into more high-consequence predictions – such as in the healthcare industry– we will need to have better accountability. To do this, we must give more thought to what technologies need to be in place to ensure that we can make the best predictions.
We’re Still Figuring Out How to Fully Leverage Data
Looking to the future, we are aiming for a world where subject matter experts can share their knowledge freely and easily, and data scientists can share their predictions with everyone seamlessly without people worrying about where the data has come from.
We still have so much data that is not being leveraged because many of us are too busy to figure out how to do it. We shouldn’t need to spend time creating a new dataset, and then creating another project to integrate it, and it shouldn’t be necessary for people to know what’s happening behind the curtain.
If we can all spend more time thinking about how to leverage our data, we will be better off for it.
Watch our panel discussion with Ben here to learn more about his thoughts on how data scientists can leverage AtScale’s semantic layer.
SHARE
ANALYST REPORT