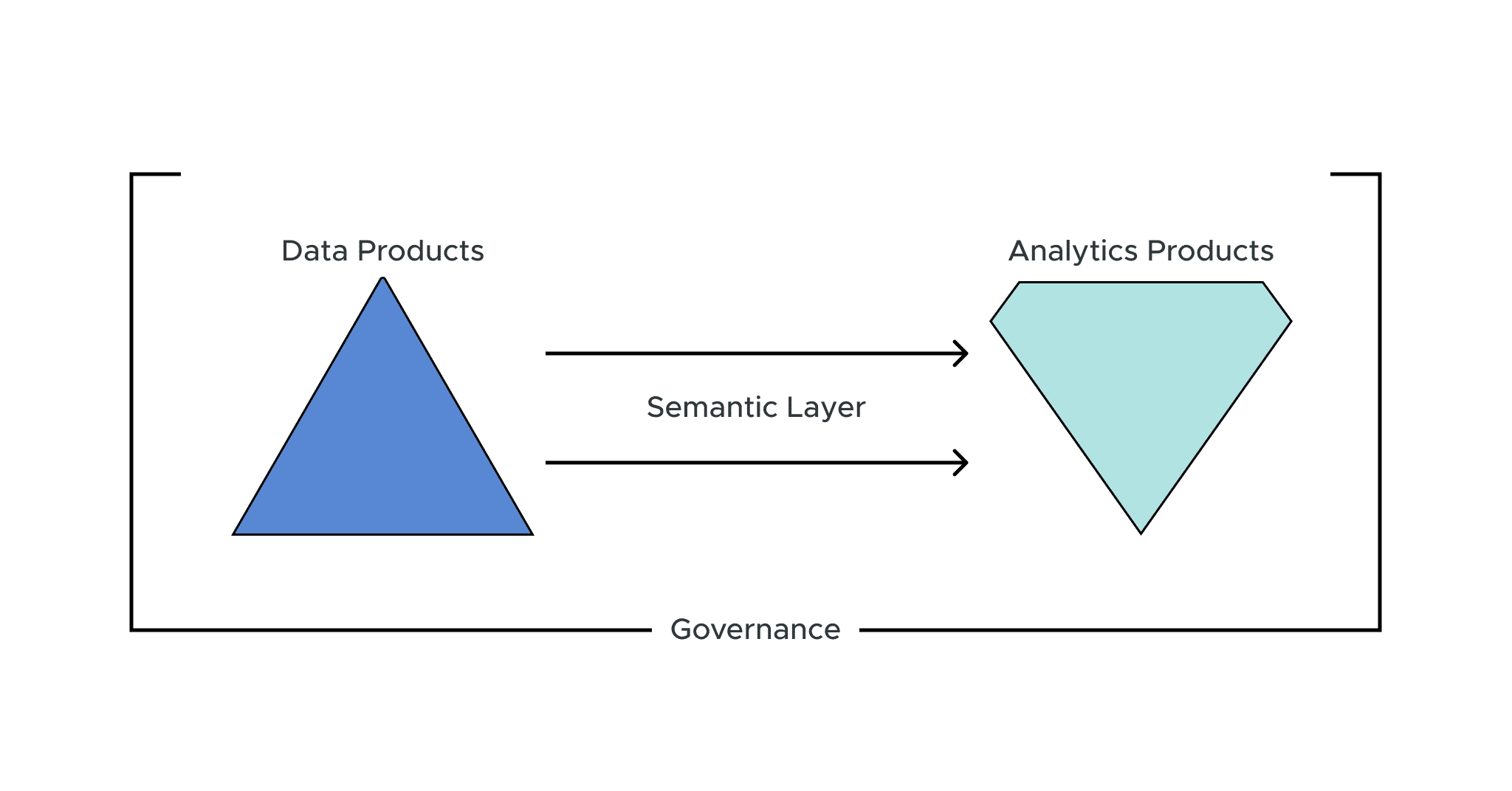
This post was written by Donald Farmer. Donald Farmer is a seasoned data and analytics strategist, with over 30 years of experience designing data and analytics products. He has led teams at Microsoft and Qlik Technologies and is now the Principal of TreeHive Strategy, where he advises software vendors, enterprises, and investors on data and advanced analytics strategy. In addition to his work at TreeHive, Donald serves as VP of Innovation and Research at Nobody Studios, a crowd-infused venture studio. Follow him on LinkedIn.
In the data analytics market, we love metaphors. They are one way for us to convey the hidden scale and complexity of our work to executives, business users and a wider public. Otherwise, they mostly don’t know and don’t care about the details of our technical world. So we layer on the imagery. Data is the lifeblood of modern business; it’s the new oil; it’s in a warehouse or in a lake; we deal with a mountain of data, a tsunami of data! But often, it is dirty or dark data that must be cleaned, lest rogue users working in shadow IT create havoc with it.
Amidst all this drama, it was refreshing when Zhamak Dehghani defined the principles of Data Mesh in 2019 and said simply that we can treat data as a product. In business, in economics, in daily life as consumers we understand products far better than we understand lakes, warehouses or oil. For IT, delivering data sets as products reinforces that quality, timeliness and usability are paramount, while insulating business users from the complexities of data preparation and management.
Since then Data Products have become a key component of modern data architecture for organizations implementing a Data Mesh approach. The paradigm continues to gain traction as organizations adopt its principles of domain-oriented decentralized ownership, treating data as a product, and computational governance to overcome persistent data management challenges.
Governing Usage
In my own experience, the most complex principle of Data Mesh is governance: “federated computational governance” as the architects like to say. But even when teams do a good job with this approach, we still find issues. Regulations like the GDPR in Europe and the CCPA in California demonstrate that we must govern not only data but also data analysis, ensuring that usage is compliant, auditable, and aligned with business objectives.
The California Consumer Privacy Act states: “A business shall not use a consumer’s personal information for any purpose other than those disclosed in the notice at collection.” Similarly, the GDPR mandates that data shall be “collected for specified, explicit, and legitimate purposes and not further processed in a manner that is incompatible with those purposes.” In other words, it’s not just the data that needs governance, but the use of the data. A data processor may have permission from a consumer to use their data for account management, but not for sales and marketing. We have all navigated through countless cookie permissions dialogs for exactly that reason.
In my world of business intelligence and data science the implication is clear: we need to govern analytics – the dashboards, apps, reports, visualizations and models – that often comprise the most impactful use of data.
Analytics Products
This evolution from governing data to governing analytics can be simplified with a new paradigm – an evolution from Data Products to Analytics Products.
Analytics Products encapsulate business logic and analytical capabilities, not just data, providing enhanced discoverability, reusability, and oversight of data usage. Paired with a governance model tailored for analytics, they simplify innovation of business-ready insights aligned with compliance requirements.
As Dave Mariani, CTO of AtScale, writes, the future of the modern data stack requires “a technology-agnostic semantic hub capable of serving all semantic models within an organization into a single, cohesive repository.” This hub combined with an analytics mesh of composable modules will enable the best of centralized governance and decentralized innovation.
The Components of Analytics Products
Naturally enough, because all Analytics Products are built over data, that data should be well-governed to start with. In the next layer – the Semantic Layer – we can begin to govern analytic artifacts, such as measures, calculation logic, attributes, dimensional hierarchies, business rules and so on.
An analytic product also needs to take account of access controls, however and wherever they are implemented. Managing user access is critical for security and privacy compliance. Most rules and controls regarding data and analytics access should apply directly to the platforms powering Analytics Products, providing oversight into who can use data and logic assets when and for what purposes. Integrating access controls also enables easier auditing. But sometimes, access controls depend on some aspects of analytics. For example, in a large organization, managers with only a small number of direct reports (or a small number of responses to a company survey) should not be able to see the results that could too easily identify individual employees. Meanwhile other managers at the same level in the security group, but with more reports or responses, may see the results. This is governance of the analysis, not the data.
A Shift in Focus
When we shift our perspective to view analytics artifacts as products, we share some of the advantages of Data Products. Data consumers rightly demand quality and usability and that requires that Analytics Products are fit for purpose, designed to meet those specific demands. In turn, this enables us to better govern usage and purpose limitations. The overly complex BI applications that were popular in the 2010s – all those tabs, panels, filters and linked visualizations! – give way to elegantly purposeful, focused applications that enable both the best principles of both design and governance.
A Semantic Layer is crucial for enabling Analytics Products as it serves as a bridge between complex data structures and users, improving performance where needed and masking technicalities with business-focused context. This abstraction allows users to interact with data using familiar terms and concepts, enhancing accessibility across the organization. A Semantic Layer enables standardized definitions that are authoritative (being sanctioned and shared) without constraining the innovative exploration of new ways of thinking.
Moreover, the Semantic Layer plays a vital role in governance and security by enforcing access controls at the point of usage. As business needs evolve, the Semantic Layer offers the agility to integrate new data sources and adapt to changes, ensuring that Analytics Products remain relevant and valuable.
Analytics Products delivered and governed by the Semantic Layer will prove to be an indispensable component in modern strategic data initiatives.
SHARE
WHITE PAPER