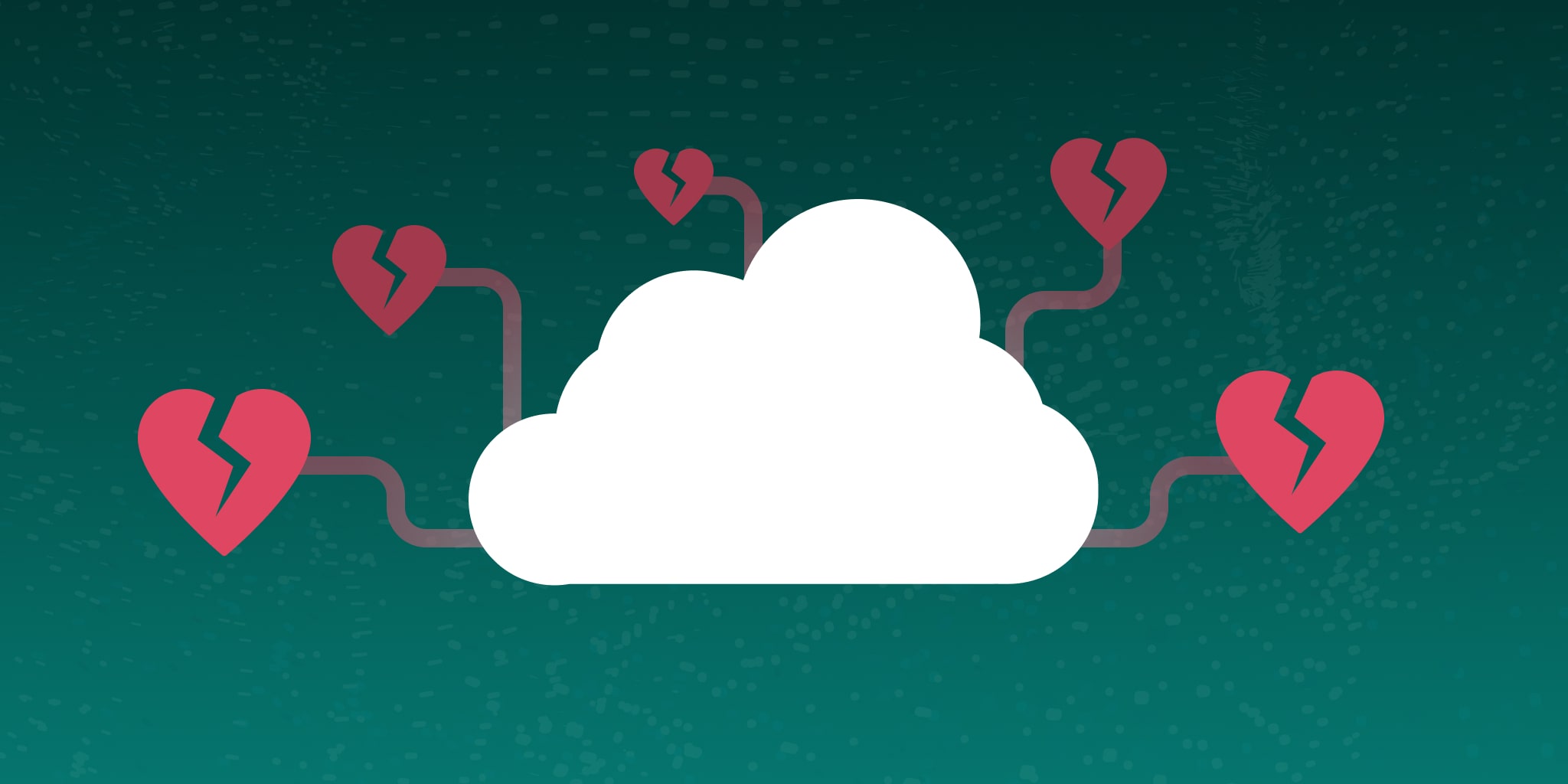
There are preconceived notions of what life will be like for someone when they switch to the Cloud. While there are many indisputable advantages, have your umbrella ready.
Why do I say this? In this blog post, I share five things I hear about what people hate about the Cloud.
1. Overall Cost of the Cloud
The most frequent conversation that occurs between AtScale and a customer are the unseen costs of the Cloud.
In the beginning, everyone thought that the original ease of the Cloud was both attractive and simple. Companies such as Salesforce, Google (Gmail), and others, made the move to the new system appear quite seamless. These companies based their applications on a consumption model that was easy to understand and did not have extreme hidden costs.
The hidden costs occur more regularly with data and analytics-based cloud options. The cost of running a data-driven company in the Cloud is similar to what one would have done when they ran Oracle database on-prem or AWS. The IT team would have to set up a system that would work uniquely with their data infrastructure, and the consumption pricing would eat into the savings of not maintaining their own data centers.
We have learned the hard way to move from a CAPEX system to an OPEX mode, which is more in line with subscription-based modeling. This model hit harder due to the peaks and valleys felt with the world of analytics and data science. The manipulation of data, as well as data scale and data locality, has created very unpredictable computed costs. And, people hate that.
2. Putting the System Together in the Cloud
In the early days of Tableau, it could be said that they were very focused on the experience of data analytics instead of the actual report. This overall experience that they created changed the business intelligence (BI) landscape for the next 20 years. Enterprises decided that there was supposed to be a premium on data discovery and knew what that meant to them.
However, the way they created this system was architecturally similar to Microsoft Excel. The systems were based on data extracts to the BI tools, and the BI tool then did their magic.
The technology shifted from on-prem medium/large-sized data to Big Data and ultimately the cloud. This does not work well with data extracts. On the one hand, these BI tools would give access to a few that could experience the data, while at the same time there was a massive increase in the amount of data and a change in the locality of data which meant they were not taking advantage of massive shifts. I speak to many customers who continue data extracts because “that’s what we have always done” and miss out on the opportunities to be found when you have access to all your data irregardless of where it lies (and it’s found on the cloud more and more these days).
So let me get a little technical here – AtScale allows and encourages BI tools to operate server-side. Meaning that calculations and Acceleration Structures (AtScale dynamic aggregates built with our Autonomous Data Engineering) prevent the need to extract record level data while still giving the BI developer the flexibility to design a powerful data consumer experience. Access to all the data while keeping it where it is – this is the key to cloud analytics.
3. Scanning Entire Data Sets
One could claim that scanning entire data sets is not a problem directly linked to the Cloud, but it is still centered around the same technological shift that we have seen in the recent years.
It was very possible five to ten years ago that you could scan an entire set of data and take advantage of all that was presented by one singular set. BI companies such as Cognos and MicroStrategy in contrast designed their systems using normalized data. By this, it could be said that the BI industry was able to break up the data in a way where one could do high-powered lookups or optimization of the data sets. Simple questions could be answered efficiently without altering other data sets.
However, a couple of things happened along the way. Data that sat too far away and/or were too extremely large; this is not exactly optimal. To combat this problem, there was a large effort to try and combine datasets together in order to unionize the numbers. This made sense; it minimizes the adhoc need to join data at run-time. As the scope of data became too large and too far, the new systems required a massive amount of ETL movement to try and denormalize the data so that the original processes could even begin to work on this new scale.
4. Cloud Era is IoT Era
Machine data can be viewed as a subset of all topics mentioned above. It is a problem that has been around since the tides turned towards Cloud and will continue to be around as we progress into the future. IoT produces a ton of machine data that is both wide and deep.
One of the companies I was visiting several years ago was a German car manufacturer. They said that each of their test cars had around 600 sensors and they had 20 drivers who drove each car all around the country. They would then proceed to collect data from the 600 sensors. If you think about it, that data table is minimum 600 columns wide for each car and a row for every millisecond. They were searching for a relationship between one part of the car to another, or, in other words, what data features impact another.
As this becomes the norm (and it is already) the challenge that it presents to the BI landscape goes back to data scale, data locality, data presentation and query performance. You have to address them all.
5. Cloud Migration Issues
The most obvious cloud migration issue is the amount of regulations that accompanied the Cloud. Depending on the type of data you view, this changes the location of which Cloud that data ends up in and who has jurisdiction to actually view the data once there.
To be fair, Cloud platforms are incredibly secure, probably more secure than they were on the original on-prem system. However, the cultural shift was still enough to rattle the thousands of people that hope to secure their data.
The other thing that is very important (that is not often addressed because it is not considered cool), is networking. By data migrating to the Cloud, you suffer from an impact on service level agreements. Report performance, collaboration, and remote computing (the home office) – all changes and all impacts your relationship with the network.
And don’t forget those typical database changes that keep everyone up at night. Data type mismatches, missing features (partitioning, indexes, etc), different SQL dialects – all gets compounded when moving to the cloud. Don’t forget the end-user. If they have to change their behavior because IT changes their data platforms, beware of “Adoption Barrier”.
While switching to the cloud is a viable and wise option given the way the present is progressing into the future. Go with your eyes wide open. Plan for some grey skies, and the sun will shine.
Related Reading:
SHARE
The Practical Guide to Using a Semantic Layer for Data & Analytics