December 8, 2020
Five Ways to Improve Your Analytics ROI on Snowflake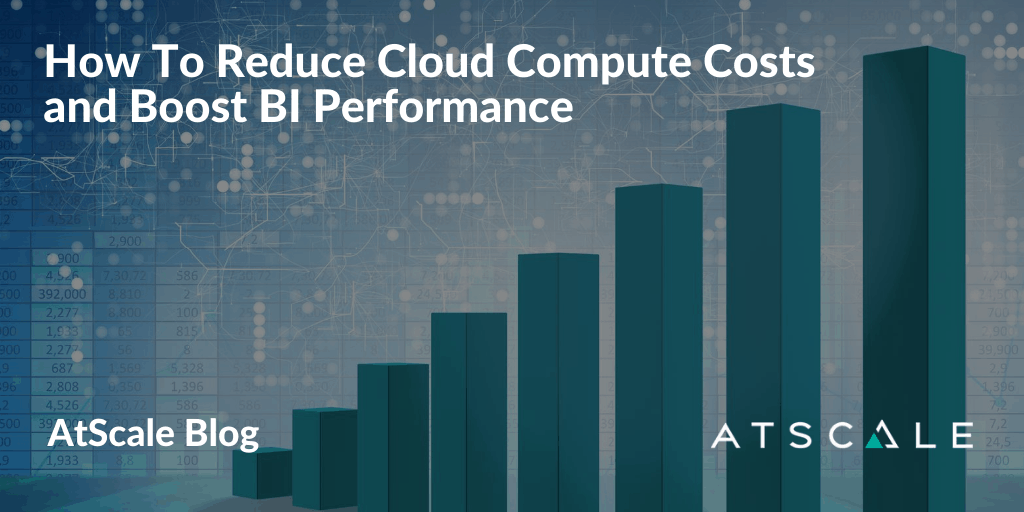
In our most recent webinar, Mark Stange Tregear, VP Analytics of Rakuten and Dave Mariani, Co-Founder and Chief Strategy Officer of AtScale share five tips to increase the ROI of your Snowflake investment while reducing compute costs.
Mark shares Rakuten Rewards’ journey to migrate to Snowflake. He recalls his team not being able to handle their workload which led them to begin working with parallel stacks. Stange-Tregear states, “For those of you that have tried to do that, you know that that comes some problems you have to replicate ETL code potentially, or your data ends up in silos. So you’ve got your marketing data in one place and your sales data in another place and your product data in another place. And your analysts and your business users start to find that to use data, the majority of the time is spent just trying to stitch the data back together and figure out where it went.” Stange-Tregear speaks to his team’s approach to solving this while keeping pace with their day-to-day needs as they turned to a Hadoop cluster. It was through working with Hadoop that led him to discovering AtScale.
As the data and organization began to grow, Mark and his team knew that it was time to start exploring the cloud. “We decided that by the time we looked at some of the core issues we were having and some of the core things we’re trying to solve, including can we load the data fast enough to get close to real-time data, can we hit SLAs, can we remove the issues with multiple concurrency that we’ve been getting on Hadoop; Snowflake presented the best solution,” says Stange-Tregear. Mark recalls the process of choosing a vendor that best suited the Rakuten Rewards team’s needs at the time. He was very pleased with the outcome, stating that “It worked so well that during 2019, we migrated our entire stack into the cloud and onto Snowflake.”
“I think the biggest single tip tipping point for us were the issues around concurrency.” Stange-Tregear continues, “With the Hadoop cluster, we could isolate CPU and isolate memory. We spent a lot of time trying to change jobs and trying to isolate workload, or even trying to block workloads so that it didn’t interfere with other things. And we had some success with that, but ultimately we even found that the hard drives couldn’t keep up. We were writing so much data to try and power real time, tight workloads, that we couldn’t get the data back off disk fast enough to service our end users.” As a result, Mark and his team didn’t get to spend their time doing business intelligence. Instead, they were debugging issues between the application and hardware lab.
“We realized pretty early, that more warehouses was going to be really helpful because what that meant we could do is we can isolate workloads,” says Stange-Tregear. He later speaks to having distinct warehouses for each team and how that prevented interruptions. The different teams gained warehouses of different sizes, but did not give them control over the size. Mark states “For any of you who have talked to some people who who’ve tried to handle Snowflake cost management in the past, you may have heard horror stories where, a company put whatever amount of money down, bought some Snowflake credits and then handed over control of warehousing sizes to their analysts or the data scientists and what happens? There’s a slow running query, or they really need to run through some heavy data or some heavy analysis. So they crank it up to an XL or two XL, three XL, etc. For those of you that don’t know the cost stretcher for Snowflake doubles for each increase in size. So, using a four XL is twice as expensive as a three XL. If you’ve got a very deliberate workload, you do it on purpose, and then you shut it and then you move it back down again. But if you allow teams to just scale that way houses, however they want, what happens over time as they move to four XLs to try and push through workload and they don’t tune them back down again. And now you’re paying a small fortune for a workload that could be executed vastly cheaper.”
To avoid this, Mark and his team created a centralized cost-management system where there are limits placed on query times.
What are you going to do with all of your warehouses? With the power of Tableau, Mark shares how he is able to identify the workloads and costs associated with his teams.
When looking at cloud data warehouse vendors who champion themselves for fast performance and infinite storage, “there’s always a catch,” says Dave Mariani. Mariani discussed four critical dimensions that you should consider when thinking about cloud data warehouses: Query Performance, User Concurrency, Compute Costs, and Semantic Complexity.” He shares that “the real key thing here is this a universal semantic layer,” and “with AtScale, you get a full multidimensional engine that makes your users life much easier to understand, and also gives them consistency when it comes to metrics and business definitions.”
In this demonstration, Dave compares the AtScale SQL and TPC-DS Raw queries and how AtScale can make the process 73% less expensive, while making your queries 76% less complex.
Related Reading:
Power BI/Fabric Benchmarks