June 16, 2022
The Future of Metrics Tooling, Open Source and Enterprise: Insights from Transform, Cube Dev, and AtScale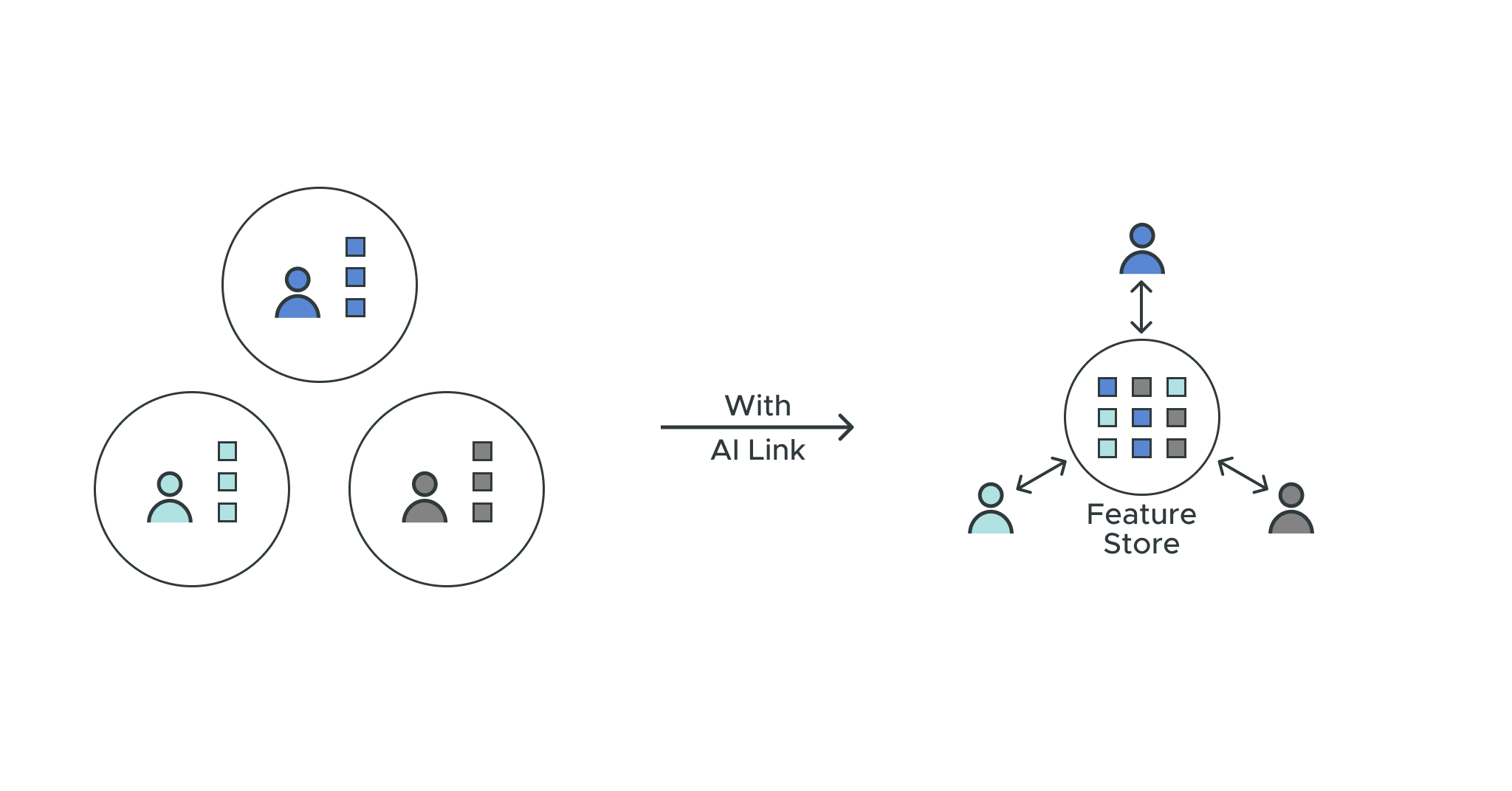
“We are drowning in information, but starved for knowledge.”
– John Naisbitt
The wheels of commerce are always churning, pushing merchants to find new opportunities, answers to questions, and the next breakthrough strategy to keep growing. One can imagine the tricky balance that a company has between its customers, its bottom line, and its competitive edge.
Companies are consistently looking for ways to innovate and learn something new to maintain product differentiation through analytics. Business analytics and data science has evolved as we know it today and dates back to the 1950s “with individual companies collecting their own offline data and manually analyzing it to look for ways to improve their operations.”¹ This early form of analysis was descriptive and diagnostic analytics done in batches, based on historical data and controlled by human interpretation. Leveraging petabytes of data and algorithms designed to mimic how our brains infer and interpret at lightning speed in real time, however, is something relatively new to companies grappling with their data. Artificial intelligence and machine learning (AI/ML) have become increasingly accessible as a result of the move to cloud and hybrid cloud, providing automation, predictions, and recommendations to help company leaders grapple these data and quench that thirst for knowledge.
As a result, companies are investing heavily in ML initiatives to create models that allow them to devise strategies for the future and to find ways to uncover more hidden gems among their data and existing processes.
Companies are trying to create supercharged cars for their leaders to more quickly get to their goals, answer customers, and beat out the competition. But before they can take it out in the world, they need to build the right car.
While access to ML modeling has improved dramatically over the past few years, there still remain some core challenges that prevent any sort of meaningful application of ML models from affecting business strategy:
- Misunderstanding: Data teams are often obfuscated from the root of the problem the business is trying to solve, and disagree on how to interpret it and correlate back to the core problem.
- Ongoing Time Investment: Support for ML initiatives can diminish as many of the core data science principles (data preparation, cleaning, exploration, etc.) take a long time, but don’t appear to produce anything meaningful for the business or even an ML model.
- Inconsistency: Many ML initiatives require multiple personas to offer input; disagreements about approach, needs, and toolkits among teams creates unreplicable processes, duplicative production, and wasted effort.
When it comes to successfully executing an ML initiative, a company really needs two things to solve these challenges:
A Universal Semantic Layer
An interface by which different data-driven personas are able to access raw data to collaboratively design data models. This allows for multi-dimensional analytical querying and serving ML models based on metrics that matter to the business. A semantic layer provides a single, common definition and representation of all data sources, metrics and models, enabling the rapid creation of consistent insights for AI and BI – with no data movement and no waiting. AtScale’s core value proposition is to provide this semantic layer to help companies’ data speak the language of their business.
A Feature Store:
These days, data scientists and data engineers face a growing number of requests they have to balance against prioritizing the needs of the business and establishing data pipelines that allow a data scientist to adhere to ethical standards for trusted and explainable AI. We often hear the 80/20 anecdote of data prep suggesting that data engineering activities represent a significantly larger amount of time than ML model creation, evaluation, and deployment.
The semantic layer provides a simple tool for teams to rapidly extract, explore, interpret, and transform elements of data or data models to serve the ML model. Features are the derivative calculations from those interpretations that ultimately influence the training and output from the ML model. However, feature engineering is still a time consuming process.
Feature engineering is a unique step in the ML workflow because it calls for contextual understanding provided by a business analyst, data preparation tactics from the data engineer, and statistical inference design from the data scientist. It takes a team to select, transform, and create the appropriate features needed to even start training a machine learning model.
Imagine, however, if a company had access to a centralized repository of these features for anyone to access and use in their ML initiatives. This would allow a company to operationalize ML initiatives based on standardized definition and dimensions across all facets of the business. This means everyone is using the same foundational layer to design their ML model. This is the core concept of a feature store and how it can benefit from the value of a semantic layer:
With a feature store data teams can:
Publish, store, and maintain features: Teams across all business units in a company can view, access, and reuse features. This means transparency among teams to resolve disagreements and faster time to ML model training.
Find and transform features: Teams can spend less time trying to figure out how to manipulate data from raw to model-ready. This means faster time to production-ready ML models.
Maintain governance and access controls: Teams need to maintain core compliance policies for their data when datasets are manipulated during the process of creating data models for ML models. This means fewer compliance breaches and legal battles when ML models go into production.
AtScale’s AI Link allows companies to create and manage a feature store within the semantic layer platform for all of the benefits above and:
- Python-native Capabilities: Allows data personas to tap into data models and downstream platforms using the most common programming in ML development.
- SME Collaboration: Encourages different teams to collaborate and work more efficiently together.
- Toolchain Maintenance: Allows data teams to continue leveraging their preferred upstream data sources and downstream analytics consumer platforms. A much smoother and repeatable process, without introducing a steep product learning curve.
At the end of the day, feature stores will constitute a core element of production-ready ML and its benefits will quickly manifest in any ML initiative a company wants to take on or scale. A feature store solves some of those key challenges mentioned above:
Misunderstanding is replaced with Collaboration
Data teams and their different users can access, review, and interpret the nuance of their company’s data.
Ongoing Time Investment is replaced with Accelerated Time to Value
Multiple business units leverage the same logic and help their data teams be more efficient in creating ML models.
Inconsistency is replaced with Consistency
Companies can maintain control of their existing toolchain and offer standardized features for all their business units to perform predictive analytics.
If an ML model is a supercharged car for a company to lead them in their quest for knowledge, a semantic layer is the garage where it gets built, and a feature store is the toolbox for the mechanics to perform their work.
See AtScale in action. Request a live demo to learn more.
- “The 4 eras of analytics: charting the evolution of data science,” Aggarwal, 2020
ANALYST REPORT